Copy Link
Add to Bookmark
Report
AIList Digest Volume 3 Issue 082
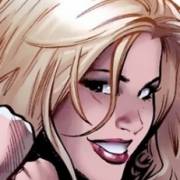
AIList Digest Monday, 24 Jun 1985 Volume 3 : Issue 82
Today's Topics:
Queries - VAX Lisp & PC Lisps & McDonnell Douglas NL Breakthrough,
Games - Optimal Scrabble,
Automata - Predation/Cooperation,
Psychology - Common Sense,
Analogy - Bibliography,
Seminar - Evaluating Expert Forecasts (NASA)
----------------------------------------------------------------------
Date: Mon, 24 Jun 85 07:38:35 EDT
From: cugini@NBS-VMS
Subject: VAX Lisp
Just looking for a little consumer information here - does anyone have
any experience with Digital's VAX LISP ? DEC advertises it as a
full-fledged implementation of CommonLisp. Any remarks on price,
performance, quality, etc are appreciated.
John Cugini <Cugini@NBS-VMS>
Institute for Computer Sciences and Technology
National Bureau of Standards
Bldg 225 Room A-265
Gaithersburg, MD 20899
phone: (301) 921-2431
------------------------------
Date: Sun 23 Jun 85 15:09:12-EDT
From: Jonathan Delatizky <DELATZ%MIT-OZ@MIT-MC.ARPA>
Subject: PC Lisps
[Forwarded from the MIT bboard by SASW@MIT-MC.]
Can some of you out there who have used Lisp implementations on IBM PC
type machines give me some recommendations as to the best PC Lisp? I
plan to run it on a PC/XT and a PC/AT if possible. Also, any expert systems
shells that run on the same machines, real or toy-like.
...jon
------------------------------
Date: 22 Jun 1985 13:20-EST
From: George Cross <cross%lsu.csnet@csnet-relay.arpa>
Subject: McDonnell Douglas NL Breakthrough
The following is the text of a full page color ad on page 49
in the June 24, 1985 New Yorker. It has also been run in the
Wall Street Journal. Does anyone know what the breakthrough
is? This was mentioned on the ailist some time ago but I
didn't notice a response. There is a photo of a hand holding
the chin of smiling boy.
BREAKTHROUGH: A COMPUTER THAT UNDERSTANDS YOU LIKE YOUR MOTHER
Having to learn letter-perfect software languages can be frustrating to the
average person trying to tap the power of a computer.
But practical thinkers at our McDonnell Douglas Computer Systems Company
have created the first computer that accepts you as you are - human.
They emulated the two halves of the brain with two-level software: One level
with a dictionary of facts and a second level to interpret them. The
resulting Natural Language processor understands everyday conversational
English. So it knows what you mean, no matter how you express yourself. It
also learns your idiosyncrasies, forgives your errors, and tells you how to
find out what you're looking for.
Now, virtually anyone who can read and write can use a computer.
We're creating breakthroughs not only in Artificial Intelligence but also in
health care, space manufacturing and aircraft.
We're McDonnell Douglas.
How can I learn more?
Write
P.O. Box 19501
Irvine, CA 92713
------------------------------
Date: 22 Jun 1985 13:07-EDT
From: Jon.Webb@CMU-CS-IUS2.ARPA
Subject: Optimal Scrabble
Anyone interested in computer Scrabble should be aware that Guy
Jacobson and Andrew Appel (some of the people that did Rog-o-matic)
have written a program which in some sense solves the problem. Using a
clever data structure, their program makes plays in a few seconds and
always makes the best possible play. Their dictionary is the official
Scrabble dictionary. The program is not completely optimal because it
doesn't take into account how the placement of its words near things
like triple word scores may help the other player, but in all other
senses it always makes the best play. I suppose some simple strategic
techniques could be added using a penalty function, but as the program
almost always wins anyway, this hasn't been done. It regularly gets
bingos (all seven letters used), makes clever plays that create three
or more words, and so on. The version they have now runs on Vax/Unix.
There was some work to port it to the (Fat) Macintosh but that is not
finished, mainly for lack of interest.
Jon
------------------------------
Date: Fri, 21 Jun 85 17:17:58 EDT
From: David_West%UMich-MTS.Mailnet@MIT-MULTICS.ARPA
Subject: Predation/Cooperation (AIL v3 #78)
Re: enquiry of sdmartin@bbng about learning cooperation in predation:
For an extensive investigation of a minimal-domain model (prisoner's
dilemma),see _The Evolution of Co-operation_ (NY: Basic Books, 1984;
LC 83-45255, ISBN 0-465-02122-0) by Robert Axelrod (of the U of Mich).
He is in the Institute of Public Policy Studies, but one of his more
interesting methods was the use of the genetic algorithms of John
Holland (also of the U of Mich) to breed automata to have improved
strategies for playing Prisoner's dilemma. A one-sentence summary of
his results is that cooperation can displace non-cooperation if
individuals remember each other's behavior and have a high enough
probability of meeting again. An intermediate-length summary can be
found in Science _211_ (27 Mar 81) 1390-1396.
------------------------------
Date: Fri 21 Jun 85 19:23:03-PDT
From: Calton Pu <CALTON@WASHINGTON.ARPA>
Subject: definition of common sense
I had a discussion with a friend on this exact topic just a few weeks
ago. My conclusions can be phrased as an elaboration of V. Pratt's
two criteria.
1. common knowledge basis (all facts depended on must be
common knowledge)
I think the (abstract) common knowledge basis can be more concretely
described as "cultural background". Your Formico's Pizza example
shows clearly that anybody not familiar with San Francisco will not
have the "common sense" to go there. The term "cultural background"
admits many levels of interpretation (national, provincial, etc.)
so most of REALLY COMMON knowledge basis will be encompassed.
2. low computational complexity (easy to check the conclusion).
I think the key here is not the checking (NP), but the finding (P) of
the solution. So here I differ from Vaughan, in that I believe common
sense is something "obvious" to a lot of people, by their own
reasoning power.
There are two factors involved: the first is the amount of reasoning
power; the second is the amount of deductive processing involved. On
the first factor, unfortunately usual words to describe people with
the adequate reasoning power such as "sensible", "reasonable", and
"objective" have also the connotation of being "emotionless". Let's
leave out the emotional aspects and use the term "reasonable" to
include everybody who is able to apply elementary logic to normal
situations. On the second factor, typical words to picture easy
deductive efforts are "obvious", "clear", and "evident".
So my definition of common sense is: that which is obvious to a
reasonable person with an adequate cultural background.
I should point out that the three parameters of common sense, cultural
background, reasoning power, and deductive effort, vary from place to
place and from person to person. If we agreed more on each other's
common sense, it might be easier to negotiate peace.
------------------------------
Date: Monday, 24 Jun 85 01:38:08 EDT
From: shrager (jeff shrager) @ cmu-psy-a
Subject: Analogy Bibliography
[Someone asked for an analogy bibliography a while back. This was compiled
about two years (maybe more) ago so it's partial and somewhat out of date,
but might serve as a starter for people interested in the topic. I've added
a couple of thing just now in looking it over. The focus is primarily
psychological, but readers will recognize some of the principle AI work as
well. I've got annotations for quite a few of these, but the remarks are
quite long and detailed so I won't burden AIList with them. -- Jeff]
ANALOGY
(A partial bibliography)
Compiled by Jeff Shrager
CMU Psychology
24 June 1985
(Send recommendations to Shrager@CMU-PSY-A.)
Bobrow, D. G. & Winograd, T. (1977). An Overview of KRL: A Knowledge
Representation Language. Cognitive Science, 1, 3-46.
Bott, R.A. A study of complex learning: Theories and Methodologies. Univ. of
Calif. at San Diego, Center for Human Information Processing report No.
7901.
Brown, D. (1977). Use of Analogy to Acheive New Experience. Technical Report
403, MIT AI Laboratory.
Burstein, M. H. (June, 1983). Concept Formation by Incremental Analogical
Reasoning and Debugging. Proceedings of the International Machine
Learning Workshop. pp. 19-25.
Carbonell, J. G. (August, 1981). A computational model of analogical problem
solving. Proceedings of the Seventh International Joint Conference on
Artificial Intelligence, Vancouver. pp. 147-152.
Carbonell, J.G. (1983). Learning by Analogy: Formulating and Generalizing
Plans from Past Experience. In Michalski, R.S., Carbonell, J.G., &
Mitchell, T.M. (Ed.), Machine Learning, an Aritificial Intelligence
Approach Palo Alto: Tioga Press.
Carnap, R. (1963). Variety, analogy and periodicity in inductive logic.
Philosophy of Science, 30, 222-227.
Darden, L. (June, 1983). Reasoning by Analogy in Scientific Theory
Construction. Proceedings of the International Machine Learning Workshop.
pp. 32-40.
de Kleer, J. & Brown, J.S. Foundations of Envisioning. Xerox PARC report.
Douglas, S. A., & Moran, T. P. (August, 1983). Learning operator semantics by
analogy. Proceedings of the National Conference on Artificial
Intelligence.
Douglas, S. A., & Moran, T. P. (December, 1983b). Learning text editor
semantics by analogy. Proceedings of the Second Annual Conference on
Computer Human Interaction. pp. 207-211.
Dunker, K. (1945). On Problem Solving. Psychological Monographs, 58, .
Evans, T. G. (1968). A program for the solution of a class of geometric
analogy intelligence test questions. In Minsky, M. (Ed.), Semantic
Information Processing Cambridge, Mass.: MIT Press. pp. 271-253.
Gentner, D. (July, 1980). The Structure of Analogical Models in Science.
Report 4451, Bolt Beraneck and Newman.
Gentner, D. (1981). Generative Analogies as Mental Models. Proceedings of
the 3rd National Cognitive Science Conference. pp. 97-100. Proceedings
of the 3rd annual conference.
Gentner, D. (1982). Are Scientific Analogies Metaphors? In D. S. Miall (Ed.),
Metaphor: Problems and Perspectives New York: Harvester Press Ltd. pp.
106-132.
Gentner, D., & Gentner, D. R. (1983). Flowing Waters or Teeming Crowds: Mental
Models of Electricity. In Gentner, D. & Stevens, A. L. (Ed.), Mental
Models Hillsdale, NJ: Lawrence Earlbaum Associates. pp. 99-129.
Gick, M. L. & Holyoak, K. J. (1980). Analogic Problem Solving. Cognitive
Psychology, 12, 306-355.
Gick, M. L. & Holyoak, K. J. (1983). Schema Induction and Analogic Transfer.
Cognitive Psychology, 15, 1-38.
Halasz, F. & Moran, T. P. (1982). Analogy Considered Harmful. Proceedings of
the Conference on Human Factors in Computer Systems, New York.
Hesse, Mary. (1955). Science and the Human Imagination. New York:
Philisophical Library.
Hesse, Mary. (1974). The Structure of Scientific Inference. Berkeley: Univ.
of Calif. Press.
Kling, R. E. (1971). A Paradigm for Reasoning by Analogy. Artificial
Intelligence, 2, 147-178.
Lenat, D.B. & Greiner, R.D. (1980). RLL: A representation language language.
Proc. of the first annual meeting. Stanford.
McDermott, J. (December, 1978). ANA: An assimilating and accomodatiing
production system. Technical Report CMU-CS-78-156, Carnegie-Mellon
University.
McDermott, J. (1979). Learning to use analogies. Sixth Internation Joint
Conference on Artificial Intelligence.
Medin, D. L. and Schaffer, M. M. (1978). Context Theory of Classification
Learning. Psychological Review, 85(3), 207-238.
Minsky, M. (1975). A Framework for Representing Knowledge. In Winston, P.H.
(Ed.), The Psychology of Computer Vision New York: McGraw Hill.
Minsky, M. (July, 1982). Learning Meaning. Technical Report, . Unpublished
MIT AI Lab techinical report.
Moore, J. & Newell, A. (1974). How can MERLIN Understand? In L.W.Gregg (Ed.),
Knowledge and Cognition Potomic, Md.: Erlbaum Associates.
Ortony, A. (1979). Beyond Literal Similarity. Psych Review, 86(3), 161-179.
Pirolli, P. & Anderson, J.R. (1985) The role of Learning from Examples in the
Acquisition of Recursive Programming Skills. Canadian Journal of
Psychology. Vol. 39, no. 4; pgs. 240-272.
Polya, G. (1945). How to solve it. Princton, N.J.: Princeton U. Press.
Quine, W. V. O. (1960). Word and Object. Cambridge: MIT Press.
Reed, S. K., Ernst, G. W., & Banerji, R. (1974). The Role of Analogy in
Transfer Between Similar Problem States. Cognitive Psychology, 6,
436-450.
Rosch, E., Mervis, C. B., Gray, W. D., Johnson, D. M., & Boynes-Braem, P.
(1976). Basic Objects on Natural Kinds. Cog Psych, 8, 382-439.
Ross, B. (1982). Remindings and Their Effects in Learning a Cognitive Skill.
PhD thesis, Stanford.
Rumelhart, D.E., & Norman, D.A. (?DATE?). Accretion, tuning, and
restructuring: Three modes of learning. In R.Klatsky and J.W.Cotton
(Eds.), Semantic Factors in Cognition Hillsdale, N.J.: Erlbaum
Associates.
Rumerlhart, D.E. & Norman, D.A. (1981). Analogical Processes in Learning. In
J.R. Anderson (Ed.), Cognitive Skills and Their Acquisition Hillsdale,
N.J.: Lawrence Earlbaum Associates. pp. 335-360.
Schustack, M., & Anderson, J. R. (1979). Effects of analogy to prior knowledge
on memory for new information. Journal of Verbal Learning and Verbal
Behavior, 18, 565-583.
Sembugamoorthy, V. (August, 1981). Analogy-based acquisition of utterances
relating to temporal aspects. Proceedings of the 7th International Joint
Conference on Artificial Intelligence. pp. 106-108.
Shrager, J. & Klahr, D. (December, 1983). A Model of Learning in the
Instructionless Environment. Proceedings of the Conference on Human
Factors in Computing Systems. pp. 226-229.
Shrager, J. & Klahr, D. Instructionless Learning: Hypothesis Generation and
Experimental Performance. In preparation.
Sternberg, R. (1977). Intelligence, information processing, and analogical
reasoning: The componential analysis of human abilities. Hillsdale,
N.J.: Lawrence Erlbaum Associates.
VanLehn, K., & Brown, J. S. (1978). Planning nets: A representation for
formalizing analogies and semantic models of procedural skills. In Snow,
R. E., Frederico, P. A. and Montague, W. E. (Ed.), Aptitude Learning and
Instruction: Cognitive Process Analyses Hillsdale, NJ: Lawrence Erlbaum
Associates.
Weiner, E. J. A Computational Approach to Metaphore Comprehension. In the
Penn Review of Linguistics.
Winston, P. H. (December, 1980). Learning and Reasoning by Analogy.
Communications of the ACM, 23(12), 689-703.
Winston, P. H. Learning and Reasoning by Analogy: The details. MIT AI Memo
number 520.
------------------------------
Date: Fri, 21 Jun 85 11:42:26 pdt
From: gabor!amyjo@RIACS.ARPA (Amy Jo Bilson)
Subject: Seminar - Evaluating Expert Forecasts (NASA)
NASA
PERCEPTION AND COGNITION SEMINARS
Who: Keith Levi
From: University of Michigan
When: 10 am, Tuesday, June 25, 1985
Where: Room 177, Building 239, NASA Ames Research Center
What: Evaluating Expert Forecasts
Abstract: Probabilistic forecasts, often generated by an expert,
are critical to many decision aids and expert systems.
The quality of such inputs has usually been evaluated in
terms of logical consistency. However, in terms of
real-world implications, the external correspondence of
probabilistic forecasts is usually much more important
than internal consistency. I will discuss recently
developed procedures for evaluating external correspondence
and present research on the topic.
Non-citizens (except permanent residents) must have prior approval from
the Directors Office one week in advance. Permanent residents must show
Alien Registration Card at the time of registration.
To request approval or obtain further information, call 415-694-6584.
------------------------------
End of AIList Digest
********************