Copy Link
Add to Bookmark
Report
Machine Learning List Vol. 6 No. 23
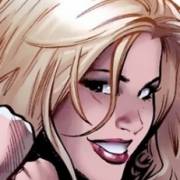
Machine Learning List: Vol. 6 No. 23
Sunday, August 21, 1994
Contents:
Employment Opportunity
CFP: Intelligent Adaptive Systems (IAS-95)
AAAI: Spring Symposium Series
ML-related paper in JAIR
Reminder: deadline approaching, special issue on ROBOT LEARNING
Final Call for Papers - IWANNT*95
Commentary on Holte's paradox
Conservation Law
Employment Opportunity
conservation law and the peaking phenomenon in PR
The Machine Learning List is moderated. Contributions should be relevant to
the scientific study of machine learning. Mail contributions to ml@ics.uci.edu.
Mail requests to be added or deleted to ml-request@ics.uci.edu. Back issues
may be FTP'd from ics.uci.edu in pub/ml-list/V<X>/<N> or N.Z where X and N are
the volume and number of the issue; ID: anonymous PASSWORD: <your mail address>
URL- http://www.ics.uci.edu/AI/ML/Machine-Learning.html
----------------------------------------------------------------------
Date: Thu, 18 Aug 94 08:45:57 EDT
From: Mike Weider <mikew@ai.iit.nrc.ca>
Subject: Employment Opportunity
R&D Opportunity in
Artifical Intelligence and Statistics
=====================================
Located in Ottawa, Ontario, Canada, Quadrillion Corporation is a
software development company specializing in advanced data analysis
tools. Our products are aimed at solving quality control and process
optimization problems in complex manufacturing environments.
We are currently seeking an individual to lead the research and
development of algorithmic improvements for our inductive
classification program, Q-Yield.
To be considered for this position you should have a M.Sc. in
Statistics or Computer Science and be familiar with at least one of the
following: quantitative machine learning techniques (decision trees,
neural nets, genetic algs.) or Bayesian networks; statistical inference
and the foundations of statistics. Strong background in C programming
techniques is essential. Knowledge of microelectronic manufacturing
processes would be considered an advantage.
Quadrillion's entrepreneurial environment encourages responsibility and
initiative. We offer a competitive salary and the chance to share in
the equity of our growing company. If you interested in this position,
please forward a cover letter and resume to: Quadrillion Corporation,
Attention: Michael Weider, 380 Pinhey Point Road, Dunrobin, Ontario K0A
1T0. Fax: 613-832-0547, Email: mikew@ai.iit.nrc.ca
------------------------------
Date: Mon, 15 Aug 94 13:18:44 EDT
Subject: CFP: Intelligent Adaptive Systems (IAS-95)
From: Janusz Wnek <jwnek@aic.gmu.edu>
CALL FOR PAPERS
FLAIRS-95 International Workshop on
Intelligent Adaptive Systems (IAS-95)
April 26, 1995, Melbourne Beach, Florida
Recently, there has been significant interest in developing intelligent
systems or agents capable of dynamically building and adapting their
knowledge-base with experience to accomplish a given task. Building such
agents is of great practical interest in view of the increased demand
for the development of knowledge-based systems, (e.g. personal assistants,
intelligent agents, information filters). However, very often the forms in
which it is easy to represent the obtained knowledge-base differ from those
which are dynamically adjustable for performing different tasks. Also,
efficient methodologies for acquiring knowledge-bases must be flexible
enough for dynamic adaptation.
The goal of this workshop is to bring together researchers working on the
development of fundamental strategies and methodologies needed for the
development of intelligent adaptive systems. The workshop seeks high
quality submissions in which there are links between the presented work,
the workshop topic, and potential applications. Papers with real-world
applications are very welcome.
The areas of interest of the workshop include, but are not limited to:
- Multistrategy Learning for Intelligent Agents
- Knowledge Discovery Systems
- Constructive Induction and Representation Space Improvement
- Adaptive Control Systems
- Implementations of Intelligent Agents
- Knowledge Acquisition via Inductive Logic Programming
Program Committee Chairs
Imam, I.F. George Mason University, USA
Wnek, J. George Mason University, USA
Program Committee Members
Gordon, D. Naval Research Laboratory, USA
Hamilton, H. University of Regina, Canada
Michalski, R.S. George Mason University, USA
Morik, K. University of Dortmund, Germany
Pazzani, M. University of California Irvine, USA
Rafea, A. Cairo University, Egypt
Ram, A. Georgia Institute of Technology, USA
Saitta, L. University of Trento, Italy
Schum, D. George Mason University, USA
Segen, J. AT&T Bell Laboratories, USA
Whitehall, B.L. United Technologies Research Center, USA
Ziarko, W. University of Regina, Canada
SUBMISSIONS
Paper submissions should not exceed ten single-spaced pages, with 1 inch
margins, 12pt font. The cover page must show author(s) full address, email
and include abstract (200 words maximum) and keywords (5 maximum).
Three copies of each paper should be sent to the contact address below.
Alternatively, one copy of a postscript file may be sent via email. The
papers will comprise a set of working notes (proceedings), copies of which
will be available at the workshop.
SCHEDULE
Paper submissions due December 1, 1994
Acceptance notice January 31, 1995
Camera ready version March 1, 1995
Workshop April 26, 1995
CONTACT ADDRESS
Ibrahim F. Imam
Machine Learning and Inference Center
George Mason University
4400 University Dr.
Fairfax, VA 22030
e-mail: iimam@aic.gmu.edu
Tel: (703) 993-1716
Fax: (703) 993-3729
------------------------------
From: Raul Valdes-Perez <valdes@carmen.kbs.cs.cmu.edu>
Date: Mon, 15 Aug 94 17:54:25 EDT
Subject: AAAI: Spring Symposium Series
AAAI 1995
Spring Symposium Series
March 27 - 29, 1995
Stanford University, California
Call for Participation
Sponsored by the American Association for Artificial Intelligence
445 Burgess Drive
Menlo Park, CA 94025
(415) 328-3123
sss@aaai.org
The American Association for Artificial Intelligence presents the 1995
Spring Symposium Series, to be held Monday through Wednesday, March 27
- 29, 1995, at Stanford University.
The topics of the nine symposia in the 1995 Spring Symposium Series
are:
o Empirical Methods in Discourse Interpretation and Generation
o Extending Theories of Action: Formal Theory and Practical Applications
o Information Gathering from Heterogeneous, Distributed Environments
o Integrated Planning Applications
o Interactive Story Systems: Plot and Character
o Lessons Learned from Implemented Software Architectures
for Physical Agents
o Representation and Acquisition of Lexical Knowledge:
Polysemy, Ambiguity, and Generativity
o Representing Mental States and Mechanisms
o Systematic Methods of Scientific Discovery
Symposia will be limited to between forty and sixty participants.
Each participant will be expected to attend a single symposium.
Working notes will be prepared and distributed to participants in each
symposium.
A general plenary session, in which the highlights of each symposium
will be presented, will be held on Tuesday, March 28, and an informal
reception will be held on Monday, March 27.
In addition to invited participants, a limited number of other
interested parties will be able to register in each symposium on a
first-come, first-served basis. Registration will be available by
January 1, 1995. To obtain registration information write to the AAAI
at 445 Burgess Drive, Menlo Park, CA 94025 (sss@aaai.org).
Submission Dates:
Submissions for the symposia are due on October 28, 1994.
Notification of acceptance will be given by November 30, 1994.
Material to be included in the working notes of the symposium must be
received by January 20, 1995. See the appropriate section below for
specific submission requirements for each symposium.
This document is available as
http://www.ai.mit.edu/people/las/aaai/sss-95/sss-95-cfp.html
**********************************************************************
SYSTEMATIC METHODS OF SCIENTIFIC DISCOVERY
Scientific discovery is surely among the most celebrated creative
processes. Discovery receives scholarly attention from several
disciplines, not least of which is artificial intelligence.
AI has explored the view that much of scientific reasoning is problem
solving, and hence is akin to more ordinary types of reasoning.
Experience has shown that some scientific reasoning can be automated:
research on discovery has already yielded competent programs that,
e.g.,, plan organic syntheses, elucidate molecular structure,
determine reaction mechanisms, make interesting graph-theoretic
conjectures, and detect patterned behavior. Where all this may lead
was foreseen by Allen Newell [Artif.Intell.; 25(3) 1985]:
[The field] should, by the way, be prepared for some radical, and
perhaps surprising, transformations of the disciplinary structure of
science (technology included) as information processing pervades it.
In particular, as we become more aware of the detailed information
processes that go on in doing science, the sciences will find
themselves increasingly taking a metaposition, in which doing
science (observing, experimenting, theorizing, testing, archiving, ...)
will involve understanding these information processes, and
building systems that do the object-level science. Then the
boundaries between the enterprise of science as a whole (the
acquisition and organization of the knowledge of the world) and AI
(the understanding of how knowledge is acquired and organized)
will become increasingly fuzzy.
The goals of this symposium are to examine how far we have come to
realizing Newell's vision, to identify fruitful current opportunities,
and to discuss the obstacles to progress in further understanding of
systematic methods for scientific inference. We solicit contributions
that advance these goals. Some examples of appropriate contributions
include:
o A program that automates a complex and creative scientific task.
o A new systematic method of scientific inference, even if its
automation is not yet feasible.
o A new representation or classification of science that enhances
efforts to systematize it.
o New opportunities for known systematic methods.
o A recent scientific achievement where the computer played an
essential creative role.
o New heuristics for scientific research, e.g., that promise to
make practicable some aspect of automated scientific reasoning.
o Computational models of historical discoveries in science.
o Cognitive studies of the scientific process that promise to
contribute to computational approaches.
Contributions that potentially bear on more than one scientific area
and that are demonstrably effective are of special interest.
SUBMISSION INFORMATION:
Prospective participants are invited to submit (in paper form) one of
the following to the symposium chair: three copies of an extended
abstract (at most 5 pages) of work to be presented, a description of
research in progress, or a statement describing what you hope to
contribute to and gain from the symposium. Please send submissions
and information requests to Raul Valdes-Perez, Computer Science
Department, Carnegie Mellon University, Pittsburgh, PA 15213 - USA
(phone: 412 268-7127, fax: 412 621-5117, email: valdes@cs.cmu.edu).
ORGANIZING COMMITTEE:
Lindley Darden Maryland
Joshua Lederberg Rockefeller
Herbert Simon Carnegie Mellon
Derek Sleeman Aberdeen
Raul Valdes-Perez (chair) Carnegie Mellon
------------------------------
Date: Mon, 15 Aug 94 16:38:56 PDT
From: Steve Minton <minton@ptolemy-ethernet.arc.nasa.gov>
Subject: ML-related paper in JAIR
JAIR is pleased to announce publication of the following article:
Murthy, S.K., Kasif, S. and Salzberg, S. (1994)
"A System for Induction of Oblique Decision Trees", Volume 2, pages 1-32.
Postscript: volume2/murthy94a.ps (475K)
Online Appendix: volume2/murthy94a-appendix.tar.Z (297K), OC1 source code
Abstract: This article describes a new system for induction of oblique
decision trees. This system, OC1, combines deterministic
hill-climbing with two forms of randomization to find a good oblique
split (in the form of a hyperplane) at each node of a decision tree.
Oblique decision tree methods are tuned especially for domains in
which the attributes are numeric, although they can be adapted to
symbolic or mixed symbolic/numeric attributes. We present extensive
empirical studies, using both real and artificial data, that analyze
OC1's ability to construct oblique trees that are smaller and more
accurate than their axis-parallel counterparts. We also examine the
benefits of randomization for the construction of oblique decision trees.
------------------------------
Date: Wed, 17 Aug 1994 17:46-EDT
From: Sebastian.Thrun@b.gp.cs.cmu.edu
Subject: Reminder: deadline approaching, special issue on ROBOT LEARNING
The deadline for submissions to the special issue on ROBOT LEARNING
(Machine Learning) is approaching. Here is the announcement again.
**************************************************************
***** CALL FOR PAPERS ******
**************************************************************
Special Issue on ROBOT LEARNING
Journal MACHINE LEARNING
(edited by J. Franklin and T. Mitchell and S. Thrun)
This issue focuses on recent progress in the area of robot learning.
The goal is to bring together key research on machine learning
techniques designed for and applied to robots, in order to stimulate
research in this area. We particularly encourage submission of
innovative learning approaches that have been successfully implemented
on real robots.
Submission deadline: October 1, 1994
Papers should be double spaced and 8,000 to 12,000 words in length,
with full-page figures counting for 400 words. All submissions will
be subject to the standard review procedure. It is our goal to also
publish the issue as a book.
Send three (3) copies of submissions to:
Sebastian Thrun
Universitaet Bonn
Institut fuer Informatik III
Roemerstr. 164
D-53117 Bonn
Germany
phone: +49-228-550-373
Fax: +49-228-550-382
E-mail: thrun@cs.bonn.edu, thrun@cmu.edu
Also mail five (5) copies of submitted papers to:
Karen Cullen
MACHINE LEARNING Editorial Office
Kluwer Academic Publishers
101 Philip Drive
Norwell, MA 02061 USA
phone: (617) 871-6300
E-mail: karen@world.std.com
Note: Machine Learning is now accepting submission of final copy
in electronic form. There is a latex style file and related files
available via anonymous ftp from world.std.com. Look in
Kluwer/styles/journals for the files README, smjrnl.doc, smjrnl.sty,
smjsamp.tex, smjtmpl.tex, or smjstyles.tar (which contains them all).
call again.
********* Please post ********
------------------------------
Date: Fri, 19 Aug 94 17:55:48 EDT
From: Lee Giles <giles@research.nj.nec.com>
Subject: Final Call for Papers - IWANNT*95
FINAL CALL FOR PAPERS
International Workshop on Applications of Neural Networks to
Telecommunications (IWANNT*95)
Stockholm, Sweden
May 22-24, 1995
You are invited to submit a paper to an international workshop
on applications of neural networks and other intelligent systems
to problems in telecommunications and information networking.
This is the second workshop in a series that began in Princeton,
New Jersey on October, 18-20 1993. This conference will take place
in the center of Stockholm at a time of the year when the beautiful
city is at its best. A tour in the famous archipelago adds to the
attraction.
This workshop will bring together active researchers in neural networks
and related intelligent systems with potential users in the telecommunications
industries. Today, telecommunications also means data transmission,
cable TV, wireless, and entertainment industries. We expect the workshop
to be a forum for discussion of applications issues relevant to the
enlarged circle of telecommunications industries.
It is sponsored by IEEE, INNS, SNNS (Swedish Neuronet Society),
Bellcore and Ericsson.
Suggested Topics:
Application of Neural Networks and other Intelligent Systems in:
Network Management
Congestion Control
Adaptive Equalization
Speech Recognition
Security Verification
Language ID/Translation
Information Filtering
Dynamic Routing
Software Reliability
Fraud Detection
Financial and Market Prediction
Adaptive User Interfaces
Fault Identification and Prediction
Character Recognition
Adaptive Control
Data Compression
Please submit 6 copies of both a 50 word abstract and a 1000 word summary
of your paper to arrive in New Jersey, USA by September 16, 1994.
Mail papers to the conference administrator:
Betty Greer, IWANNT*95
Bellcore, MRE 2P-295
445 South St.
Morristown, NJ 07960
(201) 829-4993
(fax) 829-5888
bg1@faline.bellcore.com
Abstract and Summary Due: September 16, 1994
Author Notification of Acceptance: November 1, 1994
Camera-Ready Copy of Paper Due: February 10, 1995
Organizing Committee:
General Chair
Josh Alspector
Bellcore, MRE 2P-396
445 South St.
Morristown, NJ 07960-6438
(201) 829-4342
josh@bellcore.com
Program Chair
Rod Goodman
Caltech 116-81
Pasadena, CA 91125
(818) 356-3677
rogo@micro.caltech.edu
Publications Chair
Timothy X Brown
Bellcore, MRE 2E-378
445 South St.
Morristown, NJ 07960-6438
(201) 829-4314
timxb@faline.bellcore.com
Treasurer
Anthony Jayakumar, Bellcore
Publicity
Atul Chhabra, NYNEX
Lee Giles, NEC
Local Arrangements
Miklos Boda, Ellemtel
Bengt Asker, Ericsson
Program Committee
Harald Brandt, Ellemtel
Tzi-Dar Chiueh, National Taiwan University
Francoise Fogelman, SLIGOS
Michael Gell, British Telecom
Larry Jackel, AT&T Bell Laboratories
Thomas John, Southwestern Bell
Adam Kowalczyk, Telecom Australia
S Y Kung, Princeton University
Tadashi Sone, NTT
Bernard Widrow, Stanford University
Conference Administrator
Betty Greer
Bellcore, MRE 2P-295
445 South St.
Morristown, NJ 07960
(201) 829-4993
(fax) 829-5888
bg1@faline.bellcore.com
International Workshop on Applications of
Neural Networks to Telecommunications (IWANNT*95)
Stockholm, Sweden
May 22-24, 1995
Registration Form
Name: _____________________________________________________________
Institution: __________________________________________________________
Mailing Address:
___________________________________________________________________
___________________________________________________________________
___________________________________________________________________
___________________________________________________________________
Telephone: ______________________________
Fax: ____________________________________
E-mail: _____________________________________________________________
I will attend | |
Send more information | |
Paper enclosed | |
Registration Fee Enclosed | |
($400; $500 after Apr. 15, 1995; $200 students;)
Please make sure your name is on the check (made out to IWANNT*95)
Registration includes lunch, a boat tour of the Stockholm archipelago,
and proceedings available at the conference.
Mail to:
Betty Greer, IWANNT*95
Bellcore, MRE 2P-295
445 South St.
Morristown, NJ 07960
(201) 829-4993
(fax) 829-5888
bg1@faline.bellcore.com
Deadline for submissions: September 16, 1994
Author Notification of Acceptance: November 1, 1994
Camera-Ready Copy of Paper Due: February 10, 1995
C. Lee Giles / NEC Research Institute / 4 Independence Way
Princeton, NJ 08540 / 609-951-2642 / Fax 2482
------------------------------
Received: from ics.uci.edu by paris.ics.uci.edu id aa29960; 13 Aug 94 11:49 PDT
Received: from ics.uci.edu by q2.ics.uci.edu id aa11630; 13 Aug 94 11:49 PDT
Received: from sfi.santafe.edu by q2.ics.uci.edu id aa11626; 13 Aug 94 11:49 PDT
Received: from chimayo.santafe.edu by sfi.santafe.edu (4.1/SMI-4.1)
id AA17641; Sat, 13 Aug 94 12:49:26 MDT
Received: by chimayo.santafe.edu (4.1/SMI-4.1)
id AA10131; Sat, 13 Aug 94 12:49:26 MDT
Date: Sat, 13 Aug 94 12:49:26 MDT
From: dhw@santafe.edu
Message-Id: <9408131849.AA10131@chimayo.santafe.edu>
To: ml@ics.uci.edu
Subject: Commentary on Holte's paradox
In the most recent go-round on the no-free-lunch/conservation results,
Salzberg says:
>>>
Yes, of course the law is correct. Furthermore, it is just a
corollary of the results from PAC learning theory.
I have discussed this "law" with a few theoreticians, and they agree
(with this statement).
>>>
I believe these theoreticians are
simply assuming that intuition behind independent identically
distributed (iid) error carries over to off-training set error...
***
Consider the following problem:
Fix the target f. I don't care what you fix it to. Also fix two
possible hypotheses, h1 and h2.
Now many times create an m-element training set d by sampling f. For
each such d, choose either h1 or h2 based on which is a better fit to
d (choose randomly between h1 and h2 in the case of a tie). This is
learning algorithm A. As an alternative choose h1 or h2 based on which
is a *worse* fit to d. This is algorithm B. (A possible variation:
Have A engage in random guessing unless the level of agreement for the
better fitting hypothesis is a lot higher than that of the
worse-fitting hypothesis. Or have B always guess randomly. Or
whatever.)
NOTE NO ASSUMPTION ABOUT A UNIFORM DISTRIBUTION OVER f's ENTERS INTO
THIS SCENARIO.
Q: Averaged over all data sets randomly chosen from the fixed f -
especially averaged over large data sets, so central limit theorem
stuff may come in to play - would you expect learning algorithm A to
have better off-training set behavior than B?
*Many* people (including me, originally) would say yes. (In fact, a
number of COLT researchers have corresponded to me claiming just this
scenario as a counter-example to the nfl theorems.)
However the nfl results tell us that this isn't true: there are just
as many f's (appropriately weighted) in which B beats A as vice-versa.
In addition, it turns that if you don't do the weighting (i.e., just
count up the number of f's), there are MORE f's in which B beats A
(i.e., has lower expected error) than vice-versa. (!!!!)
***
Salzberg goes on to say:
>>>
David Wolpert, arguing the other way, commented that:
2) Another important point is that PAC is quite limited in its
theoretical view of the world, whereas the no-free-lunch/conservation
results hold in a very broad context.
This criticism of PAC is completely unjustified by the actual
mathematical result. PAC results typically describe specific concept
classes, but I would argue that this makes them *more* relevant than
Schaffer's or Wolpert's results. If I want to study a specific
problem, then I'm looking at a specific concept class, not the space
of all concepts.
>>>
As I said in the full posting (only excerpted by Salzberg), I had
in mind "other theoretical views of the world" like Bayesian
analysis. The nfl results apply to the this-data view of Bayesian
analysis, PAC does not. In this PAC is limited.
As for the relevence of PAC ... I'm rather surprised that anyone would
hold it up as a shining example of relevent theory. PAC (and COLT in
general) is notorious for not being able to give practical advice of
use to real-world practitioners (in comparison to Bayesian analysis,
for example). A little bit of work with boosting, perhaps. But even
the boosting work championed by Drucker et al. has hardly taken the
applied learning community by storm.
(This isn't to say COLT is pointless. It can provide insight, and is
interesting in its own right. However it isn't very "relevent", as far
as applied learning is concerned.)
***
In any case, people are being way too glib in stating that
the no-free-lunch (nfl) results are part of current COLT lore.
Let me re-emphasize:
The kinds of PAC analysis that have been talked about in this
discussion (e.g., Dietterich's comments) are completely incapable of
giving negative results. (That kind of analysis gives upper bounds on
errors, not lower bounds.) The best it can do is say that in a
particular situation there is no positive result. In this respect, it
is far weaker than the nfl theorems. It certainly does NOT imply those
theorems. (To those claiming otherwise: some literature pointers
please?)
In fact, I would claim that many people in the COLT community
implicitly don't believe the nfl theorems. Their actions betray
them.
For example, I have had many conversations and read many papers in
which COLT researchers advocate a technique like membership-queries or
boosting. They state, in effect, that in some circumstances those
techniques can give you a win, and that in the other cases there's a
wash. In other words, they say that without making assumptions about
the real world, one can still be (probabilistically) assured of the
utility of these techniques (in comparison to random guessing, for
example).
The nfl theorems prove (!!!) that this is incorrect.
Boosting can just as readily give you *worse* performance than random
guessing as better performance. (Where of course I'm defining
"performance" as off-training set misclassification rate.) So for
example, if I use boosting when I don't have weak learnability, I will
not simply fail to get strong learnability. I will actually make the
performance worse (on average) than it would have been if I hadn't
boosted.
Similarly for membership queries. (More precisely: So long as the the
querying is such that the likelihood P(training set d | target f) is
independent of the values of f for inputs lying outside of d, then the
nfl theorems apply.)
***
Hartley says
>>>
The key is in the definition of the word "universal." There are two
relevant definitions:
Applicable independent of any assumptions.
and
Applicable throughout the entire universe.
... when asking about the real world it is the second definition
that is important. What could happen in other conceivable universes
is of no possible interest to us.
>>>>
In a similar vein, Gordon and Spears write:
>>>
... permitting the teacher to label the test set examples by
flipping a coin ... we don't believe a distribution meeting (this)
criterion is what we encounter in the world.
>>>
These comments are missing the point. Nobody said the uniform
distribution is what we encounter in this real world/universe.
Rather such a distribution can been *used*, to prove that every
learning algorithm must fail just as readily as it can succeed.
(Cullen makes this point quite well, but apparently he isn't getting
through.)
***
On a related topic, Jenkins writes:
>>>
I suspect that ... it is possible to construct robust learners for
a given (non-uniform) distribution over class probability vectors.
>>>
Definitely. This is what's called Bayesian analysis. A field
conspicuous by its absence in this discussion so far.
***
Concerning the discussion about Bob Holte's "paradox": I agree almost
exactly with what Bob wrote. He hit on many of the major reasons to
consider off-training set error.
One that he missed though is that off-training set error can be of far
more than academic interest. For example, in many real-world
situations (e.g., drug design based on computational biology) we are
explicitly only interested in off-training set error. (We already know
what happens with drugs in the training set - only drugs out of the
training set are of interest.)
More generally, it is *quite* common for the ultimate testing process
(namely, the use of the induced input-output relationship in
production) to not be an iid re-running of the process that generated
the training data.
As an example, this is always the case in active learning (aka
query-based learning).
In such situations, there is no particular reason to use the iid error
function rather than an off-training set one.
David Wolpert
------------------------------
From: Robert Holte <holte@csi.uottawa.ca>
Subject: Conservation Law
Date: Tue, 16 Aug 1994 23:06:38 -0400
I'd like to add three things to the discussion: the first two are short;
the third is longer and addresses a key issue in the discussion to date,
namely, that a Conservation Law says nothing whatsoever about EXPECTED
performance. Cullen has given us a Conservation Law, not a law about
expected performance. We would probably all prefer to have a law about
expected performance, because we can immediately see the value of such
a law, whereas the value of a Conservation Law is not nearly so obvious
(I try to elucidate it below).
But it is a Conservation Law that Cullen has given us -- we must make
the best use of it we can, and, if we want to have a law of expected
performance in addition, it is up to us all to start working on one.
My first item is simply adding to Dave Wolpert's experiences about
non-contrived circumstances in which "cross-validation failed badly".
I had such failures when I tried to add cross-validation-like
mechanisms to improve my 1R program (these failures occurred on
certain of the UCI datasets, not on a contrived dataset).
Analyzing these specific failures, I was able to identify general
circumstances in which these mechanisms would fail.
It is worth mentioning that these circumstances seem absolutely
implausible, one would never expect them to arise in practice;
but they did.
My second item is a question for Tom Dietterich, Stephen Salzberg,
or anyone else who thinks the Conservation Law follows from a PAC
result. I am not entirely ignorant of the PAC literature, but I can't
see any deductive connection between any PAC results and the
Conservation Law. Please tell me which PAC result you're referring to,
and how the Conservation Law may be DEDUCED from it.
Turning now to the distinction between a Conservation Law and a law
of expected performance, let me begin by introducing a very simple
artificial setting in which this distinction can be clearly seen.
In this little world there are exactly two kinds of two-armed bandits.
(a two-armed bandit is a gambling machine with two arms: you pick one arm
and pull it.) Bandit R pays you $1 if you pull its right arm; but if you
pull its left arm it takes $1 away from you (don't ask how). Bandit L is
the reverse: you get $1 if you pull on its left arm, you lose $1 if you
pull its right arm.
Now there are various strategies for deciding which arm to pull.
Because the variety of different bandits is so limited, I will also
restrict the kinds of strategy I will allow -- the strategy must be
"blind", that is, it is not told (= does not base its decision on)
the outcomes of any arm-pullings.
With these bandits and strategies, there is a conservation law, which I
am sure is perfectly obvious to everyone:
the amount that is won (loss = negative winning) by any blind strategy
when it plays Bandit R is exactly equal but OPPOSITE IN SIGN
to the amount it wins when it plays Bandit L.
I have stated the law in a particular form so that it would look as
much like a Conservation Law as it possibly could. But there is another
way to write down the very same thing:
For all blind strategies S: WINNINGS(S,L) + WINNINGS(S,R) = 0.
Now we're at an interesting juncture, because IN THIS FORM, this Law
is exactly analagous to Cullen's, with this correspondence:
bandits = learning situations
strategies = learning algorithms
winnings = generalization accuracy
And indeed, the correspondence is perfect, which means the questions
Cullen has been facing about "distributional assumptions" can be posed
about this law too -- all of sudden, in this form, this law looks like
it is making assumptions about the distribution of Bandits in the world.
Is it making distributional assumptions about the world ?
Absolutely not. It's a conservation law -- its exact meaning was
stated clearly, without any hidden assumptions in the first form.
Whatever a strategy would win on Bandit L it would lose on Bandit R.
That's a statement of fact that is true and has nothing to do with the
distribution of Bandits in the world.
However, this form of the law is slightly AMBIGUOUS, because there is
an entirely separate question -- one having nothing to do with Conservation
Laws -- which gives rise to a generalization of this very equation.
Suppose I were to ask this question: if I go to Las Vegas and use blind
strategy S what is my expected winnings ? Well, the answer to that
question surely does depend on the distribution of machines in Las Vegas.
I might win every single time (if I always pull the right arm and Las Vegas
happens to be full of R-bandits); or I might lose every single time.
In general,
For all blind strategies S:
Expected Winnings (S) = p(L)*WINNINGS(S,L) + p(R)*WINNINGS(S,R)
where p(L) = the probability of my playing against an L-bandit when I go
to Las Vegas
p(R) = 1 - p(L).
This is not a Conservation Law, it is the definition of "expectation";
it is a weighted average. It is not what Cullen is talking about. His
Law does not say what a system's expected generalization accuracy is,
no more than the Conversation Law above says what your expected winnings
in Las Vegas would be.
A question about expected generalization accuracy that COULD be asked is:
under what sorts of distributions do all learning systems have
an expected generalization accuracy of exactly 0 ?
In my little world, the analogous question is --
for what values of p(L) is it true that
For all blind strategies S:
Expected Winnings (S) = p(L)*WINNINGS(S,L) + p(R)*WINNINGS(S,R) = 0
(note the = 0 on the end).
As it happens, one answer to this question does FOLLOW FROM the
Conservation Law, namely when p(L)=p(R) = 1/2 (the uniform distribution).
Cullen never has posed this question, nor was his motivation for the
Law its relevance to this question. Cullen doesn't even like this question.
For him, the Conservation Law is important for its own direct consequences.
What then, are the implications of the Conservation Law ?
The whole message of the Conservation Law is the idea of "equal and
opposite reaction" -- if you change your learning algorithm and
gain 2% points in one learning situation, you can be sure there is
an exactly corresponding decrease spread out somehow over all the other
learning situations. Normally, we don't have a clue how that decrease
is spread -- ideally it would fall on situations that have a zero
probability of occurring in this world; in that case our expected
generalization accuracy (in this world) would inch up a bit.
But that is beyond the Conversation law -- all the Law says is that
there is a decrease somewhere, so watch out!
The question of where the decrease will occur is an empirical question.
It's a question we should worry about because it could well be that our
2% increase in real learning situation #1 has come at the expense of a
big decrease on an equally real, but less probable, learning situation.
The Conversation Law can't tell us where the decreases will happen,
it just warns us that we must watch out for them. If we are fortunate
(or knowledgeable about the world), the decreases will always happen
in bizarre, totally unreal learning situations. Whether this is true or
not is an empirical question. Cullen's examples show that, as a matter of
fact, it is not true. He gives examples where the "price" for increases on
some real datasets is, in this world, sometimes "paid" by other equally
real datasets.
No doubt a specific prediction about where the decreases will fall, or
in what sort of learning situations a particular learning algorithm
will fail, is more informative than the mere warning a Conservation
Law gives. But a warning is better than nothing -- I am always grateful
for the road signs "watch for falling rock". They don't predict where or
when the rocks will fall, but they serve the eminently useful purpose
of alerting the driver to danger. That's what Cullen's Conservation Law
does for machine learning.
-- Rob Holte
------------------------------
Date: Thu, 18 Aug 94 08:45:57 EDT
From: Mike Weider <mikew@ai.iit.nrc.ca>
Subject: Employment Opportunity
R&D Opportunity in
Artifical Intelligence and Statistics
=====================================
Located in Ottawa, Ontario, Canada, Quadrillion Corporation is a
software development company specializing in advanced data analysis
tools. Our products are aimed at solving quality control and process
optimization problems in complex manufacturing environments.
We are currently seeking an individual to lead the research and
development of algorithmic improvements for our inductive
classification program, Q-Yield.
To be considered for this position you should have a M.Sc. in
Statistics or Computer Science and be familiar with at least one of the
following: quantitative machine learning techniques (decision trees,
neural nets, genetic algs.) or Bayesian networks; statistical inference
and the foundations of statistics. Strong background in C programming
techniques is essential. Knowledge of microelectronic manufacturing
processes would be considered an advantage.
Quadrillion's entrepreneurial environment encourages responsibility and
initiative. We offer a competitive salary and the chance to share in
the equity of our growing company. If you interested in this position,
please forward a cover letter and resume to: Quadrillion Corporation,
Attention: Michael Weider, 380 Pinhey Point Road, Dunrobin, Ontario K0A
1T0. Fax: 613-832-0547, Email: mikew@ai.iit.nrc.ca
------------------------------
Date: Thu, 18 Aug 94 08:47:07 +0200
From: Bob Duin <bob@ph.tn.tudelft.nl>
Subject: conservation law and the peaking phenomenon in PR
The discussion on the "conservation law" strongly resembles some points
in the discussion on "the mean accuracy of statistical pattern
recognizers" during the seventies. For those who are not aware of this
and are interested, here is a summary.
In 1968 Hughes published a paper on the "mean" accuracy of statistical
pattern recognizers. This is the expected accuracy of a particular PR
method averaged over all possible problems. (The expected accuracy is
the true classification error averaged over all possible training sets
of a given size). Hughes studied the mean accuracy for a classifier
based on measurement cells: all measurement data are mapped into
measurement cells and these cells are assigned to classes using Bayes
Rule and ML probability estimates. Different classification problems
have different true cell probabilities. Hughes assumed a uniform
distribution of these probabilities constituting his universe of
classification problems. He discovered that for fixed training set
sizes and increasing measurement complexity (the number of cells) the
mean classification error first decreases and then starts to increase,
for a certain measurement complexity passing the apriori error.
The phenomenon Hughes discovered was called in the PR literature
`peaking'. It is related to what is now called `overtraining'. It had
been observed before for particular applications in which the
classification performance was studied as a function of the feature
size. Hughes was the first to study this theoretically. The fact that
the mean accuracy could be as bad as having no generalization at all
(for finite nonzero training set sizes) was the striking aspect of
Hughes study. Between 1970 and 1978 several papers appeared discussing
Hughes results and studying the mean accuracy for various models. A few
points:
1. If Bayes estimators are used instead of ML estimators, the mean
classification error still peaks, but now approaches the apriori error
just asymptotically and never equals it for finite measurement
complexities. So after a certain point the increasing complexity causes
a vanishing generalization.
2. If instead of Hughes' measurement space a k-dimensional continuous
feature space is used, the mean accuracy also peaks for increasing k.
Even for the case of independent features this appeared to be
possible.
3. Conditions can be given under which for independent features the
mean classification error does not peak, in that case showing a
monotonous decreasing behavior as a function of the feature size
(dimensionality).
4. Finally, around 1978, it became perfectly clear that Hughes used a
very strange model: due to the uniform distributed parameter values for
his universe of classification problems with constant measurement
complexity (number of cells), the universe of more complex problems
weights the more simple problems in a non-uniform way. So Hughes used a
hierarchy of incomparable models. This inconsistency can be avoided in
the feature space approaches.
5. Having solved this, it became clear that if Bayesian estimators are
used that are consistent with the parameter distribution the mean
classification error does not peak and decreases monotonically with the
feature size. So the peaking phenomenon of the mean classification
error, and thereby the situation of no generalization, is caused by
using estimators that are not consistent with the parameter
distribution (which is in practice almost always unknown).
6. What is good for the average case (a set of problems) is not
necessarily good for a particular case, a fixed problem. Therefore, it
remains possible that the optimal estimators, optimized over a set of
problems, are bad for a particular problem, thereby causing peaking of
the expected classification error (over all training sets) and the no
generalization situation. However, for a particular problem with a
finite training set, the optimal estimators can not be determined. (The
case of infinite training set is trivial). So peaking and a vanishing
generalization cannot be avoided for a fixed problem.
7. If the expected classification error can peak, the true
classification error of a fixed classification problem defined by just
a single training set can certainly peak. It thereby may arrive in the
no generalization state. This holds for instance for a training set
that is a bad representation of the true data distributions, which has
always a finite probability.
REFERENCES
G.F. Hughes, "On the mean accuracy of statistical pattern recognizers,"
IEEE Transactions on Information Theory, vol. IT-14, pp. 55-63, January
1968.
J.W. Sammon Jr. and D. Foley, "Considerations of dimensionality versus
sample size," in: Proc. IEEE Symp. Adaptive Processes, pp.
IX.2.1-IX.2.2, Austin, Texas, December 1970.
L.N. Kanal and B. Chandrasekaran, "On dimensionality and sample size in
statistical pattern classification," Pattern Recognition, vol. 3, pp.
225-234, October 1971.
D.H. Foley, "Considerations of sample and feature size," IEEE Trans. on
Information Theory, vol. IT-18, no. 5, pp. 618-626, September 1972.
S. Raudys, "On dimensionality, Learning, sample size and complexity of
classification algorithms," in: Proc. of the 3rd Int. Conf. on Pattern
Recognition, pp. 166-169, Coronado, California, November 1976.
S. Raudys, Limitations of sample size in classification problems (in
Russian), Inst. of Physics and Mathematics of the Academy of Sciences
of the Lithuanian SSR, Vilnius 1976
J.W. Van Ness and C. Simpson, "On the effects of dimension in
discriminant analysis," Technometrics, vol. 18, no. 2, pp. 175-187, May
1976.
J.W. van Ness, Dimensionality and classification performance with
independent coordinates, IEEE Trans. on Systems, Man, and Cybernetics,
vol. SMC-7, no. 3, 1977, July, 560-564.
W.G. Waller and A.K. Jain, "Mean recognition accuracy of dependent
binary measurements," in: Proc. 7th Int. Conf. on Cybern . and Society,
pp. 586-590, Washington D.C., September 1977.
R.P.W. Duin, Comments on `Independence, Measurement Complexity and
Classification Performance', IEEE Trans. on Systems, Man, and
Cybernetics, vol. SMC-7, no. 3, 1977, July, 559-560.
R.P.W. Duin, The mean recognition performance for independent
distributions, IEEE Trans. on Information Theory, vol. IT-24, no. 3,
1978, May, 394-395.
A.K. Jain and W.G. Waller, "On the optimal number of features in the
classification of multivariate gaussian data," Pattern Recognition,
vol. 10, pp. 365 - 374, 1978.
W.G. Waller and A.K. Jain, "On the monotonicity of the performance of
Bayesian classifiers," IEEE Trans. on Information Theory, vol. IT-24,
pp. 392 - 394, 1978.
R.P.W. Duin, On the accuracy of statistical pattern recognizers (Ph.D.
thesis), Technische Hogeschool Delft, 1978.
J.M. van Campenhout, "On the peaking of the Hughes mean recognition
accuracy: the resolution of an apparent paradox," in: Proc. of the 4th
Int. Conf. on PR, pp. 224 - 229, Kyoto, 1978.
A.K. Jain and B. Chandrasekaran, "Dimensionality and Sample Size
Considerations in Pattern Recognition Practice," in: Handbook of
Statistics, vol. 2, ed. P.R. Krishnaiah and L.N. Kanal, pp. 835 - 855,
North-Holland, Amsterdam, 1987.
Bob Duin
R.P.W. Duin Phone: (31) 15 786143
Faculty of Applied Physics Fax: (31) 15 626740
Delft University of Technology E-mail: duin@ph.tn.tudelft.nl
P.O. Box 5046, 2600 GA Delft
The Netherlands
------------------------------
End of ML-LIST (Digest format)
****************************************