Copy Link
Add to Bookmark
Report
AIList Digest Volume 4 Issue 107
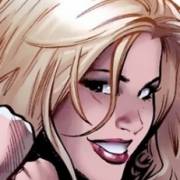
AIList Digest Thursday, 1 May 1986 Volume 4 : Issue 107
Today's Topics:
Seminars - Multivalued Logics (UPenn) &
Mechanisms of Analogy (UCB) &
Recursive Self-Control for Rational Action (SU) &
Reasoning about Multiple Faults (SU) &
Knowledge in Shape Representation (MIT) &
GRAPHOIDS: A Logical Basis for Dependency Nets (SU) &
Decentralized Naming in Distributed Computer Systems (SU) &
Learning in Time (Northeastern) &
Characterization and Structure of Events (SRI)
----------------------------------------------------------------------
Date: Mon, 28 Apr 86 14:29 EDT
From: Tim Finin <Tim%upenn.csnet@CSNET-RELAY.ARPA>
Subject: Seminar - Multivalued Logics (UPenn)
Colloquium - University of Pennsylvania
3:00 pm 4/29 - 216 Moore School
MULTI-VALUED LOGICS
Matt Ginsberg - Stanford University
A great deal of recent theoretical work in inference has involved extending
classical logic in some way. I argue that these extensions share two
properties: firstly, the formal addition of truth values encoding intermediate
levels of validity between true (i.e., valid) and false (i.e., invalid) and,
secondly, the addition of truth values encoding intermediate levels of
certainty between true or false on the one hand (complete information) and
unknown (no information) on the other. Each of these properties can be
described by associating lattice structures to the collection of truth values
involved; this observation lead us to describe a general framework of which
both default logics and truth maintenance systems are special cases.
------------------------------
Date: Tue, 29 Apr 86 08:46:36 PDT
From: admin%cogsci@berkeley.edu (Cognitive Science Program)
Subject: Seminar - Mechanisms of Analogy (UCB)
BERKELEY COGNITIVE SCIENCE PROGRAM
Spring 1986
Cognitive Science Seminar - IDS 237B
Tuesday, April 29, 11:00 - 12:30
2515 Tolman Hall
Discussion: 12:30 - 1:30
3105 Tolman (Beach Room)
``Mechanisms of Analogy''
Dedre Gentner
Psychology, University of Illinois at Champaign-Urbana
Analogy is a key process in learning and reasoning. This
research decomposes analogy into separable subprocesses and
charts dependencies. Evidence is presented that (1) once an
analogy is given, people map predicates and judge soundness
chiefly on the basis of common relational structure, as
predicted by the structure-mapping theory; (2) in contrast,
access to potential analogue depends heavily on common surface
features.
Accessibility and inferential power appear to be governed
by different kinds of similarity. This finer-grained analysis
of similarity helps resolve conflicting evidence concerning the
role of similarity in transfer.
------------------------------
Date: Mon 28 Apr 86 14:31:52-PDT
From: Anne Richardson <RICHARDSON@SU-SCORE.ARPA>
Subject: Seminar - Recursive Self-Control for Rational Action (SU)
DAY: May 5
EVENT: AI Seminar
PLACE: Jordan 050
TIME: 4:15
TITLE: Recursive Self-Control:
A Computational Groundwork for Rational Action
PERSON: John Batali
FROM: MIT AI Lab
Human activity must be understood in terms of agents interacting with
the world, those interactions subject to the details of the situation
and the limited abilities of the agents. Rationality involves an
agent's deliberating about and choosing actions to perform. I suggest
that deliberation and choice are themselves best viewed as activities of
the agent. This leads to a view of rationality based on "recursive
self-control" wherein the agent controls the activity of its body in
much the same way as a programmer controls a computational mechanism.
To prove that this view is really recursive, rather than just
meaninglessly circular, I describe a computer program whose architecture
illustrates how recursive self-control could work.
------------------------------
Date: Tue 29 Apr 86 13:12:59-PDT
From: Christine Pasley <pasley@SRI-KL>
Subject: Seminar - Reasoning about Multiple Faults (SU)
CS529 - AI In Design & Manufacturing
Stanford University
Instructor: Dr. J. M. Tenenbaum
Title: Reasoning About Multiple Faults
Speaker: Johan de Kleer
From: XEROX Palo Alto Research Center
Date: Wednesday, April 30, 1986
Time: 4:00 - 5:30
Place: Terman 556
Diagnostic tasks require determining the differences between a model
of an artifact and the artifact itself. The differences between the
manifested behavior of the artifact and the predicted behavior of the
model guide the search for the differences between the artifact and
its model. The diagnostic procedure presented in this paper reasons
from first principles, inferring the behavior of the composite device
from knowledge of the structure and function of the individual
components comprising the device. The system has been implemented
and tested on examples in the domain of troubleshooting digital
circuits.
This research makes several novel contributions: First, the system
diagnoses failures due to multiple faults. Second, failure candidates
are represented and manipulated in terms of minimal sets of violated
assumptions, resulting in an efficient diagnostic procedure. Third,
the diagnostic procedure is incremental, reflecting the interactive
nature of diagnosis. Finally, a clear separation is drawn between
diagnosis and behavior prediction, resulting in a domain (and
inference) independent diagnostic procedure which can be incorporated
into a wide range of inference procedures.
Visitors welcome!
------------------------------
Date: Tue, 29 Apr 86 19:13 EDT
From: Eric Saund <SAUND@OZ.AI.MIT.EDU>
Subject: Seminar - Knowledge in Shape Representation (MIT)
Thursday, 1 May 4:00pm Room: NE43-8th floor playroom
--- AI Revolving Seminar ---
KNOWLEDGE, ABSTRACTION, AND CONSTRAINT
IN SHAPE REPRESENTATION
Eric Saund
MIT AI Lab
What can make a profile look like an apple?
Early Vision teaches that you must know something if you are going to
see. The physics of light, the geometry of the eyes, the smoothness
of surfaces, all impose >constraint< on images. It is only through
the application of >knowledge< about this structure in the visual
world that early vision may invert the imaging process and recover
surface orientations, light sources, and reflectance properties. We
should take this lesson seriously in attempting intermediate and later
vision such as shape understanding.
Key to using knowledge in vision is building representations to
reflect the structure of the visual world. The mathematically
expressed laws of early vision do not help for later vision. How is
one to express the constraint on a profile that might qualify it as an
apple? In this talk I will discuss steps toward construction of a
vocabulary for shape representation rich enough to express the complex
and subtle relationships between locations and sizes of boundaries and
regions that give rise to object parts and shape categories. I will
describe three computational tools, "scale-space", "dimensionality-
reduction", and "functional role abstraction", for building symbolic
descriptors to capture constraint in shape information. Examples of
their use will be shown in a one-dimensional, model, shape domain.
------------------------------
Date: Tue 29 Apr 86 15:51:12-PDT
From: Benjamin N. Grosof <GROSOF@SU-SCORE.ARPA>
Subject: Seminar - GRAPHOIDS: A Logical Basis for Dependency Nets (SU)
JUDEA PEARL of the UCLA Computer Science Department will be speaking on
probabilistic reasoning
FRIDAY MAY 2 2:15pm JORDAN 040
GRAPHOIDS: A Logical Basis
for Dependency Nets
or
When would x tell you more about y
if you already know z
ABSTRACT:
We consider statements of the type:
I(x,z,y) = "Knowing z renders x independent of y",
where x and y and z are three sets of propositions.
We give sufficient conditions on I for the existence
of a (minimal) graph G such that I(x,z,y) can be validated
by testing whether z separates x from y in G. These
conditions define a GRAPHOID.
The theory of graphoids uncovers the axiomatic basis of
probabilistic dependencies and extends it as a formal
definition of informational dependencies. Given an
initial set of dependency relations, the axioms
established permit us to infer new dependencies by
non-numeric, logical manipulations, thus identifying
which propositions are relevant to each other in a
given state of knowledge. Additionally,
the axioms may be used to test the legitimacy of
using networks to represent various types of
data dependency, not necessarily probabilistic.
------------------------------
Date: 29 Apr 1986 1915-PDT (Tuesday)
From: Tim Mann <mann@su-pescadero.ARPA>
Subject: Seminar - Decentralized Naming in Distributed Computer Systems (SU)
This is to announce my PhD oral, scheduled for Tuesday May 6, 2:15 pm,
building 160, room 163B.
Decentralized Naming in Distributed Computer Systems
Timothy P. Mann
A key component in distributed computer systems is the naming facility:
the means by which global, user-assignable names are bound to objects,
and by which objects are located given only their names. This work
proposes a new approach to the construction of such a naming facility,
called \decentralized naming/. In systems that follow this approach,
the global name space and name mapping mechanism are implemented by the
managers of named objects, cooperating as peers with no central
authority. I develop the decentralized naming model in detail and
characterize its fault tolerance, efficiency, and security. I also
describe the design, implementation, and measured performance of a
decentralized naming facility that I have constructed as a part of the
V distributed operating system.
------------------------------
Date: Wed, 30 Apr 86 16:30 EST
From: SIG%northeastern.edu@CSNET-RELAY.ARPA
Subject: Seminar - Learning in Time (Northeastern)
Learning in Time
Richard Sutton (rich@gte-labs.csnet)
GTE Labs, Waltham, Ma.
Most machine learning procedures apply to learning problems in which
time does not play a role. Typically, training consists of the
presentation of a sequence of pairs of the form (Pattern, Category),
where each pattern is supposed to be mapped to the associated category,
and the ordering of the pairs is incidental. In real human learning, of
course, the situation is very different: successive input patterns are
causally related to each other, and only gradually do we become sure of
how to categorize past patterns. In recognizing spoken words, for
example, we may be sure of the word half way through it, after it has
all been heard, or not until several words later; our certainty of the
correct classification changes and becomes more certain over time. In
learning to make such classifications, is it sufficient to just
correlate pattern and category, and ignore the role of time? In this
talk, I claim that the answer is NO. A new kind of learning is
introduced, called Bootstrap Learning, which can take advantage of
temporal structure in learning problems. Examples and results are
presented showing that bootstrap learning methods require significantly
less memory and communication, and yet make better use of their
experience than conventional learning procedures. Surprisingly, this
seems to be a case where consideration of an additional complication --
the temporal nature of most real-world problems -- results in BOTH
better performance AND better implementations. These advantages appear
to make bootstrap learning the method of choice for a wide range of
learning problems, from predicting the weather to learning evaluation
functions for heuristic search, from understanding classical
conditioning to constructing internal models of the world, and, yes,
even to routing telephone calls.
Wednesday, May 14, 12:00 noon
161 Cullinane Hall
Sponsored by:
College of Computer Science
Northeastern University
360 Huntington Ave.
Boston, Ma
Host: Steve Gallant
(sig@northeastern.csnet)
------------------------------
Date: Wed 30 Apr 86 17:08:07-PDT
From: LANSKY@SRI-AI.ARPA
Subject: Seminar - Characterization and Structure of Events (SRI)
THE CHARACTERIZATION AND STRUCTURE OF EVENTS
Douglas D. Edwards (EDWARDS@SRI-AI)
SRI International, Artificial Intelligence Center
11:00 AM, MONDAY, May 5
SRI International, Building E, Room EJ228 (new conference room)
Events were raised to prominence as a basic ontological category in
philosophy by Davidson, who used quantified variables ranging over
events in the logical analysis of assertions about causality and
action, and of sentences with adverbial modifiers. Drew McDermott
used the category of events in AI planning research to model changes
more complex than state transformations.
Despite the common use of events as an ontological category in
philosophy, linguistics, planning research, and ordinary language,
there is no standard characterization of events. Sometimes, as in
Davidson, they are taken to be concrete individuals. Other authors
think of them as types or abstract entities akin to facts,
propositions, or conditions; as such they are often subjected to
truth-functional logical operations, which Davidson considers to be
inapplicable. McDermott, following Montague in broad outline, thinks
of them as classes of time intervals selected from various possible
histories of the world. Other authors emphasize individuation of
events not just by time but also by spatial location, by the objects
or persons participating, or (Davidson) by their location in a web of
causes and effects.
In this talk I sketch a scheme for characterizing types of events
which illuminates the relationship between type and token events, the
internal structure and criteria of individuation of events, and the
relationship of events to other categories of entities such as
objects, facts, and propositions. Events turn out to be structured
entities like complex objects, not simple temporal or spatiotemporal
regions or classes of such.
------------------------------
End of AIList Digest
********************