Copy Link
Add to Bookmark
Report
Machine Learning List Vol. 6 No. 21
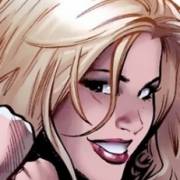
Machine Learning List: Vol. 6 No. 21
Friday, July 29, 1994
Contents:
On-line working notes available
addition to CFP: AIJ Special Issue Devoted to Empirical AI
UCI - ML - WWW
PhD on Bayesian methods in ML (Glasgow)
Conservation law (6)
Clarification: Conservation Law
A comment on Hunter's comment on Schaffer's Law
Re: A comment on Hunter's comment on Schaffer's Law
Generalization and Kolmogorov Complexity
A paradox related to the Conservation Law
The Machine Learning List is moderated. Contributions should be relevant to
the scientific study of machine learning. Mail contributions to ml@ics.uci.edu.
Mail requests to be added or deleted to ml-request@ics.uci.edu. Back issues
may be FTP'd from ics.uci.edu in pub/ml-list/V<X>/<N> or N.Z where X and N are
the volume and number of the issue; ID: anonymous PASSWORD: <your mail address>
URL- http://www.ics.uci.edu/AI/ML/Machine-Learning.html
----------------------------------------------------------------------
Date: Tue, 26 Jul 94 16:00:08 EDT
From: Tom Fawcett <fawcett@nynexst.com>
Subject: On-line working notes available
Electronic working notes are now available for the ML-COLT '94
Workshop on Constructive Induction and Change of Representation. This
workshop was held on July 10 in conjunction with the combined Machine
Learning and Computational Learning Theory conference at Rutgers.
The working notes are available through the World-Wide Web. The URL is:
http://cobar.cs.umass.edu/mlc94/CICR.html
Titles, abstracts and Postscript copies of the papers are available
through this page.
Thanks to Jamie Callan at UMass/Amherst for making space available for
these pages.
------------------------------
Date: Tue, 26 Jul 94 14:57:14 -0500
From: David Hart <dhart@cs.umass.edu>
Subject: addition to CFP: AIJ Special Issue Devoted to Empirical AI
The CFP for a special issue of AIJ devoted to empirical AI
posted yesterday suggested that potential authors contact
the editors, but unfortunately omitted their addresses. The
editors are Paul Cohen (cohen@cs.umass.edu) and Bruce Porter
(porter@cs.utexas.edu).
------------------------------
Subject: UCI - ML - WWW
Date: Wed, 27 Jul 1994 16:04:19 -0700
From: Tim Hume <hume@ruffles.ICS.UCI.EDU>
******************************************
** University of California, Irvine **
** **
** Machine Learning **
** **
** now available through WWW **
******************************************
The Machine Learning group of the Department of Information and
Computer Science at the University of California - Irvine now has
information available via WWW. The URL is
http://www.ics.uci.edu/AI/ML/Machine-Learning.html
From here you can access UCI's repository of databases for machine
learning research, digests of the Machine Learning List, programs
(FOCL, Occam, and HYDRA) developed at UCI, and papers by authors from UCI.
The Machine Learning List digests are searchable, and we plan to have
the repository searchable later this year.
Comments and suggestions for improvements are welcome. Assistance
in making the suggested improvements is also welcome. Please send
correspondence to
Tim Hume
hume@ics.uci.edu
UC, Irvine
------------------------------
From: "J.Cussens" <jcu1@glasgow-caledonian.ac.uk>
Date: Thu, 28 Jul 94 16:39:39 BST
Subject: PhD on Bayesian methods in ML (Glasgow)
Dear All,
Is anyone interested in, or know anyone else who is interested in the
following PhD studentship which is available here in Glasgow?
Regards,
James Cussens
********************************************************************
GLASGOW CALEDONIAN UNIVERSITY
SOFTWARE MEASUREMENT LABORATORY (SML), DEPT. OF MATHEMATICS
RESEARCH STUDENTSHIP IN MACHINE LEARNING
Applications are invited for a PhD studentship in the area of
`Applying Bayesian Statistics to Inductive Learning'. The post is
available from October 1994, but could start several months later, and
will last for three years. The studentship will be supervised by
James Cussens.
Possible research topics include, but are not restricted to:
(1) The role of Bayesian statistics in unifying Knowledge Acquisition
and Machine Learning.
(2) Applying Bayesian Statistics to Inductive Logic Programming.
(3) Bayesian approaches to handling noisy and incomplete data in
Machine Learning.
(4) Bayesian approaches to learning control rules for dynamic
environments.
Applicants should have a good first degree (at least an upper second)
in either Mathematics, Statistics or Computer Science. Knowledge of
Bayesian statistics is essential. Knowledge of machine learning would
be an advantage, but is not essential.
Further details, including information on machine learning research
at the SML are available from James Cussens.
Informal discussions are welcome. Applicants should send a brief CV
and an outline of the line of research in which they are interested.
Email is the preferred means of communication, but post, phone or fax
is also OK.
Send all communications to:
James Cussens, Software Measurement Laboratory, Glasgow Caledonian
University, Cowcaddens Rd, Glasgow, G4 0BA, UK
EMAIL: j.cussens@gcal.ac.uk
TEL: +44 41 331 3329 (direct line)
FAX: +44 41 331 3171
------------------------------
Date: Mon, 25 Jul 94 22:59:38 EDT
From: gordon@aic.nrl.navy.mil
Subject: Conservation law
1) We believe Schaffer's Conservation Law is correct, and is a more
rigorous reminder of statements that have been made already by
Utgoff, Mitchell, Gordon, Dietterich, etc. Even the GA community
has been known to say "Furthermore, there is no `universal' bias
that facilitates induction across all problem domains" (Booker, 1992).
2) However, we have serious doubts about its usefulness. This law appears
to be essentially a counting argument, where we count over all situations
S. Thus, this law says something about the real world if all possible
situations occur, and if all possible situations occur with
equal probability. In that case, then, this law would be 100%
predictive of generalization performance. The law becomes less
predictive as the real world deviates from a uniform distribution over
all situations. Schaffer states the analogy between this law and physical
laws of conservation. To continue this analogy, we should remember there
are many other physical laws, and most of them actually preclude many
hypothetical situations in the world as we understand it (e.g., we can
typically ignore situations where speed exceeds the speed of light, or
situations where temperature is lower than 0 Kelvin). Furthermore, our
conceptualization of the world imposes even more constraints.
A more interesting and useful statement would concern the generalization
performance over problems that actually occur in the world.
Unfortunately, this is a much more difficult task. Rendell (1986)
suggests a useful framework for practical induction, but much more needs
to be done in this area.
3) What *does* appear useful from Schaffer's paper is the reminder that
generalization is unlikely to succeed without prior information to
help bias the learner in a good direction.
4) The conservation law should not discourage research in bias and induction.
Since it is highly unlikely that all situations will occur uniformly, some
biases can in fact yield a net positive generalization performance.
It seems apparent to us that only a uniform distribution over all possible
situations would make the counting argument completely true in the world.
The further from uniform, the less applicable the law seems to be. We have
stressed this because it does not appear to be stressed at all in the ML94
paper, yet it is a crucial consideration in gauging the relevance of the
law to the world. However, we'd be glad to be corrected if we are wrong in
concluding this.
By the way, there is a special MLJ issue on "Bias Evaluation and Selection"
coming out soon with some excellent papers on methods for evaluating biases
in common learning paradigms (ILP, speedup learning, concept learning, etc.).
Diana F. Gordon
William M. Spears
------------------------------
From: Tom Dietterich <tgd@chert.cs.orst.edu>
Date: Tue, 26 Jul 94 07:08:17 PDT
Subject: Conservation law
Cullen accuses me of "slipping up" and "not being careful":
Second, Tom slips up too, in his note. After agreeing that general
purpose learning is impossible, he goes on say that, however,
We never apply machine learning algorithms to data unless we believe
there is a pattern in that data.
This statement--though often paraphrased in arguments regarding the
conservation law--is highly misleading. The point is not whether
there is a pattern in the data, but whether it is one of the ones our
algorithms are biased toward guessing. I'm sure Tom understands this
distinction completely, when he takes the time to be careful.
Nevertheless, like a Freudian slip, the particular way his informal
statement strays from the key point suggests that, when he is not being
careful, Tom falls into the same trap as the rest of us--assuming that
the simple fact of an underlying regularity is enough to justify the
generalization biases of standard induction algorithms.
I'll let the reader judge.
The conservation law is averaging out the performance of a learning
algorithm over all possible target concepts (and distributions). This
is equivalent (as Mike Kearns observed at ML94) to permitting the
teacher to label the test set examples by flipping a coin. I think we
can all agree that data sets constructed this way do not contain any
"pattern".
This is not the situation we face in real applications or that humans
face in daily life. That is why I don't lose sleep worrying about the
conservation law.
Cullen is correct that the mere fact that the data are not random does
not mean that any particular learning algorithm will succeed. But it
does mean that *some* learning algorithm will succeed. One goal of
machine learning research is to study classes of learning problems and
find algorithms that will succeed on those problems.
Machine learning research has been particularly interested in very
broad classes of learning problems. These broad classes are
characterized by weak assumptions about the underlying patterns (e.g.,
knowing the class of example x tells us the class of most "nearby"
examples x', where "nearby" is measured by Euclidean distance in
feature space). Interestingly, even weak assumptions of this sort are
sufficient, from a theoretical perspective, to permit learning. And
of course algorithms embodying these assumptions work quite well on a
wide range of real problems, which provides evidence that these
assumptions hold for these problems.
This is where Cullen starts to feel uncomfortable. He can prove that
there are many many more target concepts that violate such weak
assumptions than there are target concepts that satisfy them. He
wants us to worry about all of those other target concepts. I *do*
worry about *some* of them, because on occasion I have encountered a
learning problem where algorithms such as nearest neighbor and C4.5 do
not do as well as I would like (although they do better than random
guessing). This pushes me to shift my weak assumptions so that they
match better the target concepts of interest. But I do not worry
about most of the other target concepts, because I believe that our
weak assumptions hold in most situations.
So I urge researchers who seek "general learning algorithms" to ask
themselves the following question: "What is the weakest assumption
that I am willing to make about the target concept?" You can then
apply computational learning theory to determine whether learning is
possible under this assumption. If the answer is "yes", you can then
try to develop an efficient algorithm that implements this assumption
as an inductive bias.
When you apply your algorithm, the conservation law should encourage
you to identify two kinds of target concepts:
(a) target concepts that your algorithm cannot learn (but that arise
in practice), and
(b) target concepts that your algorithm does learn (but that don't
arise in practice).
You should then modify your assumptions to include (a) and exclude (b)
and repeat the process. [I think that target concepts of type (b) are
interesting, because prior to Cullen's paper, I'm not sure I would
have worried as much about them as I would have about target concepts
of type (a).]
Three notes in closing:
1. Assessing what target concepts arise in practice is a major
challenge. We need much more research in applications and in
human learning to determine this.
2. This process of "assumption adjustment" will only converge if the
target concepts that arise in practice do indeed satisfy some
sufficiently strong set of assumptions. That is a big open question!
3. Adopting general, weak assumptions will not usually give the best
performance on any particular learning problem. Better
performance will be obtained by adopting stronger assumptions
based on knowledge of the particular learning problem. There is
a tradeoff between generality (of the learning algorithm), accuracy,
and sample size.
------------------------------
Date: Tue, 26 Jul 94 10:10:39 -0400
From: Larry Hunter <hunter@work.nlm.nih.gov>
Subject: Conservation Law
Clearly, Cullen and I do agree more than we disagree. Although my
paraphrase of his result may be weaker than the law, his statement is too
strong. We may be picking at nits here, but let me try to clarify what the
differences are.
In response to my comment
Schaffer's Law states that there is no perfect learning algorithm for all
learning tasks.
You said
In fact, this is a much weaker statement, since it leaves open the
possibility of induction algorithms that are, if not perfect everywhere,
at least considerably better than chance for a large majority of all
learning tasks. The conservation law imposes much stricter limits on what
can be achieved.
Now there are infinitely many distributions of generalizations over learning
tasks in which the sum of generalizations is zero. It is possible for a
large number of the elements of that sum to be "considerably better than
chance" and it to still sum to zero. It is certainly possible for an
algorithm to generalize considerably better than chance on a majority of
learning tasks, so long as its generalization performance on the remaining
minority of tasks is enough poorer to balance out the "good" performance.
If the "considerably" in the "considerably better than chance" and the
"large" in the "large majority" both get big enough, then even zero
generalization on all of the remaining tasks will not be enough to force the
sum to zero, but your law is certainly compatible with the idea that there
are learning methods that work well on large classes of (distributions of)
problems.
My advice to the community is not to get discouraged by this or any similar
theoretical result (e.g. Wolpert's). The class of problems (and problem
distributions) in which some learning system can generalize effectively is
extremely large. I stand by the suggestion that humans are an existance
proof of the possibility of "considerably better than chance" generalization
in the large number of complex and difficult learning tasks that tend to
appear in our world. To strengthen this practical point, let me also
suggest that existing ML methods have already demonstrated considerably
better than chance generalization on important classes of learning problems
that are practically significant. My own experience is in applying these
tools to molecular biology, where scientifically important contributions
include the current best methods for protein structure prediction (neural
networks), multiple gene sequence alignment (Viterbi fitted HMMs) and
finding genes in anonymous DNA sequence (learning how to combine noisy
sensors). The fact that there are many other problems on which these
methods do not work does not reduce the significance of their effectiveness
when they do.
------------------------------
From: Tom Bylander <bylander@trurl>
Date: Tue, 26 Jul 1994 11:06:21 +0000 (BST)
Subject: Conservation Law
Tom Dietterich points out that one line of research should be that:
* we must understand the range of applicability of our current
algorithms (what assumptions do they make? How can we decide in
advance whether the target hypothesis is likely to be found by the
algorithm?)
I think one broad, common class of learning problems is when the
attributes are roughly "evidential" in nature. That is, each
attribute can be interpreted as evidence for or against the concept
independent of other attributes, depending on the attribute's value.
How much for or against might be context-dependent.
The information gain and similar decision tree splitting heuristics
depend on this property because in some sense they try to decide which
attribute will provide the most evidence at each node. Holte's 1R
algorithm hopes there is one attribute that is especially good.
Perceptrons and the like will also be good for this kind of problem.
I don't think it is surprising that many of the standard datasets have
this property. To construct a dataset, one must decide what the
attributes are. I think it is natural to prefer attributes that are
evidential.
For harder problems, no attribute by itself is evidential (or few
are), but combinations of attributes are. So we have to search over
possible combinations. E.g., within neural networks, hidden units
correspond to weighted combinations of attributes; note that the
hidden units are then linearly combined.
Of course, the combinations can become arbitrarily complicated, and so
can learning. Read about (or remind yourself of) the concept of order
in Minsky and Papert's Perceptrons book. I think the same idea can be
applied to any learning algorithm. E.g., in TDIDT, order corresponds
to lookahead (or the complexity of the predicate at each node), and
the typical algorithm only does 1-lookahead. So to construct lots of
learning problems where information loss is the right decision tree
splitting heuristic, pick a predicate on a small combination of
attributes for which information gain gets you nowhere (order = size
of combination). Now make all the other attributes weakly evidential
(order = 1). The information gain heuristic will inevitably prefer
the weak attributes; information loss will stumble onto the target.
In some sense, perceptrons or TDIDT using information gain are indeed
general-purpose learning algorithms. It's the order of the learning
problem that makes life difficult.
------------------------------
Date: Tue, 26 Jul 94 12:35:27 MDT
From: David Wolpert <dhw@aztec.santafe.edu>
Subject: Conservation Law
I'd like to second a lot of the comments Cullen posted concerning the
no-free-lunch/conservation results he and I have been pushing. In
particular, I agree wholeheartedly with his pointing out how Larry and
Tom (like all the rest of us!) tend to discuss these issues in ways
that are variance with the basic results.
There are lots of other rather trenchant points in Cullen's
posting. I'd like to extend some of them a bit, to draw attention to
certain subtleties in Tom Dietterich's message.
The basic problem with Tom is that he knows too much! There are a
number of issues with which I know he's familiar, but which I think
most researchers in ML are unaware of. Yet understanding those issues
is crucial to fully appreciate the context of some of what Tom wrote.
***
Tom writes that
>>>
A learning
algorithm must "place its bets" on some space of hypotheses. If the
target concept is in (or near) this space, it will do well, otherwise
it will do poorly (worse than random).
>>>
This is true in a general sense, but glosses over some important
subtleties. For example, it isn't hard to construct scenarios in which
1) If one uses the Bayes-optimal learning algorithm (i.e., the best
you can possibly do), with no noise, and perfect knowledge of the
correct prior;
2) As one gets more data, off-training set misclassification cost
*rises*, on average. (I.e., the learning curve gets worse.)
The point is not that it isn't generally good to have target concepts
"in or near" the space of hypotheses; as Tom points out, such
correspondence between target and hypothesis is usually a good
idea. Rather the point is that there are many subtle issues involved
once one switches to an off-training set error function, even if there
is the correspondence Tom advocates.
>>>
Yes, of course the law is correct. Furthermore, it is just a
corollary of the results from PAC learning theory.
>>>
The second statement isn't exactly true, and *how* it isn't true is
important. Here I'll list 3 such ways that the statement falls short:
1) The most important way in which the statement is misleading is that
PAC tells us that for a big concept class, small data set, etc., we
have no *assurances* about good generalization. In contrast, the
no-free-lunch/conservation results provide assuraces that there
*isn't* good generalization. This is much more powerful statement.
2) Another important point is that PAC is quite limited in its
theoretical view of the world, whereas the no-free-lunch/conservation
results hold in a very broad context.
For example, PAC averages over data, and (in one guise) fixes the
target. However in practice we only have 1 data set in front of us,
and know what it is. Moreover, in practice, we know rather little
about the target. (In particular, in the real world we rarely know
that a target definitely - with probability 1.000000 - falls in some
particular concept class.) Getting around this problem by going to a
this-data case from an average-data case starts taking us over to the
Bayesian framework. And the no-free-lunch/conservation results apply
there as well. (The results also apply for most other modifications of
the basic PAC framework.)
3) A third problem is that the PAC assuraces simply do not apply for
off-training set error, even if one does operate in an average-data
scenario. The difficulty is that PAC's error measure is iid rather
than off-training set.
In other words, if one is interested in off-training set error, then
although PAC's pessimisms (might) still apply, its optimisms do not,
in general.
***
This third issue is in fact one of the major points of the
no-free-lunch/conservation results; that previously widely accepted
optimisms (both theoretical and heuristic) often do not apply if one
switches to an off-training set error function. Two prominent
examples, which I think might even give Tom some pause:
1) In VC theory, one need not make any a priori assumptions about how
well the hypothesis class matches the target function. (The kind of
assumptions Tom advocates.) That theory says, *in a certain sense*,
that so long as your hypothesis class is small, if it matches the data
set, you can have confidence that it is a good fit to the target.
The no-free-lunch/conservation results show us that as far as
off-training set behavior is concerned, this is false (if one is
concerned with expected cost rather than confidence intervals, etc.).
Investigating how this is possible given the VC theorems sheds much
light on those theorems.
2) *Everyone* (me included!) uses cross-validation. And people do so
w/o any particular explicit assumptions about hypothesis classes
matching targets or the like. (In fact, it is often used to choose a
hypothesis class/model.)
The no-free-lunch/conservation results show that averaged over all
scenarios, cross-validation fails as often as it succeeds, if one is
interested in expected off-training set error (where I'm including the
validation set in the definition of "training set").
This leads one to wonder whether cross-validaton might be justified in
terms other than expected error (e.g., if it might have a minimax
justification.) Cullen and I have banged our heads over this issue a
bit already. (Manny Knill and Tal Grossman have also gotten into this
issue.) Things are quite subtle.
------------------------------
Date: Thu, 28 Jul 94 23:31:35 EDT
From: Cullen Schaffer <schaffer@cs.rutgers.edu>
Subject: Conservation Law
By now there are too many discussion threads for me to respond
comprehensively. Instead, I'd like to concentrate on just a few
key points.
******************************************************************
* 1. The conservation law is a distribution-independent result. *
* In particular, it does not depend on our assuming a uniform *
* distribution over the space of all possible concepts. *
******************************************************************
Diana Gordon commented at the conference that the conservation law
seemed to her to make the untenable assumption that all concepts are
equally likely. She and her colleague William Spear reiterate the
point in writing as follows:
This law appears to be essentially a counting argument, where we count
over all situations S. Thus, this law says something about the real
world if all possible situations occur, and if all possible situations
occur with equal probability. In that case, then, this law would be
100% predictive of generalization performance. The law becomes less
predictive as the real world deviates from a uniform distribution over
all situations.
Tom Dietterich makes a similar point:
The conservation law is averaging out the performance of a learning
algorithm over all possible target concepts (and distributions). This
is equivalent to permitting the teacher to label the test set
examples by flipping a coin. I think we can all agree that data
sets constructed this way do not contain any "pattern".
This is not the situation we face in real applications or that humans
face in daily life.
Both of these comments interpret the conservation law as making a
statement about ~average performance over all concepts. But to take
an average we need to know how likely each concept is and that's something
I think I've made clear I'm not comfortable with.
Rather than depend on distributional assumptions, I've presented the
conservation law simply as a sum. The fact that this sum is zero
implies (1) that positive elements must be balanced by negative ones
and (2) that increases in some elements must be balanced by decreases
in others. In other words, the conservation law tells us that
building induction algorithms involves unavoidable tradeoffs: (1) An
algorithm can't do better than chance on some problems without doing
worse than chance on others and (2) the better you make an algorithm
for learning some problems, the worse it gets for others.
In building algorithms, we naturally do make distributional
assumptions and try to allocate positive generalization performance to
concepts we think likely and negative generalization performance to
those we think unlikely. To me, the main point of the conservation
law paper is to draw attention to the fact that this is ~all we are
doing. Bias is not just an important element in induction--it's the
whole story. Information theory, statistical hypothesis testing, the
minimum description length principle, cross-validation and so on are
all more or less beside the point, as far as generalization is
concerned. All success ultimately depends on what we know beforehand
about the likelihood of various concepts.
Incidentally, it's not strictly wrong to view the conservation law as
an average with each element weighted equally--any sum can be
interpreted that way. I'm only arguing that this perspective is out
of place here. As I suggested in my original answer to Diana, the
same is true for conservation of momentum. The conservation law for
momentum tells us, for example, that, when three hard objects collide,
their momentum changes sum to zero. It's possible to cast that as a
statement that the average change in momentum is zero ~if we assume
that all three objects are equally important. And then we might
question whether "in the real world" we are likely to care equally
about all three objects in a collision.... Of course, this is an
important practical consideration, but a negative answer certainly
doesn't decrease the value of this conservation law, at least, in real
world applications.
***************************************************************
* 2. I don't view the conservation law as a negative result. *
***************************************************************
Larry Hunter, Tom Dietterich and many others communicating with me
directly have gone to some lengths to stress that we often can make
assumptions about our problems and hence that induction is not a lost
cause. It seems strange to me that that point needs defending;
certainly I agree wholeheartedly.
I guess the fault lies with me for starting my talk by summing up
several hundred years of philosophy with the statement "induction is
impossible." I followed up immediately with some points meant to
clarify the sense in which it's impossible, but subtleties seem to
have been lost in the shuffle.
More precisely then, the basic fact is that ~pure induction is
impossible. Observed cases alone can't tell us anything about
unobserved cases. As I've stressed above, success in generalization
is always due to knowledge external to the data.
A problem--one the paper was meant to address--is that our algorithms
look and feel as if they perform pure induction. A program like C4.5,
for example, takes only cases as input. If it performs well, it's
natural to think that there's something inherently good about the
fixed mapping it implements from training sets to prediction models.
The conservation law reminds us that pure induction is impossible,
that no mapping is inherently better or worse than any other. If
we're having success with a mapping like C4.5, it must be because
we're choosing our problems well. The power of the algorithm isn't in
how it extracts information from data, but rather in its implicit
encoding of knowledge of the situations in which we intend to apply
it.
On the other hand, this credit reassignment doesn't imply anything
negative about the utility of C4.5 or deny our inductive successes.
To me the message of conservation law is simply that induction is no
more or less than than the application of bias. This suggests a very
different perspective than the one implicit in many current research
reports, but not that we should abandon our work.
******************************************************************
* 3. Yes, but in the real world... *
******************************************************************
Tom Dietterich and others suggest that the negative effects of the
conservation law apply only when certain basic, weak assumptions about
the underlying concept are violated. Here's an extension of my
previous challenge that puts this idea to a (very informal) test.
The original challenge was to find a concept for which C4.5 using the
standard information gain ratio splitting criterion is significantly
worse in generalization than C4.5 using information ~loss ratio.
I have such a concept in mind. Assuming you have not guessed what it
is, try to state a weak assumption about target concepts that justifies
the use of information gain over information loss. If correct, such
an assumption should not hold for my concept.
Mail proposed assumptions to me (schaffer@roz.hunter.cuny.edu) and
I'll post a summary to the Machine Learning list saying for each
whether it does or does not hold for the challenge concept.
------------------------------
Date: Fri, 29 Jul 94 10:40:05 EDT
From: gordon@aic.nrl.navy.mil
Subject: Clarification: Conservation Law
Clarification:
In our comment (above) we stated that the Conservation Law is correct
because, as Cullen says, it is a counting argument - a sum without
any reference to a distribution. We understood this very clearly
after reading the paper (although it was not as clear at the talk).
What we were referring to in our comments was that although this
law says nothing about distributions, to apply it to the real world
one has to consider distributions. This is where the degree of
applicability depends upon the degree to which situations are
uniformly distributed.
Diana F. Gordon
William M. Spears
------------------------------
From: Michael de la Maza <mdlm@ai.mit.edu>
Date: Tue, 26 Jul 94 09:54:34 EDT
Subject: A comment on Hunter's comment on Schaffer's Law
In the Machine Learning list (Vol. 6 No. 20) Larry Hunter writes:
:I have
:been looking at the problem of automatically determining what learning
:method to use, and how best to apply it for more than five years, and I
:welcome Schaffer's result as validation of the appropriateness of this
:research agenda.
In fact, Schaffer's result does not validate Hunter's approach.
Multistrategy algorithms are not exempt from Schaffer's law. A method
that "intelligently" selects amongst learning algorithms will also
have a generalization performance of 0.
Cheers,
Michael de la Maza
------------------------------
Date: Tue, 26 Jul 94 10:54:58 -0400
From: Larry Hunter <hunter@work.nlm.nih.gov>
Subject: Re: A comment on Hunter's comment on Schaffer's Law
Mike de la Maza misunderstands a key point in his response to my ML posting.
He is correct that having a planner select among and combine learning
methods does not in any way change the fact that its generalization accuarcy
over all possible learning tasks must be zero. However, Schaffer's result
and both Wolpert's and Dietterich's related work all demonstrate the
dependence of generalization performance on the algorithm used AND on the
distribution of learning tasks it is applied to. That is the purpose of
planning to learn: identifying characteristics of the available data and of
the desired knowledge that make it possible to select learning method(s)
that are likely to generate the desired knowledge for a given learning task.
It's a framework for automating decisions, e.g. about inductive biases, that
ideally leads to generalization performance that is better for more tasks of
interest than the best single approach in the toolkit. Littlestone's
weighted majority algorithm is in the same vein, although the assumptions
necessary for his quite general theoretical results are much stronger than
those necessary in my more practically aimed work.
Schaffer raises a more sophisticated version of this idea in his response to
the first round of discussion. He agrees with me and Dietterich that a
useful research direction is to define important measurable data set
characteristics that are useful in selecting biases. He goes on to say
though, of course, these [assessments of the data] can't be used to
circumvent the conservation law; only information external to the data can
be used to justify the bias inherent in any generalization.
That is one of the main ideas behind planning to learn: that a statement of
a specific goal for knowledge (which is, of course, external to the data) is
a key factor in learning well.
------------------------------
From: Juergen Schmidhuber <schmidhu@informatik.tu-muenchen.de>
Subject: Generalization and Kolmogorov Complexity
Date: Fri, 29 Jul 1994 09:56:41 +0200
From the perspective of the theory of Kolmogorov
complexity, some of the statements by D. Wolpert,
C. Schaffer, T. Dietterich, and others may be
viewed as consequences of certain basic facts.
To be more specific, let the task be to learn a mapping
from finite bitstrings to finite bitstrings. A training
set is chosen randomly. In most cases, the shortest
algorithm computing a (non-overlapping) test set
essentially will have the size of the whole test set.
This is because nearly all computable objects are random
or incompressible. The shortest algorithm computing the
test set, given the training set, won't be any shorter
(the ``mutual algorithmic information'' between test set
and training set will be zero in nearly all cases).
There won't be any hope for generalization.
Juergen Schmidhuber
Fakultaet fuer Informatik, H2
Technische Universitaet Muenchen
80290 Muenchen, Germany
schmidhu@informatik.tu-muenchen.de
phone: (0049) (89) 2105 2406
fax: (0049) (89) 2105 8207
------------------------------
Date: Tue, 26 Jul 1994 16:47:31 -0400
From: Robert Holte <holte@csi.uottawa.ca>
Subject: A paradox related to the Conservation Law
Schaffer's results were based on batch learning, but Tom Dietterich
got me thinking about incremental learning (under what conditions do
learning curves rise monotonically, was his question), and there is an
interesting "paradox" here.
We have a finite dataset from which we draw one example at a time.
The examples we have not yet drawn define the generalization accuracy
at any moment in time. Suppose at some point I have a hypothesis whose
accuracy is
C/U
where C is the number of unseen things our hypothesis classifies correctly
and U is the total number of unseen things.
Now I draw my next example, so U -> U-1.
What happens to the generalization accuracy ?
If the new example is one my hypothesis classifies correctly, C -> C-1.
But, except in the degenerate case where the hypothesis is exactly correct
and C=U, we have
C/U > (C-1)/(U-1)
In other words, if the hypothesis is correct on the example,
generalization accuracy drops! On the other hand, if the new example
is one the hypothesis classifies incorrectly, C remains the same and
generalization accuracy rises!
This leads to the counterintuitive result that, if I want my accuracy
always to increase, then when I get a training example "confirming" my
hypothesis I MUST CHANGE my hypothesis, and when I get an example
"refuting" my hypothesis, I need not change my hypothesis!
I am not sure of the exact connection between this observation
and the Conservation Law; both are counterintuitive properties
of generalization accuracy.
-- Rob Holte holte@csi.uottawa.ca
------------------------------
End of ML-LIST (Digest format)
****************************************