Copy Link
Add to Bookmark
Report
Machine Learning List Vol. 4 No. 03
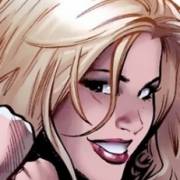
Machine Learning List: Vol. 4 No. 3
Monday Feb. 10, 1992
Contents:
Workshop on Machine Learning at CSCSI
Asymmetric Neural Networks and Structure Design by Genetic Algorithms
NEC Symposium: Computational Learning and Cognition
The Effective Number of Parameters in Nonlinear Learning Systems
Degrees of Freedom for Signal
The Machine Learning List is moderated. Contributions should be relevant to
the scientific study of machine learning. Mail contributions to ml@ics.uci.edu.
Mail requests to be added or deleted to ml-request@ics.uci.edu. Back issues
may be FTP'd from ics.uci.edu in pub/ml-list/V<X>/<N> or N.Z where X and N are
the volume and number of the issue; ID: anonymous PASSWORD: <your mail address>
------------------------------
From: Stan Matwin <stan@csi.uottawa.ca>
Subject: WORKSHOP ON MACHINE LEARNING at CSCSI
Date: Fri, 7 Feb 92 19:25:46 EST
The previous announcement looked to some people as if it was limited to
Canadians, which was not at all the intent.
WORKSHOP ON MACHINE LEARNING (PLS. POST/PASS AROUND)
Announcement and call for Papers
The workshop will be held May 12, 1992, in Vancouver, in conjunction
with AI'92, the Ninth Biennial conference of the Canadian Society for
Computational Studies of Intelligence (CSCSI).
The workshop will consist of presentations, discussions, invited talk
and a panel. Steve Minton (PRODIGY, utility, learning and planning)
will be giving the invited talk, and Peter Clark (CN2, OPTIMIST) is in
charge of the panel.
Attendance at the workshop will be by invitation only. Participants
from all countries are invited. Persons interested in attending are
requested to submit a short (maximum 500 words) description of their
current work in Machine Learning. Those interested in making a
presentation should also submit an extended abstract (maximum 2000
words) describing the proposed presentation. Fax submissions are
possible. A selection of the submitted material will be distributed to
all workshop attendees.
The organizers also invite suggestions for discussion topics. All submissions
are due on February 15, 1992, and should be sent to:
Robert Holte
Computer Science Department
University of Ottawa
Ottawa, Ontario
CANADA K1N 6N5
email: holte@csi.uottawa.ca
phone: (613)-564-9194
FAX: (613)-564-9486
Organizing Committee:
Robert Holte (holte@csi.uottawa.ca)
Computer Science Department, University of Ottawa
Charles Ling (ling@csd.uwo.ca)
Computer Science Department, University of Western Ontario
Stan Matwin (stan@csi.uottawa.ca)
Computer Science Department, University of Ottaw
Stan Matwin Department of Computer Science
email: stan@csi.uottawa.ca University of Ottawa
phone: (613) 564-5069 Ottawa, Ont.
fax: (613) 564-9486 K1N 6N5 Canada
------------------------------
Date: MON, 27 JAN 92 15:55:31 MEZ
From: Stefan Bornholdt <T00BOR%DHHDESY3.bitnet@cunyvm.cuny.EDU>
Subject: Asymmetric Neural Networks and Structure Design by Genetic Algorithms
The following paper is available, hardcopies only.
GENERAL ASYMMETRIC NEURAL NETWORKS AND
STRUCTURE DESIGN BY GENETIC ALGORITHMS
Stefan Bornholdt
Deutsches Elektronen-Synchrotron DESY, Notkestr. 85, 2000 Hamburg 52
Dirk Graudenz
Institut f\"ur Theoretische Physik, Lehrstuhl E, RWTH 5100 Aachen,
Germany.
A learning algorithm for neural networks based on genetic algorithms
is proposed. The concept leads in a natural way to a model for the
explanation of inherited behavior. Explicitly we study a simplified
model for a brain with sensory and motor neurons. We use a general
asymmetric network whose structure is solely determined by an
evolutionary process. This system is simulated numerically. It turns
out that the network obtained by the algorithm reaches a stable state
after a small number of sweeps. Some results illustrating the
learning capabilities are presented. [to appear in Neural Networks]
preprints available from:
Stefan Bornholdt, DESY-T, Notkestr. 85, 2000 Hamburg 52, Germany.
Email: t00bor@dhhdesy3.bitnet (hardcopies only, all rights reserved)
------------------------------
Date: Mon, 3 Feb 92 10:12:58 EST
From: "Eric B. Baum" <eric@research.nj.nec.COM>
Subject: NEC Symposium: Computational Learning and Cognition
Third Annual NEC Symposium:
COMPUTATIONAL LEARNING AND COGNITION
PRINCETON NJ
MAY 27-28, 1992
NEC is pleased to announce that the Third Annual NEC Symposium
will be held at the Hyatt Regency Hotel in Princeton NJ on May 27
and 28, 1992. The title of this year's symposium is Computational
Learning and Cognition. The conference will feature 12 invited
talks. The speakers are:
Dana Angluin, Yale U.
"Learning with Queries"
Kunihiko Fukushima, Osaka U.
"An Improved Neocognitron Architecture and Learning Algorithm"
Charles Gross, Princeton U.
"Inferior Temporal Cortex is a Pattern Recognition Device"
David Haussler, UCSC
"How Well Do Bayes Methods Work?"
Mitsuo Kawato, ATR
"Supervised Learning for Coordinative Motor Control"
Hector Levesque, U. Toronto
"Is Reasoning Too Hard?"
Tom Mitchell, CMU
"Software Agents that Learn from Users"
David Rumelhart, Stanford U.
To be announced
Stuart Russell, UC Berkeley
"On Rational Agents with Limited Performance Hardware"
Haim Sompolinsky, Hebrew U.
"Continuous and Discontinuous Learning"
Manfred Warmuth, UCSC
"On Weak Learnability"
Kenji Yamanishi, NEC
"Statistical Approach to Computational Learning Theory"
There will be no contributed papers. Registration is free of charge but space
is limited. Registrations will be accepted on a first come first served basis.
YOU MUST PREREGISTER. There will be no onsite registration.
To preregister by e-mail send a request to dale@research.nj.nec.com. You will
receive an acknowledgement and an invitation, space allowing. Preregistration
is also possible by regular mail to Ms. Dale Ronan, NEC Research Institute,
4 Independence Way, Princeton NJ 08540.
Registrants are expected to make their own arrangements for
accomodations. As a service, we provide below a list of hotels in the
area, together with corporate rates. You should ask for the Corporate
Rate when reserving your room. Sessions will start at around 8:30 AM
Wednsday, and will be scheduled to finish at around 3:30 PM on
Thursday May 28.
Red Roof Inn, South Bruswick-(908)821-8800 $29.99
McIntosh Inn, Lawrenceville-(609)896-3700 $39.95
Days Inn, South Bruswick-(908)329-4555 $44.95
Palmer Inn, Princeton-(609)452-2500 $65.00
Novotel Hotel, Princeton-(609)520-1200 $85.00
Summerfield Suites, Princeton-(609)951-0009 $89.00*
Ramada Inn, Princeton-(609)452-2400 $89.50
Marriott Residence Inn, Princeton-(908)329-9600 $94.00*
Hyatt Regency, Princeton-(609)987-1234 $105.00*
Marriott Hotel, Princeton-(609)452-7900 $140.00
*In order to obtain these rates, the person making the reservation
must state that he or she is attending the NEC Research Symposium
to the reservationist.
------------------------------
From: John Moody <moody-john@cs.yale.EDU>
Date: Mon, 10 Feb 92 09:24:57 -0800
Subject: The Effective Number of Parameters in Nonlinear Learning Systems
The following paper has been placed in the Neuroprose archive in file
moody.p_effective.ps.Z . Retrieval instructions follow the abstract.
Thanks to Jordan Pollack for continuing to maintain this very useful archive.
John Moody
The Effective Number of Parameters:
An Analysis of Generalization and Regularization
in Nonlinear Learning Systems
John E. Moody
Department of Computer Science, Yale University
P.O. Box 2158 Yale Station, New Haven, CT 06520-2158
We present an analysis of how the generalization performance (expected test
set error) relates to the expected training set error for nonlinear learning
systems, such as multilayer perceptrons and radial basis functions. The
principal result is the following relationship (computed to second order)
between the expected test set and training set errors:
<E_test(l)> \approx <E_train(l)> + 2 (s_eff)^2 p_eff(l) / n
Here, n is the size of the training sample, (s_eff)^2 is the effective
noise variance in the response variable(s), l is a regularization or
weight decay parameter, and p_eff(l) is the effective number of
parameters in the nonlinear model. The expectations < > of training
set and test set errors are taken over possible training sets and
training and test sets respectively. The effective number of
parameters p_eff(l) usually differs from the true number of model
parameters p for nonlinear or regularized models; this theoretical
conclusion is supported by Monte Carlo experiments. In addition to
the surprising result that p_eff(l) is not equal to p, we propose an
estimate of <E_test(l)> called the generalized prediction error (GPE)
which generalizes well established estimates of prediction risk such
as Akaike's FPE and AIC, Mallows C_P, and Barron's PSE to the
nonlinear setting.
To retrieve the paper by anonymous ftp:
unix> ftp archive.cis.ohio-state.edu # (128.146.8.52)
Name: anonymous
Password: neuron
ftp> cd pub/neuroprose
ftp> binary
ftp> get moody.p_effective.ps.Z
ftp> quit
unix> uncompress moody.p_effective.ps.Z
unix> lpr -P <printer name> moody.p_effective.ps
------------------------------
Date: Mon, 10 Feb 92 12:10:39 -0600
From: Grace Wahba <wahba@stat.wisc.EDU>
Subject: Degrees of Freedom for Signal
[Editor's Note: A previous draft of this message was sent to John
Moody, who helped clarify some of the issues raised in the note. This
note also indicates that people outside of computer science are
starting to care about machine learning. In my opinion, it's about
time that people in machine learning start caring about methods for
classification and prediction that have been developed in other
fields- Mike]
This message is related to the paper "The Effective Number of
Parameters: An Analysis of Generalization and Regularization in
Nonlinear Learning Systems" by John E. Moody, obtainable by ftp (see
previous message).
Moody presents a general expression in his equation (15) for what
he calls the "effective number of parameters" for a nonlinear model.
It is the trace of what he calls the "generalized influence matrix".
We would like to note that the special case of Moody's (15) which is
called p_{lin}(\lambda) in Moody's equation (18) and given the name
"linearized effective number of parameters" by him is known in the
quadratic case in the Statistics and Meteorological literature as the
"degrees of freedom for signal" (see G. Wahba, Bayesian "Confidence
Intervals" for the cross-validated smoothing spline, J. Royal
Statistical Society B, 45, 1, 1983, p 139; Buja, Hastie and
Tibshirani, Linear Smoothers and Additive Models, Ann. Statist. 17,
1989, p. 470; G. Wahba, Design criteria and eigensequence plots for
satellite computed tomography, J. Atmos. Ocean Technology, 2, 125-132,
1985). In this last paper the degrees of freedom for signal is proposed
as a design criteria when choosing what information to collect.
Moody proposes a very interesting nonlinear generalization of the
influence matrix in his (15). Two other nonlinear generalizations of
the influence matrix, in conjunction with the GCV method for choosing
the regularization parameter, are discussed in my book "Spline Models
for Observtional Data", v 59 in the CBMS-SIAM Regional Comference
Series in Applied Mathematics, (1990), equations (8.3.8) and (9.2.18)
(available from SIAM, pubs@siam.org, cheap!) and
G. Wahba, Ill posed problems: Numerical and statistical methods for mildly,
moderately, and severely ill posed problems with noisy data. University of
Wisconsin-Madison Statistics Department Technical Report No. 595, February
1980. (Hard copy available if you send your s-mail address to
gao@stat.wisc.edu with TR595 in the body).
Equation (8.3.8) involves only first derivatives and not the inverse
hessian as Moody (wisely) suggests. Equation (9.2.18) appears to be
consistent with Moody's definition in the special case considered in
(9.2.18) which involves independent observations which are random
variables with a density from an exponential family, and a purely
quadratic penalty functional. It differs from Moody's definition in
(15), which was intended to cover observations with a common variance,
by a diagonal matrix which weights for differing variances. Moody
(personal communication) has obtained a more general result for
non-uniform variance. which contains (9.2.18) as a special case.
Recent work by Chong Gu (A note on cross- validating non Gaussian
data, mss, Nov. 16, 1991, available from the author by writing
chong@pop.stat.purdue.edu) gives a better method for estimating the
smoothing parameter in the (9.2.18) case with binomial data, than is
given in following (9.2.18). Gu's method generalizes the unbiassed
risk estimate given in Craven and Wahba, Numer. Math. 31, 377-403,
(1979) which can be used when the variance is known. Gu also has a
different iteration than that implied following (9.2.18). It appears
that in the purely quadratic and Gaussian case Moody's criteria (14)
reduces to the unbiassed risk estimate of Craven and Wahba, equation
(1.8). (You have to collect all the trace terms in (1.8) to see
this.) This equation (1.8) can also be found in Wahba(1990), (4.7.1).
There are 13 pages of references to the smoothing and ill-posed
problems literature in the book above- I think a lot of this
work will be of interest to connectionists who are
interested in the bias-variance tradeoff, and, of course
a lot of statisticians will, likewise be very interested
in Moody's results.
------------------------------
END of ML-LIST 4.3