Copy Link
Add to Bookmark
Report
Machine Learning List Vol. 6 No. 13
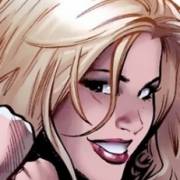
Machine Learning List: Vol. 6 No. 13
Tuesday, May 10, 1994
Contents:
Proc. Fam. WS Theory Revision at ECML-94
postdoctoral opening/invitation
Recruitment of Ph.D Neural Net Scientists
Advanced Tutorial on Learning DNF
Industrial applications of ML and comprehensibility
ML94/COLT94 advance program and registration
ANZIIS-94 Call for Papers
The Machine Learning List is moderated. Contributions should be relevant to
the scientific study of machine learning. Mail contributions to ml@ics.uci.edu.
Mail requests to be added or deleted to ml-request@ics.uci.edu. Back issues
may be FTP'd from ics.uci.edu in pub/ml-list/V<X>/<N> or N.Z where X and N are
the volume and number of the issue; ID: anonymous PASSWORD: <your mail address>
----------------------------------------------------------------------
Subject: Proc. Fam. WS Theory Revision at ECML-94
Date: Fri, 06 May 94 15:26:16 +0200
From: Stefan.Wrobel@gmd.de
As announced at ECML, the proceedings of the MLNet
Familiarization Workshop on Theory Revision
and Restructuring in Machine Learning at ECML-94, Catania, Italy,
are available via FTP and will appear as a GMD technical report:
"Proc. MLNet Familiarization Workshop on Theory Revision and
Restructuring in Machine Learning (at ECML-94, Catania, Italy),
ed. Stefan Wrobel, Arbeitspapiere der GMD, GMD, Pf. 1316,
53754 Sankt Augustin, Germany, 1994. Available via FTP from
ftp.gmd.de as /ml-archive/MLnet/Catania94/theory-revision.ps.gz."
If you cannot access FTP, or would like a printed copy, send
mail to stefan.wrobel@gmd.de.
------------------------------
From: Pat Langley <langley@flamingo.stanford.edu>
Subject: postdoctoral opening/invitation
Date: Mon, 9 May 94 19:20:36 -0700
I anticipate funding for a postdoctoral position in support of two
research projects, one focusing on machine learning for robotic
localization/navigation and the other on machine learning for computer
vision. Probabilistic approaches to induction will play a central role
in both efforts; thus, I am specifically looking for someone with
background in the formation of probabilistic concept hierarchies
and/or the construction of Bayesian networks. The position would
start in late 1994 or early 1995, and it would require significant
immersion in both the vision and robotics domains.
If you are interested in such a postdoctoral position, please contact
me at my new address:
Pat Langley
Robotics Laboratory
Computer Science Dept.
Stanford University
Stanford, CA 94305
(415) 725-8813 (work)
(415) 725-1449 (fax)
langley@cs.stanford.edu
For those who had not heard, I moved back to the bay area in January,
where I am splitting my time between Stanford University and ISLE, a
nonprofit corporation that specializes in machine learning research.
Although Stanford has considerable activity in machine learning, this
is distributed across many departments, and we have started a weekly
colloquium series on the topic as a way to increase communication among
local researchers. Naturally, we are always looking for speakers, so
if you plan to visit the bay area and would like to present your work
to an interdisciplinary audience that represents computer science,
statistics, pychology, electrical engineering, biochemistry, and other
fields, please let me know.
------------------------------
Date: Fri, 6 May 94 11:57 EDT
From: Teresa Kipp <kipp@nvl.army.mil>
Subject: Recruitment of Ph.D Neural Net Scientists
JOBS FOR TALENTED NEURAL NET Ph.D's
The Computer Vision Research Branch of the US Army Research Laboratory is
composed of Ph.D's in both theory and experimentation in the field of
theoretical computer science, probability and statistics. Our branch
has also contracts with top theoretical computer scientists and mathematicians
from various universities providing continuous interaction through regular
visits. Our research is to design algorithms to recognize military
targets in complex imagery generated by a variety of sensors. This research
also includes commercial applications such as handwriting and face recognition.
We are in the process of enlarging our branch by hiring neural net
scientists on our in-house staff and by contracting with university neural net
scientists. A Part of the new research effort is the comparison between neural
net algorithms and those presently designed by our branch that are model-based
with combinatorial trees in order to stimulate the cross fertilization, hybrid
systems, and the unification between these two approaches.
Talented neural net Ph.D's are invited to submit a copy of their curriculm
vitae by regular or electronic mail. Vitae's sent by electronic mail are
acceptable as either latex or postscript files. Send all
communications to Ms. Teresa Kipp at any of the following addresses:
electronic mail to:
kipp@nvl.army.mil
or send by regular mail to:
DEPARTMENT OF THE ARMY
US ARMY RESEARCH LABORATORY
AMSRL SS SK (T. KIPP)
10221 BURBECK RD STE 430
FT BELVOIR VA 22060-5806
or contact Ms. Kipp at (703)-704-3656.
------------------------------
From: Dan Roth <danr@das.harvard.edu>
Subject: Advanced Tutorial on Learning DNF
Date: Fri, 29 Apr 94 00:35:46 EDT
Advanced Tutorial on the State of the Art in Learning DNF Rules
===============================================================
Sunday, July 10, 1994
Rutgers University
New Brunswick, New Jersey
Held in Conjunction with the Eleventh International
Conference on Machine Learning (ML94, July 11-13, 1994)
and the Seventh Annual Conference on Computational
Learning Theory (COLT94, July 12-15, 1994).
Learning DNF rules is one of the most important problems
and widely-investigated areas of inductive learning from examples.
Despite its long-standing position in both the Machine Learning
and COLT communities, there has been little interaction between
them. This workshop aims to promote such interaction.
The COLT community has studied DNF extensively under its standard
learning models. While the general problem is still one of the main
open problems in COLT, there have been many exciting developments
in recent years, and techniques for solving major subproblems
have been developed.
Inductive learning of subclasses of DNF such as production rules,
decision trees and decision lists has been an active research topic
in the Machine Learning community for years, but theory has had almost
no impact on the experimentalists in machine learning working in this area.
The purpose of this workshop is to provide an opportunity for
cross-fertilization of ideas, by exposing each community to the other`s
ideas: ML researchers to the frameworks, results and techniques
developed in COLT; the theoretical community to many problems that are
important from practical points of view, but are not currently
addressed by COLT, as well as to approaches that were shown to work in
practice but lack a formal analysis.
To achieve this goal the workshop is organized around a set
of invited talks, given by some of the prominent researchers
in the field in both communities.
Our intention is to have as much discussion as possible during the
formal presentations.
The speakers are:
Nader Bshouty, University of Calgary, Canada
Learning via the Monotone Theory
Wray Buntine, NASA
Generating rule-based algorithms via graphical modeling
Tom Hancock, Siemens
Learning Subclasses of DNF from examples
Rob Holte, University of Ottawa, Canada
Empirical Analyses of Learning Systems
Jeff Jackson, Carnegie Mellon University
Learning DNF under the Uniform Distribution
Michael Kearns, AT&T Bell Labs
An Overview of Computational Learning Theory
Research on Decision Trees and DNF
Yishay Mansour, Tel-Aviv University, Israel
Learning boolean functions using the Fourier Transform.
Cullen Schaffer, CUNY
Learning M-of-N and Related Concepts
PARTICIPATION
The Workshop is open to people who register to the COLT/ML
conference. We hope to attract researchers that are active
in the area of DNF as well as the general COLT/ML audience.
WORKSHOP ORGANIZERS
Jason Catlett Dan Roth
AT&T Bell Laboratories Harvard University
Murray Hill, NJ 07974 Cambridge, MA 02138
+1 908 582 4978 +1 617 495 5847
catlett@research.att.com danr@das.harvard.edu
------------------------------
Date: Mon, 9 May 94 15:55:42 +0200
From: Yves.Kodratoff@lri.fr
Subject: Industrial applications of ML and comprehensibility
ECML'95 workshop on
Industrial applications of ML and comprehensibility
The importance of explanations and of comprehensibility of the results
provided by an expert system or a machine learning (ML) algorithm is by
no means a new idea. To my knowledge, it has been around since the 80's
(see details below), but I am almost sure that others realized its importance
before. This old idea did not attract much attention from a scientific
community more interested in measuring the complexity of the algorithms
and the accuracy of their results than the comprehensibility of the software
and of the results. This attitude can be explained by the fact that we have
no precise definition of what an explanation really is, that we have no way
of measuring or even analyzing what a "good" explanation is:
Comprehensibility is a badly defined concept, presently non measurable.
This state of facts seems to me unbearable now in view of the analysis of
the industrial applications done of the various ML approaches. Each time
one of our favorite ML approaches has been applied in industry, each time
the comprehensibility of the results, though ill-defined, has been a decisive
factor of choice over an approach by pure statistical means, or by neural
networks. To confirm this opinion, think over that, very recently, answering
questions about the difference between ML and more application
oriented data mining, G. Piatetski-Shapiro claimed that "Knowledge
discovery in Data Bases (KDD) is concerned with finding *understandable*
knowledge, while ML is concerned with improving performance of an
agent." Rather than discussing what properly belongs to ML or not, let us
rather ask the KDD community to join us.
This manifesto induces from these examples (and here is its weakness) that
a large number of industrial application of ML will demand good
explanations, as long as the domain is understood by the experts. We are well
aware that mechanizing one stage of a complex process may not require
comprehensibility, but we claim that the whole process, as soon as the
decisions it helps to make are important, will request a high level of
comprehensibility, for the experts to validate the system, for its
maintenance, and for its evolution in view of changes in the external
world. Now, let us deduce the consequences of our claim.
The problem we are left with is that we do not understand
comprehensibility! This is why I propose to stop fleeing before the
problem and define comprehensibility as an acknowledged research topic.
It is a hard problem, sure enough, but are we supposed to tackle with the
easy ones only? Now that it seems to be well identified as an industrial
problem, we so to say cannot "afford" to go on shunning it.
What kind of forces do we need to join in order to hope finding a solution?
We obviously need the MLists who developed the symbolic/numeric
systems able to generate understandable knowledge. Notice that we cannot
work in isolation from the users, the industrialists, who know empirically
what a good explanation is, or rather what a bad one is, and who are the
only ones able to attribute scores to the results of our future experiments.
Just as an example, the explanatory value of special "nuggets" has been
introduced in the ML community by P. Riddle because of her study of
manufacturing at Boeing, not to ease a tension internal to the ML field. The
KDD community, cited above, is obviously concerned. We need also
specialists in knowledge acquisition (KA), the research topic of which is
how to help a user to make his/her knowledge understandable to the
machine. They are thus used to work on the inverse problem to ours, and
their experience in the topic will be invaluable. Specialists in explanations
for the expert systems (ES) have already provided definitions and taxo-
nomies of explanations, they are the pioneers of the field: There exists now
a large body of workers that follow and deepen the ideas that led Clancey
to NEOMYCIN. Our problem would be more specifically to define a
measure of comprehensibility on the explanations generated by their systems.
Psychologists and more particularly pedagogists should be also part of this
game since they are used to analyze what a student understands really out
of a set of explanations, that is, what are for a human the internally genera-
ted explanations. Another type of interesting knowledge should come from
the specialists in the social sciences who could help us to define the social
contexts in which comprehensibility can take place. Finally, it is obvious
that statistics do not demand obscurity, and some efforts are done to ease
up the interpretations of the results. Those statisticians interested in these
efforts would be most welcome.
All this looks pretty well as a new theme for Cognitive Science, and we
must acknowledge that AI in general is deeply embedded in Cognitive
Science. Nevertheless, the ambiguous status of AI is again very typical
here since there are yet many problems, all relative to comprehensibility,
that are to be solved in the frame of Computer Science.
Let us start by underlining a few important problems related to Cognitive
Science. Since comprehension is perhaps the most context-dependent of all
human activities we cannot avoid holding positions in the symbolic/situated
cognition debate.
Can we define situated comprehensibility? Are we able to start an ontology
of the different contexts in which comprehension is possible?
What is the exact status of comprehensibility in a situated cognition? Do we
believe that the situated character of comprehension precludes communication,
that we must thus confuse lack of comprehensibility and situated? My
personal answer is no, but it is clearly an important debate, illustrated by
the industrial applications that rejected neural networks on the ground of
their lack of comprehensibility.
Do we follow Clancey in thinking that symbolic representation are simple
shadows of what we must explain? In our (symbolic) implementations
how can we evaluate the loss due to symbolization, and how can we
translate it to make it understandable to the human expert? How could it be
possible that the situated knowledge representations generated during
problem solving combine efficiency and comprehensibility?
I would also like to insist on four issues related to Computer Science be-
cause they are sometimes hidden by other concerns.
The first one, to the best of my knowledge, to work on these topics has
been R. S. Michalski who stated a "comprehensibility postulate" in his
famous paper on the star methodology. This work requests two remarks.
First remark is that the star algorithm can be well perceived as a statistical
classification method in which comprehensibility as been introduced as a
constraint on the description obtained. This shows that Michalski can be
credited of being the first scientist to create a program in which efficiency
and comprehensibility have been synthesized in the same algorithm. This
effort, which I think very important, opposes several subsequent attempts
to disconnect efficiency and comprehensibility in different and even
possibly unrelated modules. At any rate, this choice should be discussed
and explained. The second remark is that when Michalski gives an
overview of ML a few pages before, co-authoring with others, he
describes ML surprisingly enough without the smallest hint to his own
concept of comprehensibility. This shows how still shocking is for some
people the idea of a work taking into account ill-defined comprehensibility.
The first to work on industrial applications of ML, D. Michie, often stated
in front of our community, for instance in his address at EWSL'87 in
Bled, that one of the main features of ID3-like algorithms, as opposed to
so many statistical systems that use also information compression, is their
ability to generate easy to understand decision trees. I remember also that at
this meeting, I. Bratko argued that, depending on the experts, decision
trees might be more understandable than the rules one extracts from them.
All these are early examples of the realization that comprehensibility is an
essential factor to an ML algorithm. As stated above, this has been
confirmed many times by subsequent industrial applications. From the
research point of view, it underlines that comprehensibility-decreasing
changes in the representation should be carefully considered before
acceptance. A thorough discussion of the importance of learning
hyperrectangles, obviously leading to understandable results is needed, together
with a look at the possible ways to make understandable other approaches
using other shapes to cover the examples. People that used diagonals or
ellipses have always justified their approach by an increase in accuracy. It
is not sure at all that they always kill comprehension, it is probable that a
representational change is needed in such a way that it will lead to even
better further understanding. More generally, all people concerned by
changes in representation or invention of new predicates, as for instance
people working on constructive induction, should be also interested by our
proposal.
Another topic of interest should be "knowledge architectured" neural
networks (NN) la Shavlik who has shown very neatly that introducing
knowledge to built the network, and to compute its initial weights and
activation thresholds, not only increases accuracy, but also helps
subsequent interpretation of the learned NN by rules containing n-of-m
conditions. Even more easily, genetic algorithms (GA) can be tuned in
such a way that the strings of bits that are learned are easily translated back
into meaningful information.
A last example, yet not acknowledged as linked to comprehensibility, I
would like to cite is the effort for avoid absurd classifications that
recognize an irrelevant item as belonging to a class. Such is, for example,
W. Emde's reaction to the old car recognized has being sick of the measles
by a knowledge-based system. Even if the system is supposedly equipped
of the best explanatory mechanisms, it would have hard times explaining
this result in any convincing way. This example shows well that they are
other measurable quantities than accuracy, here the amount of falsely
recognized items in the test set, that measure some amount of
comprehensibility. From Emde's preliminary experiments it is clear that
decreasing the amount of false recognition may also decrease dramatically
accuracy. What are we to choose, accuracy or no false recognition? Is it
possible to preserve accuracy in some ways? What is the best architecture
that would allow us to get alternatively accurate, or (exclusive and not
exclusive or) non absurd results? Similarly, let us cite G. Nakhaeizadeh
results at Daimler-Benz. I know that he was not at all inspired by
comprehensibility of results, but by immediate industrial concerns. Yet, he
and his group devised a cost-driven ID3 which avoids performing false
recognition that would be very expensive. As Emde, he acknowledges that
in some cases he obtains huge decreases in accuracy when optimizing low
cost.
As you can see, the community is not really empty-handed when facing the
problem of understandable learning, and I am convinced that we shall be
able to find very soon objective definitions of what comprehensibility is,
with the help of the users to judge our results, with the combined forces of
ML, KDD, KA, explanatory ES, knowledge intensive NN and GA,
pedagogy, sociology, and of statisticians eager to communicate better with
their users.
This is why I invite all interested parties to join a workshop on
"Industrial applications of ML and comprehensibility", I plan to organize
next to ECML'95 in Heraklion. Would not it be a beautiful symbol that a
new science comes to existence so near to Knossos where the Labyrinth
has been built a few years ago? Before sending papers, send me your view
of the problem of comprehensibility or your industrial experience, and how
you could contribute to the workshop, even if you cannot join physically
(we have to set up a programme committee, define topics, evaluation
criteria, etc.).
The topic of the workshop should be essentially an in-depth discussion of
new industrial applications from the point of view of comprehensibility,
and the experimental settings by which we could start measuring the value
of an explanation, and the comprehensibility of a string of symbols. This
includes all kinds of discussions relative to the definition of what an
explanation is, and how to evaluate the comprehensibility of an
explanation. Optimists can even start thinking on which kind of theories
we should use to take comprehensibility into account: the hunt for
"probably approximately comprehensible" learning is open!
------------------------------
Date: Thu, 28 Apr 94 16:53 EDT
From: William Cohen <wcohen@research.att.com>
Subject: ML94/COLT94 advance program and registration
This advance program for COLT and ML replaces a preliminary
announcement that was distributed in March.
An unabbreviated version of this announcement in Latex or postscript
can be obtained via anonymous ftp from cs.rutgers.edu in the directory
/pub/learning94/registration-information, and also from our www site
at http://www.cs.rutgers.edu/pub/learning94/learning94.html. If you
do not have access to ftp/www, send email to ml94@cs.rutgers.edu or
colt94@research.att.com.
*=*=*=*=*=*=*=*=*=*=*=*=*=*=*=*=*=*=*=*=*=*=*=*=*=*=*=*=*=*=*=*=*=*=*=*=*
--- Advance Program ---
ML '94 COLT '94
Eleventh International Conference Seventh ACM Conference on
on Machine Learning Computational Learning Theory
July 10-13, 1994 July 12-15, 1994
Rutgers, The State University of New Jersey, New Brunswick
*-*-*-*-*-*-*-*-*-*-*-*-*-*-*-*-*-*-*-*-*-*-*-*-*-*-*-*-*-*-*-*-*-*-*-*-*
The COLT and ML conferences will be held together this year at Rutgers
University in New Brunswick. This is the first time that COLT and ML
will be held in the same location, and we are looking forward to a
lively and interdisciplinary meeting of the two communities. Please
come and help make this exciting experiment a success.
Among the highlights of the conferences are three invited lectures,
and, on Sunday, July 10, a day of workshops and tutorials on a variety
of topics relevant to machine learning. The tutorials are sponsored
by the Center for Discrete Mathematics and Theoretical Computer
Science (DIMACS), and are free and open to the general public. COLT
is sponsored by the ACM Special Interest Groups on Algorithms and
Computation Theory (SIGACT) and on Artificial Intelligence (SIGART).
In addition, COLT and ML received generous support this year from AT&T
Bell Laboratories and the NEC Research Institute.
>>>> WARNING <<<< The dates of the conferences coincide this year
with the World Cup soccer matches being held at Giants Stadium in East
Rutherford, New Jersey. These games are expected to be the largest
sporting event ever held in the New York metropolitan area, and it is
possible that the volume of soccer fans in the area could adversely
affect your ability to make travel reservations. Therefore, IT IS
EXTREMELY IMPORTANT THAT YOU MAKE ALL YOUR TRAVEL ARRANGEMENTS AS
EARLY AS POSSIBLE.
GENERAL INFORMATION
LOCATION. The conferences will be held at the College Avenue Campus
of Rutgers University in downtown New Brunswick, which is easily
accessible by air, train, and car. For air travel, New Brunswick is
35 minutes from Newark International Airport, a major U.S. and
international airline hub. By rail, the New Brunswick train station
is located less than four blocks from the conference site and is on
Amtrak's Northeast corridor. For travel by car, the conference site
is approximately three miles from Exit 9 of the New Jersey Turnpike.
See instructions below for obtaining a map of the campus. Most
conference activities will take place in Scott Hall (#21 on map) and
Murray Hall (#22). Conference check-in and on-site registration will
take place in Scott Hall (follow signs for exact room location) on
Saturday, July 9 at 3-6pm, and everyday after that beginning at 8am.
REGISTRATION. Please complete the attached registration form, and
return it with a check or money order for the full amount. The early
registration (postmark) deadline is May 27, 1994.
HOUSING. We have established the group rate of $91/night for a single
or a double at the HYATT REGENCY HOTEL (about five blocks from the
conference site). This rate is only guaranteed through June 10, 1994,
and, due to limited availability, it is strongly recommended that you
make reservations as soon as possible. To reserve a room, please
contact the Hyatt directly and be sure to reference ML94/COLT94 (phone
908-873-1234 or 800-233-1234; fax 908-873-1382; or write Hyatt
Regency, 2 Albany Street, New Brunswick, NJ 08901 USA). Parking is
available at the hotel for a discounted $3/night.
We have also reserved dormitory space in two dorms, both of which are
an easy walk to the main conference site. Dorm reservations must be
made by the early registration deadline of May 27, 1994. Both dorms
include daily maid service (linens provided first day for the week and
daily fresh towels and beds made). The Stonier Hall dorms (#56 on
map) are air-conditioned with private bath and are situated in the
center of the campus. Due to limited availability, only shared double
rooms are available in Stonier. Only a block away, the Campbell Hall
dorms (#50) are one of a set of three "river dorms" overlooking the
Raritan River. Although Campbell Hall is not air-conditioned, the
view of the river is quite pleasing and rooms on the river side should
offer good air flow. Baths in Campbell are shared on each floor, with
single and double rooms available.
Please specify your dorm preference on your registration form, and we
will assign space accordingly on a first come, first served basis as
long as rooms are available. Unfortunately, because there are only a
finite number of rooms within each dormitory, we cannot absolutely
guarantee your request. Check-in for the dorms will take place at the
Housing Office in Clothier Hall (#35) which is located next to the
Hurtado Health Center (#37) on Bishop Place. Check-in hours will be
4pm to midnight, July 9-13.
TRAVEL BY AIR. Newark International Airport is by far the most
convenient. A taxi from the airport to New Brunswick costs about $36
(plus nominal tolls) for up to four passengers. (This is the
flat-rate fare for a _licensed_ taxi from the official-looking taxi
stand; it is strongly recommended that you refuse rides offered by
unlicensed taxi drivers who may approach you elsewhere in the
airport.) Shuttle service to New Brunswick is available from ICS for
$23 per person. ICS shuttles run direct to the Hyatt, and require at
least one-day advance reservations (908-566-0795 or 800-225-4427). If
renting a car, follow signs out of the airport to New Jersey Turnpike
South, and continue with the directions below. By public
transportation, take the Airlink bus ($4 exact fare) to Newark Penn
Station and follow the "by rail" directions below. (New Jersey
Transit train fare is $5.25 one-way or $8 round trip excursion; trains
run about twice an hour during the week, and less often in the evening
and on weekends.)
TRAVEL BY CAR. Take the New Jersey Turnpike (south from Newark or New
York, north from Philadelphia) to Exit 9. Follow signs onto Route 18
North or West (labeled differently at different spots) toward New
Brunswick. Take the Route 27, Princeton exit onto Albany Street
(Route 27) into downtown New Brunswick. The Hyatt Regency Hotel will
be on your left after the first light. If staying at the Hyatt, turn
left at the next light, Neilson Street, and left again into the front
entrance of the hotel. If staying in the dorms, continue past this
light to the following light, George Street, and turn right. Stay on
George Street to just before the fifth street and turn left into the
Parking Deck (#55 on map). Walk to the Housing Office in Clothier
Hall (#35) for dormitory check-in. Parking passes will be provided to
all conference registrants.
TRAVEL BY RAIL. Take either an Amtrak or a New Jersey Transit train
to the New Brunswick train station. This is located at the corner of
Albany Street and Easton Avenue. If staying at the Hyatt Regency
Hotel, it is a (long) three block walk to the left on Albany Street to
the hotel. If staying in the dorms it is a (long) six block walk to
the Housing Office in Clothier Hall (#35 on map) for dormitory
check-in. (The taxi stand is in front of the train station on Albany
Street.)
MEALS. Continental breakfast is included with registration, but not
lunch or dinner. Restaurants abound within walking distance of the
conference and housing venue, ranging from inexpensive food geared to
college students to more expensive dining.
A reception on July 12 is scheduled at the rustic Log Cabin, situated
next to the experimental gardens of the agricultural campus, as part
of the registration package for all ML94 and COLT94 attendees. The
banquet on July 13 is included in the registration package for
everyone except students.
CLIMATE. New Jersey in July is typically hot, with average daily
highs around 85 degrees, and overnight lows around 70. Most days in
July are sunny, but also come prepared for the possibility of
occasional rain.
THINGS TO DO. The newly opened Liberty Science Center is a fun,
hands-on science museum located in Liberty State Park, about 30-45
minutes from New Brunswick (201-200-1000). From Liberty State Park,
one can also take a ferry to the Statue of Liberty and the Immigration
Museum at Ellis Island.
New York City can be reached in under an hour by rail on New Jersey
Transit. Trains run about twice an hour during the week, and once an
hour on weekends and at night. Fare is $7.75 one-way, $11.50 round
trip excursion.
New Brunswick has a number of theaters, including the State Theater
(908-247-7200), the George Street Playhouse (908-246-7717), and the
Crossroads Theater (908-249-5560).
The New Jersey shore is less than an hour from New Brunswick. Points
along the shore vary greatly in character. Some, such as Point
Pleasant, have long boardwalks with amusement park rides, video
arcades, etc. Others, such as Spring Lake, are quiet and
uncommercialized with clean and very pretty beaches. Further south,
about two hours from New Brunswick, are the casinos of Atlantic City.
You can walk for miles and miles along the towpath of the peaceful
Delaware and Raritan Canal which runs from New Brunswick south past
Princeton.
Your registration packet will include a pass for access to the College
Avenue Gymnasium (near the dormitories, #77 on map).
FURTHER INFORMATION. If you have any questions or problems, or if you
have special needs due to a disability, please send email to
colt94@research.att.com or ml94@cs.rutgers.edu.
Further conference information is available via anonymous ftp from
cs.rutgers.edu in the directory /pub/learning94. Also, users of www
information servers, such as mosaic, can find the information at
http://www.cs.rutgers.edu/pub/learning94/learning94.html. Available
information includes a map of the campus, abstracts of
workshops/tutorials, updates of this announcement, and an application
for ACM/SIG membership.
For New Jersey Transit fare and schedule information, call
800-772-2222 (in New Jersey) or 201-762-5100 (out-of-state).
TECHNICAL PROGRAM
The combined technical program for COLT and ML is given below. All
talks will be held in Scott Hall (#21 on map) and Murray Hall (#22),
exact room locations to be announced.
Monday, July 11
==============================================================================
| Welcome: 8:45
|-----------------------------------------------------------------------------
| ML SESSION 1: 9:00-10:00 Chair: R. Greiner
|
| 9:00 Rule induction for semantic query optimization--
| Chun-Nan Hsu, Craig Knoblock
| 9:30 Heterogeneous uncertainty sampling for supervised learning--
| David D. Lewis, Jason Catlett
|-----------------------------------------------------------------------------
| BREAK: 10:00-10:30
|-----------------------------------------------------------------------------
| ML SESSION 2: 10:30-12:00
|
| Chair: T. Dietterich
| 10:30 Prototype and feature selection by sampling and random
| mutation hill climbing algorithms--David B. Skalak
| 11:00 Irrelevant features and the subset selection
| problem--George H. John, Ron Kohavi, Karl Pfleger
| 11:30 Greedy attribute selection--Richard A. Caruana, Dayne Freitag
|
| Chair: C. Sammut
| 10:30 A constraint-based induction algorithm in FOL--Michele Sebag
| 11:00 Learning recursive relations with randomly selected small
| training sets--David W. Aha, Stephane Lapointe,
| Charles Ling, Stan Matwin
| 11:30 Improving accuracy of incorrect domain theories--Lars Asker
|
| Chair: E. Baum
| 10:30 The generate, test and explain discovery system architecture--
| Michael de la Maza
| 11:00 Learning disjunctive concepts by means of genetic algorithms--
| A. Giordana, L. Saitta, F. Zini
| 11:30 Hierarchical self-organization in genetic programming--
| Justinian Rosca, Dana Ballard
|-----------------------------------------------------------------------------
| LUNCH: 12:00-2:00
|-----------------------------------------------------------------------------
| ML SESSION 3: 2:00-3:30 Chair: M. Pazzani
|
| 2:00 Combining top-down and bottom-up techniques in inductive logic
| programming--John M. Zelle, Raymond Mooney, Joshua Konvisser
| 2:30 Towards a better understanding of memory-based reasoning systems--
| John Rachlin, Simon Kasif, Steven Salzberg, David Aha
| 3:00 Using genetic search to refine knowledge-based neural networks--
| David W. Opitz, Jude Shavlik
|-----------------------------------------------------------------------------
| BREAK: 3:30-4:00
|-----------------------------------------------------------------------------
| ML SESSION 4: 4:00-5:30
|
| Chair: A. Prieditis
| 4:00 Consideration of risk in reinforcement learning--Matthias Heger
| 4:30 Markov games as a framework for multi-agent reinforcement learning--
| Michael Littman
| 5:00 Learning without state-estimation in partially observable Markovian
| decision processes--Satinder Pal Singh, Tommi Jaakkola,
| Michael I. Jordan
|
| Chair: L. Saitta
| 4:00 Comparing methods for refining certainty-factor rule-bases--
| J. Jeffrey Mahoney, Raymond Mooney
| 4:30 Getting the most from flawed theories--Moshe Koppel, Alberto
| Segre, Ronen Feldman
| 5:00 Revision of production system rule-bases--Patrick M. Murphy,
| Michael J. Pazzani
|
| Chair: J. Catlett
| 4:00 A new method for predicting protein secondary structures based
| on stochastic tree grammars--Naoki Abe, Hiroshi Mamitsuka
| 4:30 A powerful heuristic for the discovery of complex patterned
| behavior--Raul E. Valdes-Perez, Aurora Perez
| 5:00 Reducing misclassification costs--Michael J. Pazzani, Christopher
| Merz, Patrick M. Murphy, Kamal M. Ali, Timothy Hume, Clifford Brunk
==============================================================================
Tuesday, July 12
==============================================================================
|
| ML SESSION 5: 8:30-10:00 Chair: L. Kaelbling
|
| 8:30 A modular Q-learning architecture for manipulator task
| decomposition--Chen Tham, Richard Prager
| 9:00 On the worst-case analysis of temporal-difference learning
| algorithms--Robert E. Schapire, Manfred K. Warmuth
| 9:30 To discount or not to discount in reinforcement learning: A case
| study comparing R learning and Q learning--Sridhar Mahadevan
|-----------------------------------------------------------------------------
| BREAK: 10:00-10:30
|-----------------------------------------------------------------------------
| ML SESSION 6: 10:30-12:00
|
| Chair: L. Pratt
| 10:30 A Baysian framework to integrate symbolic and neural learning--
| Irina Tchoumatchenko, Jean Gabriel Ganascia
| 11:00 Boosting and other machine learning algorithms--Harris Drucker,
| Corinna Cortes, L. D. Jackel, Yann LeCun, Vladimir Vapnik
| 11:30 Using sampling and queries to extract rules from trained neural
| networks--Mark W. Craven, Jude Shavlik
|
| Chair: K. Yamanishi
| 10:30 The minimum description length principle and categorical theories--
| J. R. Quinlan
| 11:00 An efficient subsumption algorithm for inductive logic programming--
| Jorg-Uwe Kietz, Marcus Lubbe
| 11:30 Selective reformulation of examples in concept learning--
| Jean-Daniel Zucker, Jean Gabriel Ganascia
|
| Chair: C. Schaffer
| 10:30 Small sample decision tree pruning--Sholom Weiss, Nitin Indurkhya
| 11:00 An improved algorithm for incremental induction of decision trees--
| Paul Utgoff
| 11:30 Incremental reduced error pruning--Johannes Furnkranz, Gerhard Widmer
|-----------------------------------------------------------------------------
| LUNCH: 12:00-1:50
|-----------------------------------------------------------------------------
| INVITED TALK: 1:50-3:00
|
| 1:50 Stephen Muggleton--Recent advances in inductive logic programming.
|-----------------------------------------------------------------------------
| BREAK: 3:00-3:30
|-----------------------------------------------------------------------------
| COLT SESSION 1: 3:30-4:40 Chair: A. Blum
|
| 3:30 Classic learning--Michael Frazier, Leonard Pitt
| 3:55 Learning probabilistic automata with variable memory length--
| Dana Ron, Yoram Singer, Naftali Tishby
| 4:20 On a learnability question associated to neural networks with
| continuous activations--Bhaskar DasGupta, Hava T. Siegelmann,
| Eduardo Sontag
| 4:30 Learning with malicious membership queries and exceptions--
| Dana Angluin, Martins Krickis
|-----------------------------------------------------------------------------
| BREAK: 4:40-4:50
|-----------------------------------------------------------------------------
| COLT IMPROMPTU TALKS: 4:50 onward
|-----------------------------------------------------------------------------
| RECEPTION: time and location TBA
==============================================================================
Wednesday, July 13
==============================================================================
| ML SESSION 7: 8:30-10:00 Chair: D. Aha
|
| 8:30 Efficient algorithms for minimizing cross validation error--
| Mary Lee, Andrew W. Moore
| 9:00 A conservation law for generalization performance--Cullen Schaffer
| 9:30 In defense of C4.5: notes on learning one-level decision trees--
| Tapio Elomaa
|-----------------------------------------------------------------------------
| BREAK: 10:00-10:25
|-----------------------------------------------------------------------------
| COLT SESSION 2: 10:25-12:05 Chair: P. Tadepalli
|
| 10:25 Efficient agnostic PAC-learning with simple hypotheses--
| Wolfgang Maass
| 10:50 Rigorous learning curve bounds from statistical mechanics--
| David Haussler, Michael Kearns, H. Sebastian Seung, Naftali Tishby
| 11:15 Efficient reinforcement learning--Claude-Nicolas Fiechter
| 11:40 An optimal-control application of two paradigms of on-line
| learning--V. G. Vovk
|-----------------------------------------------------------------------------
| LUNCH: 12:05-1:50
|-----------------------------------------------------------------------------
| INVITED TALK: 1:50-3:00
|
| 1:50 Fernando Pereira--Frequencies vs. biases: Machine learning
| problems in natural language processing.
|-----------------------------------------------------------------------------
| BREAK: 3:00-3:30
|-----------------------------------------------------------------------------
| ML SESSION 8: 3:30-4:30
|
| Chair: D. Kibler
| 3:30 An incremental learning approach for completable planning--
| Melinda T. Gervasio, Gerald F. DeJong
| 4:00 Learning by experimentation: incremental refinement of incomplete
| planning domains--Yolanda Gil
|
| Chair: S. Mahadevan
| 3:30 Reward functions for accelerated learning--Maja Mataric
| 4:00 Incremental multi-step Q-learning--Jing Peng, Ronald Williams
|-----------------------------------------------------------------------------
| BREAK: 4:30-4:45
|-----------------------------------------------------------------------------
| COLT IMPROMPTU TALKS: 4:45 onward
|-----------------------------------------------------------------------------
| ML BUSINESS MEETING: 4:45 onward
|-----------------------------------------------------------------------------
| BANQUET: time and location TBA
==============================================================================
Thursday, July 14
==============================================================================
| COLT SESSION 3: 8:30-10:10 Chair: T. Zeugmann
|
| 8:30 On learning read-k-satisfy-j DNF--Avrim Blum, Roni Khardon,
| Eyal Kushilevitz, Leonard Pitt, Dan Roth
| 8:55 On the limits of proper learnability of subclasses of DNF formulas--
| Krishnan Pillaipakkamnatt, Vijay Raghavan
| 9:20 Oracles and queries that are sufficient for exact learning--
| Nader H. Bshouty, Richard Cleve, Sampath Kannan, Christino Tamon
| 9:45 Learning structurally reversible context-free grammars
| from queries and counterexamples in polynomial time--Andrey Burago
|-----------------------------------------------------------------------------
| BREAK: 10:10-10:35
|-----------------------------------------------------------------------------
| COLT SESSION 4: 10:35-12:05 Chair: N. Bshouty
|
| 10:35 Inference and minimization of hidden Markov chains--
| David Gillman, Michael Sipser
| 11:00 Playing the matching-shoulders lob-pass game with
| logarithmic regret--Joe Kilian, Kevin Lang, Barak Pearlmutter
| 11:25 Learning monotone log-term DNF formulas--Yoshifumi Sakai, Akira Maruoka
| 11:35 Minimum L-complexity algorithm and its applications to learning
| non-parametric rules--Kenji Yamanishi
| 11:45 Approximate methods for sequential decision making using
| expert advice--Thomas H. Chung
| 11:55 Co-learning of total recursive functions--Rusins Freivalds,
| Marek Karpinski, Carl H. Smith
|-----------------------------------------------------------------------------
| LUNCH: 12:05-1:50
|-----------------------------------------------------------------------------
| INVITED TALK: 1:50-3:00
|
| 1:50 Michael Jordan--Hidden decision tree models.
|-----------------------------------------------------------------------------
| BREAK: 3:00-3:30
|-----------------------------------------------------------------------------
| COLT SESSION 5: 3:30-4:30 Chair: T. Hancock
|
| 3:30 Learning unions of boxes with membership and equivalence
| queries--Paul W. Goldberg, Sally A. Goldman, H. David Mathias
| 3:40 An optimal parallel algorithm for learning DFA--
| Jose L. Balcazar, Josep Diaz, Ricard Gavalda, Osamu Watanabe
| 3:50 On learning counting functions with queries--
| Zhixiang Chen, Steven Homer
| 4:00 Geometrical concept learning and convex polytopes--Tibor Hegedus
| 4:10 Learning with queries but incomplete information--
| Robert H. Sloan, Gyorgy Turan
| 4:20 Learning one-dimensional geometric patterns under one-sided random
| misclassification noise--Paul W. Goldberg, Sally A. Goldman
|-----------------------------------------------------------------------------
| BREAK: 4:30-4:45
|-----------------------------------------------------------------------------
| COLT IMPROMPTU TALKS: 4:45 onward
|-----------------------------------------------------------------------------
| COLT POSTER SESSION: 7:00-8:30
|-----------------------------------------------------------------------------
| COLT BUSINESS MEETING: 8:30
==============================================================================
Friday, July 15
==============================================================================
| COLT SESSION 6: 8:25-10:05 Chair: W. Gasarch
|
| 8:25 The representation of recursive languages and its impact
| on the efficiency of learning--Steffen Lange
| 8:50 The strength of noninclusions for teams of finite learners--
| Martin Kummer
| 9:15 On the intrinsic complexity of language identification--
| Sanjay Jain, Arun Sharma
| 9:40 Inclusion problems in parallel learning and games--
| Martin Kummer, Frank Stephan
|-----------------------------------------------------------------------------
| BREAK: 10:05-10:25
|-----------------------------------------------------------------------------
| COLT SESSION 7: 10:25-12:05 Chair: M. Kearns
|
| 10:25 Fat-shattering and the learnability of real-valued functions--
| Peter L. Bartlett, Philip M. Long, Robert C. Williamson
| 10:50 On learning arithmetic read-once formulas with exponentiation--
| Daoud Bshouty, Nader H. Bshouty
| 11:15 Exploiting random walks for learning--Peter L. Bartlett,
| Paul Fischer, Klaus-Uwe Hoffgen
| 11:40 Learning from a consistently ignorant teacher--Mike Frazier,
| Sally Goldman, Nina Mishra, Leonard Pitt
|-----------------------------------------------------------------------------
| LUNCH: 12:05-1:50
|-----------------------------------------------------------------------------
| COLT SESSION 8: 1:50-3:30 Chair: S. Solla
|
| 1:50 Learning linear threshold functions in the presence of
| classification noise--Tom Bylander
| 2:15 Efficient learning of continuous neural networks--Pascal Koiran
| 2:40 Generalization in partially connected layered neural networks--
| Kyung-Hoon Kwon, Kukjin Kang, Jong-Hoon Oh
| 3:05 Lower bounds on the VC-dimension of smoothly parametrized function
| classes--Wee Sun Lee, Peter L. Bartlett, Robert C. Williamson
==============================================================================
WORKSHOPS AND DIMACS-SPONSORED TUTORIALS
On Sunday, July 10, we are pleased to present four all-day workshops,
five half-day tutorials, and one full-day advanced tutorial. The
DIMACS-sponsored tutorials are free and open to the general public.
Participation in the workshops is also free, but is at the discretion
of the workshop organizers. Note that some of the workshops have
quickly approaching application deadlines. Please contact the
workshop organizers directly for further information. Some
information is also available via ftp/www (see "further information"
above).
Morning sessions will be held 8:45am-12:15pm, with a half hour break
at 10:15. Afternoon sessions will be held 2-5:30pm, with a half hour
break at 3:30. For workshops W1 and W2, please contact the workshop
organizers for times of evening sessions.
All workshops and tutorials will be held in Scott Hall (#21 on map)
and Murray Hall (#22); exact room locations will be posted.
TUTORIALS:
T1. State of the art in learning DNF rules morning/afternoon
(advanced tutorial)
Dan Roth danr@das.harvard.edu
Jason Catlett catlett@research.att.com
T2. Descriptional complexity and inductive learning morning
Ed Pednault epdp@research.att.com
T3. Computational learning theory: introduction and survey morning
Lenny Pitt pitt@cs.uiuc.edu
T4. What does statistical physics have to say about learning? morning
Sebastian Seung seung@physics.att.com
Michael Kearns mkearns@research.att.com
T5. Reinforcement learning afternoon
Leslie Kaelbling lpk@cs.brown.edu
T6. Connectionist supervised learning--an engineering afternoon
approach
Tom Dietterich tgd@research.cs.orst.edu
Andreas Weigend andreas@cs.colorado.edu
WORKSHOPS:
W1. Robot Learning morning/afternoon/evening
Sridhar Mahadevan mahadeva@csee.usf.edu
W2. Applications of descriptional complexity to afternoon/evening
inductive, statistical and visual inference
Ed Pednault epdp@research.att.com
W3. Constructive induction and change of morning/afternoon
representation
Tom Fawcett fawcett@nynexst.com
W4. Computational biology and machine learning morning/afternoon
Mick Noordewier noordewi@cs.rutgers.edu
Lindley Darden darden@umiacs.umd.edu
REGISTRATION FOR COLT94/ML94
Please complete the registration form below, and mail it with your
payment for the full amount to:
Priscilla Rasmussen, ML/COLT'94
Rutgers, The State University of NJ
Laboratory for Computer Science Research
Hill Center, Busch Campus
Piscataway, NJ 08855
(Sorry, registration cannot be made by email, phone or fax.) Make
your check or money order payable in U.S. dollars to Rutgers
University. For early registration, and to request dorm housing, this
form must be mailed (via airmail, if outside the U.S.) by May 27,
1994. For questions about registration, please contact Priscilla
Rasmussen (rasmussen@cs.rutgers.edu; 908-932-2768).
Name: _____________________________________________________
Affiliation: ______________________________________________
Address: __________________________________________________
___________________________________________________________
Country: __________________________________________________
Phone: _______________________ Fax: _______________________
Email: ____________________________________________________
Confirmation will be sent to you by email.
REGISTRATION. Please circle the *one* conference for which you are
registering. (Even if you are planning to attend both conferences,
please indicate the one conference that you consider to be "primary.")
COLT94 ML94
The registration fee includes a copy of the proceedings for the *one*
conference circled above (extra proceedings can be ordered below).
Also included is admission to all ML94 and COLT94 talks and events
(except that student registration does not include a banquet ticket).
Regular advance registration: $190 $_______
ACM/SIG member advance registration: $175 $_______
Late registration (after May 27): $230 $_______
Student advance registration: $85 $_______
Student late registration (after May 27): $110 $_______
Extra reception tickets (July 12): _____ x $17 = _______
Extra banquet tickets (July 13): _____ x $40 = _______
Extra COLT proceedings: _____ x $35 = _______
Extra ML proceedings: _____ x $35 = _______
Dorm housing (from below): $_______
TOTAL ENCLOSED: $_______
How many in your party have dietary restrictions?
Vegetarian: _____ Kosher: _____ Other: ______________
Circle your shirt size: small medium large X-large
HOUSING. Please indicate your housing preference below. Descriptions
of the dorms are given under "housing" above. Dorm assignments will
be made on a first come, first served basis, so please send your
request in as early as possible. We will notify you by email if we
cannot fill your request.
_____ Check here if you plan to stay at the Hyatt (reservations must
be made directly with the hotel by June 10).
_____ Check here if you plan to make your own housing arrangements
(other than at the Hyatt).
_____ Check here to request a room in the dorms and circle the
appropriate dollar amount below:
Dorm: Stonier Campbell
Length of stay: dbl. sing. dbl.
ML only (July 9-13): $144 144 108
COLT only (July 11-15): 144 144 108
ML and COLT (July 9-15): 216 216 162
If staying in a double in the dorms, who will your roommate
be? ____________________________________
For either dorm, please indicate expected day and time of arrival and
departure. Note that check-in for the dorms must take place between
4pm and midnight on July 9-13.
Expected arrival: ______ ______
(date) (time)
Expected departure: ______ ______
(date) (time)
TUTORIALS. The DIMACS-sponsored tutorials on July 10 are free and
open to the general public. For our planning purposes, please circle
those tutorials you plan to attend.
Morning: T1 T2 T3 T4
Afternoon: T1 T5 T6
To participate in a workshop, please contact the workshop organizer
directly. There is no fee for any workshop, and all workshops will be
held on July 10.
REFUNDS. The entire dorm fee, and one-half of the registration fee
are refundable through June 24. Send all requests by email to
rasmussen@cs.rutgers.edu.
------------------------------
Date: Mon, 25 Apr 1994 23:37:46 -0500 (EST)
From: "R. Uthurusamy" <SAMY@gmr.com>
Subject: ANZIIS-94 Call for Papers
To: ml@ics.uci.edu
Cc: J.Sitte@qut.edu.au
Message-id: <01HBLZ3OUE1I9C0BYA@gmr.com>
X-VMS-To: NET%"ml@ics.uci.edu"
X-VMS-Cc: SITTE,SAMY
MIME-version: 1.0
Content-transfer-encoding: 7BIT
CALL FOR PAPERS
-----------------
ANZIIS-94
=========
Second Australian and New Zealand Conference
on Intelligent Information Systems
Brisbane, Queensland, Australia
29 November - 2 December 1994
Tutorials: 29 November,
Conference: 30 November - 2 December 1994
Major fields: Artificial Intelligence
Fuzzy Systems
Neural Networks
Evolutionary Computation
The Second Australian and New Zealand Conference on Intelligent Information
Systems (ANZIIS-94) will be held in Brisbane, from 29 November to 2 December
1994. This follows the successful inaugural conference, ANZIIS-93, held in
Perth in December 1993. The Conference will offer an international forum
for discussion of new research on the key methods of intelligent information
processing: conventional artificial intelligence, fuzzy logic, artificial
neural networks, and evolutionary algorithms.
The conference will include invited keynote presentations and contributed
papers in oral and poster presentations. All papers will be refereed and
published in the proceedings.
TUTORIALS AND PANEL SESSIONS
The Organising Programme Committee cordially invites proposals for tutorials
and special interest sessions relevant to the scope of the conference.
Proposals should include details of the proponent including mailing, e-mail
and fax addresses, and research record.
ABOUT BRISBANE
Brisbane is a cosmopolitan and pleasant subtropical city. It is the heart of
the vibrant south-east Queensland region that streches over 200 Km from the
Gold to the Sunshine Coasts. It is not only a focal point for national and
international tourists but tens of thousands Australians every year decide
to set up home here. We recommed conference participants to set aside a few
extra days to explore the region, either on their own leisure or by taking
part in the special pre and post conference activities to be announced.
Application areas will include, but will not be limited to:
Adaptive Systems
Artificial Life
Autonomous Vehicles
Data Analysis
Factory Automation
Financial Markets
Intelligent Databases
Knowledge Engineering
Machine Vision
Pattern Recognition
Machine Learning
Neurobiological Systems
Control Systems
Optimisation
Parallel and Distributed Computing
Robotics
Prediction
Sensorimotor Systems
Signal Processing
Speech Processing
Virtual Reality
INFORMATION
ANZIIS-94 Secretariat
School of Computing Science
Queensland University of Technology
GPO Box 2434
Brisbane, Q 4001, Australia.
Telephone: + 61 7 864 2925
Fax: + 61 7 864 1801
e-mail: anziis94@qut.edu.au
SUBMISSION OF PAPERS
For the speedy processing of the papers authors are requested to submit their
contributions camera-ready on paper and by mail only. Papers should be laser
printed on A4 size pages with 25 mm margins on all four sides using a Roman
font not smaller than 10 points. The maximum allowed length of an article is
5 pages. The paper should be set in two column format, using the LaTex
"article" style or following the style of the IEEE Transaction journals.
The papers should contain an abstract and the complete mailing addresses
of the authors. Papers will be reviewed internationally. Accepted articles
will be published as submitted, as there is no opportunity for revision.
Only those papers for which the presenting author has registered as a
conference delegate will be printed in the proceedings. Extra copies of
the Proceedings will be marketed through the IEEE book brokerage program.
IMPORTANT DATES
Papers due: 15 July 1994
Tutorial proposals due: 15 July 1994
Notification of acceptance: 15 September 1994
Registration for authors due: 1 October 1994
FEES before 1 Oct after 1 Oct
---- ------------ -----------
Member of IEEE/IEAust/ACS A$400 A$450
Other A$450 A$500
Student member of IEEE/IEAust/ACS A$150 A$200
Other Student A$200 A$250
GOVERNMENT TRAINING LEVY
The conference programme will meet the requirements of the Australian
Government Training Levy for inclusion in an employer's training programme.
ANZIIS-94 ORGANISED BY : IEEE Australia Council
IEEE New Zealand Council
IEEE Queensland Section
IN CO-OPERATION WITH : IEAust - The Institution of Engineers, Australia
Australian Computer Society
Queensland University of Technology -
School of Computing Science
------------------------------
End of ML-LIST (Digest
format)
****************************************