Copy Link
Add to Bookmark
Report
AIList Digest Volume 5 Issue 185
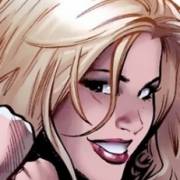
AIList Digest Monday, 20 Jul 1987 Volume 5 : Issue 185
Today's Topics:
Perception - Seeing-Eye Robots,
Philosophy - Searchability in Humans vs. Machines
----------------------------------------------------------------------
Date: 16 Jul 87 17:54:49 GMT
From: ihnp4!homxb!houdi!marty1@ucbvax.Berkeley.EDU (M.BRILLIANT)
Subject: Seeing-Eye robots
Suppose one wanted to build a robot that does what a Seeing-Eye dog
does (that is, helping a blind person to get around), but communicates
in the blind person's own language instead of by pushing and pulling.
Clearly this robot does not have to imitate a human being. But it does
have to recognize objects and associate them with the names that humans
use for them. It also has to interpret certain situations in its
owner's terms: for instance, walking in one direction leads to danger,
and walking in another direction leads to the goal.
What problems will have to be solved to build such a robot? Will its
hypothetical designers have to deal with the problem of mere
recognition, or the deeper problem of grounding symbols in meaning?
Could it be built by hardwiring sensors to a top-down symbolic
processor, or would it require a hybrid processor?
M. B. Brilliant Marty
AT&T-BL HO 3D-520 (201)-949-1858
Holmdel, NJ 07733 ihnp4!houdi!marty1
------------------------------
Date: 17 Jul 87 19:31:00 GMT
From: ihnp4!inuxc!iuvax!merrill@ucbvax.Berkeley.EDU
Subject: Re: Seeing-Eye robots
In comp.ai, marty1@houdi (M.B. Brilliant) writes:
> Suppose one wanted to build a robot that does what a Seeing-Eye dog
> does (that is, helping a blind person to get around), but communicates
> in the blind person's own language instead of by pushing and pulling.
> [Commentary on some of the essential properties of the robot.]
> What problems will have to be solved to build such a robot? Will its
> hypothetical designers have to deal with the problem of mere
> recognition, or the deeper problem of grounding symbols in meaning?
> Could it be built by hardwiring sensors to a top-down symbolic
> processor, or would it require a hybrid processor?
I seriously doubt that recognition itself would be adequate. As
Brilliant observes, one of the functions that the robot must perform
is the detection of "danger to its master." Consider the problem of
crossing a street. Is it enough to recognize cars (and trucks, and
motorcycles, and other already--known objects?) No.
The robodog has to generalize beyond simply cars and trucks and
busses, since their shapes change, to "things travelling along this
stretch of road {and what's a stretch of road?} which are a) moving
{and what does it mean to move?} b) fast {and what is fast? Why,
fast enough to be dangerous...which begs the question} c) in this
direction." At this point, I think that we have exceeded the bounds
of recognition and entered a realm where "judgement" is required, but,
if not, I imagine that I can probably extend this situation to meet most
specific objections. (I assume that the blind woman needs to cross roads
without undue delay. Traffic lights don't eliminate these problems,
since the robodog must "recognize" drivers who are turning, some of
whow would be safe, since they're either stopped or slow--moving, but some
of whom (at least, here in Bloomington) would run *any* pedestrian
down. !-))
BTW: I like this example very much. It raises quite nicely the
underlying issue in the symbol grounding problem discussion without
using the terminology that many of the readers of comp.ai seem to have
objected to. Congratulations, Mr. Brilliant!
John Merrill
merrill@iuvax.cs.indiana.edu UUCP:seismo!iuvax!merrill
Dept. of Comp. Sci.
Lindley Hall 101
Indiana University
Bloomington, Ind. 47405
------------------------------
Date: 14 Jul 87 21:21:33 GMT
From: berke@locus.ucla.edu
Subject: An Unsearchable Problem of Elementary Human Behavior
An Unsearchable Problem of Elementary Human Behavior
Peter Berke
UCLA Computer Science Department
The Artificial Intelligence assumption that all human behavior
can eventually be mimicked by computer behavior has been stated
in various ways. Since Newell stated his Problem Space
Hypothesis in 1980, it has taken on a clearer, and thus, more
refutable form. Newell stated his hypothesis thus:
"The fundamental organizational unit of all human goal-oriented
symbolic activity is the problem space." - Newell, 1980.
In the 1980 work, Newell says his claim "hedges on whether
all cognitive activity is symbolic." Laird, Rosenbloom, and
Newell (1985) ignore this hedge and the qualification "goal-
oriented symbolic" when they propose: "Our approach to
developing a general learning mechanism is based on the
hypothesis that all complex behavior - which includes
behavior concerned with learning - occurs as search in problem
spaces." They reference Newell (1980), but their claim is larger
than Newell's original claim.
The purpose of this note is to show that, to be true, Newell's
hypothesis must be taken to mean just that goal-search in a
state-space is a formalism that is equivalent to computing. Then
Newell's Problem Space Hypothesis is simply a true theorem. The
reader is invited to sketch a proof of the mutual
simulatability of Turing computation and a process of goal-search
in a state space. Such a proof has been constructed for every
other prospective universal formalism, e.g., lambda calculus,
recursive function theory, and Post tag systems. That such
universal formalisms are equivalent in this sense led Church
(1936, footnote 3) to speculate that human calculating activity
can be given no more general a characterization.
But human behavior is not restricted to calculating activity
(though it seems that at least some human behavior is
calculating). If the Problem Space Hypothesis is taken to
be a stronger statement, that is, as a statement about human
behavior rather than about the formalism of goal-search in a
state-space, then I claim that the following counter-example
shows it to be false.
Understanding a name is an inherently unsearchable problem; It
cannot be represented as search in a state or problem space.
Well, it can be so represented, but then it is not the same
problem. In searching our states for our goal we are solving a
different problem than the original one.
To understand that understanding is (or how it can be) inherently
unsearchable, it is necessary to distinguish between ambiguity
and equivocacy. At first the distinction seems contrived, but
it is required by the assumption that there are discrete
objects called 'names' that have discrete meaning (some other
associated object or objects, see Church 1986, Berke 1987).
An equivocal word/image has more than one clear meaning, an
ambiguous word/image has none. What is usually meant by the
phrase "lexical ambiguity" is semantic equivocacy. Equivocacy
occurs even in formal languages and systems, though in setting up
a formal system one aims to avoid equivocacy. For example, an
expression in a computer language may be equivocal ("of equal
voices"), such as: 'IF a THEN IF b THEN c ELSE d'. The whole
expression is equivocal depending on which 'IF' the 'ELSE' is
paired with. In this case there are two clear meanings, one for
each choice of 'IF'.
On the other hand, 'ELSE' taken in isolation, is ambiguous
("like both"), it's meaning is not one or many alternatives, but
it is like all of them. [The reader, especially one who may
claim that 'ELSE' has no meaning in isolation, may find it
valuable to pause at this point to write down what 'ELSE' means.
Several good attempts can be generated in very little time,
especially with the aid of a dictionary.]
Resolving equivocacy can be represented as search in a state
space; it may very well BE search in a state space. Resolving
ambiguity cannot be represented as search in a state space.
Resolving environmental ambiguity is the problem-formulation
stage of decision making; resolving objective ambiguity is the
object-recognition phase of perception.
The difference between ambiguity and equivocacy is a reason why
object-recognition and problem-formulation are difficult
programming and management problems, only iteratively
approximable by computation or rational thought. A state space
is, by definition, equivocal rather than ambiguous. If we
confuse ambiguity with equivocacy, ambiguity resolution may
seem like search in goal space, but this ignores the process of
reducing an ambiguous situation to an equivocal one much
the way Turing (1936) consciously ignores the transition of a
light switch from OFF to ON.
A digital process can approximate an analog process yet we
distinguish the digital process from the analog one. Similarly,
an equivocal problem can approximate an ambiguous problem, but
the approximating problem differs from the approximated one.
Even if a bank of mini-switches can simulate a larger light
switch moving from OFF to ON, we don't evade the problem of
switch transition, we push it "down" a level, and then ignore
it. Even if we can simulate an ambiguity by a host of
equivocacies, we don't thereby remove ambiguity, we push it
"down" a level, and then ignore it.
Ambiguity resolution cannot be accomplished by goal-search in a
state space. At best it can be pushed down some levels.
Ambiguity must still be resolved at the lower levels. It doesn't
just go away; ambiguity resolution is the process of it going
away. Representation may require ambiguity resolution, so the
general problem of representing something (e.g., problem solving,
understanding a name) as goal-search in a state space can not
be represented as goal-search in a state space.
This leads me to suspect what may be a stronger result:
"Representing something" in a given formalism cannot be
represented in that formalism. For example, "representing a
thought in words," that is, expression, cannot be represented in
words. "What it is to be a word" cannot be expressed in words.
Thus there can be no definition of 'word' nor then of 'language'.
Understanding a word, if it relies on some representation of
"what it is to be a word" in words, cannot be represented in
words.
The meaning of a word is in this way precluded from being (or
being adequately represented by) other words. This agrees with
our daily observations that "the meaning of a word," in a
dictionary is incomplete. Not all words need be impossible to
completely define, just some of them for this argument to hold.
It also agrees with Church's 1950 arguments on the contradictions
inherent in taking words to be the meaning of other words.
If understanding cannot be represented in words, it can never be
well-defined and cannot be programmed. In programming, we can
and must ignore the low-level process of bit-recognition because
it is, and must be, implemented in hardware. Similarly,
hardware must process ambiguities into equivocacies for
subsequent "logical" processing.
We are thus precluded from saying how understanding works, but
that does not preclude us from understanding. Understanding a
word can be learned as demonstrated by humans daily. Thus
learning is not exhausted by any (word-expressed) formalism.
One example of a formalism that does not exhaust learning
behavior is computation as defined (put into words) by Turing.
Another is goal-search in a state-space as defined (put into
words) by Newell.
References:
Berke, P., "Naming and Knowledge: Implications of Church's
Arguments about Knowledge Representation," in revision for
publication,1987.
Church, A., An Unsolvable Problem of Elementary Number Theory
(Presented to the American Mathematical Society, April 19, 1935),
Journal of Symbolic Logic, 1936.
Church, A., "On Carnap's Analysis of Statements of Assertion
and Belief," Analysis, 10:5, pp. 97-99, April, 1950.
Church, A., "Intensionality and the Paradox of the Name
Relation," Journal of Symbolic Logic, 1986.
Laird, J.E., P.S. Rosenbloom, and A. Newell, "Towards Chunking as
a General Learning Mechanism," CMU-CS-85-110.
Newell, A. "Reasoning, Problem Solving, and Decision Processes:
The problem space as a Fundamental Category. Chapter 35 in R.
Nickerson (Ed.), Attention and Performance VIII. Erlbaum, 1980.
Turing, A.M., On Computable numbers, with an application to the
Entscheidungsproblem. Proceedings of the London Mathematical
Society 42-2 (1936-7), 230-265; Correction, ibid., 43 (1937),
544-546.
------------------------------
Date: 16 Jul 87 09:23:07 GMT
From: mcvax!botter!roelw@seismo.css.gov (Roel Wieringa)
Subject: Berke's Unsearchable Problem
In article 512 of comp.ai Peter Berke says that
1. Newell's hypothesis that all human goal-oriented symbolic activity
is searching through a problem-space must be taken to mean that human
goal-oriented symbolic activity is equivalent to computing, i.e. that
it equivalent (mutually simulatable) to a process executed by a Turing
machine;
2. but human behavior is not restricted to computing, the process of
understanding an ambiguous word (one having 0 meanings, as opposed to
an equivocal word, which has more than 1 meanings) being a case in
point. Resolving equivocality can be done by searching a problem
space; ambiguity cannot be so resolved.
If 1 is correct (which requires a proof, as Berke says), then if 2 is
correct, we can conclude that not all human behavior is searching
through a problem space; the further conclusion then follows that
classical AI (using computers and algorithms to reach its goal)
cannot reach the goal of implementing human behavior as search
through a state space.
There are two problems I have with this argument.
First, barring a quibble about the choice of the terms "ambiguity" and
"equivocality", it seems to me that ambiguity as defined by Berke is really
meaninglessness. I assume he does not mean that part of the surplus
capacity of humans over machines is that humans can resolve meaninglessness
whereas machines cannot, so Berke has not said what he wants to say.
Second, the argument applies to classical AI. If one wishes to show
that "machines cannot do everything that humans can do," one should
find an argument which applies to connection machines, Boltzmann
machines, etc. as well.
Supposing for the sake of the argument that it is important to show
that there is an essential difference between man and machine, I
offer the following as an argument which avoids these problems.
1. Let us call a machine any system which is described by a state
evolution function (if it has a continuous state space) or a state
transition function (discrete state space).
2. Let us call a description explicit if (a) it is communicable to an
arbitrary group of people who know the language in which the
description is stated, (b) it is context-independent, i.e. mentions
all relevant aspects of the system and its environment to be able to
apply it, (c) describes a repeatable process, i.e. whenever the same
state occurs, then from that point on the same input sequence will
lead to the same output sequence, where "same" is defined as
"described by the explicit description as an instance of an input
(output) sequence." Laws of nature which describe how a natural process
evolves, computer programs, and radio wiring diagrams are explicit
descriptions.
Now, obviously a machine is an explicitly described system.
The essential difference between man and machine I propose is that
man possesses the ability to explicate whereas machines do not. The
*ability* to explicate is defined as the ability to produce an
explicit description of a range of situations which (i.e. the range
is) not described explicitly. In principle, one can build a machine
which produces explicit descriptions of, say, objects on a conveyor
belt. But the set of kinds of objects on the belt would then have to
be explicitly described in advance, or at least it would in
principle be explicitly describable, even though the description
would be large, or difficult to find. the reason for this is that a
machine is an explicitly described system, so that, among others, the
set of possible inputs is explicitly described.
On the other hand, a human being in principle can produce
reasonably explicit descriptions of a class of systems which has no
sharp boundaries. I think it is this capability which Berke means
when he says that human beings can disambiguate whereas algorithmic
processes cannot. If the set of inputs to an explication process carried
out by a human being is itself not explicitly describable, then
humans have a capability which machines don't have.
A weak point in this argument is that human beings usually have a
hard time in producing totally explicit descriptions; this is why
programming is so diffcult. Hence, the qualification "reasonably
explicit" above. This does not invalidate the comparison with
machines, for a machine built to produce reasonably explicit
descriptions would still be an explicitly described system, so that
the sets of inputs and outputs would be explicitly described (in
particular, the reasonableness of the explicitness of its output
would be explicitly described as well).
A second argument deriving from the concepts of machine and
explicitness focuses on the three components of the concept of
explicitness. Suppose that an explication process executed by a human
being were explicitly describable.
1. Then it must be communicable; in particular the initial state must be
communicable; but this seems one of the most incommunicable mental states
there is.
2. It must be context-independent; but especially the initial stage
of an explication process seems to be the most context-sensitive
process there is.
3. It must be repeatable; but put the same person in the same
situation (assuming that we can obliterate the memory of the previous
explication of that situation) or put identical twins in the same
situation, and we are likely to get different explicit descriptions
of that situation.
Note that these arguments do not use the concept of ambiguity as
defined by Berke and, if valid, apply to any machine, including
connection machines. Note also that they are not *proofs*. If they
were, they would be explicit descriptions of the relation between a
number of propositions, and this would contradict the claim that the
explication process has very vague beginnings.
Roel Wieringa
------------------------------
End of AIList Digest
********************