Copy Link
Add to Bookmark
Report
AIList Digest Volume 8 Issue 126
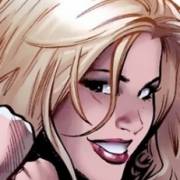
AIList Digest Tuesday, 15 Nov 1988 Volume 8 : Issue 126
Queries:
Classifier Systems
NEXPERT OBJECT experiences
Help finding a reference
Sample expert systems
Backward or forward chaining ES
Applied AI and CAM (1 response)
Seth R. Goldman's e-mail address
ES in Electric Power Distribution
Smalltalk opinions
Learning arbitrary transfer functions (2 responses)
----------------------------------------------------------------------
Date: 2 Nov 88 23:48:00 GMT
From: mailrus!caen.engin.umich.edu!brian@ohio-state.arpa (Brian
Holtz)
Subject: Classifier Systems
Does anyone know of any references that describe classifier systems whose
messages are composed of digits that may take more than two values?
For instance, I want to use a genetic algorithm to train a classifier
system to induce lexical gender rules in Latin. Has any work been done
on managing the complexity of going beyond binary-coded messages, or
(better yet) encoding characters in messages in a useful, non-ASCIIish way?
I will summarize and post any responses.
------------------------------
Date: 3-NOV-1988 13:55:24
From: CDTPJB%CR83.NORTH-STAFFS.AC.UK@MITVMA.MIT.EDU
Subject: NEXPERT OBJECT experiences
I am forwarding this query on behalf of one of my research students, Anton
Grashion.
"I am currently engaged in a research project which combines causal geological
models with stochastic mathematical ones. We are proposing to use NEXPERT
OBJECT as a front/back-end to our present system written in 'C'. I would be
grateful if anyone who has experience of Nexpert to let me know of their
opinions/complaints/plaudits regarding its use."
Many Thanks
Phil Bradley. | Post: Dept. of Computing
JANET: cdtpjb@uk.ac.nsp.cr83 | Staffordshire Polytechnic
DARPA: cdtpjb%cr83.nsp.ac.uk@cunyvm.cuny.edu | Blackheath Lane
Phone: +44 785 53511 | Stafford, UK
| ST18 0AD
------------------------------
Date: 7 Nov 88 05:28:49 GMT
From: pollux.usc.edu!burke@oberon.usc.edu (Sean Burke)
Subject: need help finding a reference
Dear Netland Folks,
I've got a reference which I've had trouble locating in libraries and
publisher's catalogs, presumably because I've got some or all of it wrong.
If anyone can fill in the missing parts of this puzzle, I would be grateful.
The book is
Title: "Knowledge Aquisition for Rule-Based Systems"
Editor: Sandra L Marcus
Publisher: Kluwer Academic Publishing
If you recognize this work, please email me the correct details.
Thanx,
Sean Burke
------------------------------
Date: 7 November 1988 23:15:53 CST
From: U23405 at UICVM (Michael J. Steiner )
Subject: Sample expert systems...
I am trying to learn about expert systems, and I have found PILES of
literature/articles about expert system theory, but few actual sample
programs that I could pick apart (not literally) to learn about expert
systems. If anyone has any examples of expert systems (forward- or
backward-chaining), could you possibly send me a copy to fool around with?
The program should preferably be in C, although PASCAL, BASIC, FORTRAN,
LISP, and PROLOG would be acceptable also. (If anyone sends a program in
BASIC, I promise not to ruin his reputation by telling everyone :-))
Send all replies to:-----------------+ Michael Steiner
+------>> Email: U23405@UICVM.BITNET
------------------------------
Date: 8 Nov 88 05:26:00 GMT
From: osu-cis!killer!texbell!merch!cpe!hal6000!tdpvax!miker@ohio-state
.arpa
Subject: backward or forward expert
I am investigating the building of an expert system as a
diagnostic tool (for a production software/hardware system).
>From the literature I have read, it appears that a backward-chaining
expert shell would be the best method. Have I come to the wrong
conclusion? Is it feasable to use a forward-chaining inference
engine?
------------------------------
Date: 11 Nov 88 09:45:40 GMT
From: martin@prodix.liu.se (Martin Wendel)
Subject: Applied AI and CAM
I am doing a project on applied AI and CAM.
It concerns building an AI planning system for automating
NC-Lathe operations planning. My goal is that the system
will be competent enough to support unmanned manufacturing
on our NC-Lathe.
I would be most grateful if You could send references
concerning this area of AI. If anyone is working on a
similar project mail me.
P.S. Does anyone know the mail-adress to Caroline Hayes
at Carnegie Mellon.
------------------------------
Date: 13 Nov 88 05:13:52 GMT
From: nau@mimsy.umd.edu (Dana S. Nau)
Subject: Re: Applied AI and CAM
In article <76@prodix.liu.se> martin@prodix.liu.se (Martin Wendel) writes:
>I am doing a project on ... building an AI planning system for automating
>NC-Lathe operations planning. ...
>I would be most grateful if You could send references
>concerning this area of AI.
I and my students have done lots of research in this area. A few of the
published papers are listed below.
D. S. Nau, ``Automated Process Planning Using Hierarchical Abstraction,''
{\em TI Technical Journal}, Winter 1987, pp. 39-46. Award winner, Texas
Instruments 1987 Call for Papers on AI for Industrial Automation.
D. S. Nau and M. Gray, ``Hierarchical Knowledge Clustering: A Way to
Represent and Use Problem-Solving Knowledge,'' J. Hendler, ed., {\em Expert
Systems: The User Interface}, Ablex, Norwood, NJ, 1987, pp. 81-98.
D. S. Nau, R. Karinthi, G. Vanecek, and Q. Yang,
``Integrating AI and Solid Modeling for Design and Process Planning,''
{\em Second IFIP Working Group 5.2 Workshop on Intelligent CAD},
University of Cambridge, Cambridge, UK, Sept. 1988.
--
Dana S. Nau
Computer Science Dept. ARPA & CSNet: nau@mimsy.umd.edu
University of Maryland UUCP: ...!{allegra,uunet}!mimsy!nau
College Park, MD 20742 Telephone: (301) 454-7932
------------------------------
Date: 9 Nov 88 09:35:06 GMT
From: andreas@kuling.UUCP (Andreas Hamfelt)
Reply-to: andreas@kuling.UUCP (Andreas Hamfelt)
Subject: Seth R. Goldman's e-mail address
Does anyone know the e-mail address to Seth R. Goldman at UCLA?
------------------------------
Date: Wed, 09 Nov 88 14:45:14 EST
From: <ganguly@ATHENA.MIT.EDU>
Subject: ES in Electric Power Distribution
I am posting this notice for getting information on existing expert
systems in the area of operation and maintainance of electric power
distribution networks (state wide). Any information would be highly
appreciated.
Thanks in advance,
Jaideep Ganguly
ganguly@athena.mit.edu
------------------------------
Date: Thu, 10 Nov 88 08:35:30 EST
From: "Bruce E. Nevin" <bnevin@cch.bbn.com>
Subject: Smalltalk opinions
Offline, 'cause this is old news to most I'm sure, could you send me
pros, cons, shrugs about:
Smalltalk as an OO programming environment.
Application area is network management w/ graphical
interface, a desideratum is providing a shell for
supporting visual design input from nonprogrammers
manipulating icons and widgets more or less directly.
Specifically, the PC implementation of Smalltalk
from Digitalk Inc.
Send replies to:
bn@bbn.com
Bruce Nevin
If this is an inappropriate forum for this query, please let the
lack of response indicate that. Thanks
------------------------------
Date: 10 Nov 88 18:54:52 GMT
From: mailrus!uflorida!haven!uvaarpa!uvaee!aam9n@ohio-state.arpa
(Ali Minai)
Subject: Learning arbitrary transfer functions
I am looking for any references that might deal with the following
problem:
y = f(x); f(x) is nonlinear in x
Training Data = {(x1, y1), (x2, y2), ...... , (xn, yn)}
Can the network now produce ym given xm, even if it has never seen the
pair before?
That is, given a set of input/output pairs for a nonlinear function, can a
multi-layer neural network be trained to induce the transfer function
by being shown the data? What are the network requirements? What
are the limitations, if any? Are there theoretical bounds on
the order, degree or complexity of learnable functions for networks
of a given type?
Note that I am speaking here of *continuous* functions, not discrete-valued
ones, so there is no immediate congruence with classification. Any attempt
to "discretize" or "digitize" the function leads to problems because the
resolution then becomes a factor, leading to misclassification unless
the discretizing scheme was chosen initially with careful knowledge
of the functions characteristics, which defeats the whole purpose. It
seems to me that in order to induce the function correctly, the network
must be shown real values, rather than some binary-coded version (e.g.
in terms of basis vectors). Also, given that neurons have a logistic
transfer function, is there a theoretical limit on what kinds of functions
*can* be induced by collections of such neurons?
All references, pointers, comments, advice, admonitions are welcome.
Thanks in advance,
Ali
Ali Minai
Dept. of Electrical Engg.
Thornton Hall
University of Virginia
Charlottesville, VA 22901
aam9n@uvaee.ee.Virginia.EDU
aam9n@maxwell.acc.Virginia.EDU
------------------------------
Date: 11 Nov 88 16:33:46 GMT
From: bbn.com!aboulang@bbn.com (Albert Boulanger)
Subject: Re: Learning arbitrary transfer functions
Check out the following report:
"Nonlinear Signal Processing Using Neural Networks:
Prediction and System Modelling"
Alan Lapedes and Robert Farber
Los Alamos Tech report LA-UR-87-2662
There was also a description of this work at the last Denver
conference on Neural Networks. Lapedes has a nice demonstration of
recovering the logistic map given a chaotic time series of the map. He
has also done this with the Macky-Glass time-delay equation.
It is rumored that techniques like this (Doyne Farmer as well as James
Crutchfield have non neural-based dynamical-systems techniques for
doing this, cf "Equations of Motion From a Data Series, James
Crutchfield & Bruce McNamera, Complex Systems, Vol #3, June 1987,
417-452.) are being used by companies to predict the stock market.
Albert Boulanger
BBN Systems & Technologies Corp.
10 Moulton St.
Cambridge MA, 02138
aboulanger@bbn.com
------------------------------
Date: 11 Nov 88 22:21:10 GMT
From: mailrus!umich!itivax!dhw@ohio-state.arpa (David H. West)
Subject: Re: Learning arbitrary transfer functions
In a previous article, Ali Minai writes:
>
>I am looking for any references that might deal with the following
>problem:
>
>y = f(x); f(x) is nonlinear in x
>
>Training Data = {(x1, y1), (x2, y2), ...... , (xn, yn)}
>
>Can the network now produce ym given xm, even if it has never seen the
>pair before?
>
>That is, given a set of input/output pairs for a nonlinear function, can a
>multi-layer neural network be trained to induce the transfer function
^^^
An infinite number of transfer functions are compatible with any
finite data set. If you really prefer some of them to others, this
information needs to be available in computable form to the
algorithm that chooses a function. If you don't care too much, you
can make an arbitrary choice (and live with the result); you might
for example use the (unique) Lagrange interpolation polynomial of
order n-1 that passes through your data points, simply because it's
easy to find in reference books, and familiar enough not to surprise
anyone. It happens to be easier to compute without a neural network,
though :-)
-David West dhw%iti@umix.cc.umich.edu
{uunet,rutgers,ames}!umix!itivax!dhw
CDSL, Industrial Technology Institute, PO Box 1485,
Ann Arbor, MI 48106
------------------------------
End of AIList Digest
********************