Copy Link
Add to Bookmark
Report
VISION-LIST Digest Volume 10 Issue 37
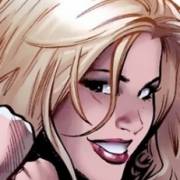
VISION-LIST Digest Thu Sep 05 13:37:53 PDT 91 Volume 10 : Issue 37
- Send submissions to Vision-List@ADS.COM
- Vision List Digest available via COMP.AI.VISION newsgroup
- If you don't have access to COMP.AI.VISION, request list
membership to Vision-List-Request@ADS.COM
- Access Vision List Archives via anonymous ftp to ADS.COM
Today's Topics:
New editors for IJCV
Linear arrays
Image quality Metrics
Gray-scale Image Segmentation using Mathematical Morphology
Call for participation in a Joint Report
Need DB of engineering drawings or handwritten characters
Research Assistant Wanted
CFP: IAPR Workshop on Structural and Syntactic Pattern Recognition
CFP: Session Theme on Automated Inspection Technology and Techniques
Applying Intelligence to Segmentation (LONG)
----------------------------------------------------------------------
Date: Wed, 4 Sep 1991 13:46-EDT
From: Takeo.Kanade@IUS5.IUS.CS.CMU.EDU
Subject: New editors for IJCV
International Journal of Computer Vision
announces
NEW EDITORS-IN-CHIEF
The International Journal of Computer Vision (IJCV) is pleased to announce
the appointment of two new Editors-in-Chief. Robert Bolles of SRI
Artificial Intelligence Center and Olivier Faugeras of INRIA will join
Takeo Kanade as Editors-in-Chief. Rod Brooks, one of the founding Editors
who has served for five years, is stepping down.
The International Journal of Computer Vision provides a forum for the
dissemination of new research results in the rapidly growing field of
computer vision. IJCV, which began as a quarterly journal in 1987 and has
expanded to a bimonthly in 1990, publishes papers of the highest quality in
the field.
The journal has had special unique features, including the Marr prize
special issues, color issues, and special issues on research institutions.
We plan to initiate two more new features: papers with open peer
commentary and papers for experimental replication. Details will be
announced in the editorials of the editorials of the coming journal issues.
Please send paper submissions, as well as opinions and suggestions, to any
one of the following Editors-in-Chief:
Takeo Kanade Robert Bolles
School of Computer Science Artificial Intelligence Center
Carnegie Mellon University 333 Ravenswood Avenue
Pittsburgh, PA 15213 Menlo Park, CA 94025
kanade@cs.cmu.edu bolles@iu.ai.sri.com
phone: (412) 268-3016 phone: (415) 859-4620
fax: (412) 621-1970 fax: (415) 859-3735
Olivier Faugeras
INRIA SOPHIA-Antipolis
Unite de Recherche
2004 route des Lucioles
06565 Valbonne Cedex
FRANCE
faugeras@mirsa.inria.fr
phone: (33) 93-65-7831
fax: (33) 93-65-7845
Instructions for Authors are printed in issues of the journal or may be
obtained from the Editors-in-Chief.
------------------------------
Date: Wed, 4 Sep 91 11:48:25 -0400
From: Al Boehnlein <ajb@iti.org>
Subject: Linear arrays
I am looking for linear arrays, linear array drivers, and a digitizing
board for a pc. I need a stable, low noise system that does not have
any phase shift in the digitization process because I am looking for
sub-pixel accuracy using sub-pixel correlation. We will most likely
be using something on the order of three 4096 or 5000 element arrays
with 7 micron spacing. Do you have any hints(good or bad) about
hardware? I have done some preliminary work with a 2048 pixel
Fairchild camera and driver electronics digitized and driven by a
metrabyte DASH 16 board. The DASH 16 seems too slow, and the signal
is noisy and the two video channels have independent base line drift.
I don't know if the drift and noise are caused by running the system
so slow (~60 kHz) or if its just the nature of the Fairchild system.
Any info is appreciated
ajb
------------------------------
Date: 27 Aug 91 19:06:20 GMT
From: abirenbo@adara.cel.scg.hac.com (Aaron Birenboim)
Organization: Hughes Aircraft Colorado Engineering Labs
Subject: Image quality Metrics
Summary: do you know any measures for image quality?
Has anybody out there ever heard of a paper on Image quality
metrics? I am most interested in metrics which could be used to
quantify the quality of a compressed image vs. the original. I am
looking for measures which are a bit off the main track. For example,
I have been trying to invent measures which quantify how well
textureal properties are preserved by some compressor.
I have already used most of the standard methods...
(RMS, MSE, MAE, SNR, and others)
Aaron Birenboim |I am just too tired to think
abirenbo%rigel.cel.scg.hac.com@hac2arpa.hac.com|of a good quote.
abirenbo@isis.cs.du.edu | - ChickenHead
w (303) 344-6486 |
------------------------------
Date: Fri, 30 Aug 91 12:12:19 -0400
From: econrad@valhalla.cs.wright.edu (Eric Conrad)
Subject: Gray-scale Image Segmentation using Mathematical Morphology
In my master's thesis, I will be surveying techniques for segmenting
gray-scale images with an emphasis on techniques based on mathematical
morphology. During the course of my research so far, I have come
across references to reports by the Center for Mathematical Morphology
in Paris, France. How can such reports be obtained or requested?
Also, any pointers to *recent* publications in the area would be
greatly appreciated.
Thanks,
Eric Conrad
------------------------------
Date: Sun, 1 Sep 91 4:34:39 BST
From: A.Etemadi@ee.surrey.ac.uk
Subject: Call for participation in a Joint Report
G'Day
This is a call for participation in a joint report. I would like to
compare as many techniques for the extraction of line and arc features
from edgemaps as possible. Rewriting code to do this is not possible
given the large number of techniques, and their complexity. I have not
seen such a survey anywhere probably for this reason. Another
possibility is that authors do not release their code since it maybe
mishandled by other people, leading to poor results. The proposal is
to:
1. Send 3 synthetic, and 3 "real" edgemaps to participants by Email.
These will be raw images with edge pixels set to 255, and 0 background.
2. Get back by Email files containing :
a) Reference(s) to paper(s) describing the algorithm
b) ASCII lists of segments and the file format
c) The values of any associated user supplied parameters (eg. thresholds)
I will then create:
1. A database of the results
2. Histograms of the line/arc segments derived by the various algorithms
applied to the Synthetic images.
3. Write the report and distribute it, in the first instance, only to the
contributors
Hopefully, if there is sufficient response and all contributors agree, the
report can be distributed to any other interested parties.
regards
Dr A. Etemadi <(|)>
Dr. A. Etemadi, | Phone: (0483) 300-800 Ext. 2311
V.S.S.P. Group, | Fax : (0483) 300-803
Dept. of Electronic and Electrical Eng., | Email:
University of Surrey, | Janet: a.etemadi@ee.surrey.ac.uk
Guildford, | ata@c.mssl.ucl.ac.uk
Surrey GU2 5XH | SPAN : ata@mssl
United Kingdom | ata@msslc
------------------------------
Date: 30 Aug 91 17:11:48 GMT
From: ss4@condo.cis.ufl.edu (Sergio Schuler)
Organization: UF CIS Dept.
Subject: Need DB of engineering drawings or handwritten characters
Hello!!!
We are looking for a public domain database of Engineering Drawings or
Hand-Written characters. We are working on recognition of enginnering
drawings, etc. We need a database with at least 2000 characters to train
a neural network.
Any help in this matter would be appreciated.
Sergio Schuler
email: ss4@reef.cis.ufl.edu
------------------------------
Date: Fri, 30 Aug 91 22:35:13 GMT
From: donohoe%jemez.eece.unm.edu@lynx.unm.edu (Greg W. Donohoe)
Organization: University of New Mexico, Albuquerque
Subject: Research Assistant Wanted
Research Assistant Wanted
We are looking for a graduate student to apply image processing and
computer vision techniques to the analysis of sequences of images. The
student will develop algorithms and implement enhancements to a
computer program used to automatically analyze the motion of soil
grains in a sequence of video frames taken during an experiment. The
job pays $800 for half-time work, plus a tuition waiver.
Qualifications:
o Graduate Student in Electrical or Computer Engineering
o Course work or experience in image processing or computer vision
o Excellent programming skills, especially Unix and C
Experience with pattern recognition, artificial intelligence, computer
graphics, or Khoros programming would be helpful. The sponsor prefers a
U.S. Citizen.
Please submit a resume to
Prof. Greg Donohoe
EECE Department
University of New Mexico
Albuquerque, NM 87131
Phone: (505) 277-6724
e-mail: donohoe@chama.eece.unm.edu
------------------------------
Date: Thu, 5 Sep 1991 13:57:53 +0200
From: Thomas Glauser <glauser@iam.unibe.ch>
Subject: CFP: IAPR Workshop on Structural and Syntactic Pattern Recognition
CALL FOR PAPERS
IAPR International Workshop on
Structural and Syntactic Pattern Recognition
Bern, Switzerland
August 26--28, 1992 (immediately prior to the 11th ICPR)
Chairman: H. Bunke, University of Bern
Program Committee: H. Baird (AT\&T Bell Labs, Murray Hill, USA)
R.E. Haralick (University of Washington, Seattle, USA)
R.L. Kashyap (Purdue University, West Lafayette, USA)
R. Mohr (Institut National Polytechnique, Grenoble, France)
R. de Mori (Mc Gill University, Montreal, Canada)
H. Niemann (University of Erlangen, Germany)
H. Noltemeier (University of W\"{u}rzburg, Germany)
L.F. Pau (Digital Equipment Co., Sophia Antipolis, France)
A. Rosenfeld (University of Maryland, College Park, USA)
A. Sanfeliu (Polytechnical University of Catalonia,
Barcelona, Spain)
S. Tsuji (University of Osaka, Japan)}
Traditionally, structural and syntactic pattern recognition refers
to concepts from formal language theory and their generalization to
fit various needs in pattern recognition. In this workshop the
discipline of structural and syntactic pattern recognition will be
understood in a broad sense and will include any type of matching,
search, optimization, inference, reasoning etc. procedure that is
mainly based on a symbolic or mixed symbolic-numerical representation
of the patterns under study. Topics of interest include, but are not
limited, to the following:
- symbolic data structures for pattern representation
- matching of symbolic structures
- new concepts in syntactic parsing
- inference and reasoning for pattern recognition
- learning of structural models and grammatical inference
- hybrid methods
- parallel algorithms
- traditional and new applications in speech understanding and
1-D signal analysis, 2-D and 3-D computer vision, image sequence analysis,
document image understanding, and other fields.
Please, submit three copies of your paper (maximum length 15 pages, including
figures, tables, references etc.) to
H. Bunke
Institut fuer Informatik und angewandte Mathematik
University of Bern
Laenggassstrasse 51
CH-3012 Bern, Switzerland
Phone: +41-31-654451 or -658681
Fax: +41-31-653965
Email: bunke@iam.unibe.ch (no electronic submissions, please!)
Accepted papers will be included in the proceedings to be published
after the workshop.
Schedule: April 1, 1992 deadline for submitted papers
June 15, 1992 notification of acceptance
July 15, 1992 deadline for advance registration
August 26, 1992 (at the workshop) revised versions of papers
Important note: If you wish to attend the workshop without presenting
a paper, please inform H. Bunke at the address given above before June 15,
1992. You will then get the necessary material for registration.
For further questions, please contact H. Bunke.
------------------------------
Date: Wed, 4 Sep 91 21:48:01 EDT
From: aar@vnet.ibm.com
Subject: CFP: Session Theme on Automated Inspection Technology and Techniques
CALL FOR PAPERS
Session Theme
on
AUTOMATED INSPECTION TECHNOLOGY AND TECHNIQUES
A special session on this theme has been planned for the upcoming
Machine Vision and Robotics conference. Automated inspection and
measurement plays a major role in product manufacturing as it
increases production rates, and it helps achieve high-quality
assurance and long-term product reliability. With increasing
product complexity, quality demands, and throughput demands,
special machine vision technologies and techniques for automated
inspection have continuously emerged. Papers are solicited in all
areas of automated inspection, including, but not limited to:
- high-speed and specialized architectures
- inspection of aerospace parts
- reference-based inspection systems
- design-rule inspection systems
- measurement techniques
- machine vision performance verification: accuracy and precision
- defect detection and verification
- defect classification rules
- illumination techniques
- calibration, alignment, and registration techniques
- reference database design
Papers describing successful applications, case studies, and
integration issues will be also welcomed.
The Machine Vision and Robotics conference will be held April
20-24, 1992, at the Marriott Orlando World Center Resort and
Convention Center. The deadline for paper submissions is
September 23, 1991. (Please also send an e-mail note to
aar@vnet.ibm.com if you plan to submit a paper for this
special theme session.) Four copies of a 2000 word (or greater)
extended summary should be submitted to:
SPIE
Applications of AI X / Machine Vision & Robotics
P.O. Box 10
Bellingham, WA 98227-0010 , USA
Shipping address: 1000 20th St., Bellingham, WA 98225
Please indicate that the paper is a submission to the conference
on Machine Vision and Robotics following the authors' address.
Papers submitted to the Machine Vision and Robotics conference should
NOT also be submitted to the Expert and Knowledge-Based Systems part
of Applications of AI X. Each paper will be reviewed by two members
of the program committee and reviews returned to the authors.
The program committee and the conference chair will make a
selection of the best papers accepted for the Machine Vision and
Robotics Conference, and these authors will be invited to submit
a revised version of their paper to a special issue of the
journal Machine Vision & Applications.
Conference Chair:
Kevin Bowyer, Univ of South Florida (kwb@csee.usf.edu)
Program Committee:
Ron Arkin, Georgia Tech Bir Bhanu, Univ of California at Riverside
Kim Boyer, Ohio State Univ Horst Bunke, Univ of Berne (Switzerland)
Chuck Dyer, Univ of Wisconsin Henrik Christensen, Univ of Aalborg (Denmark)
Ramesh Jain, Univ of Michigan Dmitry Goldgof, Univ of South Florida
Howard Moraff, NSF Worthy Martin, Univ of Virginia
Prasanna Mulgaonkar, S.R.I. Arturo Rodriguez, IBM Corporation
Ishwar Sethi, Wayne State Univ Mubarak Shah, Univ of Central Florida
Wes Snyder, Wake Forest Univ Louise Stark, Univ of South Florida
Susan Stansfield, Sandia Labs George Stockman, Michigan State Univ
Tzay Young, Univ of Miami Mohan Trivedi, Univ of Tennessee
The Automated Inspection Technology and Techniques session is being
organized by:
Arturo Rodriguez, IBM Corporation
Jorge Sanz, IBM Almaden Research
Thank you,
Ed Delp, Purdue University
------------------------------
From: buck@gris.informatik.uni-tuebingen.de (Thomas Buck)
Date: Tue, 3 Sep 91 15:29:32 MES
Subject: Applying Intelligence to Segmentation (LONG)
SOME CONSIDERATIONS FOR APPLYING "INTELLIGENCE" TO SEGMENTATION
Hi, everybody. My name is Thomas Buck, and I'm studying Digital Image
Processing at the University of Tuebingen, West Germany. Since I'm not from
an english country, I apologize for my poor english.
I'm now working towards a PhD in the field of Computer Graphics and Image
Processing in the sense of better segmenting images, specially in the field
of medicine. We have implemented here on our institute a lot of algorithms
like region growing, filtering, histogram manipulation, edge detection, and
so on. But it still far, far away from the capacity of a human expert in
segmenting images.
So my real intent herewith is to discuss and change opinions with people that
have some interest in what I'm going to talk about. First I will express my
(ONLY MINE) opinion and then try to pose some questions, because I feel that
I cannot answer it alone.
My idea is to discuss the advantages and disadvantages of using algorithms
from the field of Artificial Intelligence (AI) and Natural Intelligence
(NI) into the segmentation process.
Let's begin with the definition of segmentation.
In the article [1.1] it is stated that if I is the set of all pixels,
that means, the image to be segmented, the process of partitioning the
set I in subsets {R1, R2, ..., Rn} must be so, that the 5 following rules
must be fulfilled:
[1] Union Ri = I;
[2] Ri is a connected region, for all i, i = 1, 2, ..., n;
[3] Ri Intersection Rj = NULL, for all i, j, i <> j;
[4] P(Ri) = TRUE, for all i, i = 1, 2, ..., n;
[5] P(Ri Union Rj) = FALSE, for all i, j, i <> j.
Some direct consequences can be extracted from this definition. The first,
and most important (I think), is that the partitioning of the pixels does NOT
depend on their color values. They depend on the property P(.), so that we
can have in one region pixels with approximattely constant value (after a
region growing process, for example), pixels very different in the neighborhood
of two regions (after a gradient detection process, for example), but we can
ALSO have both types above in the same region. It just have to satisfy the
predicate P.
In [1.2] it is stated that if I is the set of all pixels,
a segmentation of I is a partitioning set of connected subsets,
or image regions, {R1, R2, ..., Rn} such that
n
[1] Union Rl = I, where Rl Intersection Rm = NULL, for all l <> m.
l=1
In addition, for identification purposes, a "uniformity predicate" P(.) is
defined on groups of connected pixels such that
[2] P(Rl) = TRUE, for all l;
[3] P(Rl Union Rm) = FALSE, for all Rl adjacent to Rm.
A partition is defined to be "correct" when every region hypothesis Hl for
Rl satisfies the smoothness predicate P(.) and contains no gross error. A
partition is "complete" when every Hl contains EXACTLY all pixels p(i,j) of
Rl.
So, the predicate P is the "center" of all the process. It is P that has the
capacity of separating our "regions of interest" (ROIs).
So, I'm trying to put some "intelligence" (let's call it so) in the segmen-
tation process, so that it will separate our ROIs, in my case of study, the
organs from CT images.
I don't want to discuss the quality of the mathematics of operators like Canny,
Marr and Hildreth, nor the searching capacity of the A* algorithm in a graph,
nor the learning capability of a Artificial Neural Network or a Genetic
Algorithm. What I want to discuss is the capacity of all this together in
helping me finding the ROIs that I want.
I think that, for example, algorithms for modeling objects, filtering noise,
detecting imperfections, finding edges, representing a set of points as a
cubic spline, and so on, are quite well solved, but given a raw image, speci-
fically a 2D array of pixels, how can we be sure that after some processing
time, the ROIs will be found ?
I see the segmentation process, in a very gross model, as a "black box".
The figure below will help my explanation.
+------------------------+
Image -> | Segmentation Process | -> ROIs
+------------------------+
Fig. 1: Black Box Model for the Segmentation Process
It is not important what in the black box, the only thing that matters is
that at the end of the process, we have our image regions as we "a priori"
wanted. Inside of the black box can be anything, it just has to find
the ROIs.
The data structures above are the following: first an image, composed of a 2D
array with NxM pixels, each of P graylevels. Here I will make my first res-
triction, that the image is not color, so it has just 1 value for the gray,
anmd not 3 for RGB system. But I think it is not so important for my discu-
ssion. The other data structure is the description of our ROIs, in the form
of a labeled graph, B-spline descriptions of the contours, another image with
n graylevels, in which each graylevel corresponds to a region, and whatsoever.
Just for simplicity I will make another restriction, that is, my images come
from medicine. I'm thinking about CT, MRI or DSA images. So I will not
touch the field of Shape-from-X, nor Range Images.
So, I see the segmentation algorithm as a process that transforms one data
structure in another one, that can be later used for other purposes, such
as visualising, analysing, describing, etc.
Thinking about the use of, for example, edge detectors in my image, I
realized that it is not well for some reasons. Maybe the gradient of some very
small neighborhood in my image is not a very good source for separating some
ROIs from anothers in a medical image. I'm not trying to say that it is ALWAYS
that our regions have just some very near pixel values or very distant ones,
but my segmentation black box has to have the capacity of sometimes separating
as well as sometimes NOT separating regions in the sense of a gradient.
So they seem to be NOT optimal for finding my ROIs. We have to have something
more robust, more reliable.
We have implemented some of the most famous algorithms, but the results from
them were not encouraging. Our implementation begins with the original
image, filtered, and whatsoever, detects the edges of the image and returns
with another image with every region represented by one different graylevel.
So I was wondering about the use of other sources of knowledge in my black box.
Let's say an Expert System (ES), an Artificial Neural Network (ANN), a Genetic
Algorithm (GA), and so many other things. But here we come to another source
of errors, I mean, transforming the image into another representation. Let's
see in a little bit more detail.
To let an A* algorithm run through my graph representing the image, I have
to first transform my 2D data structure into a graph. To use a neural network
to find my ROIs, I have to transform my 2D image into some coeficients to be
given to my neurons. The same has to be done in the case of a genetic algorithm
or an expert system, in the form of "genes" and rules or frames, respectively.
Globaly said, I cannot give my 2D image into these kind of algorithms, that
means, I have to have some interfaces in the black box model, as stated gra-
phically in the next figure, where Analysis means transforming the image data
structure into some kind of representation understandable to my new algorithms,
ans Synthesis as the inverse process.
+-----------------------------------------------------------+
| +----------+ +----------------------+ +-----------+ |
Image ->| | Analysis | -> | Segmentation Process | -> | Synthesis | |-> ROIs
| +----------+ +----------------------+ +-----------+ |
+-----------------------------------------------------------+
Fig. 2: Modified Black Box Model for 'Intelligent' Segmentation
Of course, it is clear that in these transformation processes (I mean, Analysis
and Synthesis) one looses information. Representing the pixel values in the
form of some coeficients is NOT the same as given the image itself to the black
box, nor in the form of some rules, etc. But I win (supposely) in flexibility,
adaptability, robustness and learning capacity.
So my new bottleneck in the whole segmentation process would be now how well
one has to transform the 2D image as to give to these new algorithms (GA, ES
or ANN). I am "a priori" supposing that if one represents well enough the
image, the corresponding algorithm will correctly output the ROIs, which will
be inverse transformed in the Synthesis block of the black box.
I think that there must be some way for these AI and NI algorithms to know
how to process an image. For example, the representation of the image in the
form for an ANN could result in a number, at the output layer, that represents
the following statement: "After filtering the image with a 5x5 median filter,
make the histogram and threshold it with the value 120".
Or I could have a set of rules in the form for an ES that would do something
like above. The same for an GA.
If we have a digital image processing system written without this paradigms,
the only way of "imitating intelligence" is to implement explicitly it in
form of C code, for example. So, the programmer of the system will have to take
into account all this kind of things, and whatever good the programmer will
put his "knowledge" into the code, it will just follow the reasoning process
of its creator. I don't think it's the solution of everything. Maybe it will
help a lot, may even solve some problems, but that's not sufficient.
But, if we build some intellligence in the system, in form of ANN, ES, or even
GA, we give the system the possibility of adaptation and reasoning for itself.
So it will modify its parameters and its way of thinking
(what I called "hardcoding in the C program" above) depending on each problem.
It will not be deterministic anymore, like a C program.
So, supposing that I can transform my image into rules, neurons, genes and so
on, what is wrong in my black box model ? Will I achieve what I want, I mean,
find the ROIs according to my P(.) predicate ?
Has anybody out there tried it once before ? What were the results ? Does
it have future ? Can we expect better results if we continue our research ?
The references [1.1] and [1.2] will be given in the very near future,
since I will post my BibTeX for this list.
Thanks a lot for your time,
Thomas Buck
THOMAS DE ARAUJO BUCK :-)
Universitaet Tuebingen
Wilhelm Schickard Institut fuer Informatik
Auf der Morgenstelle 10, C-9
7400 Tuebingen 1 - DEUTSCHLAND
Telefon: +49 (0) 7071 / 29-6356 - 29-5464
Fax: +49 (0) 7071 / 29-5466
Internet : buck@gris.informatik.uni-tuebingen.de
------------------------------
End of VISION-LIST digest 10.37
************************