Copy Link
Add to Bookmark
Report
VISION-LIST Digest Volume 13 Issue 11
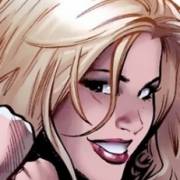
VISION-LIST Digest Mon Mar 07 12:54:06 PDT 94 Volume 13 : Issue 11
- ***** The Vision List host is TELEOS.COM *****
- Send submissions to Vision-List@TELEOS.COM
- Vision List Digest available via COMP.AI.VISION newsgroup
- If you don't have access to COMP.AI.VISION, request list
membership to Vision-List-Request@TELEOS.COM
- Access Vision List Archives via anonymous ftp to FTP.TELEOS.COM
Today's Topics:
A measure of symmetry?
software for detecting watershed boundaries
Eye images needed
In response to your query of "Science/Tech" conference
Looking for DARPA IU proceedings paper
Need open S/W for FAX image
Job Advert - Researcher in Automatic Geometric Model Acquisition
CFP: Vision Geometry III
Update: First IEEE International Conference on Image Processing
CFP: Computer Vision Related Conferences
ISIKNH'94 (Advance Program and Registration Information)
Extended Kalman Filter Initiaiization (long)
Tech reports from CBCL at MIT (long)
----------------------------------------------------------------------
Date: Tue, 1 Mar 94 14:51:51 MET
From: Jose Manuel Inesta Quereda <inesta@vents.uji.es>
Subject: A measure of symmetry?
Hi everyone.
I'm dealing with this problem, any suggestion would be appreciated:
Suppose you have a part of an adge expressed as a sequence of points (x,y
coordinates). How can I establish a measure of symmetry that is maximum for
a point such as P (in the next example)
P
**o**
*** *
* **
* *
* *
* *
As can be seen, I don't want a precise definition of symmetry, but a
property that becomes a maximum value for a given point in a part of
a digital curve.
Thank you for your attention.
Jose M. Inesta | e-mail: inesta@inf.uji.es
Departamento de Informatica | Tfn...: +34-64-345769 (8523)
Universitat Jaume I | Fax...: +34-64-345848
E-12071 Castellon (SPAIN) |
------------------------------
Date: Tue, 01 Mar 1994 12:11:17 +0100
From: G.POLDER@cpro.agro.nl
Subject: software for detecting watershed boundaries
Does anyone know where I can get a program (or code) that will
calculate watershed boundaries in an greyvallue image? Watershed
boundaries indentify the tops of ridges and bottoms of valleys in an
image. More information can be found in: Gauch, J.M. and Pizer, S.M.,
Multiresolution analysis of ridges and valleys in grey-scale images,
PAMI (15)6: June 1993, pp 635-646
Thanks,
Gerrit Polder.
g.polder@cpro.agro.nl
------------------------------
Date: Mon, 7 Mar 1994 00:17:33 GMT
From: cph@dmu.ac.uk (Chris Hand)
Organization: De Montfort University, Leicester, UK
Subject: Eye images needed
A student here is working on a project to perform software-based
eye-tracking. We're having great difficulty in tracking down some
reasonable-resolution images of *just* an eye (say 100x100 to 512x512).
The biggest problem with making our own images is that we don't seem
to have the right sort of lenses lying around to be able to get close
enough and still let some light in... :-(
If anyone has some images we could borrow, could they get in touch please.
Ideally we'd like some pictures where the eye is looking in different
directions, with a specular reflection visible somewhere (off the cornea).
Thanks.
Chris Hand, Lecturer | Dept of Computing Science,
Internet e-mail: cph@dmu.ac.uk | De Montfort University, The Gateway
"In Cyberspace nobody knows you're bald" | LEICESTER, UK LE1 9BH
WWW: <A HREF="http://www.cms.dmu.ac.uk:9999/People/cph/cphroot.html">cph</A>
------------------------------
Date: Fri, 4 Mar 1994 22:42:20 UTC+0100
From: TK0JUT2@mvs.cso.niu.edu
Subject: In response to your query of "Science/Tech" conference
>I need information about de Workshop "Science and Technology
> through Science Fic tion". When is the deadlinepapers. Thanks
> in advance.
>
> Vmctor Federico Herrero
> U.N.M.d.P (Argentina)
For information on the Science/Tech Through Science Fiction, see
CuD #5.54. We don't keep back issues here, but you can obtain
it via ftp at ftp.eff.org (anonymous logon) in the
pub/Publications/CuD directory.
Jim Thomas
------------------------------
Date: Fri, 04 Mar 94 11:42:48 EST
From: dutta@cognex.com
Subject: Looking for DARPA IU proceedings paper
Can anybody help me find this article. These proceedings seem to
be quite rare:
A.J. Heller and J.R. Stenstrom, "Verifcation of Recognition and
Alignment Hypothesis by Means of Edge Verification Statistics",
Proceedings of the DARPA IU Workshop, pp 957-966, Palo Alto CA, 1989.
If you have the paper I would be happy to pay you for the trouble of
copying and sending it to me.
Paul Dutta-Choudhury
Cognex Corp.
dutta@cognex.com
------------------------------
Date: Wed, 2 Mar 94 15:17:19 KST
From: faxdb@violet.kotel.co.kr (FAX-SVC [MGR])
Subject: Need open S/W for FAX image
Hi,
I'm finding an open software which displays and edits FAX images(G3 type)
on X window environment. In addition I need some conversion programs to
convert ps-to-FAX, FAX-to-ps, tiff-to-FAX and FAX-to-tiff .
Please let me know where I can get them.
Thanks in advance.....
Doo-man Yoon
Korea Telecom | Tel: +82-2-526-6758
S/W research Laboratories | FAX: +82-2-526-6785
HiTEL DB section. | E-mail:dmyoon@rcunix.kotel.co.kr
------------------------------
Date: Thu, 3 Mar 94 10:58:11 GMT
From: rbf@aifh.ed.ac.uk
Subject: Job Advert - Researcher in Automatic Geometric Model Acquisition
University of Edinburgh
Department of Artificial Intelligence
One Research Post - Computer Vision
Applications are invited for one researcher to work in the
Department of Artificial Intelligence on a SERC funded project entitled
"Acquisition of Surface-based Geometric Models for Object Manipulation and
Location". The researcher will investigate topics related to acquiring
geometric descriptions of mainly industrial objects from range data and
fusing descriptions from multiple views to make a coherent single model.
Applicants for the post should have a PhD (or a MSc plus suitable experience)
in an appropriate area, such as computer vision or image processing and
should have experience with the C or C++ programming languages.
The post is on the AR1A scale (12828 -- 18855 pounds/annum) and is
available immediately, with an end date of 15 October 1995.
Placement for the post is according to age, experience and qualifications.
Application by letter is invited, including a curriculum vitae (3 copies)
and the names and addresses of two referees. Applications should quote
reference 940090.
Further particulars from, and applications to:
Ms. Alison Fleming,
Department of Artificial Intelligence,
University of Edinburgh,
5 Forrest Hill,
Edinburgh,
EH1 2QL
Closing date for applications is 24 March 1994
------------------------------
Date: Tue, 1 Mar 1994 13:57:52 +0500
From: ykong%euclid@iris.cs.qc.edu (Yung Kong)
Subject: CFP: Vision Geometry III
CALL FOR PAPERS AND ANNOUNCEMENT
VISION GEOMETRY III
Boston, Massachusetts USA
Part of SPIE's BOSTON '94 Symposium
October 31 - November 4, 1994
Conference Chairs: Robert A. Melter, Long Island Univ.
Angela Y. Wu, The American Univ.
Program Committee:
A. Gross (CUNY/Queens Coll. & Columbia Univ.), T. Y. Kong (CUNY/Queens Coll.)
J. Koplowitz (Clarkson Univ.), D. M. Mount (Univ. of Maryland/College Park),
I. Stojmenovic (Univ. of Ottawa, Canada).
This conference is designed to bring together workers who use geometric theory
and techniques to solve problems related to computer vision. Specific
solutions as well as overviews of more general topics are welcome.
Topics of interest are:
Digital geometry and topology
Morphology related to vision
Computational geometry related to vision
Other vision-related geometry
Prospective contributors are invited to submit 250-word abstracts of their
papers, in accordance with the instructions below, by April 4, 1994.
Authors will be allowed 15 minutes plus a 5-minute discussion period for oral
presentation of a paper. Provision will also be made for poster presentation.
Contributors who prefer this method should so indicate on abstract submissions.
Proceedings of this meeting will be published by SPIE. Authors of accepted
abstracts will be notified by June 20, 1994, and will be required to submit
camera-ready manuscripts in English by October 3, 1994.
INSTRUCTIONS FOR SUBMISSION OF ABSTRACTS:
There are three ways to submit an abstract:
1. By e-mail to abstracts@mom.spie.org (ASCII format)
2. By fax (1 copy) to SPIE at 206 647 1445
3. By mail (4 copies) to:
Boston '94, SPIE, P.O. Box 10, Bellingham, WA 98227-0010 USA
Your abstract should include the following:
1. Abstract title.
2. Full names and affiliations of authors, with the principal author
listed first.
3. Mailing address, telephone number, fax number and e-mail address of
EACH author.
4. The following words: "SUBMIT TO: Vision Geometry III (Melter/Wu)"
5. Indication of whether you would prefer to present your paper orally or
as a poster.
6. Text of the abstract--250 words. This should contain enough detail
to clearly convey the approach and the results of the research.
7. 50-100 word biography of the principal author.
Abstract due date: April 4, 1994
(Late abstracts may be considered subject to program time availability
and chairs' approval.)
Manuscript due date: October 3, 1994
For further information, contact:
Robert A. Melter
Long Island University
Southampton, NY 11968
Tel. 516 283 4000
e-mail: melter@seaweed.liunet.edu
------------------------------
Date: Thu, 3 Mar 94 10:52:14 CST
From: icip@pine.ece.utexas.edu (International Conf on Image Processing Mail Box)
Subject: Update: First IEEE International Conference on Image Processing
FIRST IEEE INTERNATIONAL CONFERENCE ON IMAGE PROCESSING
November 13-16, 1994
Austin Convention Center, Austin, Texas, USA
[These are] current events involving ICIP-94:
First, we are developing an exciting program that will include:
4 Tutorials:
M. Vetterli & J. Kovacevic on Wavelets
B. Girod on Compression of Still and Moving Images
R. Haralick on Mathematical Morphology
R. Blahut on Imaging Systems
3 Plenary Talks:
Paul Lauterbaur, Univ. of Illinois at Urbana-Champaign
Peter Burt, Sarnoff Labs
Gary Starkweather, Apple Computer
6 Special Sessions:
Image Processing Education (R. Bamberger & J. Cozzens)
Signal Processing in Magnetic Resonance Imaging (Z. Liang)
Mathematical Morphology (D. Schonfeld & I. Pitas)
Nonlinear Dynamics in Image Processing (G. Sapiro & A. Tannenbaum)
Imaging Modalities (J. Quistgaard)
Electronic Imaging (C. Bouman and J. Allebach)
In addition there will be an exciting Product Exhibition featuring
over 25 booths displaying today's state-of-the-art commercial image
processing hardware, software, and accessories. This will be held in
the Exhibit Hall at the Austin Convention Center.
As of February 23, 1994 (today) we have recieved over 700 paper
submissions to ICIP-94 - attesting to the excitement that the
conference has engendered in the image processing community. And
certainly, presenting a challenge to the ICIP-94 Technical Program
Committee to conduct a timely review process!
Nevertheless, it is necessary to announce an EXTENDED DUE DATE for
further submissions to ICIP-94. This is made necessary because of an
error by IEEE Publishing - the ICIP Call for Papers was inadvertently
omitted from the recent issues of the IEEE Transactions on Image
Processing and the IEEE Transactions on Signal Processing. Since
these are our primary means of communicating with the members of
the IEEE Signal Processing Society, we felt it necessary to give
all SP Society members a chance to participate by advertising in
the next available issue - with an extended due date:
EXTENDED ICIP-94 SUBMISSION DEADLINE: MARCH 15, 1994.
Naturally, this applies to everyone. If you would like another copy
of the electronic version of the ICIP-94 Call for Papers, please let
us know at icip@pine.ece.utexas.edu with the message "CFP Please."
We look forward to seeing you in Austin!
THE ORGANIZING COMMITTEE
OF THE
FIRST IEEE INTERNATIONAL CONFERENCE ON IMAGE PROCESSING
November 13-16, 1994
Austin Convention Center, Austin, Texas, USA
------------------------------
Date: Fri, 4 Mar 1994 19:12:45 GMT
From: spie@henson.cc.wwu.edu (SPIE Staff)
Organization: Western Washington University
Subject: CFP: Computer Vision Related Conferences
Keywords: SPIE computer machine vision conferences robots
Announcement & Call for Papers
COMPUTER VISION RELATED CONFERENCES AT:
SPIE'S INTERNATIONAL SYMPOSIUM ON
PHOTONIC SENSORS & CONTROLS FOR COMMERCIAL APPLICATIONS
Part of SPIE's Photonics East
31 Oct. - 4 Nov. 1994
Hynes Convention Center
Boston, Massachusetts USA
CONTENTS
1.0 Abstract and Manuscript Due Dates
2.0 Computer Vision Related Conferences
3.0 Program Tracks
4.0 General Information
5.0 Submission of Abstracts
6.0 How to Receive More Information
1.0 ABSTRACT AND MANUSCRIPT DUE DATES
* Abstract Due Date: 4 April 1994
Late abstract submissions may be considered, subject to
program time availability and chair's approval.
* Manuscript Due Date: 3 October 1994 or 8 August 1994+
+ Proceedings will be available on site. Abstract and
manuscript due dates must be strictly observed.
2.0 COMPUTER VISION RELATED CONFERENCES
MACHINE VISION AND ROBOTIC APPLICATIONS IN GREENHOUSES AND
LIVESTOCK FACILITIES
Conference Chair: Peter P. Ling, Rutgers Univ.
---------------------------------------------
Machine Vision Applications, Architectures, and Systems
Integration III*
---------------------------------------------
Conference Chairs: Bruce G. Batchelor, Univ. of Wales College
Cardiff (UK); Susan Snell Solomon, CSPI; Frederick M. Waltz,
Univ. of Minnesota
Program Committee: Chris C. Bowman, Industrial Research Ltd. (New
Zealand); Donald W. Braggins Machine Vision Systems Consultancy
(UK); Aziz Chihoub, Siemens Corporate Research, Inc.; John W. V.
Miller, Univ. of Michigan; A. David Marshall, Univ. of Wales
College Cardiff (UK); Wolfgang Poelzleitner, Joanneum Research
(Austria); Paul F. Whelan, Dublin City Univ. (Ireland); Inna Y.
Zayas, USDA Agricultural Research Service
* Manuscript Due Date: 8 August 1994
Proceedings will be available at the meeting.
---------------------------------------------
Telemanipulator and Telepresence Technologies
---------------------------------------------
Conference Chair: Hari Das, Jet Propulsion Lab.
Program Committee: Bernard D. Adelstein, NASA Ames Research Ctr.;
Robert J. Anderson, Sandia National Labs.; Thomas P. Caudell,
Univ. of New Mexico; Janez Funda, IBM Thomas J. Watson Research
Ctr.; Blake Hannaford, Univ. of Washington; Won Soo Kim, Jet
Propulsion Lab.; James M. Manyika, Oxford Univ. (UK); Thomas B.
Sheridan, Man-Machine Systems Lab./MIT
Cosponsoring organization: IEEE NNC-Virtual Reality Technical
Committee
--------------------------------------------------------
Intelligent Robots and Computer Vision XIII: Algorithms,
Techniques, Active Vision, Materials Handling*
--------------------------------------------------------
Conference Chair: David P. Casasent, Carnegie Mellon Univ.
Cochair: Ernest L. Hall, Univ. of Cincinnati
Program Committee: Mongi A. Abidi, Univ. of Tennessee/Knoxville;
Rolf-Jurgen Ahlers, Rauschenberger Metallwaren GmbH (FRG); Bruce
G. Batchelor, Univ. of Wales College Cardiff (UK); Madan M.
Gupta, Univ. of Saskatchewan (Canada); Ian Horswill, AI Lab./MIT;
Sunanda Mitra, Texas Tech Univ.; Prasanna G. Mulgaonkar, SRI
International; Daniel Raviv, Florida Atlantic Univ.; Ellen M.
Reid, Motorola; Steven K. Rogers, Air Force Institute of
Technology; Juha Roning, Univ. of Oulu (Finland); Scott A.
Starks, Univ. of Texas/El Paso; M. A. Taalebinezhaad, Univ. Laval
(Canada); Hemant D. Tagare, Yale Univ.; Andrew K. C. Wong, Univ.
of Waterloo (Canada)
* Manuscript Due Date: 8 August 1994
Proceedings will be available at the meeting.
------------------
Sensor Fusion VII*
------------------
Conference Chair: Paul S. Schenker, Jet Propulsion Lab.
Program Committee: Terrance E. Boult, Columbia Univ.; Su-Shing
Chen, Univ. of North Carolina/Charlotte; David B. Cooper, Brown
Univ.; Gregory D. Hager, Yale Univ.; Martin Herman, National
Institute of Standards and Technology; Terrance L. Huntsberger,
Univ. of South Carolina; Ren C. Luo, North Carolina State Univ.;
James M. Manyika, Oxford Univ. (UK); Suresh B. Marapane, Univ. of
Tennessee/Knoxville; Gerard T. McKee, Univ. of Reading (UK);
Evangelos E. Milios, York Univ. (Canada); Robin R. Murphy,
Colorado School of Mines; Bobby S. Y. Rao, Univ. of
California/Berkeley; Michael Seibert, Lincoln Lab./MIT; W. Brent
Seales, Univ. of Kentucky; Charles V. Stewart, Rennselaer
Polytechnic Institute; Stelios C. A. Thomopoulos, The
Pennsylvania State Univ.
* Manuscript Due Date: 8 August 1994
Proceedings will be available at the meeting.
----------------
Mobile Robots IX
----------------
Conference Chairs: William J. Wolfe, Univ. of Colorado/Denver;
Wendell H. Chun, Martin Marietta Astronautics Group
Program Committee: Ronald C. Arkin, Georgia Institute of
Technology; David J. Braunegg, MITRE Corp.; David P. Casasent,
Carnegie Mellon Univ.; Douglas W. Gage, Naval Command Control and
Ocean Surveillance Ctr.; Surender K. Kenue, General Motors
Research and Environmental Staff; William Y. Lim, Grumman Corp.;
Bijan G. Mobasseri, Villanova Univ.; David W. Parish, Omnitech
Robotics, Inc.
-------------------
Vision Geometry III
-------------------
Conference Chairs: Robert A. Melter, Long Island Univ.; Angela Y.
Wu, The American Univ.
Program Committee: Ari Gross, CUNY/Queens College and Columbia
Univ.; T. Yung Kong, CUNY/Queens College; Jack Koplowitz,
Clarkson Univ.; David Mount, Univ. of Maryland/College Park; Ivan
Stojmenovic, Univ. of Ottawa (Canada)
3.0 PROGRAM TRACKS
Including those listed above, twenty-two conferences are
scheduled for this symposium. The conferences are organized into
five program tracks:
* Photonic Devices and Materials
* Smart Highways
* Agriculture, Forestry & Biological Processing
* Automated Inspection
* Intelligent Robots & Computer Vision
4.0 GENERAL INFORMATION
* Advance Technical Program
The comprehensive Advance Technical Program for this symposium
will list conferences, paper titles and authors in order of
presentation, education program schedule including course
descriptions and instructor biographies, and an outline of all
planned special events. Call SPIE at 206/676-3290 (Pacific Time),
or e-mail spie@mom.spie.org to request that a copy be sent to you
when it becomes available in July 1994.
* Hotel Accommodations
SPIE will be reserving rooms at discounted rates at several
hotels near the Hynes Convention Center for this symposium.
Meeting headquarters hotel will be The Sheraton Boston Hotel and
Towers. In order to book rooms at hotels offering the special
symposium rates, you will need to make these arrangements through
the Boston Housing Bureau. By calling hotels directly, you will
not receive the special symposium rates. Information about how
to make your hotel accommodation reservations will be included in
the Advance Program. Hotel rates are expected to range from $90-
$145.
* Registration
Registration fees for conferences and short courses, a
registration form, technical and general information for SPIE's
Boston Symposium & Exposition: Photonic Sensors and Controls for
Commercial Applications will be available in the Advance Program.
* How to exhibit
Companies interested in exhibiting at this symposium may contact
Sue Davis, Director of Conferences and Exhibits, at 503/663-1284,
or Diane Robinson, Exhibits Manager, at SPIE headquarters, phone
206/676-3290, fax 206/647-1445, e-mail diane@mom.spie.org.
5.0 SUBMISSION OF ABSTRACTS
Abstract Due Date: 4 April 1994
* Send abstract via E-mail to
abstracts@mom.spie.org (ASCII format);
* or fax one copy to SPIE at 206/647-1445;
* or mail four copies to:
Photonics East
SPIE, P.O. Box 10
Bellingham, WA 98227-0010
Shipping address:
1000 20th St.
Bellingham, WA 98225
Telephone 206/676-3290
Telex 46-7053
Anonymous FTP: mom.spie.org
E-mail: spie@mom.spie.org
CONDITIONS FOR ACCEPTANCE
* Authors are expected to secure travel and accommodation
funding, independent of SPIE, through their sponsoring
organizations before submitting abstracts.
* Only original material should be submitted.
* Commercial papers, descriptions of papers, with no research
content, and papers where supporting data or a technical
description cannot be given for proprietary reasons will not
be accepted for presentation in this symposium.
* Abstracts should contain enough detail to clearly convey the
approach and the results of the research.
* Government and company clearance to present and publish
should be final at the time of submittal.
* Applicants will be notified of acceptance by 20 June 1994.
Your abstract should include the following:
I. ABSTRACT TITLE
II. AUTHOR LISTING (principal author first)
Full names and affiliations.
III. CORRESPONDENCE FOR EACH AUTHOR
Mailing address, telephone, telefax, e-mail address.
IV. SUBMIT TO: (Conference Title) (Conference Chair)
at Photonic Sensors
V. PRESENTATION
Indicate your preference for "Oral Presentation" or "Poster
Presentation." Placement subject to chairs' discretion.
VI. ABSTRACT TEXT
250 words.
VII. BRIEF BIOGRAPHY (principal author only)
50 to 100 words.
* Paper Review
To assure a high quality conference, all abstracts and
Proceedings papers will be reviewed by the Conference Chairs for
technical merit and content.
* Proceedings of These Meetings
These meetings will result in published Proceedings that can be
ordered through the Advance Program. Manuscripts are required of
all accepted applicants and must be submitted in English by 8
August 1994 or 3 October 1994. Copyright to the manuscript is
expected to be released for publication in the conference
Proceedings. Note: If an author does not attend the meeting and
make a presentation, the chair may opt not to publish the
author's manuscript in the conference proceedings. Proceedings
papers are indexed in leading scientific databases including
INSPEC, Compendex Plus, Physics Abstracts, Chemical Abstracts,
International Aerospace Abstracts, and Index to Scientific and
Technical Proceedings.
* Publishing Policy
Manuscript due dates must be strictly observed. Whether the
Proceedings volume will be published before or after the meeting,
late manuscripts run the risk of not being published. The
objective of this policy is to better serve the conference
participants and the technical community at large. Your
cooperation in supporting this objective will be appreciated by
all.
* Chair/Author Benefits
Chairs/authors/co-authors are accorded a reduced-rate
registration fee. Included with fee payment are a copy of the
Proceedings in which the participant's role or paper appears, a
complimentary one-year nonvoting membership in SPIE (if never
before a member), and other special benefits.
* Poster Presentation
Interactive poster sessions will be scheduled. All conference
chairs encourage authors to contribute papers with technical
content that lends itself well to the poster format. Please
indicate your preference on the abstract.
* Oral Presentation
Each author is generally allowed 15 minutes plus a five-minute
discussion period. SPIE will provide the following media
equipment free of charge: 35 mm carousel slide projectors,
overhead projectors, electric pointers, and video equipment
(please give at least two weeks advance notice).
6.0 HOW TO RECEIVE MORE INFORMATION
The complete text of the printed announcement and call for papers
for Photonic Sensors & Controls for Commercial Applications is
available via anonymous FTP at:
mom.spie.org meetings/calls/sensors_controls.txt
It is also available through SPIE's automated e-mail server:
Send an e-mail message to,
info-optolink-request@mom.spie.org
with the following text in the message body:
send [optolink.meetings.programs]FILENAME.txt
To request a printed announcement and call for papers via e-mail
contact:
spie@mom.spie.org
For information regarding this meeting or other SPIE symposia or
publications, contact SPIE at:
P.O. Box 10
Bellingham, WA 98227-0010 USA
Telephone: 206/676-3290 (Pacific Time)
Telefax: 206/647-1445
Telex: 46-7053
E-mail: spie@mom.spie.org
Anonymous FTP: mom.spie.org.
This announcement and call for papers is based on commitments
received up to the time of publication and is subject to change
without notice.
SPIE is a nonprofit society dedicated to advancing engineering
and scientific applications of optical, electro-optical, and
optoelectronic instrumentation, systems and technology. Its
members are scientists, engineers, and users interested in the
reduction to practice of these technologies. SPIE provides the
means for communicating new developments and applications to the
scientific, engineering, and user communities through its
publications, symposia, and short courses.
SPIE is dedicated to bringing you quality electronic media and
online services.
------------------------------
Date: Mon, 28 Feb 94 12:57:32 -0500
From: fu@cis.ufl.edu
Subject: ISIKNH'94 (Advance Program and Registration Information)
ISIKNH'94 Conference Program:
(Sponsored by AAAI and University of Florida)
Time: May 9-10 1994; Place: Pensacola Beach, Florida, USA.
MAY 9, 1994:
Keynote Speech:
May 9, 9:00-9:45 a.m.
"Representation, Cognitive Architectures and Knowledge and Symbol Levels"
B. Chandrasekaran
Plenary Speech:
May 9, 10:00-10:45 a.m.
"Fuzzy Logic as a Basis for Knowledge Representation in Neural Networks"
Ronald R. Yager
Plenary Speech:
May 9, 11:00-11:45 a.m.
"Hybrid Models for Fuzzy Control"
Jim Bezdek
**** Lunch Break ****
Technical Session 1: (Integration Methodology I)
Chair: Armando F. da Rocha
May 9, 1:15-2:00 p.m.
``Integrating temporal symbolic knowledge and recurrent networks''
Christian W. Omlin, C. Lee Giles
``Implementing schemes and logics in connectionist models''
Ron Sun
``Integrating rules and neurocomputing for knowledge representation''
Ioannis Hatzilygeroudis
Technical Session 2: (Learning)
Chair: Ron Sun
May 9, 2:15-3:00 p.m.
``Symbolic knowledge from unsupervised learning''
Tharam S. Dillon, S. Sestito, M. Witten, M. Suing
``Genetically refining topologies of
knowledge-based neural networks''
David W. Opitz, Jude W. Shavlik
``On using decision tree as feature selector for feed-forward
neural networks''
Selwyn Piramuthu, Michael Shaw
**** Snack Break ****
Technical Session 3: (Fuzziness and Uncertainty)
Chair: Lee Giles
May 9, 3:30-4:15 p.m.
``Modifying network architectures for certainty-factor
rule-base revision''
Jeffrey Mahoney, Raymond Mooney
``Learning EMYCIN semantics''
K.D. Nguyen, R.C. Lacher
``Special fuzzy relational methods for the recognition of speech
with neural networks''
Carlos A. Reyes, Wyllis Bandler
Technical Session 4: (Integration Methodology II)
Chair: Ron Sun
May 9, 4:30-5:30 p.m.
``Learning knowledge and strategy of a generic neuro-expert system model''
Rajiv Khosla, T. Dillon
``Integrating symbolic and neural methods for building intelligent systems''
Ricardo Jose Machado, Armando Freitas da Rocha
``Modular integration of connectionist and symbolic processing
in knowledge-based systems''
Melanie Hilario
``Symbolic computation with monotonic maps of the interval''
Ron Bartlett, Max Garzon
Poster Session:
May 9, 1:30-4:30 p.m.
``The KoDiag system Case-based diagnosis with Kohonen networks''
Jurgen Rahmel, A. von Wangenheim
``Deriving conjunctive classification rules from neural networks''
Chris Nikolopoulos
``Generalization and fault tolerance in rule-based neural networks''
Hyeoncheol Kim, L. Fu
``Low level feature extraction and hidden layer neural network training''
T. Windeatt, R.G. Tebbs
``Sleeping staging by expert networks''
Hui-Huang Hsu, L. Fu, J. Principe
``Comparison of neural network and symbolic approaches
for predicting electricity generation requirements''
Terry Janssen, Eric Bleodorn, Ron Capone, Sue Kimbrough
``Reconciling connectionism with symbolism''
Roman Pozarlik
MAY 10, 1994:
Registration: 7:30-11:00a.m.
Plenary Speech:
May 10, 9:00-9:45 a.m.
"Teaching the Multiplication Tables to a Neural Network: Flexibility vs. Accuracy"
James Anderson
Plenary Speech:
May 10, 10:00-10:45 a.m.
"Words and Weights: What the Network's Parameters
Tell the Network's Programmers"
Steve Gallant
########################################################################
Panel Discussions:
May 10, 11:00 a.m. - 12:20 p.m.
"The Future Direction of AI"
Chair: Chris Lacher
Panelists: James Anderson, Steve Gallant, Ronald Yager, Ron Sun,
Lawrence Bookman.
########################################################################
**** Lunch Break ****
Technical Session 5: (Application Methodology I--Finance and Medicine)
Chair: Sylvian R. Ray
May 10, 1:30-2:15 p.m.
``Building a knowledge base from on-line corpora''
Lawrence A. Bookman
``Multivariate prediction using prior knowledge and
neural heuristics''
Kazuhiro Kohara, Tsutomu Ishikawa
``Applying artificial neural networks to medical knowledge domain''
Harry Burke, Philip Goodman, David Rosen
Technical Session 6: (Application Methodology II--Engineering)
Chair: Lawrence Bookman
May 10, 2:30-3:15 p.m.
``Integrating knowledge from multichannel signals''
Sylvian R. Ray
``Using partitioned neural nets and heuristics for
optical character recognition''
Kai Bolik, Steven Shoemaker, Divyendu Sinha, Miriam Tausner
``An expert network approach for material selection''
Vivek Goel, Jianhua Chen
**** Snack Break ****
Technical Session 7: (Language, Psychology, and Cognitive Science)
Chair: Steven Walczak
May 10, 3:45-4:30 p.m.
``From biological learning to machine learning''
Iver H. Iversen
``RAAMs that can learn to encode words from
a continuous stream of letters''
Kenneth A. Hester, Michael Bringmannm,
David Langan, Marino Niccolai, William Nowack
``The psychology of associative and symbolic reasoning''
Steven Sloman
Technical Session 8: (Integration Methodology III)
Chair: Ioannis Hatzilygeroudis
May 10, 4:45-5:30 p.m.
``Situation awareness assessments as a means of defining
learning tasks for neural networks''
Thomas English
``Integrating neural networks and expert systems for
intelligent resource allocation in academic admissions''
Steven Walczak
``Rule constraint and game playing heuristic embedded
into a feed forward neural network''
Walter H. Johnson
*******
Wrap-Up
*******
Please send your registration including a registration fee to:
Rob Francis
ISIKNH'94
DOCE/Conferences
2209 NW 13th Street, STE E
University of Florida
Gainesville, FL 32609-3476
USA
(Phone: 904-392-1701; fax: 904-392-6950)
[Registration fee: $250 by April 8, $300 on site, $150 for students]
For registration, please submit the following
information to the above address:
NAME: _______________________________________
ADDRESS: ____________________________________
INSTITUTION/COMPANY: ________________________
PHONE: ______________________________________
FAX: ________________________________________
E-MAIL: _____________________________________
------------------------------
Date: 6 Mar 1994 20:37:48 GMT
From: hansen@judy.eng.uci.edu (Jon M. Hansen)
Organization: University of California, Irvine
Subject: Extended Kalman Filter Initiaiization (long)
I recently posted the following:
> I am attempting to use an Extended Kalman Filter to simultaneously
> estimate the state variables (glucose, cell, ethanol, invertase) and
> model parameters for a fed-batch fermentation process. I am having
> trouble choosing the proper initial conditions and covariance matrices
> for convergence.
>
> From what I have read so far, it seems as though there is no general
> rule for initialization. In fact, some articles mention that the
> covariance matrices were determined by trial and error. Can anyone
> recommend a good article or reference which discusses the initialization
> of Extended Kalman Filters? I do not have a lot of time to get this
> working. Any additional comments or suggestions will be greatly
> appreciated.
Below is a summary of the replies I received. The comments and
references were very helpful. My filter is working for the
estimation problem without parameter estimation. Because most of
the measurements must be determined off-line, I am also faced with
the problem of few data points (less than 25). Any advice on this and
solving the parameter estimation problem would be greatly appreciated.
Thanks,
Jon
_/ _/_/_/_/ _/ _/ Jon M. Hansen, hansen@judy.eng.uci.edu
_/ _/ _/ _/_/ _/ Department of Chemical and
_/ _/ _/ _/ _/ _/_/ Biochemical Engineering
_/_/_/ _/_/_/_/ _/ _/ University of California, Irvine
Status: R
In theory, you can estimate the initial conditions, although technically
the estimates are not consistent (i.e. when the sample size goes to
infinity the variance doesn't go to zero). But this requires a
specialized program.
I believe it's fairly standard to plug the unconditional mean and
variance from the end of the run back into the beginning of the next
run. Repeat until convergence.... Another way would be to "back forecast"
the initial conditions from the first couple of observations, using
coefficient estimates from a previous run. But getting the variance
is probably hard.
Clint Cummins
If, by "covariance matrices" you mean measurement-noise covariance and
process-noise covariance, then there are a couple of papers by Barbara
La Scala, Bob Bitmead and Matt James which may be of use.
Let me know and I'll get Barbara to send you copies if you're interested.
Her e-mail address is "bls101@syseng.anu.edu.au"
As for selection of an initial value for the _state_error_ "covariance", that's
a different kettle of fish. This quantity is only an approximate covariance
in the EKF.
Hi Jon,
I believe you are looking for information on how to initialize an EKF.
I've been looking at the performance of the EKF as a nonlinear observer as
part of my PhD work. I have written a paper giving sufficient conditions
for the stability of the EKF when the system has linear output and bounded
noise and another paper discussing how to tune an EKF so that it tracks the
time-varying frequency of a sinusoidal signal. Would you like copies of
these and if so in what format? (I've written them in LaTeX but can send
you Postscript versions if you prefer).
Basically what I've concluded (which would hold for the case of nonlinear
state and measurement equations) is that the initial choice of the
covariance matrix of the state estimates (P(0) in my notation) is not
crucial to the stability of the EKF. I just set it to the identity matrix
and ignore it. This is because P is only a first order approximation to
the covariance of the state estimates and is probably an underestimate.
What is crucial is that this matrix doesn't get to be too small (ie smaller
than the true covariance value). If this is the case the system becomes
too confident of poor estimates and stops paying attention to the data and
so the EKF estimates get worse and worse. You want to monitor the size of
this matrix. You can ensure that it doesn't get too small by sensible
choices of the noise covariance matrices.
The crucial factors for good performance are :-
1) good initial state estimates;
2) a large enough measurement noise covariance matrix, (R in my
notation); and
3) a large enough state noise covariance matrix, (Q in my notation).
In my work I argue that it is important to regard these two matrices purely
as tuning parameters and not as estimates of the true covariance matrices.
I show that if you set these matrices to their true values you don't get
good performance because in practice they need to account for not only the
noise effects but also the effects of errors introduced due to linearizing
the system to construct the EKF. I show that (at least for the case of a
frequency tracker but it should hold in general) that it is disastrous to
make this values too small but the penalty for making them too large is
relatively small.
The stability result I've come up with is only a sufficiency theorem and
so it is fairly conservative. However it does give you 4 "stability
parameters" which are functions of R and Q which specify the maximum size
of the errors in the system (the EKF errors don't tend to zero but will
remain bounded) and the largest initial error for which the EKF will be
stable. They can give you an idea of good choices of Q and R to maximize
the region over which your EKF will be stable (they tend to be highly
nonlinear functions of Q and R so they aren't easy to analyse but at least
they're something).
Basically you need some idea of the magnitude of the true Q and R values
and then use trial and error.
Hope this helps
Barbara
Hi Jon,
In two other e-mails I've sent you compressed, uuencoded versions of my
two papers. Another paper you might want to take a look at is:
"Nonlinear and Adaptive Control in Biotechnology: A Tutorial" by G. Bastin
from the Proceedings of the European Control Conference, Grenoble, France,
July 1991, pages 2001-2012. It mentions using the EKF as a state observer.
The list of references at the end may also be of use to you.
I see you are planning to use the EKF to estimate model parameters as
well as the state of your system. It is known that using the EKF to
estimate model parameters of a linear system as well as the states produces
biased estimates. A paper discussing this and ways around it is
"Asymptotic Behaviour of the EKF as a Parameter Estimator for Linear
Systems" by Lennart Ljung in the IEEE Transactions on Automatic Control,
Vol 24, No 1, Feb 1979, pages 36-50.
Thank you very much for the papers, they printed fine. I will look at
the papers which you referenced above. I increased the size of my
Q and R matrices and chose different P matrices and have had much
better results than before. Your advice was very good. I think that
it may work well after some additional trial and error. You mentioned
that biased estimates will result from the parameter estimation, which
I will investigate this further. I believe that an EKF will still
perform better than method I used before, especially if I apply an
iterative back-calculation of the initial conditions so that the
initial condition error no longer has a significant affect on the
parameters at the end of each stage. I will keep you informed on
my progress. Thanks again,
Jon
To bls101@syseng.anu.edu.au Tue Mar 1 15:40:53 1994
I have successfully applied a linear KF to a spring-mass-damper
problem and applied an EKF to a simple fermentation problem. When
the same model parameters were used to generate my data as in the
EKF, the fluctuations of the estimates was the smallest when Q was
set to a zero matrix. You also observed large fluctuations in the
estimates when Q was large and the slew rate was low. Thus, the
proper selection of Q depends on how well the model describes the
process. I am still working on the parameter estimation problem.
Perhaps the value of the Q matrix can be lower when the parameters
are allowed to vary, assuming that the filter converges and the
potential bias in the parameters from the 'true' values is low.
want me to post your e-mail address with your reply.
Hi Jon,
glad to know that your work is going OK. One thing to note is that with
the KF (and the EKF) it is important that Q be non-zero. If it is zero the
filter will be unstable and eventually blow up. Very, very small is OK but
zero is a no-no.
Try posting to sci.image.processing, or send it to the moderator
of comp.ai.vision. Several shape-from-shading techniques use
Extended Kalman Filters, so someone should be able to help.
One source to look at for Extended Kalman Filters is:
@ARTICLE{AYACHE89,
author = "N. Ayache and O.D. Faugeras",
title = "Maintaining representations of the environment of
a mobile robot",
journal = "IEEE Transactions on Robotics and Automation",
volume = 5,
pages = "804-819",
year = 1989
}
In front of me I have a technical report ("Depth from Photomotion",
by R.Zhang, P. Tsai, and M. Shah, CS-TR-93-04, UCF), which says
this:
"Our formulation is suitable for the Extended Kalman Filter.
The basic process of the Kalman filter is as follows: a set
of measurements of a fixed number of parameters are taken
as input to estimate a number of unknown parameters, based
on how good the current measurements are, and how accurate
the current estimations are. The estimations from the previous
iteration are used together with the new measurements in the
current iteration in order to gradually refine the new estimates.
A major advantage of the Kalman filter is that it can be started
at any point, stopped at any point, and continued at any time."
In the technical report, Zhang et al. set the 1x1 covariance
matrix S to 1 to indicate a poor initial guess, but it seems
as though they could have set it to anything they pleased. They
use an iterative method with the Extended Kalman Filter, however,
which updates both the covariance matrix and the Kalman gain
on each iteration, so the choice of covariance matrix
will be updated.
I have a great interesting in your posting.
I am a student in biology, I had some experience using Kalman
Filter(not extended) in the analysis a population dynamic. In some
initialization conditions, the maximum likelihood function does not
exist, especially for several state variables (I did not try the
least square technique). Sometimes, I must try lots of times to set
the initial values to find the solution when I use the constrain or
non-constrain optimalization method.
Based on my reading in linear filter, I think you are correct that
there is no general rule for initialization, somebody mentioned
arbitral setting the state variables and setting bigger values
about covariance matrix, and let Kalman Filter to choose a suitable
step (you certainly can visualize to choose a appropriate step).
The only thing hunted me is that the performance of the linear
filter is not always well. I will involve this problem again,
because I think this is a great technique that has great potential
using in Ecology.
My model actually is a nonlinear model, I will try to use the
nonlinear model to do the estimation (Maybe considering the least
square technique), I find some papers using Extended Kalman filter
in Chemical area(Biotechnology and Bioengineering, Vol 41, p55-66
1993), There are still some points unclear for me, I need to
contact this person in Shanghai to know that their noise matrix
estimation process.
I am also very interested in any application of the Extended Kalman
Filter, can you provide more information like the reference and the
main points of the response you get in the net. I appreciate your
help very much because it is difficult for me to find help in this
area.
I read your post and have used Kalman filters on occasion.
Regarding initialization, the initial covariance represents prior
knowledge about the state vector. In an extended Kalman Filter
this is pretty much the same, you shout be able to map from
your prior knowledge about a quantity to your states. It is
important not to put in erroneous prior knowledge, in general
infinite covariance elements are OK since after the first
measurement you process the covariances represent the bounds
of error of the first measurement. I do position estimation
and an infinite variance allows the first measurement to essentially
initialize the Kalman for you. It may take a couple measurements
to get the covariance set up if you model derivative states etc.
Regrettably I cannot help you with your problem, but I was hoping that you
wouldn't mind mailing to me a list of references you get for your request,
and if possible also those you refer to in your post.
I have the exact same problem, but I find that I can solve it by working
iteratively, using both the kalman filter and the fixed-interval smoothing
algorithm. With respect to the state vector, some of my series are observed,
so I have no problem setting them - the unobserved series I can make good
guesses about, I run the filter and get my parameter estimates, then
run the smoothing algorithm. If you have a large number of observations, it
is usually pretty obvious what sort of correction is needed in the initial
t=0 estimates. (eg the estimated series moves wildly for the first two or so
next t's and then setttles into what seems to be a trend, so just ignore the
estiamtes from the first few steps and extrapolate backwords). Then I start
over, using my corrected initial conditions, fitting the parameters, and
smoothing the series again to get a new corrected set of initial conditions.
After doing this a couple times, the inputed initial conditions and the
outputed smoothed t=0 estimates are the same. I usually don't worry about
the initial variance covariance matrix too much (at least in my situation,
I did some simulations and found that I usually got the same results
as long as I had something reasonaable along the diagonals), but the fixed-
interval smoothing algorithm also gives you an estimate of it, so you can
do the same iteration as I described above. However, I have tried this same
method on a smaller dataset (only 80 obs) and I couldn;t get the estimates
to settle down. Let me know what you find out about this, I am also very
curious to know what the rigorous way to do it is.
Good luck, Mike Anderson mwande@qal.berkeley.edu
Hi Mike,
On Monday you responded to my post regarding Extended Kalman Filter
initialization.
Your comments are greatly appreciated. I can summarize the responses to
my posting as follows:
The crucial factors for good performance are :
1) good initial state estimates;
2) a large enough measurement noise covariance matrix, (R in my
notation); and
3) a large enough state noise covariance matrix, (Q in my notation).
By setting R and Q large enough, the initial state error covariance matrix
(P in my notation) can be determined by trial and error (unfortunately
I still have not seen a systematic approach). Several people mentioned that
the initial state estimate can be back calculated, as you did above. I am
interested in learning more about the fixed-interval smoothing method
which you are using to obtain your updated initial conditions. Because
some of my measurements must be determined off-line, the number of
observations is quite low (less than 20 observations). You mentioned that
you had problems with 80 observations, so any advice you can offer would
be greatly appreciated. Someone mentioned to me that they fabricated data
using a linear interpolation between data points. I believe that this
would result in poor parameter estimates although convergence may be
facilitated. For my problem, the parameter estimation is most important.
The fixed interval smoothing algorithm is given on page 154 (section 3.6.2)
of Harvey's book, "Forecasting structural time series models and the kalman
filter."
Mike Anderson
I would be interested in knowing of any responses that you got by e-mail.
Actually I am working on a similar problem . What I did was to use the
covariance matrices as "tuning knobs" for the estimator. However, I
believe Kwakernaak and Sivan's book on Optimal control does talk
about how to designing these matrices for the basic Kalman filter. As
far as the extended version of the Kalman filter is concerned, it is
a difficult proposition as sometimes time varying parameters are involved
and you need to know apriori how these parameters evolve .(i.e some info.
on the density function is required).
I am sure that , for the problem you mention, you must have looked at
the four papers by Stephanopoulos and San that appeared in Biotech. and Bioeng
in 1984. If you need the complete refs. please let me know. Also I
would myself be very keen to know the responses that you got from other
nettors.
I'm not sure if you can use an EKF to estimate model parameters
as well as states, but to initialise for state estimation,
you could use least squares over the first few data points.
A small discussion of initialisation is in Bar-Shalom and Fortmann's
book on "tracking and data association".
There is some discussion of this is our paper on time series of
continuous proportions: Grunwald, Raftery and Guttorp (1993) JRSS B 55,
103-116 (see p.109.
Adrian Raftery
After my (quite limited) knowledge about Extended Kalman Filters, they are used for estimating the state simultaneously with the disturbances. That means, a model for the disturbances is added to the model of the system and an estimator is constructed for> this "extended" system. Now, as I understand from your post, you are trying to estimate some parameters of your model and thsi, in my opinion, does not fit very well with the aim of the Extended Kalman Filter.
If nobody, more knowledgeable, shows up, tell me and I will look for references on the Extended Kalman Filter, although it seems as though you need beter an adaptive scheme.
Excuse me if I misunderstood your problem. Good luck,
Dear Martin,
The term 'extended'is used here to denote the linearization of the
original nonlinear dynamic system model for the application of the
standard discrete linear Kalman Filter (KF). The EKF can be used
for state estimation as well as parameter identification by defining
additional state variables for the model parameters which are to be
estimated. This technique has been applied to chemical reactors
(and fermentors) and many articles are available. However, the
selection of the initial covariance matrices (filter error, process
noise, and measurement noise) and the initial state vector is
critical for convergence. This subject is not discussed much
in the literature that I have read. If I have some convergence I
can back calculate the initial state vector, however, I am still
having trouble with the covariance matrices.
Thank you very much for your comments.
Jon
As I was presuming, I confused the term. Well, there is no standard
terminology. As to your problem (that I understand better now), this
is the kind of information that articles do not contain. It seems to
be a problem of previous experience and some inspiration. A more
practical advice: why don't you post your question on
sci.engr.control. It is a more appropriate group. You see, there are
not so many control engineers in math departments (like myself), and
when they are, they are surely not the most qualified to answer this
type of questions.
I hope that helps. Best luck.
Martin.
O-Matrix includes functions for performing Kalman filters with
discussion of the technique. Please respond if you would like
more information.
>
> O-Matrix is an object-oriented analysis and visualization tool that
> uses a simple interpreted language that operates on matrices instead
> of individual numbers. Operations on variables are performed in a matrix
> context.
>
> Features:
> - Diverse set of built-in functions including:
> Algebraic and trigonometric functions, interpolation, FFTs,
> inverse FFTs and 2D FFTs, QR factorization, Bessel functions,
> singular value decomposition, sorting, random number generation
> - Comprehensive graphics including:
> x-y plots with multiple viewports,contour plots, mesh plots,
> polar plotting, histograms, bar plots, stair plots,
> mouse manipulation of plotted data, and publication quality output
> - Built in debugger with extensive error messages
> - Extensive on-line help
> - 32-bit implementation allows matrices and programs up to 32 MB.
> - Extensive library of functions including:
> wavelets ,spectral analysis, Kalman filter, normal distributions,
> circuit simulator, circuit optimizer,
> splines, optimization, two-dimensional integration, convolution,
> auto-regressive correlation, special functions, Cholesky factorization,
> spectral estimation, differential equation solvers,
> F-test, t-test, means, medians, eigenvalue solver,
> matrix exponentiation, discrete prolate spheroidal sequences ...
> - User-definable functions with variable numbers of arguments and recursion
> - Six matrix types including character, integer, real, double-precision,
> logical, and complex double-precision
>
> If you have further questions, or wish to order O-Matrix for only $95.00
> please contact us at:
> Harmonic Software
> 12223 Dayton Avenue North, Seattle, WA 98133-8141
> (206) 367-8742
> fax (206) 367-1067
> harmonic@world.std.com
> (VISA and MasterCard orders welcome)
Organization: Control Enterprises, Inc.
Return-Receipt-To: "Charles Isdale" <CHARLES@cei.com>
Bart Kosko, a professor at USC, claims to have a more robust Kalman
Filter technique utilizing Fuzzy Logic and Neural Net Back
Propagation. Though I haven't seen his book, a reference that I have
indicates that this is discussed in Chapter 11 of his book (Neural Nets
and Fuzzy Systems, Englewood Cliffs, N.J., Prentice-Hall, 1991).
He states that had his method been used for aiming the Patriot
Missles, no one would have been killed in Israel by the Scud
Missles during Desert Storm.
_/ _/_/_/_/ _/ _/ Jon M. Hansen, hansen@judy.eng.uci.edu
_/ _/ _/ _/_/ _/ Department of Chemical and
_/ _/ _/ _/ _/ _/_/ Biochemical Engineering
_/_/_/ _/_/_/_/ _/ _/ University of California, Irvine
------------------------------
From: reza@ai.mit.edu (Reza Shadmehr)
Date: Tue, 1 Mar 94 16:48:51 EST
Subject: Tech reports from CBCL at MIT (long)
Would you please post the following note on the vision list?
Thanks. Reza Shadmehr
Hello,
Following is a list of technical reports from the Center for
Biological and Computational Learning at M.I.T. These reports are
available via anonymous ftp. (see end of this message for details)
:CBCL Paper #78/AI Memo #1405
:author Amnon Shashua
:title On Geometric and Algebraic Aspects of 3D Affine and Projective
Structures from Perspective 2D Views
:date July 1993
:pages 14
:keywords visual recognition, structure from motion, projective
geometry, 3D reconstruction
We investigate the differences --- conceptually and algorithmically
--- between affine and projective frameworks for the tasks of visual
recognition and reconstruction from perspective views. It is shown
that an affine invariant exists between any view and a fixed view
chosen as a reference view. This implies that for tasks for which a
reference view can be chosen, such as in alignment schemes for visual
recognition, projective invariants are not really necessary. We then
use the affine invariant to derive new algebraic connections between
perspective views. It is shown that three perspective views of an
object are connected by certain algebraic functions of image
coordinates alone (no structure or camera geometry needs to be
involved).
--------------
:CBCL Paper #79/AI Memo #1390
:author Jose L. Marroquin and Federico Girosi
:title Some Extensions of the K-Means Algorithm for Image Segmentation
and Pattern Classification
:date January 1993
:pages 21
:keywords K-means, clustering, vector quantization, segmentation,
classification
We present some extensions to the k-means algorithm for vector
quantization that permit its efficient use in image segmentation and
pattern classification tasks. We show that by introducing a certain
set of state variables it is possible to find the representative
centers of the lower dimensional manifolds that define the boundaries
between classes; this permits one, for example, to find class
boundaries directly from sparse data or to efficiently place centers
for pattern classification. The same state variables can be used to
determine adaptively the optimal number of centers for clouds of data
with space-varying density. Some examples of the application of these
extensions are also given.
--------------
:CBCL Paper #81/AI Memo #1432
:title Conditions for Viewpoint Dependent Face Recognition
:author Philippe G. Schyns and Heinrich H. B\"ulthoff
:date August 1993
:pages 6
:keywords face recognition, RBF Network Symmetry
Face recognition stands out as a singular case of object recognition:
although most faces are very much alike, people discriminate between many
different faces with outstanding efficiency. Even though little is known
about the mechanisms of face recognition, viewpoint dependence, a recurrent
characteristic of many research on faces, could inform algorithms and
representations. Poggio and Vetter's symmetry argument predicts that learning
only one view of a face may be sufficient for recognition, if this view allows
the computation of a symmetric, "virtual," view. More specifically, as faces
are roughly bilaterally symmetric objects, learning a side-view---which always
has a symmetric view--- should give rise to better generalization performances
that learning the frontal view. It is also predicted that among all new
views, a virtual view should be best recognized. We ran two psychophysical
experiments to test these predictions. Stimuli were views of 3D models of
laser-scanned faces. Only shape was available for recognition; all other face
cues--- texture, color, hair, etc.--- were removed from the stimuli. The first
experiment tested wqhich single views of a face give rise to best
generalization performances. The results were compatible with the symmetry
argument: face recognition from a single view is always better when the
learned view allows the computation 0f a symmetric view.
--------------
:CBCL Paper #82/AI Memo #1437
:author Reza Shadmehr and Ferdinando A. Mussa-Ivaldi
:title Geometric Structure of the Adaptive Controller of the
Human Arm
:date July 1993
:pages 34
:keywords Motor learning, reaching movements, internal models, force fields,
virtual environments, generalization, motor control
The objects with which the hand interacts with may significantly change the
dynamics of the arm. How does the brain adapt control of arm movements
to this new dynamics? We show that adaptation is via composition of a
model of the task's dynamics. By exploring generalization capabilities
of this adaptation we infer some of the properties of the computational
elements with which the brain formed this model:
the elements have broad receptive fields and encode the learned
dynamics as a map structured in an intrinsic coordinate system closely related
to the geometry of the skeletomusculature. The low--level nature of
these elements suggests that they may represent a set of primitives
with which movement are represented in the CNS.
--------------
:CBCL Paper #83/AI Memo #1440
:author Michael I. Jordan and Robert A. Jacobs
:title Hierarchical Mixtures of Experts and the EM Algorithm
:date August 1993
:pages 29
:keywords supervised learning, statistics, decision trees, neural
networks
We present a tree-structured architecture for supervised learning. The
statistical model underlying the architecture is a hierarchical mixture model
in which both the mixture coefficients and the mixture components are
generalized linear models (GLIM's). Learning is treated as a maximum
likelihood problem; in particular, we present an Expectation-Maximization (EM)
algorithm for adjusting the parameters of the architecture. We also develop
an on-line learning algorithm in which the parameters are updated
incrementally. Comparative simulation results are presented in the robot
dynamics domain.
--------------
:CBCL Paper #84/AI Memo #1441
:title On the Convergence of Stochastic Iterative Dynamic Programming
Algorithms
:author Tommi Jaakkola, Michael I. Jordan and Satinder P. Singh
:date August 1993
:pages 15
:keywords reinforcement learning, stochastic approximation,
convergence, dynamic programming
Recent developments in the area of reinforcement learning have yielded a
number of new algorithms for the prediction and control of Markovian
environments. These algorithms, including the TD(lambda) algorithm of Sutton
(1988) and the Q-learning algorithm of Watkins (1989), can be motivated
heuristically as approximations to dynamic programming (DP). In this paper
we provide a rigorous proof of convergence of these DP-based learning
algorithms by relating them to the powerful techniques of stochastic
approximation theory via a new convergence theorem. The theorem establishes
a general class of convergent algorithms to which both TD (lambda) and
Q-learning belong.
--------------
:CBCL Paper #86/AI Memo #1449
:title "Formalizing Triggers: A Learning Model for Finite Spaces"
:author Patha Niyogi and Robert Berwick
:pages 14
:keywords language learning, parameter systems, Markov chains,
convergence times, computational learning theory
:date November 1993
In a recent seminal paper, Gibson and Wexler (1993) take important
steps to formalizing the notion of language learning in a (finite)
space whose grammars are characterized by a finite number of {\it
parameters\/}. They introduce the Triggering Learning Algorithm (TLA)
and show that even in finite space convergence may be a problem due to
local maxima. In this paper we explicitly formalize learning in finite
parameter space as a Markov structure whose states are parameter
settings. We show that this captures the dynamics of TLA completely
and allows us to explicitly compute the rates of convergence for TLA
and other variants of TLA e.g. random walk. Also included in the paper
are a corrected version of GW's central convergence proof, a list of
``problem states'' in addition to local maxima, and batch and
PAC-style learning bounds for the model.
--------------
:CBCL Paper #87/AI Memo #1458
:title "Convergence Results for the EM Approach to Mixtures of Experts
Architectures"
:author Michael Jordan and Xei Xu
:pages 33
:date September 1993
The Expectation-Maximization (EM) algorithm is an iterative approach to maximum likelihood parameter estimation. Jordan and Jacobs (1993) recently proposed an EM algorithm for the mixture of experts architecture of Jacobs, Jordan, Nowlan and Hinton (1991) and the hierarchical mixture of experts architecture of Jordan and Jacobs (1992). They showed empirically that the EM algorithm for these architectures yields significantly faster convergence than gradient ascent. In the current paper we provide a theoretical analysis of this algorithm. We show that the algorithm can be regarded as a variable metric algorithm with its searching direction having a positive projection on the gradient of the log likelihood. We also analyze the convergence of the algorithm and provide an explicit expression for the convergence rate. In addition, we describe an acceleration technique that yields a significant speedup in simulation experiments.
--------------
:CBCL Paper #89/AI Memo #1461
:title Face Recognition under Varying Pose
:author David J. Beymer
:pages 14
:date December 1993
:keywords computer vision, face recognition, facial feature detection,
template matching
While researchers in computer vision and pattern recognition have
worked on automatic techniques for recognizing faces for the last 20
years, most systems specialize on frontal views of the face. We
present a face recognizer that works under varying pose, the difficult
part of which is to handle face rotations in depth. Building on
successful template-based systems, our basic approach is to represent
faces with templates from multiple model views that cover different
poses from the viewing sphere. Our system has achieved a recognition
rate of 98% on a data base of 62 people containing 10 testing and 15
modelling views per person.
--------------
:CBCL Paper #90/AI Memo #1452
:title Algebraic Functions for Recognition
:author Amnon Shashua
:pages 11
:date January 1994
In the general case, a trilinear relationship between three perspective views
is shown to exist. The trilinearity result is shown to be of much practical
use in visual recognition by alignment --- yielding a direct method that cuts
through the computations of camera transformation, scene structure and epipolar
geometry. The proof of the central result may be of further interest as it
demonstrates certain regularities across homographies of the plane and
introdues new view invariants. Experiments on simulated and real image data
were conducted, including a comparative analysis with epipolar intersection
and the linear combination methods, with results indicating a greater degree
of robustness in practice and higher level of performance in re-projection
tasks.
============================
How to get a copy of a report:
The files are in compressed postscript format and are named by their
AI memo number. They are put in a directory named as the year
in which the paper was written.
Here is the procedure for ftp-ing:
unix> ftp publications.ai.mit.edu (128.52.32.22, log-in as anonymous)
ftp> cd ai-publications/1993
ftp> binary
ftp> get AIM-number.ps.Z
ftp> quit
unix> zcat AIM-number.ps.Z | lpr
Best wishes,
Reza Shadmehr
Center for Biological and Computational Learning
M. I. T.
Cambridge, MA 02139
------------------------------
End of VISION-LIST digest 13.11
************************