Copy Link
Add to Bookmark
Report
Machine Learning List Vol. 6 No. 15
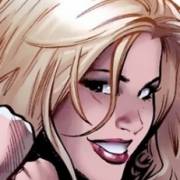
Machine Learning List: Vol. 6 No. 15
Friday, June 10, 1994
Contents:
COLT94/ML94 hotel reservation deadline
Special Issueof Knowledge-Based Systems
Postdoctoral Position: Applying Machine Learning to Ecosystem Modeling
New Book and Videotape on Genetic Programming
AI'94 Tutorial on Intelligent Learning Database Systems
The Machine Learning List is moderated. Contributions should be relevant to
the scientific study of machine learning. Mail contributions to ml@ics.uci.edu.
Mail requests to be added or deleted to ml-request@ics.uci.edu. Back issues
may be FTP'd from ics.uci.edu in pub/ml-list/V<X>/<N> or N.Z where X and N are
the volume and number of the issue; ID: anonymous PASSWORD: <your mail address>
----------------------------------------------------------------------
Sender: Haym Hirsh <hirsh@athos.rutgers.edu>
Date: Wed, 8 Jun 94 22:46:59 EDT
From: Haym Hirsh <hirsh@cs.rutgers.edu>
To: ml@ics.uci.edu
Subject: COLT94/ML94 hotel reservation deadline
>> REMINDER <<
The deadline to reserve a hotel room at the Hyatt for COLT94/ML94 is
FRIDAY, JUNE 10.
After this date, the negotiated conference rate of $91/night for a
single or double will no longer be available, and it may become more
difficult to get a room at all.
To reserve a room, contact Hyatt directly at: 908-873-1234 or
800-233-1234; fax 908-873-1382. Be sure to reference ML94/COLT94.
Further information on COLT94/ML94 (including registration
information) is available via anonymous ftp from cs.rutgers.edu in the
directory /pub/learning94, and also from our www site at
http://www.cs.rutgers.edu/pub/learning94/learning94.html. If you do
not have access to ftp/www, send email to ml94@cs.rutgers.edu or
colt94@research.att.com.
See you in July!
Haym
------------------------------
Date: Mon, 6 Jun 1994 15:13:46 -0400
From: fu@cis.ufl.edu
To: ML@ics.uci.edu
Subject: Special Issueof Knowledge-Based Systems
CALL FOR SUBMISSIONS
Special Issue of the Journal ``Knowledge-Based Systems''
Theme: ``Knowledge-Based Neural Networks''
Guest Editor: LiMin Fu (University of Florida, USA)
A. Background:
Knowledge-based neural networks are concerned with
the use of domain knowledge to
determine the initial structure of the neural network.
Such constructions have drawn increasing attention recently.
The rudimentary idea is simple: the knowledge-based approach
models what we know and the neural-network approach does
what we are ignorant or uncertain of.
Furthermore, there is an urgent need for a bridge
between symbolic artificial intelligence and neural networks.
The main goal of this special issue is to explore the relationship between them
for engineering intelligent systems.
B. Contents:
Examples of specific research include but are not limited to:
(1) How do we build a neural network based on prior
knowledge?
(2) How do neural heuristics improve the current model
for a particular problem (e.g., classification, planning,
signal processing, and control)?
(3) How does knowledge in conjunction with neural heuristics
contribute to machine learning?
(4) What is the emergent behavior of a hybrid system?
(5) What are the fundamental issues behind the combined approach?
C. Schedule:
Four copies of a full-length Paper should be submitted, by 1 September 1994,
to
Dr. LiMin Fu
Department of Computer and Information Sciences
301 CSE
University of Florida
Gainesville, FL 32611
USA
All papers will be subject to stringent review.
------------------------------
From: Tom Dietterich <tgd@chert.cs.orst.edu>
Date: Wed, 8 Jun 94 12:47:01 PDT
To: ml@ics.uci.edu
Subject: Postdoctoral Position: Applying Machine Learning to Ecosystem Modeling
Postdoctoral Position: Applying Machine Learning to Ecosystem Modeling
Complex ecosystem models are calibrated by manually fitting them to
available data sets. This is time-consuming, and it can result in
overfitting of the models to the data. We are applying machine
learning methods to automate this calibration and thereby improve the
reliability and statistical validity of the resulting models. Our
ecosystem model--MAPSS--predicts amounts and types of vegetation that
will grow under global warming climate scenarios. An important goal
of global change research is to incorporate such vegetation models
into existing ocean-atmosphere physical models.
Under NSF funding, we are seeking a Post-Doc to assume a major role in
carrying out this research. Components of the research involve (a)
representing ecosystem models declaratively, (b) implementing
gradient and non-gradient search techniques for parameter fitting,
(c) implementing parallel algorithms for running and fitting the
ecosystem model, and (d) conducting basic research on issues of
combining prior knowledge with data to learn effectively. The ideal
candidate will have a PhD in computer science or a closely related
discipline with experience in neural networks, simulated annealing
(and similar search procedures), knowledge representation, and
parallel computing. The candidate must know or be eager to learn some
basic plant physiology and soil hydrology. Computational resources
for this project include a 16-processor 1Gflop Meiko multicomputer and
a 128-processor CNAPS neurocomputer.
Applicants should send a CV, summary of research accomplishments,
sample papers, and 3 letters of reference to
Thomas G. Dietterich
303 Dearborn Hall
Department of Computer Science
Oregon State University
Corvallis, OR 97331
tgd@cs.orst.edu
Principal investigators:
Thomas G. Dietterich, Department of Computer Science
Ron Nielson, US Forest Service
OSU is an Affirmative Action/Equal Opportunity Employer and Complies
with Section 504 of the Rehabilitation Act of 1973. OSU has a policy
of being responsive to the needs of dual-career couples.
Closing Date: July 5, 1994
------------------------------
Date: Tue, 24 May 94 11:13:47 PDT
From: John Koza <koza@cs.stanford.edu>
Subject: New Book and Videotape on Genetic Programming
Genetic Programming II and the associated videotape
are now available from the MIT Press.
GENETIC PROGRAMMING II:
AUTOMATIC DISCOVERY OF REUSABLE
SUBPROGRAMS
by John R. Koza
Computer Science Department
Stanford University
It is often argued that the process of solving complex
problems can be automated by first decomposing the
problem into subproblems, then solving the presumably
simpler subproblems, and then assembling the solutions to
the subproblems into an overall solution to the original
problem. The overall effort required to solve a problem can
potentially be reduced to the extent that the decomposition
process uncovers subproblems that are diPesproportionately
easy to solve and to the extent that regularities in the
problem environment permit multiple use of the solutions
to the subproblems. Sadly, conventional techniques of
machine learning and artificial intelligence provide no
effective means for automatically executing this alluring
three-step problem-solving process on a computer.
GENETIC PROGRAMMING II describes a way to
automatically implement this three-step problem-solving
process by means the recently developed technique of
automatically defined functions in the context of genetic
programming. Automatically defined functions enable
genetic programming to define useful and reusable
subroutines dynamically during a run. This new technique
is illustrated by solving, or approximately solving, example
problems from the fields of Boolean function learning,
symbolic regression, control, pattern recognition, robotics,
classification, and molecular biology. In each example, the
problem is automatically decomposed into subproblems;
the subproblems are automatically solved; and the solutions
to the subproblems are automatically assembled into a
solution to the original problem. Leverage accrues because
genetic programming with automatically defined functions
repeatedly uses the solutions to the subproblems in the
assembly of the solution to the overall problem. Moreover,
genetic programming with automatically defined functionsn
produces solutions that are simpler and smaller than the
solutions obtained without automatically defined functions.
CONTENTS...
1. Introduction
2. Background on Genetic Algorithms, LISP, and Genetic
Programming
3. Hierarchical Problem-Solving
4. Introduction to Automatically Defined Functions P The
Two-Boxes Problem
5. Problems that Straddle the Breakeven Point for
Computational Effort
6. Boolean Parity Functions
7. Determining the Architecture of the Program
8. The Lawnmower Problem
9. The Bumblebee Problem
10. The Increasing Benefits of ADFs as Problems are
Scaled Up
11. Finding an Impulse Response Function
12. Artificial Ant on the San Mateo Trail
13. Obstacle-Avoiding Robot
14. The Minesweeper Problem
15. Automatic Discovery of Detectors for Letter
Recognition
16. Flushes and Four-of-a-Kinds in a Pinochle Deck
17. Introduction to Molecular Biology
18. Prediction of Transmembrane Domains in Proteins
19. Prediction of Omega Loops in Proteins
20. Lookahead Version of the Transmembrane Problem
21. Evolution of the Architecture of the Overall Program
22. Evolution of Primitive Functions
23. Evolutionary Selection of Terminals
24. Evolution of Closure
25. Simultaneous Evolution of Architecture, Primitive
Functions, Terminals, Sufficiency, and Closure
26. The Role of Representation and the Lens Effect
27. Conclusion
Appendix A: List of Special Symbols
Appendix B: List of Special Functions
Bibliography
Appendix C: List of Type Fonts
Appendix D: Default Parameters for Controlling Runs of
Genetic Programming
Appendix E: Computer Implementation of Automatically
Defined Functions
Appendix F: Annotated Bibliography of Genetic
Programming
Appendix G: Electronic Newsletter, Public Repository, and
FTP Site
Hardcover. 746 pages. ISBN 0-262-11189-6.
Genetic Programming II Videotape:
The Next Generation
by John R. Koza
This videotape provides an explanation of automatically
defined functions, the hierarchical approach to problem
solving by means of genetic programming with
automatically defined functions, and a visualization of
computer runs for many of the problems discussed in
Genetic Programming II. These problems include symbolic
regression, the parity problem, the lawnmower problem, the
bumblebee problem, the artificial ant, the impulse response
problem, the minesweeper problem. the letter recognition
problem, the transmembrane problem, and the omega loop
problem.
VHS videotape. 62-Minutes. Available in VHS NTSC,
PAL, and SECAM formats.
NTSC ISBN 0-262-61099-X. PAL ISBN 0-262-61100-7.
SECAM ISBN 0-262-61101-5.
The following order form can be used to order copies of
Genetic Programming I or II, videotapes I or II, and
Kinnear's recent book.
Order Form
Send to
The MIT Press
55 Hayward Street
Cambridge, MA 02142 USA
You may order by phone 1-800-356-0343 (toll-free);
or by phone to 617-625-8569;
or by Fax to 617-625-6660;
or by-e-mail to mitpress-orders@mit.edu
Please send the following:
___copies of book Genetic Programming: On the
Programming of Computers by Means of Natural
Selection by John R. Koza (KOZGII) @$55.00
___copies of book Genetic Programming II:
Automatic Discovery of Reusable Programs by
John R. Koza (KOZGH2) @$45.00
___copies of book Advances in Genetic
Programming by K. E. Kinnear (KINDH) @$45.00
___copies of videoGenetic Programming: the Movie
in VHS NTSC Format (KOZGVV) @$34.95
___copies of videoGenetic Programming:the Movie
in VHS PAL Format (KOZGPV) @$44.95 each
___copies of videoGenetic Programming:the Movie
in VHS SECAM Format (KOZGSV) @$44.95
___copies of video Genetic Programming II
Videotape: The Next Generation in VHS NTSC
Format (KOZGV2) @$34.95
___copies of video Genetic Programming II
Videotape: The Next Generation in VHS PAL
Format (KOZGP2) @$44.95
___copies of video Genetic Programming II
Videotape: The Next Generation in VHS SECAM
Format (KOZGS2) @$44.95
Shipping and handling: Add $3.00 per item.
Outside U.S. and Canada: add $6.00 per item for
surface shipment or $22.00 per item for air
Total for items ordered ________
Shipping and handling ________
Canadian customers add 7% GST ________
Total ________
[ ] Check or money order enclosed
[ ] Purchase order attached P Number __________
[ ] Mastercard [ ] Visa
Expiration date ___________
Card Number _____________________________
Ship to:
Name _____________________________________
Address ___________________________________
__________________________________________
__________________________________________
City ______________________________________
State ______________________
Zip or Postal Code___________
Country ___________________
Daytime Phone _____________________________
For orders in the UK, Eire, Continental Europe, please
contact the London office of the MIT Press at:
The MIT Press
14 Bloomsbury Square
London WC1A 2LP
England
Tel (071) 404 0712
Fax (071) 404 0610
e-mail 100315.1423@compuserve.com
For order in Australia, please contact:
Astam Books
57-61 John Street
Leichhardt, NSW 2040 Australia
Tel (02) 566 4400
Fax (02) 566 4411
Please note that prices may be higher outside the US.
In all other areas of the world or in case of difficulty, please
contact:
The MIT Press International Department
55 Hayward Street, Cambridge, MA 02142 USA
Tel 617 253 2887
Fax 617 253 1709
e-mail curtin@mit.edu
------------------------------
From: Xindong Wu <xindong@cs.jcu.edu.au>
Subject: AI'94 Tutorial on Intelligent Learning Database Systems
Date: Wed, 25 May 1994 08:43:34 +1000 (+1000)
Seventh Australian Joint Conference on Artificial Intelligence (AI'94)
"Sowing the Seeds for the Future"
C A L L F O R P A R T I C I P A T I O N S
AI'94 Tutorial on Intelligent Learning Database Systems
by
Dr Xindong Wu
Dept. of Computer Science, James Cook University,
Townsville, Queensland 4811, Australia
xindong@coral.cs.jcu.edu.au
22 November 1994
ABSTRACT
Knowledge acquisition from databases is a research frontier for both database
technology and machine learning (ML) techniques, and has seen sustained
research over recent years. It also acts as a link between the two fields,
thus offering a dual benefit. Firstly, since database technology has already
found wide application in many fields, ML research obviously stands to gain
from this greater exposure and established technological foundation. Secondly,
ML techniques can augment the ability of existing database systems to
represent, acquire, and process a collection of expertise such as those which
form part of the semantics of many advanced applications (e.g. CAD/CAM). This
full-day tutorial will present and discuss techniques for the following 3
interconnected phases in constructing intelligent learning database systems:
(1) Translation of standard database information into a form suitable for use
by a rule-based system; (2) Using machine learning techniques to produce rule
bases from databases; and (3) Interpreting the rules produced to solve users'
problems and/or reduce data spaces. It will suit a wide audience (including
postgraduate students and industrial people) from databases, expert systems,
and machine learning.
CONTENTS
1. Knowledge Acquisition from Databases: Problem and Domain
1.1 Problems in Conventional Databases
1.2 Research Topics in Intelligent Databases
1.3 Requirements for Knowledge Discovery in Databases
2. Typical Inductive Learning Algorithms
2.1 The ID3 Family
2.2 The AQ Family
2.3 The HCV Family
3. Integrating More Semantic Information into Data Models
3.1 The E-R Model
3.2 Deductive and Object-Oriented Databases
3.3 More Expressive Representations
4. An Intelligent Learning Database System
To introduce a PC shell developed at Edinburgh
5. Conclusions and Research Directions in the Field
6. A Practical Component Using a PC Lab
COURSE MATERIAL
- Xindong Wu, Research Issues in Intelligent Learning Database Systems,
Proceedings of the Seventh Annual Florida AI Research Symposium, Pensacola
Beach, Florida, U.S.A., May 5-7, 1994, 137--141.
- Xindong Wu, Inductive Learning: Algorithms and Frontiers, Artificial
Intelligence Review, 7(1993), 2: 93-108.
- Xindong Wu, KEshell2: An Intelligent Learning Data Base System, Research and
Development in Expert Systems IX, M.A. Bramer and R.W. Milne (Eds.),
Cambridge University Press, U.K., 1992, 253--272.
BIO-DATA OF THE PRESENTER
Dr Xindong Wu received his first and Master's degrees in Computer Science from
Hefei University of Technology, China, and his Ph.D. in Artificial Intelligence
from the University of Edinburgh, Britain. In the past, he has authored 2
technical books, Expert Systems Technology (1988) and Constructing Expert
Systems (1990). He has also published over 60 papers in various periodicals
(such as Expert Systems: The International Journal of Knowledge Engineering,
Artificial Intelligence Review, Informatica, and the Journal of Computer
Science and Technology) and in conference proceedings (e.g., Research and
Development in Expert Systems IX, and the 21st ACM Computer Science
Conference). His technical interests include machine learning, expert systems,
intelligent database systems, and knowledge-based software engineering. He is
an editor on the Editorial Board of the Europe-based Informatica: An
International Journal of Computing and Informatics, and a member of the
Editorial Board of the U.S.A.-based International Journal of Computers and
Their Applications. He has taught courses in Combinatorial Mathematics,
Expert Systems, Knowledge Representation and Inference, Machine Learning,
Advanced Data Structures and Databases, Introduction to Computer Science, and
Artificial Intelligence.
PREREQUISITES
Databases, Expert Systems, and (preferably) Prolog.
------------------------------
End of ML-LIST (Digest format)
****************************************