Copy Link
Add to Bookmark
Report
AIList Digest Volume 3 Issue 185
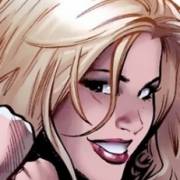
AIList Digest Friday, 13 Dec 1985 Volume 3 : Issue 185
Today's Topics:
Queries - Expert Systems for Building Configuration & LIPS,
Policy - AI&DS Name Change and Advertising,
Literature - Spang Robinson Report No. 2 &
Proceedings of the 1st Annual Conference on Law and Technology,
Seminars - Parallel Depth-First Search (MIT) &
Quantitative Operators in Design (UTexas) &
An Object Model of Information (CMU) &
Massively Parallel Networks that Learn Representations (MIT)
----------------------------------------------------------------------
Date: 11 December 1985 1207-EST
From: William Proffer@A.CS.CMU.EDU
Subject: Bldg Config. KBES
I'd like to know if anyone has any information on
expert systems used in the configuration of office
buildings for tenants. For instance, configuration of
utilities, space, parking, etc.
Please mail replies to proffer@s3sun.css.gov
Thanks. Bill Proffer
------------------------------
Date: Tue, 10 Dec 85 21:08 CST
From: Jerry Bakin <Bakin@HI-MULTICS.ARPA>
Subject: fLIPS, pLIPS, nLIPS, uLIPS, mLIPS, LIPS, KLIPS, MLIPS, GLIPS,
In many Prolog discusions, I have heard people talk about the speed of
an implementation in terms of Logical Inferences per Second (LIPS). I
don't recall ever seeing a formal definition of this.
Would someone care to discuss what a LIP is? Is it one unit of
unification? Some sort of average time it takes prolog to scan
a clause?
As benchmarks go, is apples and oranges, or red delicious and golden
delicious?
How can it be compared to lisp?
In terms of LIPS, how fast are current Prolog implementations?
DEC-10? DEC-20? Symbolics? Micro-Prolog?
And finally, in terms of LIPS, how fast are LISP implementations for the
DEC-20 and Symbolics (or other machines)?
Thanks,
Jerry Bakin <Bakin at HI-Multics>
------------------------------
Date: Tue, 10 Dec 1985 05:50 CST
From: AI.DUFFY@R20.UTEXAS.EDU
Subject: Name Change: Banned AIDS!
Date: Thursday, 5 December 1985 15:33-CST
From: clif at aids-unix (Clif McCormick)
Advanced Information & Decision Systems
... advertising deleted ...
has changed its name to:
ADVANCED DECISION SYSTEMS
... advertising deleted ...
The name change comes as Advanced Decision Systems seeks to expand
its involvement in the commercial marketplace and add to its list
of commerical clients, which currently includes
... assorted multinationals deleted ...
Come on, tell us WHY you've changed your name! All you've done is
removed the word INFORMATION from your name. What do you have against
information? Are you trying to promote RANDOMNESS? Or maybe it's
your commercial clients who don't like information. Is that it?
Hmmmm.... Maybe its the acronym. Maybe you don't like the acronym
AIDS because you're not really in the business of AIDing anybody
(except of course your commercial clients) and you don't want people
bothering you whenever they need some AID. You've now changed the
acronym to ADS. So that must be it. You aren't against information
at all. You've just decided to change your name to ADS to let people
know you like to place ADS on AIList.
Right?
[The original message was sent to AIList as a "press release". I
chose to run it under "Bindings", although much of it fell under "Lab
Descriptions". I have permitted such reports from other sites (as does
the SIGART Newsletter) -- I usually decide in favor of the transmission
of new information and the suppression of repeated information, but
do screen out job ads and anything that I consider blatantly
commercial. My criteria have been partly shaped by the feedback or
lack thereof from readers, and such feedback is always welcome. -- KIL]
------------------------------
Date: 10 Dec 1985 18:03-CST
From: leff%smu.csnet@CSNET-RELAY.ARPA
Subject: Spang Robinson Report, No 2
Summary of Spang Robinson Report, Dec 1985 Vol 1 NO. 2
AI AND MANUFACTURING
Carnegie makes 70 percent of its 8 million in revenue from custom
applications to clients in manufacturing.
Teknowledge makes 50 percent of its revenues from custom consulting
30 percent of KEE applications are in manufacturing.
A. D. Little has developed a "Smart Foreman" expert system
Arthur Anderson has developed KIOSK, which helps process special orders,
and Cell Designer which assigns parts and assigns machines to processes
and cells.
Applicon has demonstrated a knowledge based CAD/CAM workstation for
sheet metal fabrication.
International Systems Operations is developing systems to assist
in quality control and material movement.
Cognition is developing a system that captures design knowledge in
handbooks and standard textbooks as well as corporate design standards.
TIPNIS will provide manufacturing expertise to the company's designers.
LMI will offer connectivity between PICON, it's real-time expert system
tool and MAP, the new standard for factory floor networking. This
will involve a < $7,000 board set.
REVIEW of Texas Instruments satellite AI broadcast.
News Section:
ICONICS has changed its name to Transform LOGIc corporation. They are
devleoping an AI-based software engineering system.
RCA has established an AI chair at the University of Pennsylvania.
VOTAN has announced a voice system for entering or verifying data which
costs $4950.00.
Carnegie Group has introduced Version 3 of Knowledge Craft.
Artelligence and Computer*Thought have filed a law suit
regarding breaches of non-competition agreements.
Teknowledge has named Michael P. Chan, vice president of product sales
for its Knowledge Engineering
LISP machine has elected three new members of its board of directors.
Summary of Artificial Intelligence: Towards Practical Applications
------------------------------
Date: Tue, 10 Dec 85 15:09:29 pst
From: George Cross <cross%wsu.csnet@CSNET-RELAY.ARPA>
Subject: Proceedings: 1st Annual Conference on Law and Technology
Here is the table of contents of the recently published proceedings of the
First Annual Conference on Law and Technology, held at the University of
Houston in August 1984 and sponsored by the University of Houston Law Center
Program on Law and Technology. There was a Second Conference in 1985, but the
proceedings are not available yet. The book is:
%B Computing Power and Legal Reasoning
%E Charles Walter
%I West Publishing Company
%C St. Paul, MN
%D 1985
ISBN 0-314-95570-4
and is available from
West Publishing Company
50 West Kellogg Boulevard
P.O. Box 64526
St. Paul, MN 55164-1003
- - - - - - - - - - - - - - - - - - - - - -
%A Layman Allen
%A Charles Saxon
%T Computer-Aided Normalizing and Unpacking:
Some Interesting Machine-Processable Transformations of Legal Rules
%P 495-572
%A Kevin Ashley
%T Reasoning by Analogy: A Survey of Selected AI Research
with Implications for Legal Expert Systems
%P 105-127
%A Helene Bauer-Bernet
%T Beyond Keyword Interaction: Computerized European
Community Law
%P 337-374
%A Daniele Bourcier
%T About Intelligence in Legal Information Systems
%P 319-336
%A William Boyd
%T Choosing Between a Chapter 7 and a Chapter 13 Bankruptcy:
An "Expert System" to Assist an Attorney in Making the Choice
%P 699-763
%A Constantino Ciampi
%A Deirdre Exell Pirro
%A Elio Fameli
%A Giuseppe Trivisonno
%T THES/BID: An Expert System for Constructing
a Computer-Based Thesaurus for Legal Informatics and Computer Law
%P 375-412
%A Cary deBessonet
%A George Cross
%T Representation of Some Aspects of Legal Causality
%P 205-214
%A Michael Dyer
%A Margot Flowers
%T Toward Automating Legal Expertise
%P 49-68
%A Jerald Feinstein
%T A Knowledge-Based Expert System Used to Prevent
the Disclosure of Sensitive Information at the
United States Environmental Protection Agency
%P 661-697
%A Thomas Gordon
%T Object-Oriented Predicate Logic and Its Role in
Representing Legal Knowledge
%P 163-203
%A Grayfred Gray
%T Statutes Enacted in Normalized Form:
The Legislative Experience in Tennessee
%P 467-493
%A Grayfred Gray
%T Law & Technology Conference Expert System Workshop Report
%P 621-626
%A Michael Heather
%T Demand-Driven Model for a Half-Intelligent System
%P 69-103
%A Jay Hook
%T Semantic Representations of Children's Blame Rules
%P 29-47
%A Robert Krovetz
%T The Use of Knowledge Representation Formalisms in
the Modeling of Legal Concepts
%P 275-317
%A Sydney Lamb
%T Information and its Representation in English Texts
%P 145-155
%A C. Duncan MacRae
%T Tax Problem Solving with an If-Then System
%P 595-620
%A Antonio Martino
%T Why an Automated Analysis of Legislation?
%P 413-466
%A L. Thorne McCarty
%T Permissions and Obligations
%P 573-594
%A Mark Peterson
%A Donald Waterman
%T An Expert Systems Approach to Evaluating Product Liability Cases
%P 627-659
%A Edwina Rissland
%T Argument Moves and Hypotheticals
%P 129-143
%A Dean Schlobohm
%T TA -- A Prolog Program which Analyzes Income Tax Issues
under Section 318(A) of the Internal Revenue Code
%P 765-815
%A Franciszek Studnicki
%T Computational Aspects of Legal Interpretation
%P 157-161
%A Charles Walter
%T Introduction
%P 1-4
%A Charles Walter
%A Michael Parks
%T Natural Models of Intelligence
%P 5-27
%A Marshall Willick
%T Professional Malpractice and the Unauthorized Practice of Professions:
Some Legal and Ethical Aspects of the use of Computers and Decision-Aids
%P 817-863
%A Gian Piero Zarri
%T Inference Techniques for Intelligent Information Retrieval
%P 247-274
- - - - - - - - - - - - - - - - - - - - - - - - - - - - - -
George R. Cross cross@wsu.CSNET
Computer Science Department cross%wsu@csnet-relay.ARPA
Washington State University faccross@wsuvm1.BITNET
Pullman, WA 99164-1210 Phone: 509-335-6319 or 509-335-6636
------------------------------
Date: Wed, 11 Dec 85 10:50:41 EST
From: "Lisa F. Melcher" <LISA@MIT-MC.ARPA>
Subject: Seminar - Parallel Depth-First Search (MIT)
DATE: Friday, December 13, 1985
TIME: 12:45.....Refreshments
1:00.....Lecture
PLACE: NE43 - 512A
"A PARALLEL ALGORITHM FOR DEPTH FIRST SEARCH"
Richard Anderson
MSRI
A new parallel algorithm for constructing a depth first search tree in an
undirected graph will be described. The algorithm is a P-RAM algorithm and
uses several probabilistic algorithms as sub-routines.
The run time of the algorithm is 2 sq.rt. log n. This makes it an almost RNC
eps.
algorithm, since the run time is less than n for any eps.>0.
The standard sequential algorithm for depth first search can be shown to be
"inherently sequential", so this shows that substantial speed up for depth
first search is possible when a different approach is taken.
David Shmoys
Host
------------------------------
Date: Tue, 10 Dec 85 12:57:28 cst
From: Ted Briggs <briggs@SALLY.UTEXAS.EDU>
Subject: Seminar - Quantitative Operators in Design (UTexas)
Quantitative Operators in Design
by
David R Throop
noon Friday Dec. 13
PAI 3.38
Doing design problems involves reasoning about quantities.
AI methods have not made great headway into engineering
design problems so far. They have lacked the power to
reason about quantities. Meanwhile, other numeric
techniques have lacked the AI systems' abilities in handling
heuristic knowledge.
I propose to extend AI representations to allow reasoning
about quantities in design. My approach has these features:
Encoding heuristic knowledge as frames with quantita-
tive operators, operators with an action and a
strength.
Beginning design by solving subproblems, guided by the
arbitration of conflicting quantitative heuristics.
Reasoning quantitatively about the aggregate design,
modifying it using non-local knowledge.
Encoding the procedures to arbitrate conflicting
heuristics as knowledge at a general level: the para-
digm level.
To test the power of this approach, I will apply it to an
outstanding problem in Chemical Engineering: the synthesis
of heat exchanger networks. This problem has many published
non-AI computer approaches. This literature provides a test
of my representation and its adequacy for real design prob-
lems.
------------------------------
Date: 6 Dec 1985 1331-EST
From: David A. Evans <DAE@C.CS.CMU.EDU>
Subject: Seminar - An Object Model of Information (CMU)
Speaker: David Beech,
Center for Integrated Systems, Stanford University, and
Hewlett-Packard Laboratories, Palo Alto
Time: Wednesday, 11 December 1985, 1:30-3:00 PM, Wean 5409
Title: Towards an Object Model of the Representation
and Use of Information
Future general-purpose information systems will need to deal with a wide
range of information, and offer flexible access to it, if they are to
appeal to the potential millions of non-specialist users. For example,
they should process pictures and sounds as naturaly as numbers and
texts; they should answer questions which require some deduction from
the often incomplete information previously given to the system; and
they should move towards the support of natural language interfaces,
including spoken inputs.
An object-oriented model of the representation and use of information is
proposed, with the necessary generality for the desription and design of
such systems. Fundamental concepts including those of agent, object,
type, action, formula, process, transaction, predicator and generator
are intro- duced. Recursive functions, predicate calculus, and n-ary
relations are brought together in a data abstraction framework, with an
emphasis on intensional definition of concepts and their instantiation
by means of predicators and generators.
------------------------------
Date: Fri, 6 Dec 1985 10:21 EST
From: ELIZABETH%MIT-OZ@MIT-MC.ARPA
Subject: Seminar - Parallel Networks that Learn Representations (MIT)
MASSIVELY PARALLEL NETWORKS THAT LEARN REPRESENTATIONS
Geoffrey Hinton
Carnegie-Mellon University
Monday, December 9
Eighth Floor Playroom, AI Lab
4:00 pm
I shall describe a new learning procedure for massively parallel
networks of neuron-like processing elements. The procedure adjusts
the connection strengths in a multi-layered network so as to make it
give the correct output vector when given an input vector. The units
in the intermediate layers come to represent important implicit
features of the task domain that generates the pairs of input/output
vectors. As a result, the network can generalise appropriately to new
cases. I shall describe a pattern recognition example in which the
network constructs a balanced ecology of feature detectors, and a
higher level task in which the network learns a set of relationships.
The second example illustrates the ability of the network to recognize
isomorphisms and make use of them in encoding and generalizing
knowledge.
------------------------------
End of AIList Digest
********************