Copy Link
Add to Bookmark
Report
AIList Digest Volume 1 Issue 102
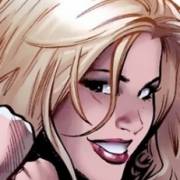
AIList Digest Tuesday, 22 Nov 1983 Volume 1 : Issue 102
Today's Topics:
AI and Society - Expert Systems,
Scientific Method - Psychology,
Architectures - Need for Novelty,
AI - Response to Challenge
----------------------------------------------------------------------
Date: 20 Nov 83 14:50:23-PST (Sun)
From: harpo!floyd!clyde!akgua!psuvax!simon @ Ucb-Vax
Subject: Re: just a reminder... - (nf)
Article-I.D.: psuvax.357
It seems a little dangerous "to send machines where doctors won't go" -
you'll get the machines treating the poor, and human experts for the privileged
few.
Also, expert systems for economics and social science, to help us would be fine,
if there was a convincing argument that a)these social sciences are truly
helpful for coping with unpredictable technological change, and b) that there
is a sufficiently accepted basis of quantifiable knowledge to put in the
proposed systems.
janos simon
------------------------------
Date: Mon, 21 Nov 1983 15:24 EST
From: MONTALVO%MIT-OZ@MIT-MC.ARPA
Subject: I recall Rational Psychology
Date: 17 Nov 83 13:50:54-PST (Thu)
From: ihnp4!houxm!mhuxl!ulysses!unc!mcnc!ncsu!fostel @ Ucb-Vax
Subject: I recall Rational Psychology
... Proper scientific method is very
hard to apply in the face of stunning lack of understanding or hard,
testable theories. Most proper experiments are morally unacceptable in
the pschological arena. As it is, there are so many controls not done,
so many sources of artifact, so much use of statistics to try to ferret
out hoped-for correlations, so much unavoidable anthropomorphism. As with
scholars such as H. Dumpty, you can define "science" to mean what you like,
but I think most psychological work fails the test.
----GaryFostel----
You don't seem to be aware of Experimental Psychology, which involves
subjects' consent, proper controls, hypothesis formation and
evaluation, and statistical validation. Most of it involves sensory
processes and learning. The studies are very rigorous and must be so
to end up in the literature. You may be thinking of Clinical Psychology.
If so, please don't lump all of Psychology into the same group.
Fanya Montalvo
------------------------------
Date: 19 Nov 83 11:15:50-PST (Sat)
From: decvax!tektronix!ucbcad!notes @ Ucb-Vax
Subject: Re: parallelism vs. novel architecture - (nf)
Article-I.D.: ucbcad.835
Re: parallelism and fundamental discoveries
The stored-program concept (Von Neumann machine) was indeed a breakthrough
both in the sense of Turing (what is theoretically computable) and in the
sense of Von Neuman (what is a practical machine). It is noteworthy,
however, that I am typing this message using a text editor with a segment
of memory devoted to program, another segment devoted to data, and with an
understanding on the part of the operating system that if the editor were
to try to alter one of its own instructions, the operating system should
treat this as pathological, and abort it.
In other words, the vaunted power of being able to write data that can be
executed as a program is treated in the most stilted and circumspect manner
in the interests of practicality. It has been found to be impractical to
write programs that modify their own inner workings. Yet people do this to
their own consciousness all the time--in a largely unconscious way.
Turing-computability is perhaps a necessary condition for intelligence.
(That's been beaten to death here.) What is needed is a sufficient condition.
Can that possibly be a single breakthrough or innovation? There is no
question that, working from the agenda for AI that was so hubristically
layed out in the 50's and 60's, such a breakthrough is long overdue. Who
sees any intimation of it now?
Perhaps what is needed is a different kind of AI researcher. New ground
is hard to break, and harder still when the usual academic tendency is to
till old soil until it is exhausted. I find it interesting that many of
the new ideas in AI are coming from outside the U.S. AI establishment
(MIT, CMU, Stanford, mainly). Logic programming seems largely to be a
product of the English-speaking world *apart* from the U.S. Douglas
Hofstadter's ideas (though probably too optimistic) are at least a sign
that, after all these years, some people find the problem too important
to be left to the experts. Tally Ho! Maybe AI needs a nut with the
undaunted style of a Nicola Tesla.
Some important AI people say that Hofstadter's schemes can't work. This
makes me think of the story about the young 19th century physicist, whose
paper was reviewed and rejected as meaningless by 50 prominent physicists
of the time. The 51st was Maxwell, who had it published immediately.
Michael Turner (ucbvax!ucbesvax.turner)
------------------------------
Date: 20 November 1983 2359-PST (Sunday)
From: helly at AEROSPACE (John Helly)
Subject: Challenge
I am responding to Ralph Johnson's recent submittal concerning the
content and contribution of work in the field of AI. The following
comments should be evaluated in light of the fact that I am currently
developing an 'expert system' as a dissertation topic at UCLA.
My immediate reaction to Johnson's queries/criticisms of AI is that of
hearty agreement. Having read a great deal of AI literature, my
personal bias is that there is a great deal of rediscovery of Knuth in
the context of new applications. The only things apparently unique are
that each new 'discovery' carries with it a novel jargon with very
little attempt to connect and build on previous work in the field. This
reflects a broader concern I have with Computer Science in general in
that, having been previously trained as a biologist, I find very little
that I consider scientific in this field. This does not diminish my
hope for, and consequently my commitment to, work in this area.
Like many things, this commitment is based on my intuition (read faith)
that there really is something of value in this field. The only
rationale I can offer for such a commitment is the presumption that the
lack of progress in AI research is the result of the lack of scientific
discipline of AI researchers and computer scientists in general. The AI
community looks much more like a heterogeneous population of hackers than
that of a disciplined, scientific community. Maybe this is symptomatic
of a new field of science going through growing pains but I do not
personally believe this is the case. I am unaware of any similar
developmental process in the history of science.
This all sounds pretty negative, I know. I believe that criticism
should always be stated with some possible corrective action, though,
and maybe I have some. Computer science curricula should require formal
scientific training. Exposure to truly empirical sciences would serve
to familiarize students with the value of systematic research,
experimental design, hypothesis testing and the like. We should find
ways to apply the scientific method to our research rather than
collecting a lot of anecdotal information about our 'programming
environment' and 'heuristics' and publishing it at first light.
Maybe the computer science is basically an engineering discipline (i.e.,
application-oriented) rather than a science. I believe, however, that
in the least computer science, even if misnamed, offers powerful tools
for investigating human information processing (i.e, intelligence) if
approached scientifically. Properly applied these tools can provide the
same benefits they have offered physicists, biologists and medical
researchers - insight into mechanisms and techniques for simulating the
systems of interest.
Much of AI is very slick programming. I'm just not certain that it is
anything more than that, at least at present.
------------------------------
Date: Mon 21 Nov 83 14:12:35-PST
From: Tom Dietterich <DIETTERICH@SUMEX-AIM.ARPA>
Subject: Reply to Ralph Johnson
Your recent msg to AILIST was certainly provocative, and I thought I'd
try to reply to a couple of the points you made. First, I'm a little
appalled at what you portray as the "Cornell" attitude towards AI. I
hope things will improve there in the future. Maybe I can contribute
a little by trying to persuade you that AI has substance.
I'd like to begin by calling attention to the criteria that you are
using to evaluate AI. I believe that if you applied these same
criteria to other areas of computer science, you would find them
lacking also. For example, you say that "While research in AI has
produced many results in side areas..., none of the past promises of
AI have been fulfilled." If we look at other fields of computer
science, we find similar difficulties. Computer science has promised
secure, reliable, user-friendly computing facilities, cheap and robust
distributed systems, integrated software tools. But what do we have?
Well, we have some terrific prototypes in research labs, but the rest
of the world is still struggling with miserable computing
environments, systems that constantly crash, and distributed systems
that end up being extremely expensive and unreliable.
The problem with this perspective is that it is not fair to judge a
research discipline by the success of its applications. In AI
research labs, AI has delivered on many of its early promises. We now
have machines with limited visual and manipulative capabilities. And
we do have systems that perform automatic language translation (e.g.,
at Texas).
Another difficulty of judging AI is that it is a "residual"
discipline. As Avron Barr wrote in the introduction to the AI
Handbook, "The realization that the detailed steps of almost all
intelligent human activity were unknown marked the beginning of
Artificial Intelligence as a separate part of computer science." AI
tackles the hardest application problems around: those problems whose
solution is not understood. The rest of computer science is primarily
concerned with finding optimum points along various solution
dimensions such as speed, memory requirements, user interface
facilities, etc. We already knew HOW to sort numbers before we had
computers. The role of Computer Science was to determine how to sort
them quickly and efficiently using a computer. But, we didn't know
HOW to understand language (at least not at a detailed level). AI's
task has been to find solutions to these kinds of problems.
Since AI has tackled the most difficult problems, it is not surprising
that it has had only moderate success so far. The bright side of
this, however, is that long after we have figured out whether P=NP, AI
will still be uncovering fascinating and difficult problems. That's
why I study it.
You are correct in saying that the AI literature is hard to read. I
think there are several reasons for this. First, there is a very
large amount of terminology to master in AI. Second, there are great
differences in methodology. There is no general agreement within the
AI community about what the hard problems are and how they should be
addressed (although I think this is changing). Good luck with any
further reading that you attempt.
Now let me address some of your specific observations about AI. You
say "I already knew a large percentage of the algorithms. I did not
even think of most of them as being AI algorithms." I would certainly
agree. I cite this as evidence that there is a unity to all parts of
computer science, including AI. You also say "An expert system seems
to be a program that uses a lot of problem-related hacks to usually
come up with the right answer." I think you have hit upon the key
lesson that AI learned in the seventies: The solution to many of the
problems we attack in AI lies NOT in the algorithms but in the
knowledge. That lesson reflects itself, not so much in differences in
code, but in differences in methodology. Expert systems are different
and important because they are built using a programming style that
emphasizes flexibility, transparency, and rapid prototyping over
efficiency. You say "There seems to be nothing more to expert systems
than to other complicated programs". I disagree completely. Expert
systems can be built, debugged, and maintained more cheaply than other
complicated programs. And hence, they can be targeted at applications
for which previous technology was barely adequate. Expert systems
(knowledge programming) techniques continue the revolution in
programming that was started with higher-level languages and furthered
by structured programming and object-oriented programming.
Your view of "knowledge representations" as being identical with data
structures reveals a fundamental misunderstanding of the knowledge vs.
algorithms point. Most AI programs employ very simple data structures
(e.g., record structures, graphs, trees). Why, I'll bet there's not a
single AI program that uses leftist-trees or binomial queues! But, it
is the WAY that these data structures are employed that counts. For
example, in many AI systems, we use record structures that we call
"schemas" or "frames" to represent domain concepts. This is
uninteresting. But what is interesting is that we have learned that
certain distinctions are critical, such as the distinction between a
subset of a set and an element of a set. Or the distinction between a
causal agent of a disease (e.g., a bacterium) and a feature that is
helpful in guiding diagnosis (e.g., whether or not the patient has
been hospitalized). Much of AI is engaged in finding and cataloging
these distinctions and demonstrating their value in simplifying the
construction of expert systems.
In your message, you gave five possible answers that you expected to
receive. I guess mine doesn't fit any of your categories. I think
you have been quite perceptive in your analysis of AI. But you are
still looking at AI from the "algorithm" point of view. If you shift
to the "knowledge" perspective, your criteria for evaluating AI will
shift as well, and I think you will find the field to be much more
interesting.
--Tom Dietterich
------------------------------
Date: 22 Nov 83 11:45:30 EST (Tue)
From: rej@Cornell (Ralph Johnson)
Subject: Clarifying my "AI Challange"
I am sorry to create the mistaken impression that I don't think AI should
be done or is worth the money we spend on it. The side effects alone are
worth much more than has been spent. I do understand the effects of AI on
other areas of CS. Even though going to the moon brought no direct benefit
to the US outside of prestige (which, by the way, was enormous), we learned
a lot that was very worthwhile. Planetary scientists point out that we
would have learned a lot more if we had spent the money directly on planetary
exploration, but the moon race captured the hearts of the public and allowed
the money to be spent on space instead of bombs. In a similar way, AI
provides a common area for some of our brightest people to tackle very hard
problems, and consequently learn a great deal. My question, though, is
whether AI is really going to change the world any more than the rest of
computer science is already doing. Are the great promises of AI going to
be fulfilled?
I am thankful for the comments on expert systems. Following these lines of
reasoning, expert systems are differentiated from other programs more by the
programming methodology used than by algorithms or data structures. It is
very helpful to have these distinctions pointed out, and has made several
ideas clearer to me.
The ideas in AI are not really any more difficult than those in other areas
of CS, they are just more poorly explained. Several times I have run in to
someone who can explain well the work that he/she has been doing, and each
time I understand what they are doing. Consequently, I believe that the
reason that I see few descriptions of how systems work is because the
designers are not sure how they work, or they do not know what is important
in explaining how they work, or they do not know that it is important to
explain how they work. Are they, in fact, describing how they work, and I
just don't notice? What I would like is more examples of systems that work,
descriptions of how they work, and of how well they work.
Ralph Johnson (rej@cornell, cornell!rej)
------------------------------
Date: Tue 22 Nov 83 09:25:52-PST
From: Tom Dietterich <DIETTERICH@SUMEX-AIM.ARPA>
Subject: Re: Clarifying my "AI Challange"
Ralph,
I can think of a couple of reasons why articles describing Expert
Systems are difficult to follow. First, these programs are often
immense. It would take a book to describe all of the system and how
it works. Hence, AI authors try to pick out a few key things that
they think were essential in getting the system to work. It is kind
of like reading descriptions of operating systems. Second, the lesson
that knowledge is more important than algorithm has still not been
totally accepted within AI. Many people tend to describe their
systems by describing the architecture (ie., the algorithms and data
structures) instead of the knowledge. The result is that the reader
is left saying "Yes, of course I understand how backward chaining (or
an agenda system) works, but I still don't understand how it diagnoses
soybean diseases..." The HEARSAY people are particularly guilty of
this. Also, Lenat's dissertation includes much more discussion of
architecture than of knowledge. It often takes many years before
someone publishes a good analysis of the structure of the knowledge
underlying the expert performance of the system. A good example is
Bill Clancey's work analyzing the MYCIN system. See his most recent
AI Journal paper.
--Tom
------------------------------
End of AIList Digest
********************