Copy Link
Add to Bookmark
Report
Machine Learning List Vol. 4 No. 07
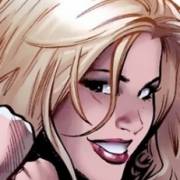
Machine Learning List: Vol. 4 No. 7
Friday, March 20, 1992
Contents:
Workshop on Machine Discovery
Banff Knowledge Acquisition Workshop
Applications of Machine Learning
The Machine Learning List is moderated. Contributions should be relevant to
the scientific study of machine learning. Mail contributions to ml@ics.uci.edu.
Mail requests to be added or deleted to ml-request@ics.uci.edu. Back issues
may be FTP'd from ics.uci.edu in pub/ml-list/V<X>/<N> or N.Z where X and N are
the volume and number of the issue; ID: anonymous PASSWORD: <your mail address>
------------------------------
Date: Wed, 18 Mar 92 13:00:33 -0600
From: jan zytkow <zytkow@wise.cs.twsu.EDU>
Subject: Workshop on Machine Discovery
A reminder:
CALL FOR PAPERS
Workshop on Machine Discovery
To be held after ML-92
Saturday, July 4, 1992 Aberdeen, Scotland
The program of the workshop will consist of paper presentations, panel
discussion, and demonstration of machine discovery systems. All papers
accepted for the workshop will be available in the workshop proceedings.
Some of those will be presented during the poster session at the
workshop.
Send 4 hard copies of your paper (The entire paper must not exceed 10
single spaced pages, including abstract of 180-220 words) or a 1-page
description of your current research in machine discovery (if you do
not wish to present a paper).
Submissions in the category of demos: 3 page description of the system
plus a sample run of the system (up to 3 pages; commented), plus answers
to the questionnaire, mailed on request.
Send all submissions to:
Jan Zytkow,
Computer Science Department,
Wichita State University,
Wichita, KS 67208
For additional information contact workshop chair:
email: zytkow@wise.cs.twsu.edu
phone: 316-689-3925
------------------------------
Date: Fri, 13 Mar 92 16:00:20 PST
From: "John Boose" <john@atc.boeing.COM>
Subject: Banff Knowledge Acquisition Workshop
7th BANFF Knowledge Acquisition for
Knowledge-Based Systems Workshop
Banff, Canada, October 11-16, 1991
In cooperation with the University of Calgary and Boeing Computer Services
A problem in the process of building knowledge-based systems is acquiring
and modeling appropriate problem-solving knowledge. The objective of this
workshop is to assemble theoreticians and practitioners who recognize the
need for developing methods and systems that assist the knowledge
acquisition process.
To encourage vigorous interaction and exchange of ideas the workshop will
be kept small - about 40 participants. There will be individual
presentations and ample time for technical discussions. An attempt will be
made to define the state-of-the-art and future research needs. Attendance
will be limited to those presenting their work, one author per paper.
Papers are invited for consideration in all aspects of knowledge
acquisition for knowledge-based systems, including (but not restricted to):
Transfer/modeling of expertise - systems that obtain and model knowledge
from experts; manual knowledge acquisition methods and techniques.
Generic ontologies and problem-solving techniques.
Apprenticeship, explanation-based, and other learning systems;
integration of such systems with other knowledge acquisition techniques.
Issues in cognition and expertise that affect the knowledge acquisition
process.
Extracting and modeling of knowledge from text.
Eliciting and modeling knowledge from multiple sources.
Integration of knowledge acquisition techniques within a single system;
integration of knowledge acquisition systems with other systems
(hypermedia, database management systems, simulators, spreadsheets...).
Knowledge acquisition methodology and training.
Validation of knowledge acquisition techniques; the role of knowledge
acquisition techniques in validating knowledge-based systems.
Five copies of a draft paper (up to 20 pages) should be sent to Brian
Gaines before April 22, 1992. Acceptance and revision notices will be
mailed by June 17. Revised papers (20 pages) should be returned to the
chairman by August 19 so that they may be bound together for distribution
before the workshop.
There will be a travel-and-expense award of up to $500.00 US for the best
paper submitted by a graduate student. Please note if the paper should be
considered for this award.
The structure of the 1992 Banff KAW will change significantly from previous
workshops. More time will be devoted to working groups. These groups will
organize and plan activities during the year . One goal of a working group
would be to produce a paper describing their work. Suggested topics
include:
Cognition (temporary coordinator: Brian Gaines, gaines@cpsc.ucalgary.ca)
Generic tasks (coordinator: Mark Musen, musen@camis.stanford.edu)
Knowledge acquisition and group decision support systems; knowledge
acquisition from multiple experts (coordinator: John Boose,
john@atc.boeing.com)
Knowledge sharing and reuse (coordinator: Jeff Bradshaw:
jbrad@atc.boeing.com)
Inductive modeling (temporary coordinator: Brian Gaines,
gaines@cpsc.ucalgary.ca)
Project Sisyphus (coordinator: Marc Linster, linster@gmdzi.gmd.de)
Please contact a coordinator if you would like to be involved in a working
group during the year. Project Sisyphus is concerned with different
research groups testing their approaches on the same datasets, and that
several statements and datasets are now available. Workshop Co-chairmen:
Send papers by April 22 to:
Brian Gaines
ATTN: Camille / KAW
Department of Computer Science
University of Calgary
2500 University Dr. NW
Calgary, Alberta, Canada T2N 1N4
(403) 220-5901
gaines@cpsc.Ucalgary.ca
John Boose
Advanced Technology Center
Boeing Computer Services, 7L-64
PO Box 24346
Seattle, Washington, USA 98124
(206) 865-3253
john@atc.boeing.com
Mark Musen
Medical Computer Science Group
Knowledge Systems Laboratory
Stanford University
School of Medicine
Stanford CA, USA 94305-5479
musen@camis.stanford.edu
------------------------------
Date: Tue, 17 Mar 92 11:11:16 CST
From: Keith Levi <levi@miu.EDU>
Subject: list of ml applications
Here is the list of ml applications that I received last year in
response to my query.
***********************************************************************
About a year ago I sent out a request to the Machine Learning list for
short descriptions to give to the Air Force Logistics Command of
completed, existing, proposed, or planned applications of machine
learning. They were considering whether machine learning technology
might be useful in their expert systems work. I do not believe that
they have yet done anything in these directions. However, they were
quite interested in some of the applications, and I understand they
are continuing to discuss possible machine learning projects.
I received about 14 email responses containing some 30 project
descriptions. These descriptions follow. I have loosely ordered them
according to three application classes: a) diagnostics, b)
database maintenance, and c) design, scheduling, and process control
sorts of applications.
I also received from Peter Clark (pete@uk.ac.turing) of the Turing
Institute a package containing a 1986 tech report describing 15 -20
applications of ID3 systems--e.g., the chemical process control system
for Westinghouse which Westinghouse states has resulted in an
additional $10M of business annually. He also included a bulletin for
the "Inductive Programming Special Interest Group" (IPSIG). The
bulletin, Pragmatica, is published biannually by the Turing Institute
Press--Jean Hayes-Michie (Editor).
This list is now about a year old, and even as of a year ago I'm sure
that it is missing a large proportion of existing and planned
applications of machine learning. Several government agencies
continue to be (or are beginning to be, or should be) interested in
applications of machine learning. I would like to compile a more
complete and up-to-date list of planned and existing machine learning
applications for the purpose of distributing to these agencies. If
people will send me short (2-3 sentence) descriptions of such projects
I will again edit the list and post it to the ML-List. Please also
include the following field descriptors for aiding in organizing the
list:
Operational Status: field-use, field-test, implementing, proposed
Application Objective: field-use, prototype, demonstration
ML Technique: EBL, SBL, GA (genetic algorithm), CBR, etc
Type of Application Problem: classification/diagnosis, monitering,
scheduling, planning, design, control, etc
Thanks much,
Keith R. Levi, Ph.D.
Assistant Professor
Computer Science Department
1000 N Fourth St
Maharishi International University
Fairfield, Iowa 52557-1143
levi@miu.edu
************************************************************************
From Angela.Hickman@ML.RI.CMU.EDU Fri Jan 31 10:17:17 1992
Date: Thu, 6 Dec 1990 10:20-EST
To: Keith Levi <levi@src.honeywell.com>
I worked on a project for Westinghouse that used ID3/C4 technology to
diagnose steam plant generator errors. For more information contact
William J. Leech
Advisory Engineer
Artificial Intelligence
Technology Enterprises
Westinghouse Electric Corporation
Power Systems Business Unit
Mosside Building 210C
2400 Mosside Boulevard
Monroeville, Pennsylvania 15146
(412) 374-7527
========
Date: Thu, 06 Dec 90 11:37:49 -0800
From: Paul O'Rorke <ororke@frost.ICS.UCI.EDU>
Keith, I'm working on machine learning and diagnosis. I have some
applications oriented projects going with Douglas Aircraft Company and
McDonnell Douglas Research Laboratories. One involves ultrasonic
non-destructive evaluation of aircraft parts. This is basically a
pattern recognition/ signal understanding task and so far we have
concentrated on inductive learning methods. We are also doing some
work on explanation-based learning for model-based diagnosis of
multiple faults. We have a paper on the first project in the upcoming
IEEE Aerospace Applications Conference and one on the second project
in the next IEEE Conference on Applications of AI.
========
Date: Tue, 18 Dec 90 09:15:08 PST
From: Leonard Friedman <friedman@venera.isi.edu>
I have developed the Learning Diagnostic Skills (LDS) system for AFLC. For a
class of devices (Ex: power supplies) LDS generates an expert system
automatically by partial evaluation of a causal model. We propose an
extension by which statistics of failure frequency are gathered and choice of
next test adjusted to maximize information gain and minimize cost.
========
Date: Mon, 20 May 91 15:42:38 PDT
From: Pat Langley <langley@ptolemy.arc.nasa.gov>
I'm particularly interested in recent work on applications to
diagnosis, since that's an area that Deepak Kulkarni and I have
recently entered.
Specifically, we're using simulated telemetry data from a component of
the space shuttle (the fuel cell), along with fault information, as
input to inductive learning algorithm. The output is a decision tree
that infers faults based on current sensor readings and recent trends
in the sensor stream.
The work is still preliminary, and we have lots of ideas about how
to make it more sophisticated, but it's something we can get off the
ground quickly. -Pat
P.S. I also heard recently about an application at Vanderbilt that
involves predicting likely sites at which oil will occur. I can
put you in touch with the people there if you want to know more.
========
Date: Wed, 12 Dec 90 09:56:58 PST
From: kew@atc.boeing.com
Below are some real-world applications of ML that I know of.
By far the biggest sort of application is the analysis of
large databases in an attempt to induce an expert system.
In addition, some work has occured at Rutgers Univeristy by
Peter Politakis and Allen Ginsberg on using ML techniques to
refine medical expert systems based on test cases coverage.
Both published their theses in the Research Notes in Artificial
Intelligence Series of books from Morgan Kaufman Publishers.
Allen's e-mail address is abg%vax135@research.att.com. He is
familiar with Peter's work. You might want to contact him.
Donald Michie has done alot for the field in terms of working
on successful applications of ML techniques.
Keith Williamson
kew@atc.boeing.com
Peter Cheeseman's AutoClass system at NASA Ames used
statistical techniques to discover classes of stellar spectra.
"AutoClass discovered classes which differed significantly from NASA's
previous analysis but clearly reflect physical phenomena in the
data....The AutoClass infrared source classification is the basis of a
new star catalog to appear shortly." (ref 5)
Leo Breiman used a similarity-based algorithm to derive a decision
system for identifying high-risk patients. "At the University of
California, San Diego Medical Center, when a heart attack patient is
admitted, 19 variables are measured during the first 24 hours. These
include blood pressure, age, and 17 other ordered and binary variables
summarizing the medical symptoms considered as important indicators of
the patients condition. The goal of a recent study was the
development of a method to identify high risk patients (those who will
not survive at least 30 days) on the basis of the initial 24 hours."
(ref 6) This decision system has subsequently been used for decision making at
this hospital.
Rulemaster, infers expert system rules from training exemplars.
Applications of the expert systems derived were installed by various
companys and include forecasting severe weather in the central US,
forecasting defaults on loan applications, decision system for using
the automatic lander for the space shuttle, transformer fault
diagnosis, forecasting credit card defaults, decision system for gilt
market switching, and GASOIL which performs hydrocarbon separation
system specification.
The work of Shapiro extended data-oriented induction into the realm
of Computer Assisted Software Engineering (CASE). "The world's
largest expert system, BMT, for configuring fire-detection equipment,
was built using this CASE-oriented style by the West Berlin company
Brainware....BMT consists of 150,000 lines of inductively
generated C code and is in routine use by the client organization.
The total figure of 9 man-years expended on the project is inclusive
of management, support staff and domain specialists as well as
programmer time." (ref 7).
"Yugoslav work ... using a computer model of the human heart
resulted in the discovery by machine of new clinical rules for
interpreting electro-cardiogram patterns. Work at Glasgow's Turing
Institute on diagnosing electronic faults in a space satelite
confirmed the methodology ... These sythetic rules-bases
comprise new diagnostic know-how beyond the achievments of human
specialists"(ref 7)
Netman (ref 8) combines similarity-based learning and explanation
based learning to adapt a network traffic management system based on
its own experiences and by observing other agents. This system has
been used at GTE and been shown to achieve lower error rates than
human controllers.
5) Cheeseman, Peter; Kelly, James; Self, Matthew; Stutz, John; Taylor,
Will; Freeman, John; "AutoClass: A Bayesian Classification System" in
{\em Proceedings of the Fifth International Confernece on Machine
Learning}, John Laird (Ed.), Morgan Kaufmann Publishers, San Mateo,
Calif., 1988.
6) Breiman, L, Friedman, J.H., Olshen R.A., and Stone, C.J., {\em
Classification and Regression Trees}, Monterey, Ca: Wadsworth and
Brooks.
7) Michie, Donald, "Inducing Knowledge From Data: First Principles"
presented at {\em Proceedings of the Seventh International Confernece
on Machine Learning}, Bruce W. Porter and Ray J. Mooney (Eds.), Morgan
Kaufmann Publishers, San Mateo, Calif., 1990.
8) Silver Bernard, "{\em NetMan}: A Learning Network Traffic
Controller" in {\em Proceedings of the Third International Conference
on Industrial \& Engineering Applications of Artificial Intelligence \&
Expert Systems}, Manton M. Matthews (Ed.), Association for COmputing
Machinary Press, 1990.
========
Date: Thu, 6 Dec 1990 7:37:56 EST
From: Tom Mitchell <mitchell@DAYLILY.LEARNING.CS.CMU.EDU>
We're doing a bunch of things at CMU. Here is one that they might find
interesting:
1. Machine learning for database maintenance. Rules are derived for
predicting attribute values in an existing database, from known attribute
values. These rules are then used to check new database entries to detect
suspicious attribute values and signal potential errors in new entries.
In preliminary tests, this system has been able to find significant rules
and detect several errors in an existing database (describing DEC computer
components). The system (based on the Theo learning framework) is now
being tested for possible use at DEC. Primary investigators: Jeff
Schlimmer, Tom Mitchell, John McDermott.
========
Date: Mon 10 Dec 90 12:24:47-PST
From: Boy Guy <BOY@PLUTO.ARC.NASA.GOV>
I am currently working on Computer Integrated Documentation at NASA.
This project includes machine learning and knowledge acquisition
methods and techniques appropriate for information access. Do you
think that such a thing would be interesting for your needs?
========
From Jeff.Schlimmer@CS.CMU.EDU Fri Jan 31 10:18:20 1992
Date: Wed, 12 Dec 90 16:39:53 EST
Self Modeling Databases (Schlimmer, Mitchell, McDermott): Inductive
machine learning methods are used to construct a set of rules that
characterize how attributes are used to describe database entries.
The rules identify unexpected values in new database entries.
Syntactic Text Transformation (Schlimmer): Apprenticeship learning
methods are used to construct syntactic text transformations. The
transformations automate the process of cataloging new documents by
converting existing, but idiosyncratic formats into a library standard
one.
========
Date: Fri, 7 Dec 90 09:58:28 PST
From: Tom Dietterich <tgd@turing.CS.ORST.EDU>
In progress:
1. Learning rules for pronouncing English words (mapping from words to
phoneme and stress strings). We have learned a set of decision
trees that out-performs the best commercial letter-to-sound rule
rule-base (DECtalk).
2. Isolated letter speech recognition. We are working with Ron Cole
at Oregon Graduate Institute to automate directory-assistance
applications. Currently, we are working on isolated letter
speaker-independent speech recognition. We have attained an
accuracy of 96.7%, which is the best known accuracy on this task.
We are employing neural network learning methods (backpropagation).
In the proposal stage:
3. Given a first-order theory of plant physiology, we are using
learning methods to refine this theory so that it can be applied to
predict the location and distribution of plant ecosystems. The
learned theory will be applied to predict the effects of global
warming on forests and farming.
Thomas G. Dietterich
Department of Computer Science
Dearborn Hall, 303
Oregon State University
Corvallis, OR 97331-3102
503-737-5559
========
Date: Fri, 21 Dec 1990 11:31:40 PST
From: William Mark <mark@sumex-aim.stanford.edu>
I don't know if this very late reply will do you any good, but we
(the Lockheed AI Center) have several ML applications that might be of
interest. Given your stated criterion, the most interesting is
probably Clavier, a system that aids in loading autoclaves as
part of the process of manufacturing composite parts. Clavier
maintains and updates a knowledge base of successful part layouts to
assist in determining how a list of prioritized parts to be produced
can be cured in the autoclave so as to maximize throughput. If the user
modifies a system-proposed layout, and that layout is successful, the
system learns the new layout by incorporating it into its knowledge base
to allow later retrieval. Clavier is a fielded application, in use
by real people doing real production.
Another application (this one being built under DARPA sponsorship) involves
learning fault recovery scenarios for fixing highly automated machine tools.
We are participating with Cincinnati Milacron on that effort. The technology
this time is EBL, applied to indexing actual fault recovery cases in a
memory. This system is about a year away from fielding.
========
Date: Fri, 21 Dec 90 15:38:54 EST
From: Usama M Fayyad <umf@caen.engin.umich.edu>
On applications of machine learning:
We have applied our system, GID3, to several tasks
with several companies in SRC. Our focus has been on
applying GID3 to the automation of semiconductor manufacturing.
Most of the applications deal with diagnosing the Reactive Ion Etching
(RIE) Process. GID3 has also been used at Harris to derive
rules for use in an Emitter Piloting Deposition expert
system. Our recent applications include deriving qualitative
rules from response surfaces (RSM) to be used in process
optimization: the rules decide which direction the parameters
should be shifted in order to compensate for drifts in
the optimal operating point derived by process engineers.
Usama Fayyad
AI Lab, EECS Dept.
The University of Michigan.
for a ref. see the paper by Irani, Cheng, Fayyad, and Qian
in proceeding of Applications of Artificial Intelligence VIII,
Orlando, Fl, April, 1990. (S.P.I.E. is publisher).
========
Date: Thu, 3 Jan 91 11:26:25 PST
From: Steve Minton <minton@ptolemy.arc.nasa.gov>
Two EBL applications-oriented projects are:
1. Scheduling system that uses EBL done here at NASA. (See Eskey and
Zweben's AAAI-90 paper)
2. Work by Manny Rayner and Christer Samuelsson using EBL as a
compilation technique for an NL parser. Samuelsson can be reached
at sam@sics.se (that's the Swedish inst. for computer science).
(See IJCAI-91).
========
Date: Tue, 5 Feb 91 22:35 CST
From: ZYTKOW@wsuiar.wsu.ukans.edu
a. FAHRENHEIT and automated discovery
FAHRENHEIT is an implemented research system that discovers
multidimensional functions from data. A number of discovery tasks
have been automated, including control over experimentation,
collection of experimental data, and theory development.
b. Forty-Niner and database discovery
Forty-Niner is an implemented system that explores relational
databases in search for useful regularities. Forty-Niner's large
scale search for multidimensional regularities can be guided by user's
knowledge.
c. Tactical planning
A software system BOGEY has been developed that makes real time
decisions for an autonomous airplane in a simulated combat
environment. The autonomous pilot monitors its own performance,
checking the safety, resources, and effectiveness of currently
executed plans and dropping those that become dangerous or
counterproductive.
d. Learning better tactics from experience
BOGEY includes a mechanism for learning tactics from experience.
Based on a large number of simulated combat situations, a search in
the space of experimental results leads to the improved performance.
========
Date: Wed, 4 Mar 92 10:03:43 CST
From: levi@miu.edu (Keith Levi)
Honeywell, in collaboration with other researchers around the
country, has been applying EBL to the problem of developing and
maintaining the knowledge bases of an intelligent automated associate.
This project is now entering its fifth year. To date, we have
developed two proof-of-principle demonstrations. One demonstration
uses a domain theory about tactical maneuvers to learn plans for the
Tactics Planner module of the Air Force's Pilot's Associate system.
The other demonstration generates information requirements for the
learned tactics plan. These information requirements are used in the
Pilot-Vehicle Interface module of the PA.
We have recently prepared a very thorough technical report on all
aspects of the project. This is available upon request. We will also
have an article in IEEE Expert's Special Track on Applications of
Machine Learning. This should be appearing in the next few months.
------------------------------
END of ML-LIST 4.7