Copy Link
Add to Bookmark
Report
Machine Learning List Vol. 3 No. 10
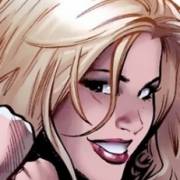
Machine Learning List: Vol. 3 No. 10
Thursday, June 6, 1991
Contents:
Machine Learning Journal: Computer Models of Human Learning
Automated Knowledge Acquisition Workshop at ML91
Research Assistant: STRATHCLYDE UNIVERSITY
Postdoc at University of Ottawa
European Summer School on Machine Learning. [Very Long, in TeX]
The Machine Learning List is moderated. Contributions should be relevant to
the scientific study of machine learning. Mail contributions to ml@ics.uci.edu.
Mail requests to be added or deleted to ml-request@ics.uci.edu. Back issues
may be FTP'd from ics.uci.edu in /usr2/spool/ftp/pub/ml-list/V<X>/<N> or N.Z
where X and N are the volume and number of the issue; ID & password: anonymous
------------------------------
Subject: Special Issue of Machine Learning Journal
Date: Tue, 04 Jun 91 22:19:51 -0700
From: Michael Pazzani <pazzani@pan.ICS.UCI.EDU>
Subject: Machine Learning Journal: Computer Models of Human Learning.
MACHINE LEARNING will be publishing a special issue on Computer Models
of Human Learning. The ideal paper would describe an aspect of human
learning, present a computational model of the learning behavior,
evaluate how the performance of the model compares to the performance
of human learners, and describe any additional predictions made by the
computational model. Since it is hoped that the papers will be of
interest to both cognitive psychologists and computer scientists,
papers should be clearly written and provide the background
information necessary to appreciate the contribution of the
computational model.
Manuscripts must be received by April 1, 1992, to assure full
consideration. One copy should be mailed to the editor:
Michael Pazzani
Department of Information and Computer Science
University of California,
Irvine, CA 92717
USA
In addition, four copies should be mailed to:
Karen Cullen
MACH Editorial Office
Kluwer Academic Publishers
101 Philip Drive
Assinippi Park
Norwell, MA 02061
USA
Papers will be subject to the standard review process. Please pass
this announcement along to interested colleagues.
------------------------------
Date: Wed, 29 May 91 08:48:30 CDT
From: Ray Bareiss <bareiss@zettel.ils.nwu.EDU>
Subject: Automated Knowledge Acquisition Workshop at ML91
The program committee of the Automated Knowledge Acquisition Workshop of
ML91 invites you to attend any or all sessions of our workshop. Because
ML91 has open registration this year, it is not necessary to notify us in
advance of your desire to attend workshop sessions. We especially would
like to commend the invited talk by Bruce Buchanan to your attention;
this talk is likely to be one of the highlights of ML91. We hope to
see you there.
Ray Bareiss
bareiss@ils.nwu.edu
(708)491-3500
Schedule for the Automated Knowledge Acquisition Workshop of ML91
Thursday, June 27
[Note: Some other workshops have paper presentations Thursday AM- MP]
5:30-7:00 ML91 combined poster session
Friday, June 28
9:00-10:30 Paper presentations
11:00-12:30 Panel: Capturing design rationale (chair - Tom Gruber)
2:00-3:00 ML91 invited talk (combined session)
3:30-5:00 Paper presentations
Saturday, June 29
9:00-10:30 ML91 invited talk (combined session)
11:00-12:00 Workshop invited talk: Bruce Buchanan <---- !!!
1:30-3:00 Working group sessions (Topics are to be determined by
workshop participants.)
3:30-5:00 ML91 summary panel session (combined session)
------------------------------
From: David Aha <aha@turing.ac.UK>
Subject:Research Assistant: STRATHCLYDE UNIVERSITY STATISTICS DEPARTMENT
Date: Wed, 29 May 91 19:17:47 BST
PLEASE DRAW THE ATTENTION OF RECENTLY GRADUATED PH.D. STUDENTS
TO THE FOLLOWING POST.
Due to the withdrawal of Prof. D. Michie, a vacancy for a
Research Assistant has arisen in the StatLog project based
at the University of Strathclyde. This is a temporary post
with duration June/July 1991 to June/July 1992, with a probable
extension for part of the following year. Alternatively, it
might be possible to arrange a part-time appointment over two years.
The project ends in March 1993.
The remuneration would be on the RA 1A scale
(11399 to 18165 pounds per annum).
A separate sum of money would be available for travel.
STATLOG PROJECT
This is an Esprit project which has two years still to run.
The STATLOG project compares many types of Machine-Learning and
statistical algorithms on problems in discrimination,
prediction and control, with an emphasis on large-scale,
complex commercial and industrial problems. The overall aim is
to give an objective assessment of the potential of the chosen
algorithms in solving significant commercial and industrial problems,
and to widen the foundation for commercial exploitation of these and
related algorithms both old and new. As part of its remit, the
project will investigate protein structure, prediction of financial
time series, and the optimal control of a complex system of
thrusters in a satellite. The methods to be used include some of
the more fashionable methods such as causal trees, neural nets and genetic
algorithms, as well as the more traditional methods like discriminant
analysis and decision trees.
STRATHCLYDE UNIVERSITY STATISTICS DEPARTMENT
Strathclyde supplies the Technical leadership in the project, with
three members of the Academic Staff involved. In addition,
two Research Assistants are already employed full-time on the
project.
At Strathclyde, we have special responsibility for classical
statistical methods, but we are also interested in
the more modern ones like projection pursuit. Also we intend
to dabble in some of the non-statistical procedures like neural nets,
and to this end we have obtained a copy of Richard Rohwer's suite
of neural net programs.
As part of our responsibilites, visits to and by the other
partners are expected. The other partners are:
Brainware (Berlin); Daimler-Benz (Ulm, Germany);
Granada University (Spain); ISoft (Gif sur Yvette, France);
Luebeck University (Germany); M.B.B. (Munich, Germany);
Porto University (Portugal); Turing Institute (Glasgow).
We would expect the person appointed to this post to help in
the application of several statistical procedures to a wide
variety of datasets. Ability to program in C, Fortran or Splus,
or familiarity with Sun workstations would be an advantage.
If this is of any interest to you, please e-mail us a
potted C.V., and even a reference or two if you feel keen.
------------------------------
From: Stan Matwin <stan@csi.uottawa.ca>
Date: Fri, 31 May 91 16:24:11 EDT
Subject: Postdoc at University of Ottawa
The Department of Computer Science, University of Ottawa, together with the
School of Computer Science, Carleton University, has obtained a
grant from the Natural Sciences and Engineering Research Council of Canada
for a project entitled "Machine Learning Applied to Software Reuse".
We invite applications for a postdoctoral position on this project.
The ideal candidate will have a record of original work in symbolic learning,
case-based or analogical reasoning, or AI-based software design tools.
The position is available immediately and guaranteed for a two and a half
year period.
Candidates must have a Ph.D. prior to accepting the position.
The postdoctoral position is a crucial part of the project's team,
consisting of three
faculty and several graduate students at both M.Sc. and PhD. level.
The postdoctoral fellow will actively participate in all aspects
of the project, including all major research decisions.
Duties include
exploratory research in the area of the project, participation in the
design and development of a prototype system, participation in supervision
of graduate students involved in the project, and participation in the
publications resulting from the project. The salary is $27,500/yr;
an excellent benefit package is provided.
The research environment in Ottawa includes a joint graduate program with
some 35 CS faculty, of which six work in AI, a weekly machine learning seminar,
and a team of some 15 graduate students actively interested in machine learning,
as well as a number of externally-funded research projects in machine learning.
Ottawa, Canada's capital, is a pleasant city of 800,000, surrounded by
beautiful countryside, with a unique bilingual atmosphere.
Interested persons please contact Stan Matwin or Rob Holte at the addresses
below.
Stan Matwin Rob Holte
stan@csi.uottawa.ca holte@csi.uottawa.ca
(613) 564 5069 (613) 564 9194
Department of Computer Science
University of Ottawa
Ottawa, Ont.
K1N 6N5 Canada
------------------------------
Date: Mon, 27 May 91 15:18:16 +0200
From: Walter Van De Velde <walter@arti9.vub.ac.be>
Subject: ES2ML
Mike, here is a full information file on the European Summer School on
Machine Learning.
Walter Van de Velde
Artificial Intelligence Laboratory
Vrije Universiteit Brussel
Pleinlaan 2, B-1050 Brussels
Tel: (+32)2/641 29 65
Fax: (+32)2/641 28 70
Email: walter@arti.vub.ac.be
%==========================================================================%
% File name ES2ML.TEX
% Authors Walter Van de Velde, walter@arti.vub.ac.be
% Contents This file contains complete information about the
% EUROPEAN SUMMER SCHOOL ON MACHINE LEARNING 1991:
% General Information
% Registration Form
% Programme
% Course Outlines
% Purpose Free distribution over network and TeX-ing
% COPYRIGHT By Walter Van de Velde and the Authors of the Course Outlines
% Released 20.05.91 walter@arti.vub.ac.be
%==========================================================================%
\documentstyle[local,11pt]{article}
% Two New Commands
\newcommand{\courseheader}[3]{%
\newpage \begin{flushleft}\vskip 2.5cm \setcounter{footnote}{0} {\Large\bf #1}
\vskip .7cm {\bf #2} \vskip .3cm #3 \vskip 1cm \end{flushleft}}
\newcommand{\stag}[2]{%
\begin{list}{}{\leftmargin 3cm \labelwidth 2.5cm \parsep 0pt \topsep 0pt
\labelsep 0.5cm} \item[\mbox{\em #1 \hfill}] #2 \end{list}}
% End Of New Commands
% Dimensions:
\textheight 22cm % Height of text (including footnotes and figures,
\textwidth 15cm % Width of text line.
\topmargin 0pt % Nominal distance from top of page to top of
\headheight 12pt % Height of box containing running head.
\headsep 25pt % Space between running head and text.
\footheight 12pt % Height of box containing running foot.
\footskip 30pt % Distance from baseline of box containing foot
% End of Dimensions
\begin{document}
% \pagestyle{myheadings}
\title{\bf European Summer School on Machine Learning}
\author{Priory Corsendonk\\ Oud-Turnhout, Belgium}
\date{July 22-31, 1991}
\maketitle
\smallskip
\section{General Information}
The European Summer School on Machine Learning (ES2ML) is a non-profit
event organized by the European Machine Learning community. Its goal
is to educate on the state of the art in Machine Learning, the
subfield of Artificial Intelligence which is concerned with
computational theories of systems that learn. The school approaches
Machine Learning both as a research discipline and as a tool for
developing real-world applications.
ES2ML emphasizes the acquisition of research and development skills in
the area of Machine Learning. In addition, it serves as a forum for
exchanging views on current practice, needs and opportunities in the
research and industrial community. The objective is to learn not only
about Machine Learning but also about the broader context in which
this research is to be oriented, organized, validated and ultimately
turned into practice.
ES2ML-91 is patronized by the following organizations:
\begin{quote}
European Coordinating Committee for Artificial Intelligence (ECCAI)\\
Belgian Association for Artificial Intelligence (BAAI)\\
Commission of the European Communities (CEC)\\
Belgian National Science Foundation (NFWO/FNRS)\\
Institute for Scientific Research in Industry and Agriculture
(IWONL/IRSIA)
\end{quote}
Additional sponsoring has been obtained from the following companies:
\begin{quote}
ALCATEL BELL\\
AMERICAN AIRLINES\\
KREDIETBANK\\
KNOWLEDGE TECHNOLOGIES
\end{quote}
ES2ML-91 is organized by Walter Van de Velde (B) and
Carl-Gustav Jansson (S).
\newpage
All mail concerning the summer
school should be sent to Walter Van de Velde at the following
address:
\begin{quote}
ES2ML-91\\
attn. Walter Van de Velde\\
Artificial Intelligence Laboratory\\
Vrije Universiteit Brussel\\
Pleinlaan 2, B-1050 Brussels, Belgium\\
Tel: (+32) 2 641 29 65 or 29 78\\
Fax: (+32) 2 641 28 70\\
Email: es2ml@arti.vub.ac.be\\
\end{quote}
\section{Teachers, assistants and advisory board}
David Aha (USA),
Ivan Bratko (Yu),
Maurice Bruynooghe (adv, B),
Yuval Davidor (Israel),
Luc De Raedt (B),
Achim Hoffmann (FRG),
Carl-Gustav Jansson (org, S),
Ryszard Michalski (USA),
Tom Mitchell (USA),
Stephen Muggleton (UK),
Enric Plaza (E),
Luc Steels (adv, B),
Katia Sycara (Greece),
Walter Van de Velde (org, B),
David Wilkins (USA).
\section{Programme}
The objectives of the third ES2ML are realized through an intensive
9-day course with hands-on practice, case- studies and plenty of time
for discussions and informal interactions. The participants have free
choice out of more than 60 hours of intensive classes, presentations,
practice, demonstrations and discussions. The various activities will
be flexibly organized and tuned to meet the audience's preferences and
interests.
The main courses will be focussed on, but not limited to, the
following areas of Machine Learning:
\begin{itemize}
\item{Empirical and Analytical Learning Techniques}
\item{Incremental and Integrated Learning}
\item{Case-based and Memory-based Reasoning}
\item{Genetic Algorithms}
\item{Computational Learning Theory}
\end{itemize}
Attendants wishing to deepen their understanding of state-of-the-art
techniques will acquire hands-on practice. Teaching assistants provide
guidance in small- scale implementation and application courses. In
addition a variety of demonstration systems will be present on-site.
Realistic case-studies are selected to emphasize emerging guidelines
and methodologies for the application of state-of-the-art techniques
to real-world tasks. Special sessions will be devoted to the following
task areas:
\begin{itemize}
\item{Knowledge Acquisition}
\item{Inductive Logic Programming}
\item{Robotics and Autonomous Systems}
\item{Diagnosis and Design Applications}
\end{itemize}
Participants are invited to present their own applications and
problems through posters and short presentations to be organized
during the school. They are also encouraged to dynamically form panels
and discussion groups. Material as well as space will be plenty.
Finally a topical day on technology transfer will focus on the
problems, opportunities and the interplay between activities in
research and industrial communities active in the area of Machine
Learning.
\section{Intended audience}
ES2ML will be interesting for researchers, developers
and managers. The attendees will learn, not only about
specific techniques but also about the opportunities and
problems of applying these to, for example, knowledge
acquisition, robotics or design problems. ES2ML is
useful for managers that are considering to start their
own applications of machine learning. Courses provide
in-depth studies of applications. The school offers
ample opportunity for informal discussion with the best
people in Machine Learning. Finally ES2ML wants to
attract anyone who is interested in the transfer process
of advanced information technology from theory to
practice.
\section{Venue}
ES2ML will take place near Turnhout (Belgium) in the
Priory Corsendonk. This conference center, among the
best in Europe, is located in the midst of unique
natural surroundings. The quaint 14th century priory
offers an exquisite combination of ancient Flemish
tradition and modern comfort. It features beautifully
restored meeting rooms, outdoor sports facilities and
excellent cooking. Overnight accommodation is
excellent.
The Priory Corsendonk is within easy reach of major
cities like Brussels and Antwerp. On July 22nd and July
31th a shuttle service to Brussels National Airport will
be available. Detailed instructions about these travel
arrangements will be sent to the participants.
\section{Leisure and tourism}
In the afternoons several hours are basically kept free.
The Priory has a large garden and outdoor sports
facilities for tennis, swimming and biking. The
surroundings are excellent for horse-riding or jogging.
A visit to one of Belgian's famous breweries is being
planned. Guided tours, for example to Antwerp, Ghent or
Brugge will be organized on Saturday and Sunday morning
or upon request.
\section{Registration fees}
\begin{itemize}
\item{Regular registration}
\begin{itemize}
\item{Before June 1, 1991: 33.000Bfr}
\item{After June 1, 1991: 36.300Bfr}
\end{itemize}
\item{Student registration (certification required)}
\begin{itemize}
\item{Before June 1, 1991: 22.000Bfr}
\item{After June 1, 1991: 24.200Bfr}
\end{itemize}
\item[\ ]{(1US\$ is appr 34Bfr; 1ECU is appr 42Bfr)}
\end{itemize}
Members of ECCAI may apply for an {\em ECCAI Travel Award}. ES2ML will
provide support to the extend possible. For more information on grants
please contact the organizers.
Full payment of the registration fee is demanded upon
registration, even if you intend to follow only part
of the school. The registration fee includes free
selection of courses, presentations, practice sessions,
demonstrations and discussions as well as all relevant
course material. The registration fee also includes the
conference dinner and reception.
\section{Accommodation}
All participants and their company are invited to stay
in the Priory Corsendonk which can accommodate up to 113
persons. Preferences can be granted on a first come
first serve basis. The following are the full-board accomodation fee
for 9 days:
\begin{itemize}
\item{Monk's room single 33.700Bfr}
\item{Luxury single 42.300Bfr}
\item{Luxury double 31.000Bfr}
\item[\ ]{(1US\$ is appr 34Bfr; 1ECU is appr 42Bfr)}
\end{itemize}
The prices listed are for the duration of the school (9
nights, arriving on 22th) and include breakfast, lunch,
coffeebreaks and dinner. If you require a special diet
mention this upon registration.
Student budget accomodation is available at a close
distance from the Priory Corsendonk in "De Linde". Either
2 or 4 bed studios are available at 1475Bfr resp. 1280Bfr
per person per day including breakfast, lunch and
coffeebreaks. The distance to the Priory is around 5km.
You can rent a bike for 150Bfr per day.
Finally, participation without accomodation costs an additional 800Bfr
per day, including coffeebreaks and lunch. A dinner at the Priory
costs 1050Bfr.
All reservations must be accompanied by a deposit of
at least 12.000Bfr.
\section{Registration and further information}
ES2ML-91 is organized by Walter Van de Velde (B) and
Carl-Gustav Jansson (S). All mail concerning the summer
school, including registration and bookings for
accommodation and requests for information should be
sent to the organizers at the following address:
\begin{quote}
ES2ML-91\\
attn. Walter Van de Velde\\
Artificial Intelligence Laboratory\\
Vrije Universiteit Brussel\\
Pleinlaan 2, B-1050 Brussels, Belgium\\
Tel: (+32) 2 641 29 65 or 29 78\\
Fax: (+32) 2 641 28 70\\
Email: es2ml\@arti.vub.ac.be\\
\end{quote}
\newpage
\begin{verbatim}
ES2ML-91 REGISTRATION FORM
==========================
DELEGATE
Name ...............................
Institution ...............................
Department ...............................
Street ...............................
Town ...............................
Country ...............................
Tel ...............................
Fax ...............................
E-mail ...............................
COMPANION (shares room)
Name ...............................
Is Companion a delegate as well? Yes / No
If yes a separate registration form is required.
ACCOMMODATION
Type of room (0) Monk's room single
(0) Luxury single
(0) Luxury double
(0) In 4 bed studio
(0) In 2 bed studio
Arrival ...............................
Departure ...............................
Number of Nights ...............................
PAYMENT
Registration .................. Bfr
Indicate regular or student (certify)
Accommodation .................. Bfr
(min 12.000Bfr)
Non-delegate companion .................. Bfr
(min 12.000Bfr)
Total Advance Payment: .................. Bfr
Please indicate one of the following methods of payment:
(0) Banker's draft or Eurocheque in Belgian Franks.
The payment should be in the name of ES2ML-91
Cheque no. enclosed: .................
(0) Direct bank transfer (add 200Bfr) to ASLK-CGER bank
Address: PO Box 1436 B-1000 Brussels 1
Bank telex: 26860 or 61189
Bank SWIFT code: CGAK BE BB
Name of Account: ES2ML-91
Account number: 001-2262479-30
Please send us a copy of all relevant documents.
Date ...............................
Signature ...............................
With your signature you guarantee full payment of all
costs related to your participation before August 1, 1991.
\end{verbatim}
\newpage
\courseheader
{Programme Overview}
{Walter Van de Velde\\
Carl-Gustav Jansson}
{ES2ML chairmen}
The following is an overview of the programme of the European Summer
School on Machine Learning. The programme is tentative and the order
of the courses may be changed according to the availability of the
teachers.
\vskip .5cm
\stag{Monday 22}
{Framework for Machine Learning\\
Learning and Memory\\
Applied Machine Learning}
\stag{Tuesday 23}
{Synthetic Learning Approaches\\
Learning from Real-World Data\\
Case-Based Learning}
\stag{Wednesday 24}
{Case-Based Techniques\\
Genetic Algorithms\\
Applied Machine Learning}
\stag{Thursday 25}
{Frontier Problems in Induction\\
Learning for Modelling}
\stag{Friday 26}
{Selecting Appl's and Paradigms\\
ML, Science and Industry\\
Introduction to Neural Nets}
\stag{Saturday 27}
{Genetic Algorithms}
\stag{Sunday 28}
{Learning Theory\\
Inductive Logic Programming}
\stag{Monday 29}
{Computational Learning Theory\\
Analytical Learning Techniques\\
Inductive Logic Programming}
\stag{Tuesday 30}
{Integrated Architectures\\
Learning Apprentices\\
Selected Applications}
\stag{Wednesday 31}
{Toward Learning Robots\\
Challenging Machine Learning}
\newpage
\courseheader
{A Unifying Theoretical Framework \\ for Machine Learning
and Recent Research on \\
Induction, Abstraction and Multistrategy Learning}
{Ryszard S. Michalski}
{Center for Artificial Intelligence\\
George Mason University\\
Fairfax, VA}
\section*{Motivation and goals of the course}
In the view of a rapid expansion of machine learning research, there
is a strong interest in understanding the relationships among
different learning methods and paradigms, and determining the best
areas for their applicability. This course presents a unifying view of
machine learning research, analyzes various algorithms, and describes
a general classification of learning methods and research directions.
The course concentrates on synthetic (inductive) learning methods,
both empirical ones that need or use little background knowledge, and
constructive methods that strongly rely on the learner's background
knowledge.
The course explains basic principles behind inductive learning
algorithms, and analyzes their relative strengths and weaknesses from
the viewpoint of practical applications. Learning algorithms are
illustrated by a recently developed visualization technique that
enables one to visualize the learned and target concepts, and display
the effects of individual learning operations in the form of graphical
images.
the participants will be able to get a hand-on experience with several
learning programs by using a unique demonstration system, called
EMERALD. This system integrates such learning methods as learning
rules from examples, learning structural descriptions, conceptual
clustering, sequence prediction and quantitative discovery (an earlier
and simpler version of this system was demonstrated at major US
Museums of Science). For those interested in further studies of
specific topics, references to relevant literature are provided.
\section*{Course Outline}
\subsection*{A. Introduction}
\begin{itemize}
\item{Goals and the scope of research in machine learning}
\item{A brief history of machine learning research}
\end{itemize}
\subsection*{B. Toward Unifying Theory of Learning}
\begin{itemize}
\item{Inference-based theory of learning}
\item{Analysis of fundamental learning operations
(generallization vs. specialization; abstraction vs. concretion,
induction vs. deduction)}
\item{A multicriterion classification of learning processes}
\end{itemize}
\subsection*{C. Synthetic Learning Approaches}
\begin{itemize}
\item{Inductive paradigm and version space}
\item{Rules for inductive inference and abstraction}
\item{Algorithms for concept learning from examples}
\item{The STAR learning methodology and the AQ family of programs}
\item{Examples of results from implemented programs}
\end{itemize}
\subsection*{D. Self-Organization of Knowledge and Discovery}
\begin{itemize}
\item{Conceptual clustering}
\item{Discovery systems}
\item{Conceptual data analysis}
\item{Learning to predict}
\end{itemize}
\subsection*{E. Analysis and Comparison of Synthetic Learning Methods}
\begin{itemize}
\item{Criteria for comparison}
\item{Visualization of concept learning processes}
\item{Experimental comparison of learning paradigms}
\item{Discussion of appicability of different methods}
\end{itemize}
\subsection*{F. Frontier Problems and Recent Research}
\begin{itemize}
\item{Coping with uncertainty and plausible reasoning}
\item{Two-tiered concept representation}
\item{Learning imprecise and flexible concepts}
\item{Constructive induction and abstraction}
\item{Multistrategy task-adaptive learning}
\end{itemize}
\newpage
\courseheader
{Course on Analyical Learning}
{Tom Mitchell}
{School of Computer Science\\
Carnegie-Mellon University\\
Pittsburgh, PA}
\section*{Outline}
The problem of automatically generalizing from examples
lies at the heart of the learning problem. There are two broad approaches to
this problem: Inductive learning methods examine many training examples of the
target concept in order to extract features common to instances of the concept.
These features are used to form the general description of the learned concept.
In contrast, analytical approaches rely on prior knowledge of the learner
(rather than large numbers of training examples) to guide the process of
generalization. This lecture will present analytical approaches to
generalization. We first present a tutorial of basic algorithms, then
consider recent research to extend this approach to learning. Finally, we
present a case study illstrating practical issues in developing an analytical
learning method within a frame-based system (Theo).
\section*{Selected contents}
The course consists of the following parts:
\begin{itemize}
\item{Inductive and analytical generalization problems}
\item{Explanation-based learning}
\item{The EBG algorithm [Mitchell et al.]}
\begin{itemize}
\item{Learning to recognize objects (e.g., cups)}
\item{Learning control knowledge (e.g., search heuristics)}
\end{itemize}
\item{Knowledge compilation without training examples}
\begin{itemize}
\item{Partial evaluation, STATIC [Etzioni]}
\end{itemize}
\item{Combining results from multiple training examples}
\begin{itemize}
\item{Induction over explanations [Dietterich and Flann]}
\item{Induction over learned generalizations }
\item{Explaining inductively derived generalizations}
\end{itemize}
\item{Imperfect domain theories}
\begin{itemize}
\item{Generalizing from abstract domain theories}
\item{Using many examples to guide explanation [Bergadano and Giordana]}
\item{Lazy EBG for complex domain theories}
\end{itemize}
\item{Combining Explanation-based and neural net learning}
\begin{itemize}
\item{Propostional domain theories to initialize neural net [Shavlik]}
\item{Extending to first-order domain theories}
\end{itemize}
\item{Practical issues and a case study: implementing EBG in Theo}
\begin{itemize}
\item{Capturing and representing explanations}
\item{Expensive learned rules: repairing them, forgetting them}
\item{Deciding what is operational}
\item{When/What to learn}
\end{itemize}
\end{itemize}
\newpage
\courseheader
{Course in Case-Based Reasoning}
{Katia P. Sycara}
{School of Computer Science\\
Carnegie Mellon University\\
Pittsburgh, PA 15213}
\section*{Case-Based Reasoning}
The objective of the course is to present the mechanisms and techniques
underlying Case-Based Reasoning (CBR).
CBR is exploring questions, such as the organization
and indexing of case memory, that are at the heart of many
nagging AI difficulties, such as the knowledge acquisition
bottleneck and the brittleness of some expert systems.
Knowing how to reason with cases should help solve such
problems as well as complement already existing techniques.
The course will
explore both theoretical and practical issues. It will identify
advantages of CBR vis a vis other planning methods. It will characterize
application domains where CBR seems to be most promising. It will identify
bottleneck areas that hamper the efficiency of proposed algorithms, as
well as areas where more theoretical research needs to be done.
Case-Based Reasoning is the planning/problem solving paradigm where
prior situational experiences (cases) are retrieved from memory and
utilized in analysis and/or development of solutions to a current problem.
CBR requires a memory where past cases are organized. Successful cases are
stored so they can be retrieved and used in similar situations. Failures
are also stored so that they can warn the problem solver of potential
difficulties and provide repairs. At the end of problem solving, the case
memory is updated with the new problem solving experience. Thus, learning is
integrated with problem solving.
CBR is a new AI planning technique and
has recently received much attention from the AI community.
This is attested
by the success of the two DARPA-sponsored Case-Based Reasoning
Workshops in 1988 and 1989, DARPA sponsorship of several CBR projects,
and an increasing number of CBR-related theses and publications.
Various industrial research AI laboratories, such as those at GTE and TI,
are now supporting projects that utilize CBR.
CBR is potentially interesting to complex real-world applications
because of the following advantages:
\begin{itemize}
\item{Once a case-based reasoner has produced a complex plan, it can use it
in the future instead of planning from scratch each time. This is in
contrast to expert systems that have to re-derive their solutions.}
\item{A case-based reasoner can avoid past mistakes. When failure
occurs during planning, the features that are predictive of the
failure as well as any appropriate repairs are associated with the
case and stored in memory. When the same features are present in the
current problem, the planner gets reminded of the failure and can
retrieve the associated repair.}
\item{A case-based reasoner can deal with open worlds and situations
not easily formalizable. This happens because new plans are built from
old ones. But the internal effect of those plans need not be known or
analyzed by the planner. This is often observed in human planning
where a plan is tried "because it worked in the past" even though the
planner does not have a causal model of the situation.}
\item{A case-based reasoner can react fast in familiar circumstances.
Experience in building case-based systems has shown that case
retrieval can be made fast. If the case memory contains experiences
that are the same with (or very close to) the current problem, the
plan used successfully in the past is immediately available (no plan
generation process is necessary).}
\item{A case-based reasoner can learn from the cumulative experience
of others and across domains. Because cases modularize and encapsulate
knowledge, if a common representation is used, case memories can be
updated and CBR can proceed (accessing separate or combined case
memories) without the harmful rule interactions that plague expert
systems.}
\end{itemize}
The course consists of the following parts:
\begin{itemize}
\item{Overview of CBR}
\item{CBR Paradigms: Extended Examples}
\item{Characteristics of CBR Domains}
\item{Indexing and retrieval of cases}
\item{Adaptation of previous cases to solve the current problem}
\item{Failure avoidance and recovery}
\item{CBR as a Learning Paradigm}
\item{Integration of CBR with other problem solving paradigms}
\item{Extended CBR Bibliography}
\end{itemize}
The principles presented in each part will be {\em illustrated using
extended examples}. A survey of implemented CBR systems will also be
presented.
\section*{Selected Contents}
\subsection*{A. Overview of CBR}
\begin{itemize}
\item{{\bf Basic underlying ideas:}
The basic idea underlying CBR is plan re-use.
Planning episodes (the cases) are organized in a Case Knowledge Base where
they can be accessed via salient features, called indices. Successful cases
similar to the input case can be reused to arrive at a solution; failed cases
similar to the input case are used to warn the planner of potential
difficulties.}
\item{{\bf Case representation:} We will present criteria to determine what
knowledge must be included in a case and ways to represent it.}
\item{{\bf Case Memory:} To be effectively used, a case memory must conform to
requirements, such as allow for efficient case retrieval. We will discuss
various ways of organizing case bases.}
\item{{\bf Basic CBR algorithm (retrieve, compare, adapt, repair, generalize):}
The CBR process starts with the extraction of appropriate indices from the
input case. These indices are used to access potentially applicable cases
from the case memory. The retrieved cases are compared to the input and
important similarities and differences are noted. Selection of the most
appropriate case is done and adaptation of the precedent case is performed
to fit constraints and specifications of the current case. If the derived
solution fails, repair is performed. If it succeeds, memory is updated with a
successfully resolved new case. As the case base is augmented with new
cases, generalizations are performed.}
\item{{\bf Comparison of CBR and other planning paradigms:} We will compare and
contrast
features of CBR and other planning paradigms, such as expert systems,
model-based reasoning, and search. We will identify advantages and
disadvantages of CBR vis a vis the other paradigms and point out for which
type of tasks/domains each is most appropriate.}
\item{{\bf Survey of existing CBR systems:} The survey will include the name,
developer, implementation status, and task domain of the
surveyed systems.}
\end{itemize}
\subsection*{B. Indexing and retrieval of cases}
\begin{itemize}
\item{{\bf Indexing strategies:} Since it is inefficient to designate every feature
in a case as an index, various strategies are needed to allow the
identification of certain features as good indices. We will present (a)
criteria that good indices should have, (b) useful types of indices, and (c)
task characteristics to guide indexing.}
\item{{\bf Static vs. dynamic index selection:} Index specification can be
(a) static, i.e., known in advance to the system and not changing, and
(b) dynamic where new indices are created during problem solving. We
will present issues associated with each indexing method.}
\item{{\bf Memory organization for efficient case retrieval:} There are
many possible ways to organize the case memory, such as flat,
hierarchical, discrimination networks. We will present different
memory organization techniques and discuss advantages and
disadvantages associated with each.}
\item{{\bf Retrieval strategies:} Retrieval algorithms depend on the type
of memory organization employed. We will present various retrieval
algorithms suitable for different memory organizations.}
\item{{\bf Case comparison mechanisms:} Since it is very rare that the input case will
be exactly the same as a retrieved case, the system should have the ability
to make comparisons of partially-matched cases. We will present various
similarity metrics and evaluation algorithms to perform comparison of
retrieved cases to the input case.}
\end{itemize}
\subsection*{C. Adaptation of previous cases to solve the current problem}
\begin{itemize}
\item{{\bf Recognition of when adaptation is
appropriate:} A case based reasoner must be able to recognize when a
precedent case is different enough from the current one to merit
adaptation. Another important issue is choosing what part of the old
plan needs to be adapted. We will present various strategies for
making this choice.)
\item{{\bf Adaptation strategies:} We will present various adaptation
strategies, such as parameter adjustment, substitution, addition,
deletion, and refinement and the algorithms associated with their use.}
\end{itemize}
\subsection*{D. Failure avoidance and recovery}
\begin{itemize}
\item{{\bf Failure avoidance strategies:} CBR can warn a problem solver of
the potential failure of plans. This can be done by various
strategies, such as comparing feature of the input case to retrieved
failed cases, by evaluating a contemplated solution using a set of
criteria and by forming expectations with respect to the chances of
success of the solution. We will present various failure avoidance
strategies in the context of CBR.}
\item{{\bf Failure recognition:} It is not always possible for a problem
solver to avoid a failure. Hence, failure recovery techniques must
also be provided. The first step in failure recovery is failure
recognition. There are various ways to recognize failure (e.g., user
feedback, matching with expectations, searching the case base for
cases where the proposed solution has failed). Techniques for failure
recognition will be presented.}
\item{{\bf Blame assignment:} Once a failure has been recognized, it must
be explained. Retrieval of previous similar failures (among other
techniques) can provide blame assignment advice.}
\item{{\bf Failure repair techniques:} If repairs are stored as part of
previous cases, they can be re-used to fix similar failures. CBR can
be used recursively in the space of failures. Multiple cases can also
be used to generate repairs. Issues and techniques associated with
failure repair will be presented.}
\end{itemize}
\subsection*{E. CBR as a Learning Paradigm}
\begin{itemize}
\item{{\bf Learning by rote:} Integration of new cases
into the case memory enables a problem solver to learn by rote. What
exactly has been learned depends on the way new cases are integrated
into the case memory. We will present various memory updating
algorithms and discuss their advantages and disadvantages.}
\item{{\bf Success-driven learning:} Success-driven learning is the
process where besides the new case, the case memory update
incorporates generalizations that have been made at the end of a
problem solving session. Algorithms for success-driven learning will
be presented.}
\item{{\bf Failure-driven learning:} In the context of CBR failure-driven
learning involves the representation, indexing, and storage of
failures, their explanation and repairs. Various ways of addressing
these issues will be presented.}
\end{itemize}
\subsection*{F. Integration of CBR with other problem solving methods}
A case-based reasoner can address various issues during problem solving by
incorporating the use of other problem solving techniques, such as
rule-based reasoning and constraint propagation. Alternatively, CBR can be
used to perform various tasks in non-CBR problem solvers. This integration
increases the robustness and flexibility of the problem solver. We will
present various such types of integration, the tasks addressed by the
non-CBR techniques within the CBR context and vice versa, and issues
associated with realizing the integration. In this outline, we present a few
examples:
\begin{itemize}
\item{{\bf CBR and rule-based techniques:} cases can be used to
disambiguate the usage of terms in rules; on the other hand rules can
be used in adaptation and repair of case-based solutions.}
\item{{\bf CBR and constraint propagation:} cases can be used to assign
values to subsets of constraints; on the other hand, constraint
propagation can be used to propagate the consequences of various
adaptation decisions of case-based solutions.}
\item{{\bf CBR and qualitative simulation:} qualitative simulation can be
used for verification of the behavior of case-based solutions in
physical domains (e.g., design of mechanical devices).}
\item{{\bf CBR and decision theoretic techniques:} decision theoretic
techniques (e.g., utility theory) can be used to evaluate various
case-based solutions.}
\end{itemize}
\newpage
\courseheader
{Introductory course on Computational Learning Theory}
{Achim G. Hoffmann}
{Technische Universit\"at Berlin\\
Franklinstr. 28/29 \\
D-1000 Berlin 10, Germany}
\section*{Outline}
\begin{itemize}
\item Introduction to the basic notions.
\begin{itemize}
\item Fixed probability distribution on the set of objects:
Formal definition, examples, interpretation of probability as relative frequencies.
\item Short introduction to the theory of NP-completeness:
Combinatorial problems, NP-hard problems, approximation algorithms.
\item Notion of PAC-learning: Restricted number of examples for convergence, computational feasibility of finding an appropriate hypothesis.
\end{itemize}
\item Important results of learnable and non-learnable concept classes.
\begin{itemize}
\item Difference of hypotheses space and concept space: Example where a concept class is only learnable, if the used hypotheses space is a superset of the concept class.
\item Approximating target concepts: Finding hypotheses that are only consistent with a major fraction of the provided sample.
\item What does a learnability result mean:
Finding approximately consistent hypotheses in polynomial time.
What does it mean for an efficient implementation of the
version space~? How to interpret approximately consistent hypotheses
in the version space model~?
\end{itemize}
\item Basic methods for proving learnability and non-learnability.
\begin{itemize}
\item Using the VC-Dimension: Examples for determining the VC-dimension for certain concept classes.
\item Proving polynomial-time solvability: Determining the runtime of
algorithms that find hypotheses that are consistent with given samples.
\item Proving NP-hardness: Reductions from related problems to the problem under consideration.
\end{itemize}
\item Critique of the PAC-learning model.
\begin{itemize}
\item Some shortcomings of the notion of PAC-learning: Worst case analysis, equal a priori plausibility of concepts, randomly chosen learning examples.
\item Some ideas to overcome these shortcomings: Different probability distributions for the learning and the subsequent classification task.
\item Defending arguments for the original approach to PAC-learning by discussing
a major open problem of COLT, the DNF-learnability: Problem of finding approximately consistent hypotheses, fixed probability distribution required for
approximations.
\end{itemize}
\item Application to Neural Networks
\begin{itemize}
\item Basic results on required examples: How many exapmles are necessary for training a network with 100 nodes~?
\item Basic results on the computational complexity of training neural networks: NP-hardness results for finding appropriate weight functions for the nodes in a network.
\item What mean NP-hardness results in massively parallel computer architectures~?
\item What mean NP-hardness results in massively parallel computer architectures for local learning algorithms~?
\end{itemize}
\item Concluding Remarks and Discussion
\begin{itemize}
\item
The benefits of computational learning theory for mainstream machine learning.
\item
What kind of results from future theoretical investigations would be useful for
practically oriented machine learning approaches.
\end{itemize}
\item Optional practice session
\begin{itemize}
\item Excercises for estimating the VC-dimension for the concept classes
that underlie given learning tasks.
\item Excercises for applying known learnability results to given
learning tasks.
\item Excercises for proving the learnability/non-learnability of
given learning tasks (perhaps as a derivation of known results).
\end{itemize}
\end{itemize}
\newpage
\courseheader
{Course in Induction of Decision Trees}
{Ivan Bratko}
{Institut Jozef Stefan\\
Jamova 39\\
61000 LJUBLANA\\
Jugoslavia}
\section*{Induction of Decision Trees}
Top-down induction of decision trees (TDIDT) is a wel established
technique for machine learning, often referred to as ID3 after
Quinlan's early implementation of this technique. This approach to
machine learning, in which the learned description is represented in
the form of a decision tree, is well inderstood and has been
implemented in commercial systems. It has been applied to many
practical problems in industry, medicine, financial decision making
etc. It is now regarded by many as a practical knowledge-acquisition
technique for expert systems. The main practical strength of TDIDT
lies in its simplicity, efficiency, and existence of techniques for
handling unreliable, incomplete or noisy data. Its main limitations
come from restrictions in the representation language and liminted
ways of using background knowledge in learning.
\section*{Selected contents}
The course consists of the following parts:
\begin{itemize}
\item{General statement of the TDIDT problem and basic tree induction
agorithm}
\item{Refinements to the basic algorithm regarding the choice among
available attributes and stopping tree expansion}
\item{Criteria for evaluating attributes, details of some evaluation
measures}
\item{Handling incomplete data}
\item{Learning from noisy data, effects of pruning decision trees}
\item{Forward pruning and post pruning, details of some pruning
techniques}
\item{The problem of estimating probabilities; effects of poor
estimates, how to estimate better}
\item{Conversion of decision trees into if-then rules}
\item{Examples of applications of TDIDT}
\item{Limitations of TDIDT, how can they be overcome}
\end{itemize}
\newpage
\courseheader
{Genetic Algorithms as Problem Solvers}
{Yuval Davidor}
{Department of Applied Mathematics and Computer Science\\
Weizmann Institute of Science\\
Rehovot 76100, Israel}
\section*{Outline}
A genetic algorithm is an adaptive search procedure based on
simplified adaptive mechanisms of natural systems. In combining the
adaptive effect of natural selection with the search in breadth of
sexual reproduction, genetic algorithm offer a robust search mechanism
for an optimal or near optimal solution in complex domains. Genetic
algorithms belong to the family of probabilistic heuristic algorithms,
but in contrast to traditional optimization procedures, they do not
require a priori knowledge about the structure of the domain. When
applying a genetic algorithm to combinatorial problems, function
optimization, or learning, the problem is viewed as a black box whose
state space is searched efficiently to locate hyperplanes
corresponding to high performance.
Genetic algorithms have been applied to a broad spectrum of
applications. From bridge and turbine blade design, through classical
combinatorial and game theory, to machine learning aspects such as
sub-goal reward and classifier systems.
The material covered in this lecture series is
interdisciplinary in nature. It combines topics from population
genetics, control theory, aspects of computer science, and machine
learning. It does this in an intuitive way, attempting to provide
first the understanding, second the knowledge how to use, and third
the theory of these systems. It is with this intention and in this
spirit that this course is presented - to attempt to provide a clear
introduction to the workings of GAs in the context of optimization of
large, complex and redundant systems.
\newpage
\courseheader
{Inductive Logic Programming}
{Stephen Muggleton\\
(hands-on instructor: Ashwin Srinivasan)}
{The Turing Institute\\
Glasgow, Scotland, UK}
\section*{Inductive Logic Programming}
Inductive Logic Programming (ILP) is an emerging research area spawned
by Machine Learning and Logic Programming. While the influence of
Logic Programming has encouraged the development of strong theoretical
foundations, the new area is inheriting its experimental orientation
from Machine Learning.
This course will have 5 parts:
\begin{enumerate}
\item Introduction to logic
\item Theoretical frameworks for ILP
\item ILP Implementations
\item Experiments and applications
\item Hands-on course
\end{enumerate}
\section*{Selected contents}
\subsection* {1. Introduction to logic}
Logic programs are defined as sets of definite clauses
in first-order predicate calculus. The
Prolog language provides an efficient interpreter for
logic programs. The basic mechanism for interpreting
logic programs is a theorem prover which uses Robinson's
resolution mechanism for all basic deductive inferences.
Resolution is in turn based on unification of terms.
Students will be introduced to the ideas of models,
interpretations and logical entailment. Logical
entailment will be used to describe the notion of
generality. It will be shown how resolution can be
used to decide the entailment relationship.
\subsection*{2. Theoretical frameworks for ILP}
ILP systems learn logic programs from examples and
background knowledge. Early theoretical frameworks for
this form of learning were developed by Plotkin and later
Shapiro. Muggleton and Buntine showed that resolution
could be inverted to make a general purpose learning mechanism.
Subsequently several other researchers have investigated the
properties of inverse resolution. Researchers have also been
studying the problem of what to do when the set of background predicates
is insufficient for constructing correct hypotheses that
agree with the given examples. In this case we need to
find a way of inventing new predicates. A general theoretical
framework will be described which includes that of Plotkin
as well as Muggleton and Buntine.
Hypothesis formation must be followed by
sufficient confirmation to allow for acceptance of the hypothesis.
A confirmation technique based on information compression will
be presented. It will be shown how this technique can be used
for predicate invention, distinguishing noise and deciding
on non-monotonic behaviour.
\subsection*{3. ILP Implementations}
In implementing ILP systems it has been found to be necessary
to constrain the learning process in various ways in order
to make learning tractable and efficient. Constraints have
taken the form of limitations on the hypothesis language,
the target theorem prover and the forms of
background knowledge. In addition a variety of domain-
dependent constraints are often used. These include
typing, modes, argument symmetry and predicate functionality.
Since many ILP systems are now in existence an overview
of selected systems will be given.
\subsection*{4. ILP experiments and applications}
Inductive logic programming is being applied in various areas
including primary-secondary prediction of protein folding,
discovery of structure activity relations for drug design,
automatic design of finite element meshes applied to
CAD/CAM, automatic construction of diagnostic models
for satellite subsystems, construction of dynamic control
models, electrical circuit design and automatic design
of mission critical software for network management systems.
Clearly the use of first-order logic representations has
opened a large number of problem areas previous inaccessible
to early machine learning methods.
Some experimental results already show that ILP systems
can do better than other machine learning techniques in
hard problem areas.
\subsection*{5. Hands-on course}
This will have two parts.
\begin{itemize}
\item[a.]{Substitutions and least-general generalisations.\\
Students will be asked to write Prolog
programs for constituent parts of a learning
program.}
\item[b.]{Golem exercises. \\
Students will use a state-of-the-art
ILP system to learn given predicates.}
\end{itemize}
\newpage
\courseheader
{Machine Learning and model-based expert systems}
{Ivan Bratko}
{Institut Jozef Stefan\\
Jamova 39\\
61000 LJUBLANA\\
Jugoslavia}
\section*{Machine Learning and model-based expert systems}
A second generation approach to expert systems involveds deep
knowledge, or a model, and exploits machine learning techniques for
acquiring and transforming between various knowledge representations
that are suitable for specific tasks (such as diagnosis or
prediction). A methodology for knowedge acquisition, introduced by the
KARDIO study, relies on a combination of these techniques whereby the
following stages complete a "knowledge-acquisition cycle":
\begin{enumerate}
\item{construct a deep model of the problem domain by consulting
experts and literature (model contains all {\em first principles}, but
may be inefficient}
\item{compile deep model, by means of deduction, into a shallow level
representation (that may be time-efficient, but large and hard to
understand as a whole)}
\item{compress shallow representation, by means of induction (using
machine learning techniques) into representations that are economical
both with respect to time and space}
\item{compare compressed representations with the original,
human-expert encodings of the same knowledge, looking to refine either
the deep model or human own expertise}
\end{enumerate}
Although KARDIO's domain is medicine, the same knoweldge-acquisition
cycle has been straightforwardly applied to the development of
industrial expert systems. In this lecture, technical details of
various aspects of this methodology will be discussed.
The course consists of the following parts:
\begin{itemize}
\item{representing deep knowedge by symbolic descriptions in predicate
logic; the role of qualitative models}
\item{synthesising compressed, task-specific descriptions employing
machine learning techniques}
\item{learning deep models from observations in problem domains;
learning models of static and dynamic systems}
\item{machine learning as a means for synthesising new, human-type
knowledge}
\end{itemize}
\newpage
\courseheader
{Integrated Architectures and Learning Apprentices}
{Tom Mitchell}
{School of Computer Science
Carnegie-Mellon University\\
Pittsburgh, PA}
\section*{Outline}
This lecture considers the question of how to incorporate methods for
generalizing from examples into larger problem solving systems so that
they improve their performance with experience. In order to develop
such systems, we must address questions beyond the central question of
how a program can generalize from examples. We must also answer
questions such as what information should be learned, how learned
knowledge should be organized to scale up to large stores of learned
knowledge, when the system should decide to invoke its learning
methods, how it will obtain its training data, etc. In this lecture
we consider three recent approaches to the problem of building
integrating problem solving and learning systems.
\section*{Selected contents}
The course consists of the following parts:
\begin{itemize}
\item{Knowledge-based integrated architectures: frameworks, or "shells" for
building knowledge-based systems with embedded learning capabilities.}
\begin{itemize}
\item{Issues}
\item{Example systems:}
\begin{itemize}
\item{PRODIGY [Carbonell]}
\item{SOAR [Newell]}
\item{THEO [Mitchell]}
\end{itemize}
\item{Comparison of methods for representation, inference, generalization,
memory indexing, self-reflection, ...}
\end{itemize}
\item{Reinforcement learning architectures: frameworks for constructing
agents with sensor/effectors, which learn reward-seeking behavior within
their environment.}
\begin{itemize}
\item{Temporal difference learning}
\item{Adaptive Heuristic Critic [Sutton]}
\item{Q-learning [Watkins]}
\item{Neural net reinforcement learning for automomous agents [Lin]}
\end{itemize}
\item{Learning apprentices: knowledge-based consultants that learn from
their users throughout their life-cycle.}
\begin{itemize}
\item{Learning apprentices as an approach to knowledge base development,
maintenance, and customization}
\item{An example: A learning apprentice for calendar scheduling [Jourdan et al.]}
\end{itemize}
\end{itemize}
\newpage
\courseheader
{Applied Machine Learning}
{David Wilkins}
{Beckman Institute\\
Illinois}
\section*{Applied Machine Learning}
This course is based in a forthcomming readings in applied Machine
Learning editted by Bruce Buchanan and David Wilkins.
(outline not yet available)
\end{document}
------------------------------
END of ML-LIST 3.10