Copy Link
Add to Bookmark
Report
Machine Learning List Vol. 6 No. 04
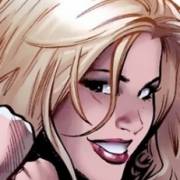
Machine Learning List: Vol. 6 No. 4
Saturday, February 12, 1994
Contents:
JAIR articles
Web Server with an Emphasis on Machine Learning Resources
MLNet workshop
Knowledge Level Models of Machine Learning
Deadline extended for ML workshop at AI94 (Banff)
ML postdoc (Australia)
PhD and Masters Programs at the Oregon Graduate Institute
The Machine Learning List is moderated. Contributions should be relevant to
the scientific study of machine learning. Mail contributions to ml@ics.uci.edu.
Mail requests to be added or deleted to ml-request@ics.uci.edu. Back issues
may be FTP'd from ics.uci.edu in pub/ml-list/V<X>/<N> or N.Z where X and N are
the volume and number of the issue; ID: anonymous PASSWORD: <your mail address>
----------------------------------------------------------------------
Date: Wed, 9 Feb 94 13:43:04 PST
From: Steve Minton <minton@ptolemy-ethernet.arc.nasa.gov>
Subject: JAIR articles
ML-LIST readers might be interested the following two ML articles,
which were recently published in the Journal of Artificial
Intelligence Research. JAIR's server can be accessed by WWW, FTP,
gopher, or automated email. For further information, check out our WWW
server (URL is gopher://p.gp.cs.cmu.edu/) or one of our FTP sites
(/usr/jair/pub at p.gp.cs.cmu.edu), or send email to jair@cs.cmu.edu
with the subject AUTORESPOND and the message body HELP.
Koppel, M., Feldman R. and Segre, A.M. (1994)
"Bias-Driven Revision of Logical Domain Theories", Volume 1, pages 159-208
Postscript: volume1/koppel94a.ps (465K)
compressed, volume1/koppel94a.ps.Z (203K)
Abstract: The theory revision problem is the problem of how best to
go about revising a deficient domain theory using information
contained in examples that expose inaccuracies. In this paper we
present our approach to the theory revision problem for propositional
domain theories. The approach described here, called PTR, uses
probabilities associated with domain theory elements to numerically
track the ``flow'' of proof through the theory. This allows us to
measure the precise role of a clause or literal in allowing or
preventing a (desired or undesired) derivation for a given example.
This information is used to efficiently locate and repair flawed
elements of the theory. PTR is proved to converge to a theory which
correctly classifies all examples, and shown experimentally to be fast
and accurate even for deep theories.
Ling, C.X. (1994)
"Learning the Past Tense of English Verbs: The Symbolic Pattern Associator
vs. Connectionist Models", Volume 1, pages 209-229
Postscript: volume1/ling94a.ps (247K)
Online Appendix: volume1/ling-appendix.Z (109K) data file, compressed
Appendix: Learning the past tense of English verbs - a seemingly minor
aspect of language acquisition - has generated heated debates since
1986, and has become a landmark task for testing the adequacy of
cognitive modeling. Several artificial neural networks (ANNs) have
been implemented, and a challenge for better symbolic models has been
posed. In this paper, we present a general-purpose Symbolic Pattern
Associator (SPA) based upon the decision-tree learning algorithm ID3.
We conduct extensive head-to-head comparisons on the generalization
ability between ANN models and the SPA under different
representations. We conclude that the SPA generalizes the past tense
of unseen verbs better than ANN models by a wide margin, and we offer
insights as to why this should be the case. We also discuss a new
default strategy for decision-tree learning algorithms.
------------------------------
Date: Thu, 10 Feb 94 12:23:30 EST
From: Peter Turney <peter@ai.iit.nrc.ca>
Subject: Web Server with an Emphasis on Machine Learning Resources
The Knowledge Systems Laboratory Announces
A New World Wide Web Server
with an Emphasis on Machine Learning Resources
The World Wide Web is a hypermedia document that spans the Internet.
If you are not familiar with the Web, you can get acquainted by
obtaining a free copy of Mosaic from the National Center for
Supercomputing Applications. For example, if you have a Sun:
unix> ftp ftp.ncsa.uiuc.edu
Name: anonymous
Password: <your e-mail address>
ftp> cd Mosaic/Mosaic-binaries
ftp> binary
ftp> get Mosaic-sun.Z
ftp> bye
unix> uncompress Mosaic-sun.Z
unix> Mosaic-sun
Like ftp (file transfer protocol), the World Wide Web is based on a
protocol for the exchange of files over the Internet. The protocol is
known as http (hypertext transfer protocol). This new protocol subsumes
older protocols, such as ftp and gopher. Mosaic uses http to
provide a hypermedia interface (text, hypertext, graphics, movies,
audio) to the Internet.
The Knowledge Systems Laboratory of the National Research Council
of Canada has set up a World Wide Web Server (analogous to an
ftp server) that delivers information relevant to AI researchers,
especially machine learning researchers. If you have Mosaic,
you may access the KSL server using the URL:
http://ai.iit.nrc.ca/home_page.html
Please let me know if you have any suggestions about information
that could be added to our server. Feedback of any kind is most
welcome.
------------------------------
Date: Thu, 10 Feb 1994 21:08:09 --100
From: Celine.Rouveirol@lri.fr
Subject:MLNet workshop
MLNET FAMILIARISATION WORKSHOP
Declarative Bias
Catania, Italy, april 9 1994
**********************************************************************
The workshop is to be organized after the European Conference on
Machine Learning (ECML 94), april 6-8 1994, in the context of the
second MLNet familiarization workshop Catania, Italy.
**********************************************************************
Call for Contributions
Control of the learning process has always been a fundamental issue in
ML because it strongly affects the complexity of the learning process
and the learning results. There has been lately a strong interest
within the Machine Learning community concerning the elicitation of
this control knowledge, referred to as Declarative Bias. It grows
with the development of real world applications that require more
adaptable learning tools and more complex representation languages.
Representing control knowledge in a declarative way allows an expert
in ML or the ML system itself to easily shift it.
Past experiences with ML applications have demonstrated that
cooperation with the user speeds up the learning process by providing
explicit control information when available. Declarative bias is
therefore a concise and powerful way for the user to explicitly
program the ML system, instead of tuning low level knowledge such as
examples and domain theory representation in order to improve learning
results.
Three types of biases may be characterised. The first class a priori
restricts the initial set of candidate definitions for the target
concept (search space), referred to as language biases, the second
class sets heuristics to improve the search for the best definition(s)
through the search space. The third class defines validation criteria
for learning.
It is obviously a difficult task for the user to find the appropriate
combination of biases to meet her/his expectations. Shifting biases
with respect to discrepancies observed between actual learning results
and expected ones is a promising research issue. Validation of
results and shift of bias may be incrementally performed, after each
learning step by submitting intermediate results to the user or at the
end of the learning process. This cyclic task will be as easy as the
relationship between biases and her/his learning goals are stated
clearly.
Authors are encouraged to submit papers describing their favorite
learning system(s) in terms of elementary learning steps and biases
belonging to each of the three above classes.
SUBMISSION REQUIREMENTS
Authors should submit a paper or an extended abstract (not less than 2
pages) fully explaining the relevance of their work to the
workshop. Persons wishing to participate but who do not wish to give a
presentation should submit an abstract (1 page) describing their
research and/or interest in the subject area and their expected
contributions to the workshop. Papers / abstracts should be sent in
five copies by March 1 to:
Celine Rouveirol
LRI Bat 490
Universite Paris-Sud
F-91405 Orsay, France
Tel : +33 (1) 69 41 64 62
Fax : +33 (1) 69 41 65 86
e-mail : celine@lri.fr
Notification of acceptance will be e-mailed or faxed by March 15
(please specify your email or fax on the submitted paper).
ORGANISATION
All attendees will receive before the workshop a list of topics and
open questions that have emerged from the accepted papers. To
stimulate discussions, presentations are strongly encouraged to refer
to these. The worshop will start with a panel presenting the key
issues that will be discussed during the sessions. Schedule will
leave time for open discussions and syntheses.
PROGRAM COMMITTEE
Rouveirol C. Univ. Paris-Sud, France
Bergadano F. Univ. Catania, Italy
Esposito F. Univ. Bari, Italy
Lavrac N. JSI, Ljubljana, Slovenia
Mozetic I. Techn. Univ. Vienna - ARIAI, Austria
Nedellec C. Univ. Paris-Sud, France
Plaza E. IIIA-CSIC, Spain
Popelinsky L. Univ. Brno, Czech Republic
Sleeman D. Univ. Aberdeen, U.K.
Van de Merckt T. Free Univ. of Brussels, Belgium
Van Someren M. Univ. Amsterdam, The Netherlands
------------------------------
Date: Fri, 11 Feb 94 16:18:12 +0100
From: Walter Van De Velde <walter@arti17.vub.ac.be>
Subject: Knowledge Level Models of Machine Learning
Knowledge Level Models of Machine Learning
This is an invitation to participate in, and submit to a workshop to
be organized in the context of the second MLNet familiarization
workshop, 9-10 april 1994, Catania, Italy. It provides the following
information: title, topic description, relevance, potential, workshop
format, organizing committee and time table.
The aim of this workshop is to discuss knowledge level modeling applied to
machine learning systems and algorithms.
An important distinction in current expert systems research is the
one be- tween knowledge level and symbol level [Newell, 1982]. Systems
can be described at either of these levels. Briefly stated, a
knowledge level description emphasizes the knowledge contents of a
system (e.g. goals, actions and knowledge used in a rational way)
whereas the symbol level describes its computational realization (in
terms of representations and inference mechanisms). There is a
consensus that modeling at the knowledge level is a useful
intermediate step in the de- velopment of an expert system [Steels and
McDermott, 1993]. So called second generation expert systems
explicitly incorporate aspects of their knowledge level structure,
resulting in potential advantages for knowledge acquisition, design,
implementation, explanation and maintenance (see [David et al., 1993]
for an overview on the state of the art). The technical goal is to
construct generic components which can be reused and refined as
needed, guided by features of the domain and the task instead of by
engineering considerations.
This workshop investigates the results on describing learning
systems at the knowledge level, hoping to gain some of the same
advantages. Although the earliest attempts to do this [Dietterich,
1986] failed to lead to useful results, later efforts provided
interesting insights [Flann and Dietterich, 1989]. Maybe a more
important reason for the exploration of the knowledge level of
learning systems is that the notion of knowledge level itself, as it
is currently used in expert systems research, is no longer equivalent
to Newell's [Van de Velde, 1993]. Currently used models are
considerably more manageable, structured and, in a sense, more
engineering oriented. Knowledge level analysis of learning systems can
directly benefit from the developments in knowledge modeling that are
currently taking place (see e.g. [Klinker, 1993] for recent
work). Moreover the knowledge level analysis of machine learning
systems can be done directly in available environments allowing for
the easy integration with problem solving or knowledge acquisition
systems.
Note that the relevance of the knowledge level ideas to machine
learning is broader than what is described here (e.g., learning of
knowledge level models). To keep the present workshop relatively
focussed It is suggested to stick closely to the main topic: knowledge
level modeling of machine learning.
The topic of this workshop is relevant for several reasons:
It provides insights into essential features, differences and
similarities of machine learning algorithms
It contributes to the flexible and problem specific configuration
of learning systems
It contributes to integrating learning into performing systems
It contributes to the bridge between ML and KA
It supports the exchange and reuse of results in machine learning.
We are looking forward to a strong interest and participation in this
workshop. Europe has a strong tradition in knowledge level modeling,
with the developments of such methodologies as KADS and Components of
Expertise, and of languages and environments for constructing
knowledge level models (KARL, MoMo, KresT, FML, and so on) and large
scale projects in this direction such as MLT and parts of KADS-II.
From the answers to the questionnaire organized by the VUB AI-Lab at
IJCAI and the previous MLNet workshop, the problems in exchange and
reuse of systems emerged as one of the key bottlenecks in current
research practice. Several papers have been seen on knowledge level
modeling of learning (e.g. at the previous familiarization workshop in
the section on integrated architectures, in the last KADS user group
meeting, in the European Workshop on Case-Based Reasoning, and so
forth). The workshop is a good opportunity to bring these results
together. The workshop works on the bridge between knowledge
acquisition and machine learning, using concepts of the KA community
to understand results in the ML community.
Workshop Format
The workshop will consist of presentations of papers and work in small
sub- groups to develop knowledge models of learning in specific
frameworks. Participants are encouraged to bring software that can be
used for the interactive construction, configuration and execution of
knowledge level models of machine learning algorithms. These
environments exist (at least KresT, the CommonKADS workbench and NOOS
can be provided) and a result of the workshop will be their
application in a real experiment of exchange, reuse and configuration
of machine learning systems and algorithms.
In addition the workshop will issue a call for knowledge level
models of machine learning systems and algorithms to be input in a
common library. As- sistance will be provided so that all the
participants in ECML or the workshops have a chance to contribute to
this effort. This aspect is depending on the avail- ability of some
computing infrastructure at the site of the conference, an issue which
will be treated in due course.
Organizing Committee
Agnar Aamodt (University of Trondheim, Norway)
Dieter Fensel (University of Karlsruhe, Germany)
Enric Plaza (IIIA, Blanes, Catalunya, Spain)
Walter Van de Velde (VUB AI-Lab, Brussels, Belgium)
Maarten Van Someren (SWI, Universtiy of Amsterdam, The Netherlands)
Timetable
March 1: submission deadline
March 15: notification of acceptance (allows for early registration fee)
March 30: copy for distribution due. Only participants that are actually reg-
istered will be included in the proceedings.
April 9-10:workshop
Please send your submission before March 1 to the address below. LaTeX
submissions by email are strongly encouraged.
Walter Van de Velde
Artificial Intelligence Laboratory Tel: +32 2 641 37 00
Vrije Universiteit Brussel Fax: +32 2 641 37 29
Pleinlaan 2, B-1050 Brussels Email: walter@arti.vub.ac.be
References
[David et al., 1993]David, J.-M., Krivine, J.-P., and Simmons, R. (Eds.).
(1993). Second Generation Expert Systems. Springer Verlag, Berlin.
[Dietterich, 1986]Dietterich, T. G. (1986). Learning at the knowledge level.
Machine Learning, 1, 287{316.
[Flann and Dietterich, 1989]Flann, N. and Dietterich, T. (1989). A study of
explanation-based methods for inductive learning. Machine Learning, 4(2),
187{226.
[Klinker, 1993]Klinker, G. (Ed.). (1993). Special Issue: Current issues in
knowledge modeling, volume 5 of Knowledge Acquisition. Academic Press.
[Newell, 1982]Newell, A. (1982). The knowledge level. Artificial Intelligence,
18, 87{127.
[Steels and McDermott, 1993]Steels, L. and McDermott, J. (Eds.). (1993). The
Knowledge Level in Expert Systems. Conversations and Commentary. Aca-
demic Press, Boston, MA.
[Van de Velde, 1993]Van de Velde, W. (1993). Issues in knowledge level mod-
eling. In J.-M. David, J.-P. K. and Simmons, R. (Eds.). , Second Generation
Expert Systems. Springer Verlag, Berlin.
------------------------------
From: Bruce Macdonald <bruce@cpsc.ucalgary.ca>
Date: Fri, 11 Feb 1994 11:11:55 -0700
Subject: Deadline extended for ML workshop at AI94 (Banff)
Final CFP: Submissions due Feb 24
Machine Learning Workshop at AI'94
Canadian Artificial Intelligence Conference
Banff Park Lodge
Banff, Alberta, Canada
May 16-20, 1994
Workshop date: May 17
The purpose of this workshop is to bring together people who are
active in applications or (theoretical or experimental) studies of
machine learning. Participants in related fields such as computational
studies of human learning, genetic algorithms and neural nets are also
welcome. The emphasis of the workshop will be on informal
communication and exchange of ideas. A small fee will be charged to
cover costs.
The key speaker will be Ming Li from the University of Waterloo, and
the general theme of the address will be "combining machine learning
theory and practice."
SUBMISSIONS: Please submit an extended abstract (of not more than 4000
words) and a short (not more than 1000 word) summary of current work
by email (text or Postscript) to bruce@cpsc.ucalgary.ca. A paper will
be required for accepted contributions, and these will be collected
together in a workshop proceedings as a technical report.
ORGANIZING COMMITTEE:
Chairman: Bruce MacDonald Rob Holte
Computer Science Department Department of Computer Science
The University of Calgary University of Ottawa
2500 University Drive NW Ottawa, Ont., K1N 6N5
Calgary, Alta., T2N 1N4 email: holte@csi.uottawa.ca
ph.(403) 220-5112
Fax: (403) 284-4707 Charles Ling
email: bruce@cpsc.ucalgary.ca Department of Computer Science
University of Western Ontario
London, Ont., N6A 5B7
email: ling@csd.uwo.ca
SPONSORSHIP: Alberta Research Council
SUBMISSIONS DUE: EXTENDED TO FEB 24.
PROCEEDINGS: distributed at the workshop (May 17)
(papers submitted by email will be
available electronically, ahead of time)
------------------------------
Date: Tue, 1 Feb 94 19:48:41 +1100
From: Kevin Korb <korb@bruce.cs.monash.edu.au>
Subject: ML postdoc (Australia)
Postdoctoral Fellowship
in Artificial Intelligence and Statistics
Department of Computer Science
Monash University
Clayton, Victoria 3168
Australia
PROJECT: A Bayesian Learning Agent
A research associate at the lecturer level is sought to help develop
computational models of inductive learning agents. The applicant should
have a Ph.D. completed or near completion in Computer Science, Statistics,
Cognitive Science or a closely allied field and be familiar with at least
one of the following: quantitative machine learning techniques (e.g.,
decision trees, evolutionary programming, neural networks) or Bayesian
networks; statistical inference and the foundations of statistics (Bayesian
methods, factor analysis, causal analysis, statistical computing); AI
programming techniques using Common Lisp, CLOS, C, C++. A familiarity with
the philosophy of science, cognitive psychology, or cognitive science
generally would be a plus. Applicants must already have permanent
residency in Australia.
TERM: The initial appointment will be for one year, commencing as soon as
possible. This project is funded by the ARC and is expected to be continued
for a total of three years.
SALARY RANGE: Research Associate ($A 36,285 - $A 38,950).
APPLICATIONS: Written applications should quote the above project title and
include a full curriculum vitae (including copies of official transcripts).
Applications should also include the names and addresses (and email
address, fax and telephone numbers if available) of at least two referees.
Applications should be sent to the address below by 4 March, 1994.
Prof. Chris Wallace
Department of Computer Science
Monash University
Clayton, Victoria 3168
Australia
csw@bruce.cs.monash.edu.au
+61 3 905 5197 (tel)
+61 3 905 5146 (fax)
------------------------------
Date: Fri, 4 Feb 94 16:00:07 -0800
From: John Moody <moody@chianti.cse.ogi.edu>
Subject: PhD and Masters Programs at the Oregon Graduate Institute
The Oregon Graduate Institute of Science and Technology (OGI) has
openings for a few outstanding students in its Computer Science
and Electrical Engineering Masters and Ph.D programs in the areas
of Neural Networks, Learning, Signal Processing, Time Series,
Control, Speech, Language, and Vision.
Faculty and postdocs in these areas include Etienne Barnard, Ron
Cole, Mark Fanty, Dan Hammerstrom, Hynek Hermansky, Todd Leen, Uzi
Levin, John Moody, David Novick, Misha Pavel, Joachim Utans, Eric
Wan, and Lizhong Wu. Short descriptions of our research interests
are appended below.
OGI is a young, but rapidly growing, private research institute
located in the Portland area. OGI offers Masters and PhD programs
in Computer Science and Engineering, Applied Physics, Electrical
Engineering, Biology, Chemistry, Materials Science and Engineering,
and Environmental Science and Engineering.
Inquiries about the Masters and PhD programs and admissions for
either Computer Science or Electrical Engineering should be addressed
to:
Margaret Day, Director
Office of Admissions and Records
Oregon Graduate Institute
PO Box 91000
Portland, OR 97291
Phone: (503)690-1028
Email: margday@admin.ogi.edu
The final deadline for receipt of all applications materials for
the Ph.D. programs is March 1, 1994, so it's not too late to apply!
Masters program applications are accepted continuously.
+++++++++++++++++++++++++++++++++++++++++++++++++++++++
Oregon Graduate Institute of Science & Technology
Department of Computer Science and Engineering
& Department of Electrical Engineering and Applied Physics
Research Interests of Faculty in Adaptive & Interactive Systems
(Neural Networks, Signal Processing, Control, Speech, Language, and Vision)
Etienne Barnard (Assistant Professor):
Etienne Barnard is interested in the theory, design and implementation
of pattern-recognition systems, classifiers, and neural networks.
He is also interested in adaptive control systems -- specifically,
the design of near-optimal controllers for real- world problems
such as robotics.
Ron Cole (Professor):
Ron Cole is director of the Center for Spoken Language Understanding
at OGI. Research in the Center currently focuses on speaker-
independent recognition of continuous speech over the telephone
and automatic language identification for English and ten other
languages. The approach combines knowledge of hearing, speech
perception, acoustic phonetics, prosody and linguistics with neural
networks to produce systems that work in the real world.
Mark Fanty (Research Assistant Professor):
Mark Fanty's research interests include continuous speech recognition
for the telephone; natural language and dialog for spoken language
systems; neural networks for speech recognition; and voice control
of computers.
Dan Hammerstrom (Associate Professor):
Based on research performed at the Institute, Dan Hammerstrom and
several of his students have spun out a company, Adaptive Solutions
Inc., which is creating massively parallel computer hardware for
the acceleration of neural network and pattern recognition
applications. There are close ties between OGI and Adaptive
Solutions. Dan is still on the faculty of the Oregon Graduate
Institute and continues to study next generation VLSI neurocomputer
architectures.
Hynek Hermansky (Associate Professor);
Hynek Hermansky is interested in speech processing by humans and
machines with engineering applications in speech and speaker
recognition, speech coding, enhancement, and synthesis. His main
research interest is in practical engineering models of human
information processing.
Todd K. Leen (Associate Professor):
Todd Leen's research spans theory of neural network models,
architecture and algorithm design and applications to speech
recognition. His theoretical work is currently focused on the
foundations of stochastic learning, while his work on Algorithm
design is focused on fast algorithms for non-linear data modeling.
Uzi Levin (Senior Research Scientist):
Uzi Levin's research interests include neural networks, learning
systems, decision dynamics in distributed and hierarchical
environments, dynamical systems, Markov decision processes, and
the application of neural networks to the analysis of financial
markets.
John Moody (Associate Professor):
John Moody does research on the design and analysis of learning
algorithms, statistical learning theory (including generalization
and model selection), optimization methods (both deterministic and
stochastic), and applications to signal processing, time series,
and finance.
David Novick (Assistant Professor):
David Novick conducts research in interactive systems, including
computational models of conversation, technologically mediated
communication, and human-computer interaction. A central theme of
this research is the role of meta-acts in the control of interaction.
Current projects include dialogue models for telephone-based
information systems.
Misha Pavel (Associate Professor):
Misha Pavel does mathematical and neural modeling of adaptive
behaviors including visual processing, pattern recognition, visually
guided motor control, categorization, and decision making. He is
also interested in the application of these models to sensor
fusion, visually guided vehicular control, and human-computer
interfaces.
Joachim Utans (Post-Doctoral Research Associate):
Joachim Utans's research interests include computer vision and
image processing, model based object recognition, neural network
learning algorithms and optimization methods, model selection and
generalization, with applications in handwritten character recognition
and financial analysis.
Lizhong Wu (Post-Doctoral Research Associate):
Lizhong Wu's research interests include neural network theory and
modeling, time series analysis and prediction, pattern classification
and recognition, signal processing, vector quantization, source
coding and data compression. He is now working on the application
of neural networks and nonparametric statistical paradigms to
finance.
Eric A. Wan (Assistant Professor):
Eric Wan's research interests include learning algorithms and
architectures for neural networks and adaptive signal processing.
He is particularly interested in neural applications to time series
prediction, adaptive control, active noise cancellation, and
telecommunications.
------------------------------
End of ML-LIST (Digest format)
****************************************