Copy Link
Add to Bookmark
Report
Machine Learning List Vol. 5 No. 26
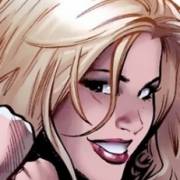
Machine Learning List: Vol. 5 No. 26
Saturday, Decemeber 18, 1993
Contents:
CFP: papers on Arts and Entertainment for AAAI-94
KDD-94
Intelligent Systems for Molecular Biology
New ML book info
The Machine Learning List is moderated. Contributions should be relevant to
the scientific study of machine learning. Mail contributions to ml@ics.uci.edu.
Mail requests to be added or deleted to ml-request@ics.uci.edu. Back issues
may be FTP'd from ics.uci.edu in pub/ml-list/V<X>/<N> or N.Z where X and N are
the volume and number of the issue; ID: anonymous PASSWORD: <your mail address>
----------------------------------------------------------------------
To: ml@ics.uci.edu
Subject: CFP: papers on Arts and Entertainment for AAAI-94
Date: Tue, 07 Dec 93 21:10:23 EST
Nils Nilsson recently presented the general Call for Papers for
AAAI-94. The conference also seeks technical papers in the area
of AI, Arts, and Entertainment. This is a new area for AAAI, and
it was not explicitly noted in the written call for participation.
Here is a brief description of the papers that are sought....
The American Association for Artificial Intelligence has decided
that the 1994 National AI conference should be different than it
sometimes has been perceived in recent years. AAAI-94 is intended
to emphasize new, exciting, innovative, and controversial research.
The reviewing process has been changed significantly to recognize
this broader spectrum of research.
One aspect of this change is the welcoming of technical papers
on AI, the Arts, and Entertainment. I will oversee reviews of
papers in this area, and I would like to encourage those of you
working on this and related themes to submit papers to AAAI-94.
The area is potentially broad, and includes basic and applied
study of AI and related technologies (such as artificial life,
neural networks, robotics, and genetic algorithms) in areas such
as:
- Film and video production
- Computer graphics and animation
- Interactive art (in any medium)
- Interactive fiction and role playing games
- Simulated worlds, virtual reality, video games
- Autonomous agents
- Believable interactive characters
- Music, sound, and speech
- Drama and story-telling
- Robotics, animatronics, toys
- Theme park applications
The Program Co-Chairs note:
Whether or not this restores the atmosphere of excitement,
innovation, controversy, and intellectual engagement we want
at our conference depends on one crucial variable: YOU. We
can accept only papers that are submitted. So, please submit
your most important, exciting, interesting, innovative, or
controversial papers to the AAAI-94 conference.
I believe this is a rare opportunity. I hope you will encourage
your colleagues and students to view AAAI-94 in this way, and to
submit an exciting paper in the area of AI, Arts, and Entertainment.
The deadline for submission is January 24. The detailed Call for
Participation is in the Fall 1993 AI Magazine (page 13). Yoy may
also receive information by sending email to NCAI@aaai.org.
Sincerely,
Joseph Bates
School of Computer Science
and College of Fine Arts
Carnegie Mellon University
------------------------------
Date: Sat, 11 Dec 93 15:52:17 PST
From: Usama Fayyad <fayyad@mathman.jpl.nasa.gov>
Subject: KDD-94
============================================================================
C a l l F o r P a p e r s
============================================================================
KDD-94: AAAI Workshop on Knowledge Discovery in Databases
Seattle, Washington, July 31-August 1, 1994
===========================================
Knowledge Discovery in Databases (KDD) is an area of common interest for
researchers in machine learning, machine discovery, statistics, intelligent
databases, knowledge acquisition, data visualization and expert systems. The
rapid growth of data and information created a need and an opportunity for
extracting knowledge from databases, and both researchers and application
developers have been responding to that need. KDD applications have been
developed for astronomy, biology, finance, insurance, marketing, medicine,
and many other fields. Core Problems in KDD include representation issues,
search complexity, the use of prior knowledge, and statistical inference.
This workshop will continue in the tradition of the 1989, 1991, and 1993 KDD
workshops by bringing together researchers and application developers from
different areas, and focusing on unifying themes such as the use of domain
knowledge, managing uncertainty, interactive (human-oriented) presentation,
and applications. The topics of interest include:
Applications of KDD Techniques
Interactive Data Exploration and Discovery
Foundational Issues and Core Problems in KDD
Machine Learning/Discovery in Large Databases
Data and Knowledge Visualization
Data and Dimensionality Reduction in Large Databases
Use of Domain Knowledge and Re-use of Discovered Knowledge
Functional Dependency and Dependency Networks
Discovery of Statistical and Probabilistic models
Integrated Discovery Systems and Theories
Managing Uncertainty in Data and Knowledge
Machine Discovery and Security and Privacy Issues
We also invite working demonstrations of discovery systems. The workshop
program will include invited talks, a demo and poster session, and panel
discussions. To encourage active discussion, workshop participation will be
limited. The workshop proceedings will be published by AAAI. As in previous
KDD Workshops, a selected set of papers from this workshop will be considered
for publication in journal special issues and as chapters in a book.
Please submit 5 *hardcopies* of a short paper (a maximum of 12 single-spaced
pages, 1 inch margins, and 12pt font, cover page must show author(s) full
address and E-MAIL and include 200 word abstract + 5 keywords) to reach the
workshop chairman on or before March 1, 1994.
Usama M. Fayyad (KDD-94) | Fayyad@aig.jpl.nasa.gov
AI Group M/S 525-3660 |
Jet Propulsion Lab | (818) 306-6197 office
California Institute of Technology | (818) 306-6912 FAX
4800 Oak Grove Drive |
Pasadena, CA 91109 |
************************************* I m p o r t a n t D a t e s **********
* Submissions Due: March 1, 1994 *
* Acceptance Notice: April 8, 1994 Final Version due: April 29, 1994 *
******************************************************************************
Program Committee
=================
Workshop Co-Chairs:
Usama M. Fayyad (Jet Propulsion Lab, California Institute of Technology)
Ramasamy Uthurusamy (General Motors Research Laboratories)
Program Committee:
Rakesh Agrawal (IBM Almaden Research Center)
Ron Brachman (AT&T Bell Laboratories)
Leo Breiman (University of California, Berkeley)
Nick Cercone (University of Regina, Canada)
Peter Cheeseman (NASA AMES Research Center)
Greg Cooper (University of Pittsburgh)
Brian Gaines (University of Calgary, Canada)
Larry Kerschberg (George Mason University)
Willi Kloesgen (GMD, Germany)
Chris Matheus (GTE Laboratories)
Ryszard Michalski (George Mason University)
Gregory Piatetsky-Shapiro (GTE Laboratories)
Daryl Pregibon (AT&T Bell Laboratories)
Evangelos Simoudis (Lockheed Research Center)
Padhraic Smyth (Jet Propulsion Laboratory)
Jan Zytkow (Wichita State University)
------------------------------
Date: Wed, 8 Dec 93 17:47:00 -0800
From: Doug Brutlag <brutlag@cmgm.stanford.edu>
Subject: Intelligent Systems for Molecular Biology
***************** CALL FOR PAPERS *****************
The Second International Conference on
Intelligent Systems for Molecular Biology
August 15-17, 1994
Stanford University
Organizing Committee Deadlines
Russ Altman, Stanford U, Stanford Papers due: March 11, 1994
Doug Brutlag, Stanford U, Stanford Replies to authors: April 29, 1994
Peter Karp, SRI, Menlo Park Revised papers due: May 27, 1994
Richard Lathrop, MIT, Cambridge
David Searls, U Penn, Philadelphia
Program Committee
K. Asai, ETL, Tsukuba A. Lapedes, LANL, Los Alamos
D. Benson, NCBI, Bethesda M. Mavrovouniotis, Northwestern U, Evanston
B. Buchanan, U of Pittsburgh G. Michaels, George Mason U, Fairfax
C. Burks, LANL, Los Alamos G. Myers, U. Arizona, Tucson
D. Clark, ICRF, London K. Nitta, ICOT, Tokyo
F. Cohen, UCSF, San Francisco C. Rawlings, ICRF, London
T. Dietterich, OSU, Corvallis J. Sallatin, LIRM, Montpellier
S. Forrest, UNM, Albuquerque C. Sander, EMBL, Heidelberg
J. Glasgow, Queen's U., Kingston J. Shavlik, U Wisconsin, Madison
P. Green, Wash U, St. Louis D. States, Wash U, St. Louis
M. Gribskov, SDSC, San Diego G. Stormo, U Colorado, Boulder
D. Haussler, UCSC, Santa Cruz E. Uberbacher, ORNL, Oak Ridge
S. Henikoff, FHRC, Seattle M. Walker, Stanford U, Stanford
L. Hunter, NLM, Bethesda T. Webster, Stanford U, Stanford
T. Klein, UCSF, San Francisco X. Zhang, TMC, Cambridge
The Second International Conference on Intelligent Systems for Molecular
Biology will take place at Stanford University in the San Francisco Bay
Area, August 14-17, 1994. The ISMB conference, held for the first time
last summer in Bethesda, MD, attracted an overflow crowd, yielded an
excellent offering of papers, invited speakers, posters and tutorials,
provided an exciting opportunity for researchers to meet and exchange
ideas, and was an important forum for the developing field. We will
continue the tradition of pre-published, rigorously refereed proceedings,
and opportunities for fruitful personal interchange.
The conference will bring together scientists who are applying the
technologies of advanced data modeling, artificial intelligence, machine
learning, probabilistic reasoning, massively parallel computing, robotics,
and related computational methods to problems in molecular biology. We
invite participation from both developers and users of any novel system,
provided it supports a biological task that is cognitively challenging,
involves a synthesis of information from multiple sources at multiple
levels, or in some other way exhibits the abstraction and emergent
properties of an "intelligent system." The four-day conference will
feature introductory tutorials (August 14), presentations of original
refereed papers and invited talks (August 15-17).
Paper submissions should be single-spaced, 12 point type, 12 pages
maximum including title, abstract, figures, tables, and bibliography with
titles. The first page should include the full postal address, electronic
mailing address, telephone and FAX number of each author. Also, please
list five to ten keywords describing the methods and concepts discussed
in the paper. State whether you wish the paper to be considered for oral
presentation only, poster presentation only or for either presentation
format. Submit 6 copies to the address below. For more information,
please contact ismb@camis.stanford.edu.
Proposals for introductory tutorials must be well documented, including
the purpose and intended audience of the tutorial as well as previous
experience of the author in presenting such material. Those considering
submitting tutorial proposals are strongly encouraged to submit a one-page
outline, before the deadline, to enable early feed-back regarding topic
and content suitability. The conference will pay an honorarium and
support, in part, the travel expenses of tutorial speakers.
Limited funds are available to support travel to ISMB-94 for those students,
post-docs, minorities and women who would otherwise be unable to attend..
Please submit papers and tutorial proposals to:
Intelligent Systems for Molecular Biology
c/o Dr. Douglas L. Brutlag
Beckman Center, B400
Department of Biochemistry
Stanford University School of Medicine
Stanford, California 94305-5307
------------------------------
Subject: New ML book info
From: alanh@xenon.dcs.kcl.ac.uk (Alan Hutchinson)
Forwarded-by: Sridhar Mahadevan <mahadeva@guardian.csee.usf.edu>
Date: Fri, 10 Dec 1993 14:32:48 GMT
The following decision tree is is heuristic, in keeping with the spirit of
the subject. Numbers in brackets are section numbers in chapters from
Algorithmic Learning (Oxford University Press, 1994) where the methods are
described in detail.
Is each example described by
- a few real numbers?
Is the problem solving task a case of
- optimizing a dynamical system?
Consider engineering control theory [3.1].
- associating outputs with inputs, where outputs depend
continuously or smoothly on inputs?
Consider a learner which remembers & interpolates [3.2].
- heuristic search with weighted rules?
Consider the bucket brigade algorithm [3.3].
- game playing, using weighted patterns?
Consider deeper search, or watching the opponent [3.4].
- prediction from algebraic laws which should be learned?
Consider Bacon [3.5].
- anything else?
Either rephrase the task or begin research.
- a lot of indistinguishable numbers or bits?
Does the training set include counter-examples, or does it
consist of input/output pairs?
Yes: Is the training set clean?
Yes: Consider WISARD or a logical neural net [4.3].
No: Consider a layered perceptron [4.2].
No: Does the task involve
- completing a blurred or partial image?
Consider a Boltzmann machine [4.4].
- discovering a decription of optimum examples?
Consider a genetic algorithm [4.6], [4.7].
- discovering a classification?
Consider a Kohonen net [4.5].
- anything else?
Either rephrase the task or begin research.
- a few distinct features whose values may be noisy?
Is there enough knowledge to partition the training set into
instances which can be regarded as clean, and other instances
which are suspect?
Yes: Consider learning from the clean and noisy subsets
separately by different methods.
No: Consider a statistical method 5.
Is the data structure used for clustering likely to be
- a context-free grammar:
Consider Wolff's method [5.2].
- a hierarchy:
Can the learner choose the kind of instance it
learns from, at each step?
Yes: Consider the Scott-Markovitch method [5.6].
No: Can the learning be non-incremental and can
each step of the classification be made on the
basis of a single feature?
Yes: Consider a decision tree algorithm [5.3].
No: Consider Al-Mathami's method [5.4].
- a graph showing interdependencies between features:
Consider the Fung-Crawford method [5.5].
- any other:
Consider designing a new clusterer, on the lines
suggested by Buntine [5.7.2].
- terms in some strict syntax, and is the learner's objective to
generalize the forms of the training instances?
Is the desired form of generalization
- a first order predicate formula in a theory not subject to
laws?
Consider Plotkin's method [6.2].
- an iterative program?
Consider program synthesis [6.3].
- a recursive definition?
Consider logic program synthesis [6.4], or
inference by the FunG/PredG method [6.5].
- any other:
Either rephrase the task or begin research.
- relations, free from noise?
Is the learner's objective to invent
- non-recursive conjunctive conditions or definitions?
Does the training set consist of
- classified examples and counter-examples?
Does the description language come in the form of
an upper semi-lattice?
Yes: Consider focussing [7.3.1].
No: Consider the version space method [7.3.2].
- only unclassified positive examples?
Consider Vere's method [6.1].
- non-recursive disjunctive conditions or definitions?
Consider decision trees [5.3], or rule ordering [7.6].
- recursive conditions?
Consider logic program synthesis [6.4], or
inference by the FunG/PredG method [6.5].
- an attempt at search, using heuristic rules?
Are all existing rules in the data base reliable?
Yes: Is the objective of learning to
- add rules with composite operators which reduce the
depth of search to a goal?
Can the search space be designed so that
- all operators are serially decomposable?
Consider macros [7.7.2].
- the task is a case of theorem proving?
Consider a deductive method:
EBG, lemma generation, or chunking [7.5].
- otherwise?
Should the learned rules be totally reliable?
Yes: Consider the STRIPS method [7.7.1].
No: Consider STRIPS [7.7.1], or
chunking [7.5.3].
- improve conflict resolution?
Does the learner have access to an ideal trace?
Yes: Consider rule ordering [7.6].
No: Consider the bucket brigade algorithm [3.3].
No: Is the objective of learning to discover
- flaws in the data base?
Does the learner have access to
- ideal traces?
Consider comparing the rule trace with an
ideal trace [7.2].
- an oracle?
Consider contradiction backtracing [7.2.2].
- neither?
Either rephrase the task or begin research.
- new rules with more reliable conditions?
Does the learner have access to an ideal trace?
Yes: Consider rule ordering [7.6].
No: Either rephrase the task or begin research.
- more reliable conflict resolution?
Does the learner have access to an ideal trace?
Yes: Consider rule ordering [7.6].
No: Consider the bucket brigade algorithm [3.3].
- any other?
Either rephrase the task or begin research.
Alan Hutchinson
Algorithmic Learning: Contents
Preface
How to choose a learning algorithm
Chapter 1
CHARACTERISTICS OF LEARNING ALGORITHMS
1.1 Characterisations of Training Sets
1.2 Forms of Individual Data
1.3 Solution Spaces
1.4 Learning and search
1.5 Classification of algorithms
1.6 What is learned information for?
1.7 Learning, aesthetics and common sense
1.8 Natural learning
1.9 Human learning
Further reading
Chapter 2
SOME BASIC IDEAS
2.1 Logic
2.2 Graphs
2.2.1 Covering trees
2.3 Search
2.3.1 Description languages
2.3.2 Reversible and irreversible search
2.3.3 Goal directed search
2.3.4 Monotonic operators and theorem proving
2.3.5 Summary properties of search tasks
2.3.6 Some primitive search algorithms
2.3.7 Heuristic functions
2.3.8 Some heuristic search algorithms
2.4 Metric Spaces
2.5 Experimenting with chance
2.6 Complexity of descriptions
Further reading
Chapter 3
LEARNING ALGORITHMS WITH NUMERIC INPUT
3.1 Optimising methods
3.2 Observing and Extrapolating
3.3 Controlling Search With Weights
3.3.1 The Bucket Brigade Algorithm
3.3.2 The Hierarchical Bucket Brigade Algorithm
3.4 Board Games
3.4.1 Learning by Deeper Search
3.4.2 Learning by Watching the Opponent
3.5 Discovering Physical Laws: Where The Classification Is Flawed
Further reading
Chapter 4
ASSOCIATION AND NEURAL NETWORKS
4.1 Perceptrons
4.2 Multi-layer perceptrons
4.2.1 Other forms of perceptrons
4.2.2 The back propagation algorithm
4.3 Logical neural nets
4.3.1 WISARD
4.3.2 A logical net which learns responses
4.4 Boltzmann Machines
4.4.1 Annealing
4.4.2 Learning in a Boltzmann Machine
4.5 Kohonen's feature maps
4.6 Optimizing Simple Behaviour
4.7 Genetic algorithms
4.8 Properties of neural nets and genetic algorithms
4.9 Comparison with Biological Systems
Further reading
Chapter 5
CLUSTERING AND CORRELATION
5.1 How Clustering Works
5.1.1 A Simple Clustering Algorithm
5.1.2 Aspects of the Clustering Process
5.1.3 Statistical and Conceptual Clustering
5.1.4 Ontological clusters
5.1.5 Combining concepts and statistics
5.2 Inference of grammars
5.2.1 Wolff's algorithm
5.3 Decision trees
5.3.1 Inventing A Decision Tree
5.3.2 Ontological nodes in decision trees
5.3.3 Entropy:
Why the Hamming distance gives a good choice of question
5.4 Building hierarchies: Winner takes all
5.4.1 Classification and prediction
5.4.2 Extending the hierarchy
5.5 Graphs of Properties
5.5.1 Finding Markov boundaries
5.6 Classifying by the effects of actions
5.6.1 Dido's representation hierarchy
5.6.2 Inheritance in the hierarchy
5.6.3 Dido's Experiments
5.6.4 Developing the hierarchy
5.6.5 Results
5.7 Survey of clustering methods
5.7.1 Comparisons
5.7.2 How to design a conceptual clusterer
5.8 Appendix: More on experiments and entropy
5.8.1 A short note on experiments
5.8.2 A more accurate estimate of entropy
5.8.3 Composite questions
5.8.4 Expected success rates
Further reading
Chapter 6
PATTERN MATCHING AND GENERALIZATION
6.1 Incorporating background information
6.1.1 Association chains
6.1.2 Background conditions for operators with side effects
6.2 Finding patterns of cause and effect
6.2.1 Underlying assumptions and restrictions
6.2.2 Substitutions
6.2.3 Generalizing terms
6.2.4 Generalizing clauses
6.2.5 Reducing a clause
6.2.6 Distinguishing murder from suicide
6.2.7 Unification
6.2.8 Generalizing and unifying subject to laws
6.3 Program synthesis
6.3.1 The programming language FP
6.3.2 Summary of program synthesis
6.3.3 Permitted input/output pairs
6.3.4 The simple program for a single input/output pair
6.3.5 Generalizing a sequence of simple programs
6.3.6 Choosing a suitable expression for d
6.3.7 Choosing a suitable expression for h
6.3.8 Choosing a suitable expression for e
6.3.9 Extended forms of synthesis
6.4 Other program synthesis methods
6.5 Inventing recursive definitions
6.5.1 The setting for inference
6.5.2 A simple form of the algorithm
6.5.3 Consequences of inappropriate bias
6.5.4 A crude solution: Broadening the search
6.5.5 A better solution: Adapting the language
6.5.6 A second version of the algorithm:
New variables and equality
6.5.7 Other aspects of the algorithm
6.6 Properties of syntactic generalization
Further reading
Chapter 7
LEARNING IN RULE BASED SYSTEMS
7.1 What Can Be Learned within a Rule Base
7.2 Tracing a Fault
7.2.1 Resolution Theorem Proving
7.2.2 Contradiction Backtracing
7.3 Inductive methods
7.3.1 Focussing
7.3.2 Version spaces and candidate elimination
7.4 Disjunctive Conditions
7.5 Deductive methods
7.5.1 Explanation based generalization
7.5.2 Lemma generation
7.5.3 Chunking
7.5.4 Some properties of deductive methods
7.6 Conflict resolution: Rule ordering
7.7 Inventing new actions
7.7.1 Composing conditions and effects of actions
7.7.2 Macros
7.8 Postscript
Further reading
Chapter 8
FURTHER DEVELOPMENTS
8.1 Theories of Learnability
8.1.1 Learnability of Grammars: Gold's Theorem
8.1.2 PAC learnability
8.1.3 Shattering and the VC dimension of a cover
8.2 Computability and learning
8.2.1 Computable extrapolations
8.2.2 Complexity and Occam's razor
8.2.3 Further aspects
8.3 The description language and bias
8.3.1 Expressive power
8.3.2 Extending a language is a learning task
8.3.3 Exploiting symmetry and redundancy
8.4 Theories of languages and logics
8.4.1 Meta languages
8.5 Some open issues
Further reading
References
------------------------------
End of ML-LIST (Digest format)
****************************************