Copy Link
Add to Bookmark
Report
Machine Learning List Vol. 4 No. 24
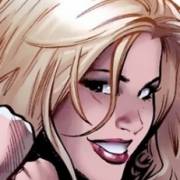
Machine Learning List: Vol. 4 No. 24
Sunday, Dec 20, 1992
Contents:
IJCAI-93 Workshop on Inductive Logic Programming
CONNECTIONIST MODELS SUMMER SCHOOL
Senior Cognitive Science Faculty Position
call for papers "AI and Genome"
Call For Papers: ACM TIS Special Issue on Text Categorization
Morgan Kaufmann book announcement
The Machine Learning List is moderated. Contributions should be relevant to
the scientific study of machine learning. Mail contributions to ml@ics.uci.edu.
Mail requests to be added or deleted to ml-request@ics.uci.edu. Back issues
may be FTP'd from ics.uci.edu in pub/ml-list/V<X>/<N> or N.Z where X and N are
the volume and number of the issue; ID: anonymous PASSWORD: <your mail address>
Administrative Note: I'm leaving on a sabbatical until April 1. I will
still moderate ML-LIST, but you can help by:
1. Sending submissions to ml@ics.uci.edu (rather than pazzani@ics.uci.edu)
2. Send submissions that look like other items in ML-LIST
a. Use a descriptive subject (Not "Please post")
b. Send text, not Tex or troff
c. Don't use dashes "---" to separate parts of your message
Happy Holidays- Mike
----------------------------------------------------------------------
Date: Fri, 18 Dec 92 13:33:04 +0100
From: " F. Bergadano" <bergadan@di.unito.it>
Subject: IJCAI-93 Workshop on Inductive Logic Programming
IJCAI-93 Workshop on Inductive Logic Programming
Call for Papers
Inductive Logic Programming is mainly concerned with the inductive
synthesis of logic programs. As such, it is closely related to Machine
Learning and Logic Programming, and has evolved from these areas to a
growing field of research for both Artificial Intelligence and
Software Engineering. For Machine Learning, the problem is a natural
extension of previous methods of inductive generalization to the case
of logic-based and recursive concept descriptions. For Logic
Programming, inductive methods provide the user with a tool for
programming not only with clauses, but also with examples and general
constraints. The workshop intends to address both aspects of ILP, and
provide a common ground for discussion and for the presentation of
algorithms and results. Attendance will be limited to 50
participants, on the basis of submitted papers and participation
requests sent to any of the program chairs. Participants will be
required to have registered at IJCAI.
Program Co-chairs:
Francesco Bergadano Luc De Raedt
Dipartimento di Matematica Departement Computerwetenschappen
Universita` di Catania Kathol. University of Leuven
Via Andrea Doria 6/a Celestijnenlaan 200a
Catania, Italy B-3001 Leuven, Belgium
tel (+39) 95 330533 tel (+32) 16200656
fax (+39) 95 330094 fax (+32) 16205308
bergadan@mathct.cineca.it lucdr@cs.kuleuven.ac.be
Stan Matwin Stephen Muggleton
Department of Computer Science Oxford University Computing Lab
University of Ottawa 11 Keble Road
Ottawa, Ontario KIN9B4, Oxford, OX1 3QD, UK
CANADA tel (+44) 865 272562
tel (+1) 613 5645069 fax (+44) 865 272582
stan@csi.uottawa.ca steve@prg.oxford.ac.uk
Papers (a maximum of 10 double spaced pages)
should be submitted in 2 copies to any of the above
program co-chairs, with the following deadlines:
submitted paper must be received before: March 25th, 1993
notification of acceptance/rejection: May 5th, 1993
final camera-ready paper: June 10th, 1993
workshop: 28th of August, in Chambery just before IJCAI-93
------------------------------
Date: Mon, 7 Dec 1992 22:22:05 -0700
From: "Michael C. Mozer" <mozer@dendrite.cs.colorado.EDU>
Subject: CONNECTIONIST MODELS SUMMER SCHOOL
CALL FOR APPLICATIONS
CONNECTIONIST MODELS SUMMER SCHOOL
University of Colorado
Boulder, Colorado
June 21 - July 3, 1993
The University of Colorado will host the 1993 Connectionist
Models Summer School from June 21 to July 3, 1993. The purpose
of the summer school is to provide training to promising young
researchers in connectionism (neural networks) by leaders of the
field and to foster interdisciplinary collaboration. This will
be the fourth such program in a series that was held at
Carnegie-Mellon in 1986 and 1988 and at UC San Diego in 1990.
Previous summer schools have been extremely successful and we
look forward to the 1993 session with anticipation of another
exciting event.
The summer school will offer courses in many areas of
connectionist modeling, with emphasis on artificial intelligence,
cognitive neuroscience, cognitive science, computational methods,
and theoretical foundations. Visiting faculty (see list of
invited faculty below) will present daily lectures and tutorials,
coordinate informal workshops, and lead small discussion groups.
The summer school schedule is designed to allow for significant
interaction among students and faculty. As in previous years, a
proceedings of the summer school will be published.
Applications will be considered only from graduate students
currently enrolled in Ph.D. programs. About 50 students will be
accepted. Admission is on a competitive basis. Tuition will be
covered for all students, and we expect to have scholarships
available to subsidize housing and meal costs, which will run
approximately $300.
Applications should include the following materials:
* a one-page statement of purpose, explaining major areas of
interest and prior background in connectionist modeling and
neural networks;
* a vita, including academic history, list of publications (if
any), and relevant courses taken with instructors' names and
grades received;
* two letters of recommendation from individuals familiar with
the applicants' work; and
* if room and board support is requested, a statement from the
applicant describing potential sources of financial support
available (department, advisor, etc.) and the estimated extent of
need. We hope to have sufficient scholarship funds available to
provide room and board to all accepted students regardless of
financial need.
Applications should be sent to:
Connectionist Models Summer School
c/o Institute of Cognitive Science
Campus Box 344
University of Colorado
Boulder, CO 80309
All application materials must be received by March 1, 1993.
Decisions about acceptance and scholarship awards will be
announced April 15. If you have additional questions, please
write to the address above or send e-mail to
"cmss@cs.colorado.edu".
Organizing Committee
Jeff Elman (UC San Diego)
Mike Mozer (University of Colorado)
Paul Smolensky (University of Colorado)
Dave Touretzky (Carnegie-Mellon)
Andreas Weigend (Xerox PARC and University of Colorado)
Additional faculty will include:
Andy Barto (University of Massachusetts, Amherst)
Jack Cowan (University of Chicago)
David Haussler (UC Santa Cruz)
Geoff Hinton (University of Toronto)
Mike Jordan (MIT)
John Kruschke (Indiana University)
Jay McClelland (Carnegie-Mellon)
Ennio Mingolla (Boston University)
Steve Nowlan (Salk Institute)
Dave Plaut (Carnegie-Mellon)
Jordan Pollack (Ohio State)
Dave Rumelhart (Stanford)
Terry Sejnowski (UC San Diego and Salk Institute)
The Summer School is sponsored in part by the American Association for
Artificial Intelligence, the International Neural Network Society, and
Siemens Research Center.
------------------------------
Date: Thu, 10 Dec 92 13:34:54 EST
From: Tony Simon <tonys@zunow.gatech.EDU>
Subject: Senior Cognitive Science Faculty Position
SENIOR FACULTY POSITION IN COGNITIVE PSYCHOLOGY
GEORGIA INSTITUTE OF TECHNOLOGY
COGNITIVE SCIENCE - The School of Psychology at the Georgia Institute
of Technology is searching for a senior faculty member in Cognitive
Psychology to be part of a major interdisciplinary thrust in Cognitive
Science. Cognitive Science at Georgia Tech includes basic and applied
research in focus areas including: (1) learning; (2) problem solving;
(3) language and communication; and (4) design. Candidates for this
position should add strength and intellectual leadership to one or
more of these areas. Assuming resources are available, the appointment
could begin in the fall of 1993. Qualifications should include
evidence of outstanding research achievement and a commitment to
working with Cognitive Scientists from other disciplines.
Responsibilities will include intellectual leadership, maintenance of
strong programmatic research, supervision of graduate student
research, and classroom instruction.
To apply, send vitae and names of references to:
Cognitive Psychology Search Committee
School of Psychology
Georgia Institute of Technology
Atlanta, GA 30332-0170
Georgia Tech is an Equal Opportunity/Affirmative Action Employer and a
member institution of the University System of Georgia.
------------------------------
Date: Thu, 17 Dec 92 19:24:58 +0100
From: "irina Tchoumatchenko 46.42.32.00 poste 433" <irina@laforia.ibp.fr>
Subject: call for papers "AI and Genome"
WORKSHOP "ARTIFICIAL INTELLIGENCE and the GENOME"
at the International Joint Conference on Artificial Intelligence
IJCAI-93
August 29 - September 3, 1993
Chambery, FRANCE
There is a great deal of intellectual excitement in molecular biology
(MB) right now. There has been an explosion of new knowledge due to
the advent of the Human Genome Program. Traditional methods of
computational molecular biology can hardly cope with important
complexity issues without adapting a heuristic approach. They enable
one to explicitate molecular biology knowledge to solve a problem as
well as to present the obtained solution in biologically-meaningful
terms. The computational size of many important biological problems
overwhelms even the fastest hardware by many orders of magnitude. The
approximate and heuristic methods of Artificial Intelligence have
already made significant progress in these difficult problems. Perhaps
one reason is great deal of biological knowledge is symbolic and
complex in their organization. Another reason is the good match
between biology and machine learning. Increasing amout of biological
data and a significant lack of theoretical understanding suggest the
use of generalization techniques to discover "similarities" in data
and to develop some pieces of theory. On the other hand, molecular
biology is a challenging real-world domain for artificial intelligence
research, being neither trivial nor equivalent to solving the general
problem of intelligence. This workshop is dedicated to support the
young AI/MB field of research.
TOPICS OF INTEREST INCLUDE (BUT ARE NOT RESTRICTED TO):
*******************************************************
*** Knowledge-based approaches to molecular biology problem solving;
Molecular biology knowledge-representation issues, knowledge-based heuristics
to guide molecular biology data processing, explanation of MB data
processing results in terms of relevant MB knowledge;
*** Data/Knowledge bases for molecular biology;
Acquisition of molecular biology knowledge, building public genomic knowledge
bases, a concept of "different view points" in the MB data processing context;
*** Generalization techniques applied to molecular biology problem solving;
Machine learning techniques as well as neural network techniques, supervised
learning versus non-supervised learning, scaling properties of different
generalization techniques applied to MB problems;
*** Biological sequence analysis;
AI-based methods for sequence alignment, motif finding, etc.,
knowledge-guided alignment, comparison of AI-based methods for
sequence analysis with the methods of computational biology;
*** Prediction of DNA protein coding regions and regulatory sites
using AI-methods;
Machine learning techniques, neural networks, grammar-based approaches, etc.;
*** Predicting protein folding using AI-methods;
Predicting secondary, super-secondary, tertiary protein structure,
construction protein folding prediction theories by examples;
*** Predicting gene/protein functions using AI-methods;
Complexity of the function prediction problem, understanding the
structure/function relationship in biologically-meaningful examples,
structure/functions patterns, attempts toward description of functional space;
*** Similarity and homology;
Similarity measures for gene/protein class construction, knowledge-based
similarity measures, similarity versus homology, inferring evolutionary trees;
*** Other perspective approaches to classify and predict properties of
MB sequences;
Information-theoretic approach, standard non-parametric statistical
analysis, Hidden Markov models and statistical physics methods;
INVITED TALKS:
**************
L. Hunter, NLM, AI problems in finding genetic sequence motifs
J. Shavlik, U. of Wisconsin, Learning important relations in
protein structures
B. Buchanan, U. of Pittsburgh, to be determined
R. Lathrop, MIT, to be determined
Y. Kodratoff, U. Paris-Sud, to be determined
J.-G. Ganascia, U. Paris-VI, Application of machine learning
techniques to the biological investigation viewed as a constructive
process
SCHEDULE
**********
Papers received: March 1, 1993
Acceptance notification: April 1, 1993
Final papers: June 1, 1993
WORKSHOP FORMAT:
******************
The format of the workshop will be paper sessions with discussion
at the end of each session, and a concluding panel.
Prospective particitants should submit papers of five to ten pages in length.
Four paper copies are required. Those who would like to attend without a
presentation should send a one to two-page description of their relevant
research interests.
Attendance at the workshop will be limited to 30 or 40 people.
Each workshop attendee MUST HAVE REGISTERED FOR THE MAIN CONFERENCE.
An additional (low) 300 FF fee for the workshop attendance (about $60)
will be required. One student attending the workshop normally
(has registered for the main conference) and being in charge of taking notes during
the entirre workshop, could be exempted from the additional 300 FF fee.
Volunteers are invited.
ORGANIZING COMMITTEE
********************
Buchanan, B. (Univ. of Pittsburgh - USA)
Ganascia, J.-G., chairperson (Univ. of Paris-VI - France)
Hunter, L. (National Labrary of Medicine - USA)
Lathrop, R. (MIT - USA)
Kodratoff, Y. (Univ. of Paris-Sud - France)
Shavlik, J. W. (Univ. of Wisconsin - USA)
PLEASE, SEND SUBMISSIONS TO:
***************************
Ganascia, J.-G.
LAFORIA-CNRS
University Paris-VI
4 Place Jussieu
75252 PARIS Cedex 05
France
Phone: (33-1)-44-27-47-23
Fax: (33-1)-44-27-70-00
E-mail: ganascia@laforia.ibp.fr
------------------------------
Date: Sun, 13 Dec 92 16:55 EST
From: David Lewis <lewis@research.att.COM>
Subject: Call For Papers: ACM TIS Special Issue on Text Categorization
This CFP may be of interest to readers. Learning techniques have been
widely used in producing text categorization systems. A range of data
sets and tasks with interesting properties are available: small to
large numbers of examples, small to large numbers of categories
(disjoint or overlapping), high dimensionality feature sets, available
knowledge bases, time-varying data, willing human judges (with varying
degrees of consistency), real applications, etc.
Dave
****************************************************************************
Call For Papers
Special Issue on Text Categorization
ACM Transactions on Information Systems
Submissions due: June 1, 1993
Text categorization is the classification of units of natural
language text with respect to a set of pre-existing categories.
Reducing an infinite set of possible natural language inputs to a
small set of categories is a central strategy in computational systems
that process natural language. Some uses of text categorization have
been:
--To assign subject categories to documents in support of text
retrieval and library organization, or to aid the human assignment of
such categories.
--To route messages, news stories, or other continuous streams
of texts to interested recipients.
--As a component in natural language processing systems, to
filter out nonrelevant texts and parts of texts, to route texts to
category-specific processing mechanisms, or to extract limited forms
of information.
--As an aid in lexical analysis tasks, such as word sense
disambiguation.
--To categorize nontextual entities by textual annotations, for
instance to assign people to occupational categories based on free
text responses to survey questions.
ACM Transactions on Information Systems is the leading forum for
presenting research on text processing systems. For this special
issue we encourage the submission of high quality technical
descriptions of algorithms and methods for text categorization.
Experiments comparing alternative methods are especially welcome, as
are results on deploying systems into regular use.
Five copies of each manuscript should be submitted to either of the
special issue editors at the addresses below:
David D. Lewis Philip J. Hayes
AT&T Bell Laboratories Carnegie Group, Inc.
600 Mountain Ave. Five PPG Place
Room 2C409 Pittsburgh, PA 15222
Murray Hill, NJ 07974 USA
USA hayes@cgi.com
lewis@research.att.com
Submission June 1, 1993
Notification October 1, 1993
Revision February 1, 1994
Publication mid-1994
The July 1990 issue of TIS contains a description of the style requirements.
****************************************************************************
David D. Lewis email: lewis@research.att.com
AT&T Bell Laboratories ph. 908-582-3976
600 Mountain Ave.; Room 2C409
Murray Hill, NJ 07974; USA
------------------------------
Date: Wed, 9 Dec 92 10:50:17 PST
From: Morgan Kaufmann <morgan@unix.sri.COM>
Subject: Morgan Kaufmann book announcement
MORGAN KAUFMANN ANNOUNCES THE PUBLICATION OF:
READINGS IN KNOWLEDGE ACQUISITION AND LEARNING:
Automating the Construction and Improvement of Expert Systems
Edited by
Bruce G. Buchanan (University of Pittsburgh) and
David C. Wilkins (University of Illinois)
ISBN 1-55860-163-5 912 pages $44.95 U.S. $49.95 International
READINGS IN KNOWLEDGE ACQUISITION AND LEARNING collects the best of
the artificial intelligence literature from the fields of machine
learning and knowledge acquisition. This book brings together for
the first time the perspectives on constructing knowledge-based
systems from these two historically separate subfields of
artificial intelligence. A key criterion for article inclusion is
an empirical demonstration that the method described in the paper
successfully automates some important aspect of creating and
maintaining a knowledge-based system. In addition to the papers,
the editors provide an introduction to the field and to each group
of papers, discussing their significance and pointing to related
work.
This book can serve as a text for courses and seminars in
artificial intelligence, expert systems, knowledge acquisition,
knowledge engineering, and machine learning. It will also provide
practical ideas for professionals engaged in the building and
maintenance of knowledge-based systems.
Table of Contents
Chapter 1 Overview of Knowledge Acquisition and Learning 1
1.1 Overviews
1.1.1 R. S. Michalski 7
Toward a unified theory of learning:
Multistrategy task-adaptive learning
1.1.2 J. H. Boose 39
A survey of knowledge acquisition techniques and tools
Chapter 2 Expertise and Expert Systems 57
2.1 Expertise and Its Acquisition 59
2.1.1 J. R. Anderson 61
Development of expertise
2.1.2 M. L. G. Shaw and J. B. Woodward 78
Modeling expert knowledge
2.1.3 B. J. Wielinga, A. Th. Schreiber, & J. A. Breuker 92
KADS: A modelling approach to knowledge engineering
2.1.4 D. E. Forsythe and B. G. Buchanan 117
Knowledge acquisition for expert systems:
Some pitfalls and suggestions
2.2 Expert Systems and Generic Problem Classes 125
2.2.1 B. G. Buchanan and R. G. Smith 128
Fundamentals of expert systems
2.2.2 J. McDermott 150
Preliminary steps toward a taxonomy of
problem-solving methods
2.2.3 B. Chandrasekaran 171
Generic tasks in knowledge-based reasoning:
High-level building blocks for expert system design
2.2.4 W. J. Clancey 176
Acquiring, representing, and evaluating a competence
model of diagnostic strategy
Chapter 3 Interactive Elicitation Tools 217
3.1 Eliciting Classification Knowledge 219
3.1.1 R. Davis 221
Interactive transfer of expertise:
Acquisition of new inference rules
3.1.2 J. H. Boose and J. M. Bradshaw 240
Expertise transfer and complex problems:
Using AQUINAS as a knowledge-acquisition workbench for
knowledge-based systems
3.1.3 L. Eshelman, D. Ehret, J. McDermott, and M. Tan 253
MOLE: A tenacious knowledge-acquisition tool
3.2 Eliciting Design Knowledge 261
3.2.1. S. Marcus and J. McDermott 263
SALT: A knowledge acquisition language for
propose-and-revise systems
3.2.2 M. A. Musen 282
Automated support for building and extending
expert models
3.2.3 T. R. Gruber 297
Automated knowledge aquisition for strategic
knowledge
Chapter 4 Inductive Generalization Methods 319
4.1 Learning Classification Knowledge 321
4.1.1 R. S. Michalski 323
A theory and methodology of inductive learning
4.1.2 J. R. Quinlan 349
Induction of decision trees
4.1.3 G. E. Hinton 362
Connectionist learning procedures
4.1.4. A. Ginsberg, S. M. Weiss, and P. Politakis 387
Automatic knowledge base refinement for
classification systems
4.2 Learning Classes Via Clustering 403
4.2.1 J. H. Gennari, P. Langley, and D. Fisher 405
Models of incremental concept formation
4.2.2 P. Cheeseman, J. Kelly, M. Self, J. Stutz, W. Taylor,
and D. Freeman 431
Autoclass: A Bayesian classification system
4.3 Measurement and Evaluation of Learning Systems 443
4.3.1 J. W. Shavlik, R. Mooney, and G. G. Towell 445
Symbolic and neural learning algorithms:
An experimental comparison
4.3.2 B. R. Gaines 462
The quantification of knowledge:
Formal foundations for knowledge acquisition
methodologies
4.3.3 T. G. Dietterich 475
Limitations on inductive learning
Chapter 5 Compilation and Deep Models 481
5.1 Compilation of Knowledge for Efficiency 483
5.1.1 R. E. Fikes, P. E. Hart, and N. J. Nilsson 485
Learning and executing generalized robot plans
5.1.2 T. M. Mitchell, P. E. Utgoff, and R. Banerji 504
Learning by experimentation:
Acquiring and refining problem-solving heuristics
5.1.3 J. E. Laird, P. S. Rosenbloom, and A. Newell 518
Chunking in SOAR:
The anatomy of a general learning mechanism
5.2 Explanation-Based Learning of
Classification Knowledge 537
5.2.1 T. M. Mitchell, R. M. Keller, S. T. Kedar-Cabelli 539
Explanation-based generalization: A unifying view
5.2.2 R. J. Mooney 556
Explanation generalization in EGGS
5.3 Synthesizing Problem Solvers from Deep Models 577
5.3.1 W. R. Swartout 579
XPLAIN: A system for creating and explaining expert
consulting programs
5.3.2 D. R. Barstow 600
Domain-specific automatic programming
5.3.3 I. Bratko 616
Qualitative modelling and learning in KARDIO
Chapter 6 Apprenticeship Learning Systems 627
6.1 Apprentice Systems for Classification Knowledge 629
6.1.1 D. C. Wilkins 631
Knowledge base refinement as improving an incomplete
and incorrect domain theory
6.2 Apprentice Systems for Design Knowledge 643
6.2.1 T. M. Mitchell, S. Mabadevan, L. I. Steinberg 645
LEAP: A learning apprentice for VLSI design
6.2.2 Y. Kodratoff and G. Tecuci 655
Techniques of design and DISCIPLE learning apprentice
Chapter 7 Analogical and Case-Based Reasoning 669
7.1 Analogical Reasoning 671
7.1.1 D. Gentner 673
The mechanisms of analogical learning
7.1.2 B. Falkenhainer, K. D. Forbus, and D. Gentner 695
The structure-mapping engine: Algorithm and examples
7.1.3 J. G. Carbonell 727
Derivational analogy: A theory of reconstructive
problem solving and expertise acquisition
7.2 Case-Based Reasoning 739
7.2.1 B. W. Porter, R. Bareiss, and R. C. Holte 741
Concept learning and heuristic classification
in weak-theory domains
7.2.2 A. R. Golding and P. S. Rosenbloom 759
Improving rule-based systems through case-based
reasoning
7.2.3 K. J. Hammond 765
Explaining and repairing plans that fail
Chapter 8 Discovery and Commonsense Reasoning 793
8.1 Discovery Learning 795
8.1.1 D. B. Lenat 797
The ubiquity of discovery
8.1.2 L. B. Booker, D. E. Goldberg, and J. H. Holland 812
Classifier systems and genetic algorithms
8.2 Commonsense Knowledge 837
8.2.2 R. Guha and D. B. Lenat 839
CYC: A midterm report
References 867
OTHER TITLES OF INTEREST FROM MORGAN KAUFMANN:
C4.5: Programs for Machine Learning, by J. Ross Quinlan (University
of Sydney)
Case-Based Reasoning, by Janet Kolodner (Georgia Institute of
Technology)
Computer Systems That Learn: Classification and Prediction Methods
from Statistics, Neural Nets, Machine Learning and Expert Systems,
by Sholom M. Weiss and Casimir A. Kulikowski (Rutgers University)
Readings in Machine Learning, edited by Jude W. Shavlik (University
of Wisconsin) and Thomas G. Dietterich (Oregon State University)
Ordering Information:
Shipping: In the U.S. and Canada, please add $3.50 for the
first book and $2.50 for each additional for surface shipping;
for surface shipments to all other areas, please add $6.50 for
the first book and $3.50 for each additional book. Air
shipment available outside North America for $35.00 on the
first book, and $25.00 on each additional book.
American Express, Master Card, Visa and personal checks drawn
on U.S. banks accepted.
MORGAN KAUFMANN PUBLISHERS, INC.
Department E17
2929 Campus Drive, Suite 260
San Mateo, CA 94403
USA
Phone: (800) 745-7323 (in North America)
(415) 578-9911
Fax: (415) 578-0672
email: morgan@unix.sri.com
------------------------------
End of ML-LIST (Digest format)
****************************************