Copy Link
Add to Bookmark
Report
Machine Learning List Vol. 2 No. 20
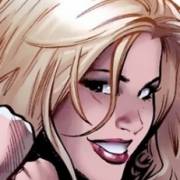
Machine Learning List: Vol. 2 No. 20
Tuesday, Oct 9, 1990
Contents:
ML91 deadline extended
m-of-n learning
NIPS*90 WORKSHOP PRELIMINARY PROGRAM
The Machine Learning List is moderated. Contributions should be relevant to
the scientific study of machine learning. Mail contributions to ml@ics.uci.edu.
Mail requests to be added or deleted to ml-request@ics.uci.edu. Back issues
may be FTP'd from ics.uci.edu in /usr2/spool/ftp/pub/ml-list/V<X>/<N> or N.Z
where X and N are the volume and number of the issue; ID & password: anonymous
------------------------------
Date: Tue, 9 Oct 90 13:43:30 CDT
From: Lawrence Birnbaum <birnbaum@fido.ils.nwu.edu>
Subject: ML91 deadline extended
ML91 -- THE EIGHTH INTERNATIONAL WORKSHOP ON MACHINE LEARNING
DEADLINE FOR WORKSHOP PROPOSALS EXTENDED
To make life a little easier, the deadline for workshop proposals for ML91
has been extended by a few days. The new deadline is MONDAY, OCTOBER 15.
Please send proposals by email to:
ml91@ils.nwu.edu
or by hardcopy to the following address:
ML91
Northwestern University
The Institute for the Learning Sciences
1890 Maple Avenue
Evanston, IL 60201 USA
fax (708) 491-5258
Please include the following information:
1. Workshop topic
2. Names, addresses, and positions of workshop committee members
3. Brief description of topic
4. Workshop format
5. Justification for workshop, including assessment of breadth of
appeal
On behalf of the organizing committee,
Larry Birnbaum
Gregg Collins
Program co-chairs, ML91
------------------------------
Date: Mon, 1 Oct 90 10:55:49 -0400 (EDT)
From: Steven Ritter <sr0o+@andrew.cmu.edu>
Subject: n-of-m-learning
I'm sorry if my first comment caused some confusion. It was meant to be
a pointer to the Pitt and Valiant paper, not an explanation of
learnability in their sense. The reason I labelled the result as
possibly "too theoretical" is that Pazzini's original request
acknowledged that n-of-m learning can be done with a perceptron, so a
proof of "non-learnability" clearly comes with some strong restrictions.
Pitt's comment clarified the situation well: n-of-m concepts are not PAC
learnable, if we search through the space of n-of-m concepts.
Perceptrons search a space much larger than that of n-of-m concepts.
That is, they can represent n-of-m concepts, but they can also represent
lots of other concepts. Thus, the proof does not apply to them.
Nevertheless, I think the non-learnability proofs do provide some help
in designing learning algorithms. Even if we knew that the entire world
was composed of n-of-m concepts, we could do better than to develop a
system that can represent only n-of-m concepts. We might think that a
good start on an efficient algorithm would be to search the smallest
possible hypothesis space. N-of-m learning is a good example of where
that strategy doesn't work. In this case, I'm not aware of anyone who
has tried to develop an algorithm that searches in the space of n-of-m
concepts, but the strategy of trying to find the smallest hypothesis
space to search does seem like a natural one (and one to avoid) for more
complex concepts.
------------------------------
[The following message is exerpted from NEURON-DIGEST]
Subject: NIPS*90 WORKSHOP PRELIMINARY PROGRAM
From: jose@learning.siemens.com (Steve Hanson)
Date: Wed, 26 Sep 90 15:17:02 -0400
NIPS*90 WORKSHOP PRELIMINARY PROGRAM
____________________________________________________________
! POST CONFERENCE WORKSHOPS AT KEYSTONE !
! THURSDAY, NOVEMBER 29 - SATURDAY, DECEMBER 2, 1990 !
!____________________________________________________________!
I am pleased to send you a preliminary description of workshops to
be held during our annual "NIPS Post-conference Workshops". Among the
many workshop proposals we have received we believe to have selected a
program of central topics that we hope will cover most of your
interests and concerns. As you know from previous years, our NIPS
Post-conference Workshops are an opportunity for scientists actively
working in the field to gather in an informal setting and to discuss
current issues in Neural Information Processing.
The Post-conference workshops will meet in Keystone, right after the
IEEE conference on Neural Information Processing Systems, on November
30 and December 1. You should be receiving an advance program, travel
information and registration forms for both NIPS and the
Post-conference workshops. Please use these forms to register for
both events. Please also indicate which of the workshop topics below
you may be most interested in attending. Your preferences are in no
way binding or limiting you to any particular workshop but will help
us in allocating suitable meeting rooms and minimizing overlap between
workshop sessions. Please mark your three most prefered workshops
(1,2 and 3) on the corresponding form in your registration package.
I look forward to seeing you soon at NIPS and its Post-conference
Workshops. Please don't hesitate to contact me with any questions you
may have about the workshops in general (phone: 412-268-7676, email
at: waibel@cs.cmu.edu.). Should you like to discuss a specific
workshop, please also feel free to contact the individual workshop
leaders listed below.
Sincerely yours,
Alex Waibel
NIPS Workshop Program Chairman
School of Computer Science
Carnegie Mellon University
Pittsburgh, PA 15217
----------------------------------------------------------------------
Thursday, November 29, 1990
5:00 PM: Registration and Reception at Keystone
Friday, November 30, 1990
7:30 - 9:30 AM: Small Group Workshops
4:30 - 6:30 PM: Small Group Workshops
7:30 - 10:30 PM: Banquet and Plenary Discussion
Saturday, December 1, 1990
7:30 - 9:30 AM: Small Group Workshops
4:30 - 6:30 PM: Small Group Workshops
6:30 - 7:15 PM: Plenary Discussion, Summaries
7:30 - 11:00 PM: Fondue Dinner, MountainTop Restaurant
----------------------------------------------------------------------
NIPS '90 WORKSHOP DESCRIPTIONS
Workshop Program Coordinator: ALEX WAIBEL
Carnegie Mellon University
phone: 412-268-7676
E-mail: waibel@cs.cmu.edu
1. OSCILLATIONS IN CORTICAL SYSTEMS
Ernst Niebur
Computation and Neural Systems
Caltech 216-76
Pasadena, CA 91125
Phone: (818) 356-6885
Bitnet: ernst@caltech
Internet: ernst@aurel.cns.caltech.edu
2. BIOLOGICAL SONAR
Herbert L. Roitblat
Department of Psychology
University of Hawaii
2430 Campus Road
Honolulu, HI 96822
Phone: (808) 956-6727
E-mail: herbert@uh.cc.uk.uhcc.hawaii.edu
Patrick W. B. Moore & Paul E. Nachtigall
Naval Ocean Systems Center
Hawaii Laboratory
P. O. Box 997
Kailua, Hawaii, 96734
Phone: (808) 257-5256 & (808) 257-1648
E-mail: pmoor@nosc.mil & nachtig@nosc.mil
3. NETWORK DYNAMICS
Richard Rohwer
Centre for Speech Technology Research
Edinburgh University
80, South Bridge
Edinburgh EH1 1HN
Scotland
Phone: (44 or 0) (31) 225-8883 x261
E-mail: rr%ed.eusip@nsfnet-relay.ac.uk
4. CONSTRUCTIVE AND DESTRUCTIVE LEARNING ALGORITHMS
Scott E. Fahlman
School of Computer Science
Carnegie-Mellon University
Pittsburgh, PA 15213
Phone: (412) 268-257
Internet: fahlman@cs.cmu.edu
Most existing neural network learning algorithms work by adjusting
connection weights in a fixed network. Recently we have seen the
emergence of new learning algorithms that alter the network's topology
as they learn. Some of these algorithms start with excess connections
and remove any that are not needed; others start with a sparse network
and add hidden units as needed, sometimes in multiple layers. The
user is relieved of the burden of guessing in advance what network
topology will best fit a given problem. In addition, many of these
algorithms claim improved learning speed and generalization.
In this workshop we will review what is known about the relationship
between network topology, expressive power, learning speed, and
generalization. Then we will examine a number of constructive and
destructive algorithms, attempting to identify the strengths and
weaknesses of each. Finally, we will look at open questions and
possible future developments.
5. COMPARISONS BETWEEN NEURAL NETWORKS AND DECISION TREES
Lorien Y. Pratt
Computer Science Department
Rutgers University
New Brunswick, NJ 08903
Phone: (201) 932-4634
E-mail: pratt@paul.rutgers.edu
Steven W. Norton
Siemens Corporate Research, Inc.
755 College Road East
Princeton, NJ 08540
Phone: (609) 734-3365
E-mail: nortonD @learning.siemens.com
The fields of Neural Networks and Machine Learning have evolved
separately in many ways. However, close examination of multilayer
perceptron learning algorithms (such as Back-Propagation) and decision
tree induction methods (such as ID3 and CART) reveals that there is
considerable convergence between these subfields. They address
similar problem classes (inductive classifier learning) and can be
characterized by a common representational formalism of hyperplane
decision regions. Furthermore, topical subjects within both fields
are related, from minimal trees and brain-damaged nets to incremental
learning.
In this workshop, invited speakers from the Neural Network and
Machine Learning communities (including Les Atlas and Tom Dietterich)
will discuss their empirical and theoretical comparisons of the two
areas. In a discussion period, we'll then compare and contrast them
along the dimensions of representation, learning, and performance
algorithms. We'll debate the ``strong convergence hypothesis'' that
these two research areas are really studying the same problem.
6. GENETIC ALGORITHMS
David Ackley
MRE-2B324
Bellcore
445, South St.
Morristown, NJ 07962-1910
Phone: (201) 829-5216
E-mail: ackley@bellcore.com
"Genetic algorithms" are optimization and adaptation techniques that
employ an evolving population of candidate solutions. "Recombination
operators" exchange information between individuals, creating a global
search strategy quite different from --- and in some ways
complementary to --- the gradient-based techniques popular in neural
network learning. The first segment of this workshop will survey the
theory and practice of genetic algorithms, and then focus on the
growing body of research efforts that combine genetic algorithms and
neural networks. Depending on the participants' interests and
backgrounds, possible discussion topics range from "So what's all
this, then?" to "How should networks best be represented as genes?" to
"Is the increased schema disruption inherent in uniform crossover a
feature or a bug?"
As natural neurons provide inspiration for artificial neural
networks, and natural selection provides inspiration for genetic
algorithms, other aspects of natural life can provide useful
inspirations for studies in "artificial life". In artificial worlds
simulated on computers, experiments can be performed whose natural
world analogues would be inconvenient or impossible for reasons of
duration, expense, danger, observability, or ethics. Interactions
between genetic evolution and neural learning can be studied over many
hundreds of generations. The consensual development of simple,
need-based lexicons among tribes of artificial beings can be observed.
The second segment of this workshop will survey several such "alife"
research projects. A discussion of prospects and problems for this
new, interdisciplinary area will close the workshop.
7. IMPLEMENTATIONS OF NEURAL NETWORKS ON DIGITAL, MASSIVELY
PARALLEL COMPUTERS
S. Y. Kung and K. Wojtek Przytula
Hughes Research Laboratories, RL69
3011 Malibu Canyon Road
Malibu, California 90265
Phone: (213) 317-5892
E-mail: wojtek@csfvax.hac.com
8. VLSI NEURAL NETWORKS
Jim Burr
Starlab Stanford University
Stanford, CA 94305
Phone: (415) 723-4087 (office)
(415) 725-0480 (lab)
(415) 574-4655 (home)
E-mail: burr@mojave.standford.edu
9. OPTICAL NEURAL NETWORKS
Kristina Johnson
University of Colorado, Boulder
Campus Box 425
Boulder, CO 80309
Phone: (303) 492-1835
E-mail: kris%fred@boulder.colorado.edu
kris@boulder.colorado.edu
10. NEURAL NETWORKS IN MUSIC
Samir I. Sayegh
Department of Physics
Purdue University
Fort Wayne, IN 46805-1499
Phone: (219) 481-6157
E-mail: sayegh@ed.ecn.purdue.edu
11. SPEECH RECOGNITION
Nelson Morgan and John Bridle
International Computer Science Institute
1947 Center Street, Suite 600
Berkeley, CA 94704
Phone: (415) 643-9153
E-mail: morgan@icsib4.berkeley.edu
12. NATURAL LANGUAGE PROCESSING
Robert Allen
MRE-2A367
Bellcore
445, South St.
Morristown, NJ 07962-1910
Phone: (201) 829-4315
E-mail: rba@flash.bellcore.comp
13. HAND-PRINTED CHARACTER RECOGNITION
Gale Martin & Jay Pittman,
MCC, 3500 Balcones Center Drive, Austin, Texas 78759
Phone: (512) 338-3334, 338-3363,
E-mail: galem@mcc.com, pittman@mcc.com
14. NN PROCESSING TECHNIQUES TO REAL WORLD MACHINE VISION PROBLEMS
Murali M. Menon & Paul J. Kolodzy
MIT Lincoln Laboratory
Lexington, MA 02173-9108
Phone: (617) 863-5500
E-mail: Menon@LL.LL.MIT.EDU
Kolodzy@LL.LL.MIT.EDU
15. INTEGRATION OF ARTIFICIAL NEURAL NETWORKS WITH EXISTING
TECHNOLOGIES EXPERT SYSTEMS AND DATABASE MANAGEMENT SYSTEMS
Veena Ambardar
15251 Kelbrook Dr
Houston, TX 77062
Phone: (713) 663-2264
E-mail: veena@shell.uucp
16. BIOPHYSICS
J. J. Atick
Institute for Advanced Study
Princeton, NY 08540
Phone: 609-734-8000
B. Bialek
Department of Physiology
University of California at Berkeley
Berkeley, CA 94720
E-mail: bialek@candide.berkeley.edu
17. ASSOCIATIVE LEARNING RULES
David Willshaw
University of Edinburgh
Centre for Cognitive Science
2 Buccleuch Place
Edinburgh EH8 9LW
Phone: 031 667 1011 ext 6252
E-mail: David@uk.ac.ed.cns
<Abstract Unavailable>
18. RATIONALES FOR, AND TRADEOFFS AMONG VARIOUS NODE FUNCTION SETS
J. Stephen Judd
Siemens Corporate Research, Inc.
755 College Road East
Princeton, NJ 08540
Phone: (609) 734-6500
E-mail: Judd@learning.siemens.com
Linear threshold functions and sigmoid functions have become very
standard node functions for our neural network studies, but the
reasons for using them are not very well founded. Are there other
types of functions that might be more justifiable? or work better? or
make learning more tractable? This workshop will explore various
issues that might help answer such questions. Come hear the experts
tell us what matters and what doesn't matter; then tell the experts
what *really* matters.
------------------------------
END of ML-LIST 2.20