Copy Link
Add to Bookmark
Report
VISION-LIST Digest 1989 07 14
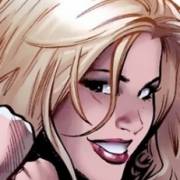
Vision-List Digest Fri Jul 14 14:08:05 PDT 89
- Send submissions to Vision-List@ADS.COM
- Send requests for list membership to Vision-List-Request@ADS.COM
Today's Topics:
Call for Papers: INNS/IEEE Conference on Neural Networks, Jan. 1990
Intensive summer school on statistical pattern recognition
----------------------------------------------------------------------
Date: 10 Jul 89 04:39:00 GMT
From: lehr@isl.stanford.edu (Michael Lehr)
Subject: Call for Papers: INNS/IEEE Conference on Neural Networks, Jan. 1990
Summary: Papers requested for joint neural net conference in Washington DC
Keywords: conference, neural networks
Organization: Stanford University EE Dept.
CALL FOR PAPERS
International Joint Conference on Neural Networks
IJCNN-90-WASH DC
January 15-19, 1990,
Washington, DC
The Winter 1990 session of the International Joint Conference on
Neural Networks (IJCNN-90-WASH DC) will be held on January 15-19, 1990
at the Omni Shoreham Hotel in Washington, DC, USA. The International
Neural Network Society (INNS) and the Institute of Electrical and
Electronics Engineers (IEEE) invite all those interested in the field
of neural networks to submit papers for possible publication at this
meeting. Brief papers of no more than 4 pages may be submitted for
consideration for oral or poster presentation in any of the following
sessions:
APPLICATIONS TRACK:
* Expert System Applications
* Robotics and Machine Vision
* Signal Processing Applications (including speech)
* Neural Network Implementations: VLSI and Optical
* Applications Systems (including Neurocomputers & Network
Definition Languages)
NEUROBIOLOGY TRACK:
* Cognitive and Neural Sciences
* Biological Neurons and Networks
* Sensorimotor Transformations
* Speech, Audition, Vestibular Functions
* Systems Neuroscience
* Neurobiology of Vision
THEORY TRACK:
* Analysis of Network Dynamics
* Brain Theory
* Computational Vision
* Learning: Backpropagation
* Learning: Non-backpropagation
* Pattern Recognition
**Papers must be postmarked by August 1, 1989 and received by August
10, 1989 to be considered for presentation. Submissions received
after August 10, 1989 will be returned unopened.**
International authors should be particularly careful to submit their
work via Air Mail or Express Mail to ensure timely arrival. Papers
will be reviewed by senior researchers in the field, and author
notifications of the review decisions will be mailed approximately
October 15, 1989. A limited number of papers will be accepted for
oral and poster presentation. All accepted papers will be published
in full in the meeting proceedings, which is expected to be available
at the conference. Authors must submit five (5) copies of the paper,
including at least one in camera-ready format (specified below), as
well as four review copies. Do not fold your paper for mailing.
Submit papers to:
IJCNN-90-WASH DC
Adaptics
16776 Bernardo Center Drive, Suite 110 B
San Diego, CA 92128 UNITED STATES
(619) 451-3752
SUBMISSION FORMAT:
Papers should be written in English and submitted on 8-1/2 x 11 inch
or International A4 size paper. The print area on the page should be
6-1/2 x 9 inches (16.5 x 23 cm on A4 paper). All text and figures
must fit into no more than 4 pages. The title should be centered at
the top of the first page, and it should be followed by the names of
the authors and their affiliations and mailing addresses (also
centered on the page). Skip one line, and then begin the text of the
paper. We request that the paper be printed by typewriter or
letter-quality printer with clear black ribbon, toner, or ink on plain
bond paper. We cannot guarantee the reproduction quality of color
photographs, so we recommend black and white only. The type font
should be Times Roman or similar type font, in 12 point type
(typewriter pica). You may use as small a type as 10 point type
(typewriter elite) if necessary. The paper should be single-spaced,
one column, and on one side of the paper only. Fax submissions are
not acceptable.
**Be sure to specify which track and session you are submitting your
paper to and whether you prefer an Oral or Poster presentation. Also
include the name, complete mailing address and phone number (or fax
number) of the author we should communicate with regarding your
paper.**
If you would like to receive an acknowledgment that your paper has
been received, include a self-addressed, stamped post-card or envelope
for reply, and write the title and authors of the paper on the back.
We will mark it with the received date and mail it back to you within
48 hours of receipt of the paper. Submission of the paper to the
meeting implies copyright approval to publish it as part of the
conference proceedings. Authors are responsible for obtaining any
clearances or permissions necessary prior to submission of the paper.
------------------------------
Date: 14 Jul 89 14:05:00 WET
From: Josef Kittler <kittler%ee.surrey.ac.uk@NSFnet-Relay.AC.UK>
Subject: Intensive summer school on statistical pattern recognition
INTENSIVE SUMMER SCHOOL
ON
STATISTICAL PATTERN RECOGNITION
11-15 September 1989
University of Surrey
PROGRAMME
The course is divided into two parts:
Course A The Fundamentals of Statistical
Pattern Recognition
Course B Contextual Statistical Pattern
Recognition
Course A will cover the basic methodology of
statistical pattern recognition. Course B will feature a number of
advanced topics concerned
with the use of contextual information in pattern recognition, with a
particular emphasis on Markov models in speech and images.
Several example classes will be aimed at familiarizing the participants
with the material presented. The course will include a seminar on
application of pattern recognition methods to specific problems in which a
step by step description of the design of practical pattern recognition
systems will be outlined. Ample time will be devoted to discussion of
algorithmic and practical aspects of pattern recognition techniques.
COURSE A: THE FUNDAMENTALS OF STATISTICAL PATTERN RECOGNITION
11-13 September 1989
ELEMENTS OF STATISTICAL DECISION THEORY
Model of pattern recognition system. Decision theoretic approach to pattern
classification. Bayes decision rule for minimum loss and minimum error rate.
Sequential and sequential compound decision theory. Optimum error
acceptance tradeoff. Learning algorithms.
NONPARAMETRIC PATTERN CLASSIFICATION
The Nearest Neighbour (NN) technique: 1-NN, k-NN, (k,k')-NN pattern
classifiers. Error acceptance tradeoff for nearest neighbour classifiers.
Error bounds. Editing techniques.
DISCRIMINANT FUNCTIONS
Discriminant functions and learning algorithms. Deterministic learning. The
least square criterion and learning scheme, relationship with the 1-NN
classifier. Stochastic approximation. Optimization of the functional form of
discriminant functions.
ESTIMATION THEORY
Probability density function estimation: Parzen estimator, k-NN estimator,
orthogonal function estimator. Classification error rate estimation:
resubstitution method, leave-one-out method, error estimation based on
unclassified test samples.
FEATURE SELECTION
Concepts and criteria of feature selection, interclass distance measures,
nonlinear distance metric criterion, probabilistic distance and dependence
measures and their properties, probabilistic distance measures for
parametric distributions, entropy measures (logarithmic entropy, square
entropy, Bayesian distance), algorithms for selecting optimal and
suboptimal sets of features, recursive calculation of parametric
separability measures. Nonparametric estimation of feature selection
criterion functions.
FEATURE EXTRACTION
Probabilistic distance measures in feature extraction, Chernoff
parametric measure, divergence, Patrick and Fisher method.
Properties of
the Karhunen-Lo\`eve expansion, feature extraction techniques based on the
Karhunen-Lo\`eve expansion. Nonorthogonal mapping methods, nonlinear
mapping methods, discriminant analysis.
CLUSTER ANALYSIS
Concepts of a cluster, dissemblance and resemblance measures, globally
sensitive methods, global representation of clusters by pivot points and
kernels, locally sensitive methods (methods for seeking valleys in
probability density functions), hierarchical methods, minimum spanning tree
methods, clustering algorithms.
***************************************************************************
COURSE B: CONTEXTUAL STATISTICAL PATTERN RECOGNITION
14-15 September 1989
INTRODUCTION
The role of context in pattern recognition. Heuristic approaches to contextual
pattern recognition. Labelling of objects arranged in networks (chains,
regular and irregular lattices). Neighbourhood systems. Elements of
compound decision theory.
MODELS
Markov chains. Causal and noncausal Markov
random fields (MRF). Gibbs distributions. Hidden Markov chain and
random field models for speech and images.
Simulation of causal Markov processes. Simulation of noncausal MRF:
The Metropolis algorithm.
DISCRETE RELAXATION
Compatibility coefficients. Concept of consistent labelling. Waltz discrete
relaxation algorithm. Maximum aposteriori probability (MAP) of joint
labelling. Viterbi algorithm for Markov chains, dynamic programming.
Iterative algorithm for local MAP optimization in MRF. Geman and Geman
Bayesian estimation by stochastic relaxation, simulated annealing.
RECURSIVE COMPOUND DECISION RULES
MAP of labelling individual objects. Filtering and fixed-lag smoothing in
hidden Markov chains. Baum's algorithm. Labelling in hidden Markov meshes
and in Pickard random fields. Unsupervised learning of underlying model
parameters.
PROBABILISTIC RELAXATION
Problem specification. Combining evidence. Support functions for specific
neighbourhood systems. Relationship with conventional compatibility and
support functions (arithmetic average and product rule). Global criterion
of ambiguity and consistency. Optimization approaches to label probability
updating (Rosenfeld, Hummel and Zucker algorithm, projected gradient
method).
APPLICATIONS
Speech recognition. Image segmentation. Scene labelling. Texture
generation.
************************************************************************
GENERAL INFORMATION
COURSE VENUE
University of Surrey, Guildford, United Kingdom
LECTURERS
Dr Pierre DEVIJVER & Philips Research Laboratory, Avenue
& Em Van Becelaere 2, B-1170 Brussels, Belgium
Dr Josef KITTLER & Department of Electronic and Electri-
& cal Engineering, University of Surrey,
& Guildford GU2 5XH, England
PROGRAMME SCHEDULE
COURSE A will commence on Monday, September 11 at 10.00 a.m. (registration
9.00 - 10.00 a.m.) and finish on Wednesday, September 13 at 4 p.m.
COURSE B will commence on Thursday, September 14 at 10.00 a.m. (registration
9.00 - 10.00 a.m.) and finish on Friday, September 15 at 4 p.m.
ACCOMMODATION
Accommodation for the participants will be available on the campus of the
University for the nights of 10-14 September at the cost of 27.80
per night covering dinner, bed and breakfast.
REGISTRATION AND FURTHER INFORMATION
Address registration forms and any enquiries to Mrs Marion Harris,
Department of Electronic and Electrical Engineering, University of Surrey,
Guildford GU2 5XH, England,
telephone 0483 571281 ext 2271. Rights reserved to cancel the course or
change the programme if minimum numbers are not obtained or to limit
participation according to capacity. All reservations handled on first-come
first-served basis.
WHO SHOULD ATTEND
The course is intended for graduate students, engineers, mathematicians,
computer scientists, applied scientists, medical physicists and social
scientists engaged in work on pattern recognition problems of practical
significance. In addition programmers and engineers concerned with the
effective design of pattern recognition systems would also benefit.
Applicants for COURSE A should have some familiarity with basic engineering
mathematics and some previous exposure to probability and statistics.
Applicants for COURSE B only should have working knowledge of basic
statistical pattern recognition techniques.
The material covered is directly relevant to applications in
character recognition, speech recognition, automatic medical diagnosis,
seismic data classification, target detection and identification,
remote sensing, computer vision for robotics, and many other
application areas.
------------------------------
End of VISION-LIST
********************