Copy Link
Add to Bookmark
Report
AIList Digest Volume 7 Issue 035
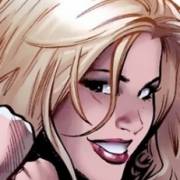
AIList Digest Thursday, 16 Jun 1988 Volume 7 : Issue 35
Today's Topics:
Queries:
Connectionist Expert Systems
Seminar:
Supercomputing Summer Institute
Philosophy:
Biological relevance and AI
Human-human communication
----------------------------------------------------------------------
Date: 14 Jun 88 18:22:56 GMT
From: ndsuvax!ncthangi@uunet.uu.net (sam r. thangiah)
Subject: Connectionist Expert Systems
>From: olivier@boulder.Colorado.EDU (Olivier Brousse)
>Could any one give me pointers about NESTOR, an expert system combining
>neural nets and symbolic AI techniques ?
>Is there any other work done in connectionist expert systems ?
I am also interested in the above area. Pointers to any references, papers
or work being conducted using such techniques will be much appreciated.
Thanks in advance,
Sam
--
Sam R. Thangiah, North Dakota State University.
UUCP: ...!uunet!ndsuvax!ncthangi
BITNET: ncthangi@ndsuvax.bitnet
ARPA,CSNET: ncthangi%ndsuvax.bitnet@cunyvm.cuny.edu
------------------------------
Date: Wed, 15 Jun 88 11:09:55 EDT
From: hendler@dormouse.cs.umd.edu (Jim Hendler)
Subject: Re: Connectionist expert systems
There are essentially two strains of work going on in this area. One
strain involves the actual implementation of expert-system-like programs
using connectionist techniques. I'll let the real connectionists
comment on those. The second strain involves the creation of hybrid
systems which have both connectionist and symbolic mechanisms
cooperating in the solution of traditional cognitivish AI problems (i.e.
language, planning, ``high level'' vision, etc.) As well as my own work
in this area, there is the work of Wendy Lehnert of UMass, Michael Dyer
of UCLA, Mark Jones of Bell Labs, and, depending on how you classify it,
the work of Dave Touretzky at CMU (Dave's work doesn't really have
symbols in the traditional sense of the word, but some of his models
use gating and other serial techniques to control a larger connectionist
system). Also, the work of the local (now often called structured)
connectionists have in some ways been hybrid. The one most related
might be that of Lokendra Shastri of U Penn.
-Jim Hendler
U. of Md. Institute for Advanced Computer Studies
UMCP
College Park, Md. 20742
------------------------------
Date: Wed, 15 Jun 88 10:18:09 EDT
From: Una Smith <Q2813@pucc.princeton.edu>
Subject: Supercomputing Summer Institute
John von Neumann National Supercomputer Center
1988 Summer Institute
"An Intensive Introduction to Vector and Parallel Supercomputing"
August 1-12, 1988
Objectives
The John von Neumann National Supercomputer Center (JvNC) will
hold its third annual Summer Institute during August 1-12, 1988
at the JvNC in Princeton, New Jersey. The principal goals of
the Institute are to teach the participants how to use
supercomputers effectively and to provide an intensive
mini-course in computational scientific research.
Facilities
The JvNC operates the first production Class VII supercomputer,
the ETA 10, installed at the JvNC in March, 1988. The ETA 10
is presently configured with 128 million words of shared memory
and four Central Processing Units (CPUs) each equipped with
four million words of local memory. The JvNC also operates two
CYBER 205 supercomputers, configured with four million words
of memory. All supercomputers are accesssed from the DEC 8600
front-end computers at the JvNC operating under VMS or Ultrix
(UNIX). The PEP software, a series of user-friendly, command
driven interactive procedures, allows users to execute programs
on the ETA 10 and CYBER 205 without the need for learning
the supercomputer's operating system. The JvNC Visualization
Facility includes two Silicon Graphics Iris 4D workstations
and two Sun 3/160 workstations with presentation and draft
quality color hardcopy.
Operation
The Summer Institute will be equally divided between the
classroom and the laboratory. The classroom portion will
include lectures by JvNC staff on the overall JvNC computer
environment, vector computing, parallel computing, advanced
performance programming, communications and networking, and
graphics. In addition, there will be invited lectures on
computational chemistry, algorithms for parallel machines,
computational fluid dynamics, plasma physics, and
visualization. The laboratory portion will include detailed
consulting by User Services on program optimization for the ETA
10 and CYBER 205, and utilization of the JvNC Visualization
Facility. The participants shall be awarded an allocation of
supercomputer time for their research projects.
Participants
Postdoctoral, graduate and advanced undergraduate students at
U.S. universities and colleges are eligible to apply for the
Summer Institute. Knowledge of Fortran is a prerequisite.
Experience with vectorization and supercomputers is not
required. Each participant is expected to be engaged in a
computational research project and bring an operational Fortran
code for further development and production runs during the
Institute.
Financial Support
The JvNC will provide reimbursement for travel, accommodations
and meals up to $1500 per participant according to NSF
policies. Air travel shall be economy class and local
accommodations will be organized through the JvNC. Meals will
be reimbursed up to a maximum per diem. There is no separate
stipend.
Application
Interested persons should submit the following information:
- Curriculum vitae, indicating university or college
affiliation, courses of study, undergraduate and graduate
transcript (as appropriate), address and telephone (office
and home)
- Two letters of recommendation from faculty advisors or
instructors
- Description of computational research project
The information should be submitted by 24 June 1988. All
applications should be sent to:
John von Neumann National Supercomputer Center
Summer Institute
P.O. Box 3717
Princeton, NJ 08543
For further information, contact David Salzman at 609/520-2000 or
<salzman@jvnca.csc.org> or <SALZMAN@JVNCD.BITNET>
------------------------------
Date: 14 Jun 88 20:57:06 GMT
From: tektronix!sequent!mntgfx!msellers@bloom-beacon.mit.edu (Mike
Sellers)
Subject: Biological relevance and AI (was Re: Who else isn't a
science?)
In article <13100@shemp.CS.UCLA.EDU>, Benjamin Thompson writes:
>In article <10510@agate.BERKELEY.EDU> weemba@garnet.berkeley.edu writes:
>> Gerald Edelman, for example, has compared AI with Aristotelian
>> dentistry: lots of theorizing, but no attempt to actually compare
>> models with the real world. AI grabs onto the neural net paradigm,
>> say, and then never bothers to check if what is done with neural
>> nets has anything to do with actual brains.
Where are you getting your information regarding AI & the neural net paradigm?
I agree that there is a lot of hype right now about connectionist/neural nets,
but this alone does not invalidate them (they may not be a panacea, but they
probably aren't worthless either). There are an increasing number of people
interested in (and to some degree knowledgeable of) both the artificial and
biological sides of sensation, perception, cognition, and (some day)
intelligence. See for example the PDP books or Carver Mead's upcoming book
on analog VLSI and neural systems (I just finished a class in this -- whew!).
There have been recent murmurings from some of the more classical AI types
(e.g. Seymour Papert in last winter's Daedalus) that the biological
paradigm/metaphor is not viable for AI research, but these seem to me to be
either overstating the case against connectionism or simply not aware
of what is being done. Others contend that anything involving 'wetware' is
not *really* AI at all, and thus shouldn't invade discussions on that subject.
This is, I believe, a remarkably short-sighted view that amounts to denying
the possibility of a new tool to use.
> This is symptomatic of a common fallacy. Why should the way our brains
> work be the only way "brains" can work? Why shouldn't *A*I workers look
> at weird and wonderful models? We (basically) don't know anything about
> how the brain really works anyway, so who can really tell if what they're
> doing corresponds to (some part of) the brain?
>
> Ben
I think Ben's second and following sentences here are symptomatic of a common
fallacy, or more precisely of common misinformation and ignorance. No one has
said or implied that biological nervous systems have a monopoly on viable
methodologies for sensation, perception, and/or cognition. There probably are
many different ways in which these types of problems can be tackled. We do
have a considerable amount of knowledge about the human brain, and (for
the time being more to the point) about invertebrate nervous systems and the
actions of individual neurons. And finally, correspondence to biological
systems, while important, is by no means a single and easily acheived goal
(see below). On the other hand, we can say at least two things about the
current state of implemented cognition:
1) The methods we now call 'classical' AI, starting from about the late
1950's or early 60's, have not made an appreciable dent in their original
plans nor even lived up to their original claims. To refresh your memory,
a quote from 1958:
"...there are now in the world machines that think, that learn and
that create. Moreover, their ability to do these things is going to
increase rapidly until --in a visible future-- the range of problems
they can handle will be coextensive with the range to which the human
mind has been applied."
This quote is from H. Simon and A. Newell in "Heuristic Problem Solving:
The Next Advance in Operations Research" in _Operations Research_ vol 6,
published in *1958*. (It was recently quoted by Dreyfus and Dreyfus in the
Winter 1988 edition of Daedalus, on page 19.) We seem to be no closer to
the realization of this claim than we were thirty years ago.
2) We do have one instance that proves that sensation, perception, and
cognition are possible: natural nervous systems. Thus, even though there
may be other ways of solving the problems associated with vision, for
example, it would seem that adopting some of the same strategies used by
other successful systems would increase the likelyhood of our success.
While it is true that there is more unknown than known about nervous
systems, we do know enough about neurons, synapses, and small aggregates
of neurons to begin to simulate their structure and function.
The issue of how much to simulate is a valid and interesting one. Natural
nervous systems have had many millions of years to evolve their solutions
(much longer than we hope to have to take with our artificial systems), but
then they have been both undirected in their evolution and constrained by
the resources and techniques available to biological systems. This would
seem to argue for only limited biological relevance to artificial solutions:
e.g., where neurons have axons, we can simply use wires. On the other hand,
natural systems also have the tendency to take a liability and make it into
a virtue. For example, while axons are not simple 'wires', and in fact are
much slower, larger, and more complex than wires, they can also act as active
parts of the whole system, enabling such things as temporal differentiation
to occur easily and without much in the way of cellular overhead. Thus,
while we probably will not want to create fully detailed simulations of
neurons, synapses, and neural structures, we do need to understand what
advantages are embodied in the natural approach and extract them for use in
our artifices while carefully excluding those things that exist only by
being carried along with the main force of the evolutionary current.
All of this is not to say that AI researchers shouldn't look at "weird and
wonderful models" of perception and cognition; this is after all precisely
what they have been doing for the past thirty years. The only assertion here
is that this approach has not yielded much in the way of fertile results
(beyond the notable products such as rule-based systems, windowed displays,
and the mouse :-) ), and that with new technology, new knowledge of biological
systems, and a new generation of researchers, the one proven method for
acheiving real-time sensation, perception, and cognition ought to be given
its chance to fail.
Responses welcomed.
--
Mike Sellers ...!tektronix!sequent!mntgfx!msellers
Mentor Graphics Corp., EPAD msellers@mntgfx.MENTOR.COM
"AI is simply the process of taking that which is meaningful, and making it
meaningless." -- Tom Dietterich (admittedly, taken out of context)
------------------------------
Date: Wed, 15 Jun 88 09:20:07 pdt
From: Ray Allis <ray@BOEING.COM>
Subject: Re: Human-human communication
In AIList Digest V7 #31, Stephen Smoliar writes:
> First of all, NO dance notation provides sufficient information for the
> exact reproduction of a movement.
Likewise there's not sufficient information in an English description
of "red" to impart knowledge to a listener.
> Ultimately, I tend to agree with Gilbert that the problem is not in the
> notation but in what is trying to be communicated. Video is as valuable
> in reconstructing dances as it is in gymnastics, but there is still no
> substitute for "shaping" bodies. What Gilbert calls "memory positions"
> I have always called "muscular memory;" and I'm afraid there is no substitute
> for physical experience when it comes to acquiring it.
Your experience with dance notation is illustrative of a characteristic
of languages in general, and a seriously flawed assumption in "AI".
"Natural" language *evokes* experience in a listener; language can't
*impart* experience. No amount of English description will produce the
experience of "red" in a congenitally blind person, or a computer, or
produce the same quality of associations with "flame" and "danger" and
"hot" and "blushing" that a sighted person can hardly avoid.
In order for a computer (read digital computer) to "understand" human
language, it must have *experience* which the language can evoke. "Data
structures" won't do, because they are symbols themselves, not experience.
In iconic languages, (e.g. the dance notations you mention) there is a
small amount of information conveyed because the perception of the icon
itself is an experience. Seeing a picture of a platypus is similar to
seeing a platypus. Reading or listening to a description of a platypus
is not. Hearing "cerulean" described in English conveys no information,
and any "understanding" on the part of the receiver must be *created*
from that receiver's experience.
It is this line of thought which led me several years ago to discard the
Physical Symbol System Hypothesis. Physical symbol systems are *not*
sufficient to explain or reproduce human thought and behavior. They are
formal systems (form-al: concerning form, eliminating content). The
PSSH is, however, a useful guide for most of what is called "AI", which is
the mechanization of formal logic, an engineering task and
properly a part of computer science. That task has nothing to do with the
creation of intelligence. I can certainly understand the irritation
of the engineers at people who want to re-think such a job after it's
started.
------------------------------
End of AIList Digest
********************