Copy Link
Add to Bookmark
Report
AIList Digest Volume 8 Issue 025
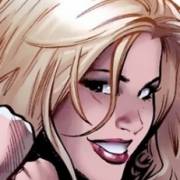
AIList Digest Tuesday, 26 Jul 1988 Volume 8 : Issue 25
Today's Topics:
Philosophy:
thesis + antithesis = synthesis
Turing machines and brains (long)
What can we learn from computers
Metaepistemology and unknowability
----------------------------------------------------------------------
Date: Fri, 22 Jul 88 17:37 O
From: <YLIKOSKI%FINFUN.BITNET@MITVMA.MIT.EDU>
Subject: thesis + antithesis = synthesis
Distribution-File:
AILIST@AI.AI.MIT.EDU
In AIList Digest V8 #9, sme@doc.ic.ac.uk (Steve M Easterbrook)
writes:
>In a previous article, YLIKOSKI@FINFUN.BITNET writes:
>>> "In my opinion, getting a language for expressing general
>>> commonsense knowledge for inclusion in a general database is the key
>>> problem of generality in AI."
>>...
>>Here follows an example where commonsense knowledge plays its part. A
>>human parses the sentence
>>
>>"Christine put the candle onto the wooden table, lit a match and lit
>>it."
>>
>> ... LOTS of stuff by me deleted ...
>>
>>Therefore, Christine lit the candle, not the table."
>
>Aren't you overcomplicating it a wee bit? My brain would simply tell me
>that in my experience, candles are burnt much more often than tables.
>QED.
There could be some kind of default reasoning going on.
Candles are burnt, not tables, unless there is something out of the
ordinary about the situation. Christine is an ordinary person and the
situation is ordinary, therefore the candle was lit.
--- Andy
"When someone comes to you and shows you are wrong, don't start an
argument, try to create a synthesis."
------------------------------
Date: Fri, 22 Jul 88 16:39 EST
From: steven horst 219-289-9067
<GKMARH%IRISHMVS.BITNET@MITVMA.MIT.EDU>
Subject: Turing machines and brains (long)
I don't recall how the discussion of Turing machines and their
suitability for "imitating" brains started in recent editions of
the Digest, but perhaps the following considerations may prove
useful to someone.
I think that you can go a long way towards answering the question
of whether Turing machines can "imitate" brains or minds by rephrasing
the question in ways that make the right sorts of distinctions.
There have been a number of attempts to provide more perspicuous
terminology for modeling/simulation/duplication of mental
processes, brains -- or for that matter any other sorts of objects,
systems or processes. (I'll use "system" as a general term for things
one might want to model.) Unfortunately, none of these attempts
at supplying a technical usage has become standard. (Though personally
I am partial to the one Martin Ringle uses in his anthology of
articles on AI.)
The GENERAL question, however, seems to be something like this:
WHAT FEATURES CAN COMPUTERS (or programs, or computers running programs,
it really doesn't matter from the perspective of functional
description) HAVE IN COMMON WITH OTHER OBJECTS, SYSTEMS AND PROCESSES?
Of course some of these properties are not relevant to projects of
modeling, simulation or creation of intelligent artifacts. (The fact
that human brains and Apple Macintoshes running AI programs both exist
on the planet Earth, for example, is of little theoretical interest.)
A second class of similarities (and differences) may or may not be
relevant, depending on one's own research interests. The fact that
you cannot build a universal Turing machine (because it would require
an infinite storage tape) is irrelevant to the mathematician, but
for anyone interested in fast simulations, it is important to have
a machine that is able to do the relevant processing quickly and
efficiently. So it might be the case that a Turing machine could
run a simulation of some brain process that was suitably accurate,
but intolerably slow.
But the really interesting questions (from the philosophical
standpoint) are about (1) what it is, in general, for a program to
count as a model of some system S, and (2) what kinds of features
Turing machines (or other computers) and brains can share. And I
think that the general answer to these questions goes something like
this: what a successful model M has in common with the system S of
which it is a model is an abstract (mathematical) form. What, after
all, does one do in modeling? One examines a system S, and attempts
to analyze it into constituent parts and rules that govern the
interactions of those parts. One then develops a program and data
structures in such a manner that every unit in the system modeled
has a corresponding unit in the model, and the state changes of
the system modeled are "tracked" by the state changes of the model.
The ideal of modeling is isomorphism between the model and the
system modeled. But of course models are never that exact, because
the very process of abstraction that yields a general theory
requires one to treat some factors as "given" or "constant" which
are not negligible in the real world.
With respect to brains and Turing machines, it might be helpful
to ask questions in the following order:
(1) What brain phenomena are you interested in studying?
(2) Can these phenomena be described by lawlike generalizations?
(3) Can a Turing machine function in a manner truly isomorphic
to the way the brain phenomenon functions? (I take it that
for many real world processes, such ideal modeling cannot be
accomplished, because to but it crudely, most of reality
is not digital.)
(4) Can a Turing machine function in a manner close enough to being
isomorphic with the way the brain processes function so as to
useful for your research project? (Whatever it may be....)
(5) Just what is the relationship between corresponding parts of
the model and the system modeled? Most notably, is functional
isomorphism the only relevant similarity beween model and
system modeled, or do they have some stronger relationships
as well- e.g., do they respond to the same kinds of input
and produce the same kinds of output?
What is interesting from the brain theorist's point of view, I should
think, is the abstract description that program and brain process
share. The computer is just a convenient way of trying to get at that
and testing it. Of course some people think that minds or brains
share features with (digital) computers at some lower level as well,
but that question is best kept separate from the questions about
modeling and simulation.
Sorry this has been so long. I hope it proves relevant to
somebody's interests.
Steven Horst
BITNET address....gkmarh@irishmvs
SURFACE MAIL......Department of Philosophy
Notre Dame, IN 46556
------------------------------
Date: Sun, 24 Jul 88 11:06:43 -0200
From: Oded Maler <oded%WISDOM.BITNET@CUNYVM.CUNY.EDU>
Subject: What can we learn from computers
What Can We Learn From Computers and Mathematics?
=================================================
A few digests ago Gilbert Cockton raised the above rhetoric question as
part of an attack on the computationally-oriented methodologies of AI
research. As a side remark let us recall that many AIers see their main
question as "What can we teach computers?" or "What are the limits to
the application of Mathematics to real-world modeling?"
Although I consider a lot of Cockton's criticism as valid, his claim
about the non-existent contribution of Mathematics and Computers to the
understanding of intelligence is at least as narrow-minded as the
practice of those AI researchers who ignore the relevance of Psychology,
Sociology, Philosophy and disciplines alike.
I claim that experience with computers, machines, formal systems, in
all levels, (starting from typing, programming and hacking, through
computer science and up to pure mathematics) can teach a person (and a
scientist) a lot, and may build relevant intuitions, metaphors and
perspectives. Some of it cannot be gained through a life-long traditional
humanistic scholarship.
Just imagine what a self-aware person can learn from using a
word-processor by introspecting his own performance: Context-sensitive
interpretation of symbols, learning by analogy (when you move from one
WP to another), reversible and unreversible operations, eye-brain-hand
coordination. I'm sure Mr. Cockton's thoughts have benefited from such
an experience, so think of those who were using or designing such devices
for some decades.
And what about programming? Algorithms, nested loops, procedures and
hierarchical organization, stacks and recursive calls, data-structures
in general, input and output, buffers, parameter passing and communication,
efficiency of programs, top-down refinement, and the most important
experience: debugging. No time before in history had so many people and
so often been involved in the process of making theories (about the
behavior of programs), watching them being refuted, fixing them and
testing again. It is very easy to criticize the simplistic incorporation
of such paradigms into models for human thinking, as many hackers and
so-called AI researchers often do, but to think that these metaphors are
useless and irrelevant to the study of intelligence is just making an
ideology out of one's ignorance.
And let's proceed to theoretical computer science: the limits of
what is computable by certain machines, the results from
complexity theory about what can be performed in principle and yet
is practically impossible, the paradigm of a finite-state machine
with input, output and internal states, mathematical logic and its
shortcomings, the theory and practice of databases, the research
in distributed systems, the formal treatment of knowledge and belief:
is none of these relevant to the humanities?
Not the mention the mathematical ideas concerning sets, infinity,
order relations, distance functions, convergence, algebraic structures,
the foundations of probability, dynamical systems, games and strategies
and many many others.
Mr. Cockton was implicitly concerned with the following question:
"What is the best selection of formal and informal experience, a
scientist must have during his/her (hopefully ongoing) development, in
order to contribute to the advance of the cognitive sciences?" Excluding
experiences of the above kind from the candidate list is not what I
expect from adherents of scholar tradition. Every scientist grows
within some sub-sub-discipline, learns its methodologies, tricks, success
criteria and its most influential bibliographic references. When we
turn later to inter-disciplinary areas, we cannot go back to the kindergarten,
and start learning a new discipline as undergraduates do. We must learn
to discover those parts of other disciplines that are essential and
relevant to our object of study. Because of our inherent limitations
(complexity..) we are doomed to neglect and ignore a lot of work done
within other disciplines and even within our own. C'est la vie.
I agree with Mr. Cockton that by reading some AI work one gets the impression
that history begun just around the production of the paper: no references
to prior work and to past philosophical and psychological treatments of
the same issues. But going the other extreme, by adopting the scholar
(and sometimes almost snobbish) tradition to the modern cognitive sciences
is ridiculous too. Does a physicist or a chemist need to give references to
pre-Newtonian works, to astrology or alchemistry? (I'm not speaking of
the historian or philosopher of science).
I got the impression that Mr. Cockton views informaticians and
mathematicians in a rather stereotyped way: technocrats, misantropes that
can only deal with machines, persons that want to put the lively world
into their cold formulae and programs, individuals who are insensitive
to the arts and to human-human interaction. All this might be partially
true with respect to a certain fraction, but to generalize to the whole
community is like saying that humanists are nothing but a bunch of guys,
incapable of clear and systematic thinking, who use the richness and
ambiguity of natural language in order to hide their either self-evident
or vacuouss and meaningless ideas. I don't want to continue this
local-patriotic type of propaganda, but one can fill several screens
with similar deficiencies of the current traditions in the humanities.
The applicability of such descriptions to any fraction of the ongoing
work in the humanities, still does not justify a claim such as "What
Can We Learn from (say) Sociology?".
To conclude, I think that a good cognitive scientist can learn A LOT
from mathematics and computers. A humanist may still do important
work in spite of his mathematical ignorance, but I suspect that
in some fields this will become harder and harder.
Oded Maler
Dept. of Applied Mathematics
Weizmann Institute
Rehovot 76100
Israel
oded@wisdom.bitnet
------------------------------
Date: 25 Jul 88 04:26:25 GMT
From: steve@comp.vuw.ac.nz (Steve Cassidy)
Reply-to: steve@comp.vuw.ac.nz (Steve Cassidy)
Subject: Re: metaepistemology and unknowability
In a previous article YLIKOSKI@FINFUN.BITNET (Andy Ylikoski) writes:
>I would say that the actual world is unknowable to us because we have
>only descriptions of it, and not any kind of absolutely correct,
>totally reliable information involving it.
This seems like a totally useless definition of Knowing; what have you
gained by saying that I do not *know* about chairs because I only have
representations of them. This seems to be a problem people have in
making definitions of concepts in cognition.
Dan Dennet tries, in Brainstorms, to provide a useful definition of
something like what we mean by "intelligence". To avoid the problems of
emotional attatchment to words he uses the less emotive "intentionality". He
develops a definition that could be useful in deciding how to make systems
act like intelligent actors by restricting that definition to accurate
concepts. (As yet I don't claim to understand what he means, but I think I
get his drift.)
Now, we can argue whether Dennet's 'intentionality' corresponds to
'intelligence' if we like, but what will it gain us? It depends on what your
goals as an AI researcher are. I'm interested in building models of cognitive
processes - in particular, reading - my premise in doing this is that
cognitive processes can be modelled computationally, and that by building
computational models we can learn some more about the real processes. I am not
interested in whether, at the end of the day I have an intelligent system, a
simulation of an intelligent system or just a dumb computer program. I will
judge my performance on results -- does it behave in a similar way to humans,
if so my model, and the theory it is based upon, is good.
Is there anyone out there who's work will be judged good or bad depending on
whether it can be ascribed `intelligence'? It seems to me that it is only
useful to make definitions to some end, rather than for the sake of making
definitions; we are, after all, Applied Epistomologists and not
Philosophers (:-)
Steve Cassidy domain: steve@comp.vuw.ac.nz|
Victoria University, PO Box 600, -------------------------------------|
Wellington, New Zealand path: ...seismo!uunet!vuwcomp!steve|
"If God had meant us to be perfect, He would have made us that way"
- Winston Niles Roomford III
------------------------------
End of AIList Digest
********************