Copy Link
Add to Bookmark
Report
NL-KR Digest Volume 05 No. 14
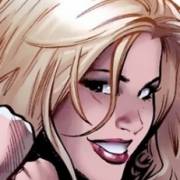
Stevan Harnad Digest (9/09/88 02:01:33) Volume 5 Number 14
Today's Topics:
Pinker & Prince Reply
Submissions: NL-KR@CS.ROCHESTER.EDU
Requests, policy: NL-KR-REQUEST@CS.ROCHESTER.EDU
----------------------------------------------------------------------
Date: Thu, 1 Sep 88 15:05 EDT
From: Stevan Harnad <harnad@mind.UUCP>
Subject: Pinker & Prince Reply (short version)
Posted for Pinker & Prince by S. Harnad
-----------------------------------------------------------
From: Steve Pinker <steve@cogito.mit.edu>
Site: MIT Center for Cognitive Science
Subject: answers to S. Harnad's questions, short version
Alluding to our paper "On Language and Connectionism: Analysis of a
PDP model of language acquisition", Stevan Harnad has posted a list of
questions and observations as a 'challenge' to us. His remarks owe
more to the general ambience of the connectionism / symbol-processing
debate than to the actual text of our paper, in which the questions
are already answered. We urge those interested in these issues to read
the paper or the nutshell version published in Trends in
Neurosciences, either of which may be obtained from Prince (address
below). In this note we briefly answer Harnad's three questions. In
another longer message to follow, we direct an open letter to Harnad
which justifies the answers and goes over the issues he raises in more
detail.
Question # 1: Do we believe that English past tense formation is not
learnable? Of course we don't! So imperturbable is our faith in the
learnability of this system that we ourselves propose a way in which
it might be done (OLC, 130-136).
Question #2: If it is learnable, is it specifically unlearnable by
nets? No, there may be some nets that can learn it; certainly any net
that is intentionally wired up to behave exactly like a rule-learning
algorithm can learn it. Our concern is not with (the mathematical
question of) what nets can or cannot do in principle, but with which
theories are true, and our conclusions were about pattern associators
using distributed phonological representations. We showed that it is
unlikely that human children learn the regular rule the way such a
pattern associator learns the regular rule, because it is simply the
wrong tool for the job. Therefore it's not surprising that the
developmental data confirm that children do not behave in the way that
such a pattern associator behaves.
Question # 3: If past tense formation is learnable by nets, but only
if the invariance that the net learns and that causally constrains its
successful performance is describable as a "rule", what's wrong with
that? Absolutely nothing! --just like there's nothing wrong with
saying that past tense formation is learnable by a bunch of
precisely-arranged molecules (viz., the brain) but only if the
invariance that the molecules learn, etc. etc. etc. The question is,
what explains the facts of human cognition? Pattern associator
networks have some interesting properties that can shed light on
certain kinds of phenomena, such as *irregular* past tense forms. But
it is simply a fact about the *regular* past tense alternation in
English that it is not that kind of phenomenon. You can focus on the
interesting empirical predictions of pattern associators, and use them
to explain certain things (but not others), or you can generalize them
to a class of universal devices that can explain nothing without an
appeal to the rules that they happen to implement. But you can't have
it both ways.
Alan Prince
Program in Cognitive Science
Department of Psychology
Brown 125
Brandeis University
Waltham, MA 02254-9110
prince@brandeis.bitnet
Steven Pinker
Department of Brain and Cognitive Sciences
E10-018
MIT
Cambridge, MA 02139
steve@cogito.mit.edu
References:
Pinker, S. & Prince, A. (1988) On language and connectionism: Analysis
of a parallel distributed processing model of language acquisition.
Cognition, 28, 73-193. Reprinted in S. Pinker & J. Mehler (Eds.),
Connections and symbols. Cambridge, MA: Bradford Books/MIT Press.
Prince, A. & Pinker, S. (1988) Rules and connections in human
language. Trends in Neurosciences, 11, 195-202.
Rumelhart, D. E. & McClelland, J. L. (1986) On learning the past
tenses of English verbs. In J. L. McClelland, D. E. Rumelhart, & The
PDP Research Group, Parallel distributed processing: Explorations in
the microstructure of cognition. Volume 2: Psychological and
biological models. Cambridge, MA: Bradford Books/MIT Press.
----------------------------------------------------------------
Posted for Pinker & Prince by:
--
Stevan Harnad ARPANET: harnad@mind.princeton.edu harnad@princeton.edu
harnad@confidence.princeton.edu srh@flash.bellcore.com harnad@mind.uucp
BITNET: harnad%mind.princeton.edu@pucc.bitnet UUCP: princeton!mind!harnad
CSNET: harnad%mind.princeton.edu@relay.cs.net
------------------------------
Date: Thu, 1 Sep 88 15:09 EDT
From: Stevan Harnad <harnad@mind.UUCP>
Subject: Pinker & Prince Reply (long version)
Posted for Pinker & Prince by S. Harnad
------------------------------------------------------------------
From: Steve Pinker <steve@cogito.mit.edu>
To: Stevan Harnad (harnad@mind.princeton.edu)
Site: MIT Center for Cognitive Science
Subject: answers to S. Harnad's questions, longer version
This letter is a reply to your posted list of questions and
observations alluding to our paper "On language and connectionism:
Analysis of a PDP model of language acquisition" (Pinker & Prince,
1988; see also Prince and Pinker, 1988). The questions are based on
misunderstandings of our papers, in which they are already answered.
(1) Contrary to your suggestion, we never claimed that pattern
associators cannot learn the past tense rule, or anything else, in
principle. Our concern is with which theories of the psychology of
language are true. This question cannot be answered from an archair
but only by examining what people learn and how they learn it. Our
main conclusion is that the claim that the English past tense rule is
learned and represented as a pattern-associator with distributed
representations over phonological features for input and output forms
(e.g., the Rumelhart-McClelland 1986 model) is false. That's because
what pattern-associators are good at is precisely what the regular
rule doesn't need. Pattern associators are designed to pick up
patterns of correlation among input and output features. The regular
past tense alternation, as acquired by English speakers, is not
systematically sensitive to phonological features. Therefore some of
the failures of the R-M model we found are traceable to its trying to
handle the regular rule with an architecture inappropriate to the
regular rule.
We therefore predict that these failures should be seen in other
network models that compute the regular past tense alternation using
pattern associators with distributed phonological representations
(*not* all conceivable network models, in general, in principle,
forever, etc.). This prediction has been confirmed. Egedi and Sproat
(1988) devised a network model that retained the assumption of
associations between distributed phonological representations but
otherwise differed radically from the R-M model: it had three layers,
not two; it used a back-propagation learning rule, not just the simple
perceptron convergence procedure; it used position-specific
phonological features, not context-dependent ones; and it had a
completely different output decoder. Nonetheless its successes and
failures were virtually identical to those of the R-M model.
(2) You claim that
"the regularities you describe -- both in the
irregulars and the regulars -- are PRECISELY the kinds of
invariances you would expect a statistical pattern
learner that was sensitive to higher order correlations to
be able to learn successfully. In particular, the
form-independent default option for the regulars should be
readily inducible from a representative sample."
This is an interesting claim and we strongly encourage you to back it
up with argument and analysis; a real demonstration of its truth would
be a significant advance. It's certainly false of the R-M and
Egedi-Sproat models. There's a real danger in this kind of glib
commentary of trivializing the issues by assuming that net models are
a kind of miraculous wonder tissue that can do anything. The
brilliance of the Rumelhart and McClelland (1986) paper is that they
studiously avoided this trap. In the section of their paper called
"Learning regular and exceptional patterns in a pattern associator"
they took great pains to point out that pattern associators are good
at specific things, especially exploiting statistical regularities in
the mapping from one set of featural patterns to another. They then
made the interesting emprical claim that these basic properties of the
pattern associator model lie at the heart of the acquisition of the
past tense. Indeed, the properties of the model afforded it some
interesting successes with the *irregular* alternations, which fall
into family resemblance clusters of the sort that pattern associators
handle in interesting ways. But it is exactly these properties of the
model that made it fail at the *regular* alternation, which does not
form family resemblance clusters.
We like to think that these kinds of comparisons make for productive
empirical science. The successes of the pattern associator
architecture for irregulars teaches us something about the psychology
of the irregulars (basically a memory phenomenon, we argue), and its
failures for the regulars teach us something about the psychology of
the regulars (use of a default rule, we argue). Rumelhart and
McClelland disagree with us over the facts but not over the key
emprical tests. They hold that pattern associators have particular
aptitudes that are suited to modeling certain kinds of processes,
which they claim are those of cognition. One can argue for or against
this and learn something about psychology while so doing. Your claim
about a 'statistical pattern learner...sensitive to higher order
correlations' is essentially impossible to evaluate.
(3) We're mystified that you attribute to us the claim that "past
tense formation is not learnable in principle." The implication is
that our critique of the R-M model was based on the assertion that the
rule is unlearned and that this is the key issue separating us from
R&M. Therefore -- you seem to reason -- if the rule is learned, it is
learned by a network. But both parts are wrong. No one in his right
mind would claim that the English past tense rule is "built in". We
spent a full seven pages (130-136) of 'OLC' presenting a simple model
of how the past tense rule might be learned by a symbol manipulation
device. So obviously we don't believe it can't be learned. The
question is how children in fact do it.
The only way we can make sense of this misattribution is to suppose
that you equate "learnable" with "learnable by some (nth-order)
statistical algorithm". The underlying presupposition is that
statistical modeling (of an undefined character) has some kind of
philosophical priority over other forms of analysis; so that if
statistical modeling seems somehow possible-in-principle, then
rule-based models (and the problems they solve) can be safely ignored.
As a kind of corollary, you seem to assume that unless the input is so
impoverished as to rule out all statistical modeling, rule theories
are irrelevant; that rules are impossible without major
stimulus-poverty. In our view, the question is not CAN some (ungiven)
algorithm 'learn' it, but DO learners approach the data in that
fashion. Poverty-of-the-stimulus considerations are one out of many
sources of evidence in this issue. (In the case of the past tense
rule, there is a clear P-of-S argument for at least one aspect of the
organization of the inflectional system: across languages, speakers
automatically regularize verbs derived from nouns and adjectives
(e.g., 'he high-sticked/*high-stuck the goalie'; she braked/*broke the
car'), despite virtually no exposure to crucial informative data in
childhood. This is evidence that the system is built around
representations corresponding to the constructs 'word', 'root', and
'irregular'; see OLC 110-114.)
(4) You bring up the old distinction between rules that describe
overall behavior and rules that are explicitly represented in a
computational device and play a causal role in its behavior. Perhaps,
as you say, "these are not crisp issues, and hence not a solid basis
for a principled critique". But it was Rumelhart and McClelland who
first brought them up, and it was the main thrust of their paper. We
tend to agree with them that the issues are crisp enough to motivate
interesting research, and don't just degenerate into discussions of
logical possibilities. We just disagree about which conclusions are
warranted. We noted that (a) the R-M model is empirically incorrect,
therefore you can't use it to defend any claims for whether or not
rules are explicitly represented; (b) if you simply wire up a network
to do exactly what a rule does, by making every decision about how to
build the net (which features to use, what its topology should be,
etc.) by consulting the rule-based theory, then that's a clear sense
in which the network "implements" the rule. The reason is that the
hand-wiring and tweaking of such a network would not be motivated by
principles of connectionist theory; at the level at which the
manipulations are carried out, the units and connections are
indistinguishable from one another and could be wired together any way
one pleased. The answer to the question "Why is the network wired up
that way?" would come from the rule-theory; for example, "Because the
regular rule is a default operation that is insensitive to stem
phonology". Therefore in the most interesting sense such a network
*is* a rule. The point carries over to more complex cases, where one
would have different subnetworks corresponding to different parts of
rules. Since it is the fact that the network implements such-and-such
a rule that is doing the work of explaining the phenomenon, the
question now becomes, is there any reason to believe that the rule is
implemented in that way rather some other way?
Please note that we are *not* asserting that no PDP model of any sort
could ever acquire linguistic knowledge without directly implementing
linguistic rules. Our hope, of course, is that as the discussion
proceeds, models of all kinds will be become more sophisticated and
ambitious. As we said in our Conclusion, "These problems are exactly
that, problems. They do not demonstrate that interesting PDP models
of language are impossible in principle. At the same time, they show
that there is no basis for the belief that connectionism will dissolve
the difficult puzzles of language, or even provide radically new
solutions to them."
So to answer the catechism:
(a) Do we believe that English past tense formation is not learnable?
Of course we don't!
(b) If it is learnable, is it specifically unlearnable by nets? No,
there may be some nets that can learn it; certainly any net that is
intentionally wired up to behave exactly like a rule-learning
algorithm can learn it. Our concern is not with (the mathematical
question of) what nets can or cannot do in principle, but about which
theories are true, and our analysis was of pattern associators using
distributed phonological representations. We showed that it is
unlikely that human children learn the regular rule the way such a
pattern associator learns the regular rule, because it is simply the
wrong tool for the job. Therefore it's not surprising that the
developmental data confirm that children do not behave the way such a
pattern associator behaves.
(c) If past tense formation is learnable by nets, but only if the
invariance that the net learns and that causally constrains its
successful performance is describable as a "rule", what's wrong with
that? Absolutely nothing! -- just like there's nothing wrong with
saying that past tense formation is learnable by a bunch of
precisely-arranged molecules (viz., the brain) such that the
invariance that the molecules learn, etc. etc. The question is, what
explains the facts of human cognition? Pattern associator networks
have some interesting properties that can shed light on certain kinds
of phenomena, such as irregular past tense forms. But it is simply a
fact about the regular past tense alternation in English that it is
not that kind of phenomenon. You can focus on the interesting
empirical properties of pattern associators, and use them to explain
certain things (but not others), or you can generalize them to a class
of universal devices that can explain nothing without appeals to the
rules that they happen to implement. But you can't have it both ways.
Steven Pinker
Department of Brain and Cognitive Sciences
E10-018
MIT
Cambridge, MA 02139
steve@cogito.mit.edu
Alan Prince
Program in Cognitive Science
Department of Psychology
Brown 125
Brandeis University
Waltham, MA 02254-9110
prince@brandeis.bitnet
References:
Egedi, D.M. and R.W. Sproat (1988) Neural Nets and Natural Language
Morphology, AT&T Bell Laboratories, Murray Hill,NJ, 07974.
Pinker, S. & Prince, A. (1988) On language and connectionism: Analysis
of a parallel distributed processing model of language acquisition.
Cognition, 28, 73-193. Reprinted in S. Pinker & J. Mehler (Eds.),
Connections and symbols. Cambridge, MA: Bradford Books/MIT Press.
Prince, A. & Pinker, S. (1988) Rules and connections in human
language. Trends in Neurosciences, 11, 195-202.
Rumelhart, D. E. & McClelland, J. L. (1986) On learning the past
tenses of English verbs. In J. L. McClelland, D. E. Rumelhart, & The
PDP Research Group, Parallel distributed processing: Explorations in
the microstructure of cognition. Volume 2: Psychological and
biological models. Cambridge, MA: Bradford Books/MIT Press.
-------------------------------------------------------------
Posted for Pinker & Prince by:
--
Stevan Harnad ARPANET: harnad@mind.princeton.edu harnad@princeton.edu
harnad@confidence.princeton.edu srh@flash.bellcore.com harnad@mind.uucp
BITNET: harnad%mind.princeton.edu@pucc.bitnet UUCP: princeton!mind!harnad
CSNET: harnad%mind.princeton.edu@relay.cs.net
------------------------------
Date: Thu, 1 Sep 88 15:13 EDT
From: Stevan Harnad <harnad@mind.UUCP>
Subject: Re: Pinker & Prince Reply (long version)
ON THEFT VS HONEST TOIL
Pinker & Prince (prince@mit.cogito.edu) write in reply:
>> Contrary to your suggestion, we never claimed that pattern associators
>> cannot learn the past tense rule, or anything else, in principle.
I've reread the paper, and unfortunately I still find it ambiguous:
For example, one place (p. 183) you write:
"These problems are exactly that, problems. They do not demonstrate
that interesting PDP models of language are impossible in principle."
But elsewhere (p. 179) you write:
"the representations used in decomposed, modular systems are
abstract, and many aspects of their organization cannot be learned
in any obvious way." [Does past tense learning depend on any of
this unlearnable organization?]
On p. 181 you write:
"Perhaps it is the limitations of these simplest PDP devices --
two-layer association networks -- that causes problems for the
R & M model, and these problems would diminish if more
sophisticated kinds of PDP networks were used."
But earlier on the same page you write:
"a model that can learn all possible degrees of correlation among a
set of features is not a model of a human being" [Sounds like a
Catch-22...]
It's because of this ambiguity that my comments were made in the form of
conditionals and questions rather than assertions. But we now stand
answered: You do NOT claim "that pattern associaters cannot learn the
past tense rule, or anything else, in principle."
[Oddly enough, I do: if by "pattern associaters" you mean (as you mostly
seem to mean) 2-layer perceptron-style nets like the R & M model, then I
would claim that they cannot learn the kinds of things Minsky showed they
couldn't learn, in principle. Whether or not more general nets (e.g., PDP
models with hidden layers, back-prop, etc.) will turn out to have corresponding
higher-order limitations seems to be an open question at this point.]
You go on to quote my claim that:
"the regularities you describe -- both in the
irregulars and the regulars -- are PRECISELY the kinds of
invariances you would expect a statistical pattern
learner that was sensitive to higher order correlations to
be able to learn successfully. In particular, the
form-independent default option for the regulars should be
readily inducible from a representative sample."
and then you comment:
>> This is an interesting claim and we strongly encourage you to back it
>> up with argument and analysis; a real demonstration of its truth would
>> be a significant advance. It's certainly false of the R-M and
>> Egedi-Sproat models. There's a real danger in this kind of glib
>> commentary of trivializing the issues by assuming that net models are
>> a kind of miraculous wonder tissue that can do anything.
I don't understand the logic of your challenge. You've disavowed
having claimed that any of this was unlearnable in principle. Why is it
glibber to conjecture that it's learnable in practice than that it's
unlearnable in practice? From everything you've said, it certainly
LOOKS perfectly learnable: Sample a lot of forms and discover that the
default regularity turns out to work well in most cases (i.e., the
"regulars"; the rest, the "irregulars," have their own local invariances,
likewise inducible from statistical regularities in the data).
This has nothing to do with a belief in wonder tissue. It was precisely
in order to avoid irrelevant stereotypes like that that the first
posting was prominently preceded by the disclaimer that I happen to be
a sceptic about connectionism's actual accomplishments and an agnostic
about its future potential. My critique was based solely on the logic of
your argument against connectionism (in favor of symbolism). Based
only on what you've written about its underlying regularities, past
tense rule learning simply doesn't seem to pose a serious challenge for a
statistical learner -- not in principle, at any rate. It seems to have
stumped R & M 86 and E & S 88 in practice, but how many tries is
that? It is possible, for example, as suggested by your valid analysis of
the limitations of the Wickelfeature representation, that some of the
requisite regularities are simply not reflected in this phonological
representation, or that other learning (e.g. plurals) must complement
past-tense data. This looks more like an entry-point problem
(see (1) below), however, rather than a problem of principle for
connectionist learning of past tense formation. After all, there's no
serious underdetermination here; it's not like looking for a needle in
a haystack, or NP-complete, or like that.
I agree that R & M made rather inflated general claims on the basis of
the limited success of R & M 86. But (to me, at any rate) the only
potentially substantive issue here seems to be the one of principle (about
the relative scope and limits of the symbolic vs. the connectionistic
approach). Otherwise we're all just arguing about the scope and limits
of R & M 86 (and perhaps now also E & S 88).
Two sources of ambiguity seem to be keeping this disagreement
unnecessarily vague:
(1) There is an "entry-point" problem in comparing a toy model (e.g.,
R & M 86) with a lifesize cognitive capacity (e.g., the human ability
to form past tenses): The capacity may not be modular; it may depend on
other capacities. For example, as you point out in your article, other
phonological and morphological data and regularities (e.g.,
pluralization) may contribute to successful past tense formation. Here
again, the challenge is to come up with a PRINCIPLED limitation, for
otherwise the connectionist can reasonably claim that there's no reason
to doubt that those further regularities could have been netted exactly
the same way (if they had been the target of the toy model); the entry
point just happened to be arbitrarily downstream. I don't say this
isn't hand-waving; but it can't be interestingly blocked by hand-waving
in the opposite direction.
(2) The second factor is the most critical one: learning. You
put a lot of weight on the idea that if nets turn out to behave
rulefully then this is a vindication of the symbolic approach.
However, you make no distinction between rules that are built in (as
"constraints," say) and rules that are learned. The endstate may be
the same, but there's a world of difference in how it's reached -- and
that may turn out to be one of the most important differences between
the symbolic approach and connectionism: Not whether they use
rules, but how they come by them -- by theft or honest toil. Typically,
the symbolic approach builds them in, whereas the connectionistic one
learns them from statistical regularities in its input data. This is
why the learnability issue is so critical. (It is also what makes it
legitimate for a connectionist to conjecture, as in (1) above, that if
a task is nonmodular, and depends on other knowledge, then that other
knowledge too could be acquired the same way: by learning.)
>> Your claim about a 'statistical pattern learner...sensitive to higher
>> order correlations' is essentially impossible to evaluate.
There are in principle two ways to evaluate it, one empirical and
open-ended, the other analytical and definitive. You can demonstrate
that specific regularities can be learned from specific data by getting
a specific learning model to do it (but its failure would only be evidence
that that model fails for those data). The other way is to prove analytically
that certain kinds of regularities are (or are not) learnable from
certain kinds of data (by certain means, I might add, because
connectionism may be only one candidate class of statistical learning
algorithms). Poverty-of-the-stimulus arguments attempt to demonstrate
the latter (i.e., unlearnability in principle).
>> We're mystified that you attribute to us the claim that "past
>> tense formation is not learnable in principle."... No one in his right
>> mind would claim that the English past tense rule is "built in". We
>> spent a full seven pages (130-136) of 'OLC' presenting a simple model
>> of how the past tense rule might be learned by a symbol manipulation
>> device. So obviously we don't believe it can't be learned.
Here are some extracts from OLC 130ff:
"When a child hears an inflected verb in a single context, it is
utterly ambiguous what morphological category the inflection is
signalling... Pinker (1984) suggested that the child solves this
problem by "sampling" from the space of possible hypotheses defined
by combinations of an innate finite set of elements, maintaining
these hypotheses in the provisional grammar, and testing them
against future uses of that inflection, expunging a hypothesis if
it is counterexemplified by a future word. Eventually... only
correct ones will survive." [The text goes on to describe a
mechanism in which hypothesis strength grows with success frequency
and diminishes with failure frequency through trial and error.]
"Any adequate rule-based theory will have to have a module that
extracts multiple regularities at several levels of generality,
assign them strengths related to their frequency of exemplification
by input verbs, and let them compete in generating a past tense for
for a given verb."
It's not entirely clear from the description on pp. 130-136 (probably
partly because of the finessed entry-point problem) whether (i) this is an
innate parameter-setting or fine-tuning model, as it sounds, with the
"learning" really just choosing among or tuning the built-in parameter
settings, or whether (ii) there's genuine bottom-up learning going on here.
If it's the former, then that's not what's usually meant by "learning."
If it's the latter, then the strength-adjusting mechanism sounds equivalent
to a net, one that could just as well have been implemented nonsymbolically.
(You do state that your hypothetical module would be equivalent to R & M's in
many respects, but it is not clear how this supports the symbolic approach.)
[It's also unclear what to make of the point you add in your reply (again
partly because of the entry-point problem):
>>"(In the case of the past tense rule, there is a clear P-of-S argument
for at least one aspect of the organization of the inflectional system...)">>
Is this or is this not a claim that all or part of English past tense
formation is not learnable (from the data available to the child) in
principle? There seems to be some ambiguity (or perhaps ambivalence) here.]
>> The only way we can make sense of this misattribution is to suppose
>> that you equate "learnable" with "learnable by some (nth-order)
>> statistical algorithm". The underlying presupposition is that
>> statistical modeling (of an undefined character) has some kind of
>> philosophical priority over other forms of analysis; so that if
>> statistical modeling seems somehow possible-in-principle, then
>> rule-based models (and the problems they solve) can be safely ignored.
Yes, I equate learnability with an algorithm that can extract
statistical regularities (possibly nth order) from input data.
Connectionism seems to be (an interpretation of) a candidate class of
such algorithms; so does multiple nonlinear regression. The question of
"philosophical priority" is a deep one (on which I've written:
"Induction, Evolution and Accountability," Ann. NY Acad. Sci. 280,
1976). Suffice it to say that induction has epistemological priority
over innatism (or such a case can be made) and that a lot of induction
(including hypothesis-strengthening by sampling instances) has a
statistical character. It is not true that where statistical induction
is possible, rule-based models must be ignored (especially if the
rule-based models learn by what is equivalent to statistics anyway),
only that the learning NEED not be implemented symbolically. But it is
true that where a rule can be learned from regularities in the data,
it need not be built in. [Ceterum sentio: there is an entry-point
problem for symbols that I've also written about: "Categorical
Perception," Cambr. U. Pr. 1987. I describe there a hybrid approach in
in which symbolic and nonsymbolic representations, including a
connectionistic component, are put together bottom-up in a principled
way that avoids spuriously pitting connectionism against symbolism.]
>> As a kind of corollary, you seem to assume that unless the input is so
>> impoverished as to rule out all statistical modeling, rule theories
>> are irrelevant; that rules are impossible without major stimulus-poverty.
No, but I do think there's an entry-point problem. Symbolic rules can
indeed be used to implement statistical learning, or even to preempt it, but
they must first be grounded in nonsymbolic learning or in innate
structures. Where there is learnability in principle, learning does
have "philosophical (actually methodological) priority" over innateness.
>> In our view, the question is not CAN some (ungiven) algorithm
>> 'learn' it, but DO learners approach the data in that fashion.
>> Poverty-of-the-stimulus considerations are one out of many
>> sources of evidence in this issue...
>> developmental data confirm that children do not behave the way such a
>> pattern associator behaves.
Poverty-of-the-stimulus arguments are the cornerstone of modern
linguistics because, if they are valid, they entail that certain
rules (or constraints) are unlearnable in principle (from the data
available to the child) and hence that a learning model must fail for
such cases. The rule system itself must accordingly be attributed to
the brain, rather than just the general-purpose inductive wherewithal
to learn the rules from experience.
Where something IS learnable in principle, there is of course still a
question as to whether it is indeed learned in practice rather than
being innate; but neither (a) the absence of data on whether it is learned
nor (b) the existence of a rule-based model that confers it on the child
for free provide very strong empirical guidance in such a case. In any
event, developmental performance data themselves seem far too
impoverished to decide between rival theories at this stage. It seems
advisable to devise theories that account for more lifesize chunks of our
asymptotic (adult) performance capacity before trying to fine-tune them
with developmental (or neural, or reaction-time, or brain-damage) tests
or constraints. (Standard linguistic theory has in any case found it
difficult to find either confirmation or refutation in developmental
data to date.)
By way of a concrete example, suppose we had two pairs of rival toy
models, symbolic vs. connectionistic, one pair doing chess-playing and
the other doing factorials. (By a "toy" model I mean one that models
some arbitrary subset of our total cognitive capacity; all models to
date, symbolic and connectionistic, are toy models in this sense.) The
symbolic chess player and the connectionistic chess player both
perform at the same level; so do the symbolic and connectionistic
factorializer. It seems evident that so little is known about how people
actually learn chess and factorials that "developmental" support would
hardly be a sound basis for choosing between the respective pairs of models
(particularly because of the entry-point problem, since these skills
are unlikely to be acquired in isolation). A much more principled way
would be to see how they scaled up from this toy skill to more and
more lifesize chunks of cognitive capacity. (It has to be conceded,
however, that the connectionist models would have a marginal lead in
this race, because they would already be using the same basic
[statistical learning] algorithm for both tasks, and for all future tasks,
presumably, whereas the symbolic approach would have to be making its
rules on the fly, an increasingly heavy load.)
I am agnostic about who would win this race; connectionism may well turn
out to be side-lined early because of a higher-order Perceptron-like limit
on its rule-learning ability, or because of principled unlearnability
handicaps. Who knows? But the race is on. And it seems obvious that
it's far too early to use developmental (or neural) evidence to decide
which way to bet. It's not even clear that it will remain a 2-man race
for long -- or that a finish might not be more likely as a
collaborative relay. (Nor is the one who finishes first or gets
farthest guaranteed to be the "real" winner -- even WITH developmental
and neural support. But that's just normal underdetermination.)
>> if you simply wire up a network to do exactly what a rule does, by
>> making every decision about how to build the net (which features to
>> use, what its topology should be, etc.) by consulting the rule-based
>> theory, then that's a clear sense in which the network "implements"
>> the rule
What if you don't WIRE it up but TRAIN it up? That's the case at
issue here, not the one you describe. (I would of course agree that if
nets wire in a rule as a built-in constraint, that's theft, not
honest toil, but that's not the issue!)
--
Stevan Harnad ARPANET: harnad@mind.princeton.edu harnad@princeton.edu
harnad@confidence.princeton.edu srh@flash.bellcore.com harnad@mind.uucp
BITNET: harnad%mind.princeton.edu@pucc.bitnet UUCP: princeton!mind!harnad
CSNET: harnad%mind.princeton.edu@relay.cs.net
------------------------------
Date: Fri, 2 Sep 88 15:06 EDT
From: Stevan Harnad <harnad@mind.UUCP>
Subject: Re: Pinker & Prince Reply (long version)
Posted for Pinker & Prince [pinker@cogito.mit.edu] by S. Harnad
--------------------------------------------------------------
In his reply to our answers to his questions, Harnad writes:
-Looking at the actual behavior and empirical fidelity of
connectionist models is not the right way to test
connectionist hypotheses;
-Developmental, neural, reaction time, and brain-damage data
should be put aside in evaluating psychological theories.
-The meaning of the word "learning" should be stipulated to
apply only to extracting statistical regularities
from input data.
-Induction has philosophical priority over innatism.
We don't have much to say here (thank God, you are probably all
thinking). We disagree sharply with the first two claims, and have no
interest whatsoever in discussing the last two.
Alan Prince
Steven Pinker
----------------------------------------------------------------------
Posted for Pinker & Prince by:
--
Stevan Harnad ARPANET: harnad@mind.princeton.edu harnad@princeton.edu
harnad@confidence.princeton.edu srh@flash.bellcore.com harnad@mind.uucp
BITNET: harnad%mind.princeton.edu@pucc.bitnet UUCP: princeton!mind!harnad
CSNET: harnad%mind.princeton.edu@relay.cs.net
------------------------------
Date: Sat, 3 Sep 88 16:06 EDT
From: Stevan Harnad <harnad@mind.UUCP>
Subject: Re: Pinker & Prince Reply (On Modeling and Its Constraints)
Pinker & Prince attribute the following 4 points (not quotes) to me,
indicating that they sharply disgree with (1) and (2) and have no
interest whatsoever in discussing (3) and (4).:
(1) Looking at the actual behavior and empirical fidelity of connectionist
models is not the right way to test connectionist hypotheses.
This was not the issue, as any attentive follower of the discussion
can confirm. The question was whether Pinker & Prince's article was to
be taken as a critique of the connectionist approach in principle, or
just of the Rumelhart & McClelland 1986 model in particular.
(2) Developmental, neural, reaction time, and brain-damage data should be
put aside in evaluating psychological theories.
This was a conditional methodological point; it is not correctly stated
in (2): IF one has a model for a small fragment of human cognitive
performance capacity (a "toy" model), a fragment that one has no reason
to suppose to be functionally self-contained and independent of the
rest of cognition, THEN it is premature to try to bolster confidence in
the model by fitting it to developmental (neural, reaction time, etc.)
data. It is a better strategy to try to reduce the model's vast degrees of
freedom by scaling up to a larger and larger fragment of cognitive
performance capacity. This certainly applies to past-tense learning
(although my example was chess-playing and doing factorials). It also
seems to apply to all cognitive models proposed to date. "Psychological
theories" will begin when these toy models begin to approach lifesize;
then fine-tuning and implementational details may help decide between
asymptotic rivals.
[Here's something for connectionists to disagree with me about: I don't
think there is a solid enough fact known about the nervous system
to warrant "constraining" cognitive models with it. Constraints are
handicaps; what's needed in the toy world that contemporary modeling
lives in is more power and generality in generating our performance
capacities. If "constraints" help us to get that, then they're useful
(just as any source of insight, including analogy and pure fantasy can
be useful). Otherwise they are just arbitrary burdens. The only
face-valid "constraint" is our cognitive capacity itself, and we all
know enough about that already to provide us with competence data
till doomsday. Fine-tuning details are premature; we haven't even come
near the station yet.]
(3) The meaning of the word "learning" should be stipulated to apply
only to extracting statistical regularities from input data.
(4) Induction has philosophical priority over innatism.
These are substantive issues, very relevant to the issues under discussion
(and not decidable by stipulation). However, obviously, they can only be
discussed seriously with interested parties.
--
Stevan Harnad ARPANET: harnad@mind.princeton.edu harnad@princeton.edu
harnad@confidence.princeton.edu srh@flash.bellcore.com harnad@mind.uucp
BITNET: harnad%mind.princeton.edu@pucc.bitnet UUCP: princeton!mind!harnad
CSNET: harnad%mind.princeton.edu@relay.cs.net
------------------------------
Date: Wed, 7 Sep 88 02:10 EDT
From: Stevan Harnad <harnad@mind.UUCP>
Subject: Re: Pinker & Prince: The final remark...
Posted for Pinker & Prince by S. Harnad:
__________________________________________________________________
From: Alan Prince <prince@cogito.mit.edu>
Cc: steve@ATHENA.MIT.EDU
Here is a final remark from us. I've posted it to connectionists and will
leave it to your good offices to handle the rest of the branching factor.
Thanks, Alan Prince.
``The Eye's Plain Version is a Thing Apart''
Whatever the intricacies of the other substantive issues that
Harnad deals with in such detail, for him the central question
must always be: "whether Pinker & Prince's article was to be taken
as a critique of the connectionist approach in principle, or just of
the Rumelhart & McClelland 1986 model in particular" (Harnad 1988c, cf.
1988a,b).
At this we are mildly abashed: we don't understand the continuing
insistence on exclusive "or". It is no mystery that our paper
is a detailed analysis of one empirical model of a corner (of a
corner) of linguistic capacity; nor is it obscure that from time
to time, when warranted, we draw broader conclusions (as in section 8).
Aside from the 'ambiguities' arising from Harnad's humpty-dumpty-ish
appropriation of words like 'learning', we find that the two modes
of reasoning coexist in comfort and symbiosis. Harnad apparently
wants us to pledge allegiance to one side (or the other) of a phony
disjunction. May we politely refuse?
S. Pinker
A. Prince
______________________________________________________________
Posted for Pinker & Prince by:
--
Stevan Harnad ARPA/INTERNET: harnad@mind.princeton.edu harnad@princeton.edu
harnad@confidence.princeton.edu srh@flash.bellcore.com harnad@mind.uucp
CSNET: harnad%mind.princeton.edu@relay.cs.net UUCP: princeton!mind!harnad
BITNET: harnad@pucc.bitnet harnad@pucc.princeton.edu (609)-921-7771
------------------------------
End of Stevan Harnad Digest
*******************