Copy Link
Add to Bookmark
Report
Neuron Digest Volume 12 Number 11
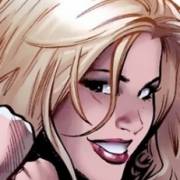
Neuron Digest Monday, 8 Nov 1993 Volume 12 : Issue 11
Today's Topics:
Proceedings available
Possible position in Finance
UK PhD connectionist studentship available
Courses
Summary of ANNs and secondary protein structures pt 1
Summary of ANNs and secondary protein structures pt 2
Send submissions, questions, address maintenance, and requests for old
issues to "neuron-request@psych.upenn.edu". The ftp archives are
available from psych.upenn.edu (130.91.68.31). Back issues requested by
mail will eventually be sent, but may take a while.
----------------------------------------------------------------------
Subject: Proceedings available
From: Joan Cabestany <cabestan@eel.upc.es>
Date: Tue, 19 Oct 93 09:59:16 -0300
Proceedings available
_____________________
After the last edition of IWANN'93 (International Workshop on Artificial
Neural Networks) held in Spain (Sitges) during June 1993, some books with
the Proceedings are still available at special price.
Reference:
New Trends in Neural Computation (IWANN'93 Proceedings)
J.Mira, J.Cabestany, A.Prieto editors
Lecture Notes in Computer Science number 686
SPRINGER VERLAG 1993
Price: 9000 pesetas (spanish currency)
Method of payment:
VISA Card number _________________________ Expiration date _______________
Name of card holder ______________________________________________________
Date ____________ Signature ___________________________________
Send this form to
ULTRAMAR CONGRESS
Att. Mr. J.Balada
Diputacio, 238, 3
08007 BARCELONA Spain
Fax + 34.3.412.03.19
------------------------------
Subject: Possible position in Finance
From: William Fulkerson <fulkersw@smtplink.de.deere.com>
Date: Tue, 19 Oct 93 09:11:36 -0600
A position is anticipated in the Finance department, Deere &
Company, Moline Illinois. The purpose of this message is to
survey the interest in such a position and to determine the
skills available among the likely candidates.
The successful candidate will have a theoretical background and 1
to 3 years professional experience in applications of
neural networks, evolutionary computing, and/or fuzzy logic.
Although professional experience in applications is required, it
need not be in finance. Applicants with advanced degrees in
engineering, statistics, or computer science are preferred.
The applicant must want to apply the above technologies to
financial problems and be willing to pursue these financial
applications for an extended period of years. The initial
assignment will be to develop trading systems for foreign
exchange and commercial paper. Future assignments will be within
the Finance department and may include pension fund management.
If your interest, application experience, training, and skills
match this description, please send a short description of your
qualifications via e-mail to:
fulkersw@smtplink.de.deere.com.
Receipt of your e-mail will be acknowledged.
------------------------------
Subject: UK PhD connectionist studentship available
From: simon@dcs.ex.ac.uk
Date: Tue, 19 Oct 93 16:23:58 +0000
PhD Studentship -- full-time, SERC funded
The Department of Computer Science at the University of Exeter has
a SERC quota award available for a suitable candidate to pursue
fulltime research for a PhD degree. Applicants should have a good
first degree in Computer Science (or a closely related dsicipline)
with a sound knowledge of neural computing and/or software
engineering. The successful applicant will join a research group
exploring the use of neural computing as a novel software
technology. Potential projects range from formal analysis of
network implementations of well-defined problems to development of
visualization techniques to facilitate efficient network training
as well as to provide support for a conceptual understanding of
neural net implementations.
Application forms and further information can be obtained from:
Lyn Shackleton,
Department of Computer Science,
University of Exeter,
Exeter EX4 4PT.
email: lyn@dcs.exeter.ac.uk;
tel: 0392 264066; FAX: 0392 264067
Informal enquiries and requests for further details of the
research group's activities may be made to:
Professor Derek Partridge,
Department of Computer Science,
University of Exeter,
Exeter EX4 4PT,
email: derek@dcs.exeter.ac.uk
tel: 0392 264061, FAX: 0392 264067,
The closing date for applications is November 19th, 1993.
Interviews will be conducted in the week beginning November 22, 1993.
It is expected that the award will be taken up in January 1994.
- --
Simon Klyne Connection Science Laboratory
email: simon@dcs.exeter.ac.uk Department of Computer Science
phone: (+44) 392 264066 University of Exeter
EX4 4QE, UK.
------------------------------
Subject: Courses
From: RAUL HECTOR GALLARD <gallardr@unslfm.edu.ar>
Date: Wed, 20 Oct 93 10:21:27 -0300
Dear colleague:
This e-mail is to ask for assistance to rise up the background of lecturers
and researchers, in specific areas.
We belong to the Department of Informatics of the Universidad Nacional de
San Luis, Argentina, where we have a Computer Science carreer. Besides that,
we conform a research group working in the area of Intelligent Distributed
Systems. In our project we are looking for applications of Genetic Algorithms
(GAs) and Neural Networks (NNs), to insert intelligence into Computer Systems.
A PhD Programme was launched in the areas of GAs and NNs for further study and
research in these topics.
Our Faculty is looking for Professors and/or Researchers who whish to come to
San Luis to give a short postgraduate course (40 to 80 hours) on GAs and NNs.
The stay is stimated in three weeks, and the courses could be given anytime
before October 1994. Travelling and reasonable living expenses will be covered
by the Faculty.
We need to have as soon as possible a brief Curriculum Vitae and course
content (current trends are advisable) of the possible candidates.
If you are prepared to give any of those courses please contact me via e-mail
or to the fax number 54 652 30224. Otherwise, if you know another colleague
willing to come please forward this message to him.
Thanking you in advance.
Prof. Raul Gallard
Proyecto 338403
Universidad Nacional de San Luis
Ej. de los Andes 950
5700 - san Luis
Argentina
e-mail : gallardr@unslfm.edu.ar
Raul Hector Gallard
gallardr@unslfm.edu.ar
------------------------------
Subject: Summary of ANNs and secondary protein structures pt 1
From: pb@cse.iitb.ernet.in (Pushpak Bhattacharya)
Date: Thu, 21 Oct 93 12:43:21 +0700
Here are some of the responses we received to our query
on prediction of secondary structure of protein. Thanks
very much to all the respondents.
Pushpak Bhattacharyya
pb@cse.iitb.ernet.in
Susmita De
susmita@cse.iitb.ernet.in
>From ajay@cgl.ucsf.edu
Hi,
Saw your note in neuron-digest. i am very surprised that you have
problems running this. i have never had any problems running the protein
structure prediction problem (in fact this is my research area) using
a BP though not the PDP software.
i guess that the problem is with the code or the specification of
the various bells and whistles with the code. Saturation problems occur
if you use LARGE learning rates. Before you go around messing with the code
i would suggest using a very small learning rate say 0.001, no momentum and
try both per-pattern and batch modes. i have found quicker convergence
with per-pattern updates.
Hope this helps.
---ajay
>From batra@zelia.Colorado.EDU Fri Oct 15 20:05:07 1993
Here are some refs (if you dont have them already)
Qian, N. and Sejnowski, T.J. (1988). Predicting the Secondary
Structure of Globular Proteins Using Neural Network Models. {\em
Journal of Molecular Biology,} 202:865-884.
Holley, L.H. and Karplus, M. (1989). Protein Secondary Structure
Prediction with a Neural Network. {\em Proceedings of the National
Academy fo Science USA,} 86:152-156.
Threre are many references on this topic but these might be the
better ones.
- --SAjeev.
>From batra@zelia.Colorado.EDU Fri Oct 15 20:05:05 1993
Hi,
I'm also working on Protein Secondary Structure Prediction... So,
I might be able to help.
I'm not exactly sure what your problem is... However, first
you should try the problem without a hidden layer in the network.
Qian and Sejnowski do very well w/o the hidden layer.
Having 20 neurons is too many. You also mention that you
have 65 input units??? Hmmm... I think if you use 21xN, it
might work better. N is the size of your sliding window (usually
N= 13).
Hope I've helped a little. If you need more info or anything,
please do not hesitate to email me. BTW hope this gets thru to
india.
Regards,
Sajeev Batra
batra@boulder.colorado.edu
>From ajay@cgl.ucsf.edu Tue Oct 12 13:28:57 1993
Hi,
Saw your note in neuron-digest. i am very surprised that you have
problems running this. i have never had any problems running the protein
structure prediction problem (in fact this is my research area) using
a BP though not the PDP software.
i guess that the problem is with the code or the specification of
the various bells and whistles with the code. Saturation problems occur
if you use LARGE learning rates. Before you go around messing with the code
i would suggest using a very small learning rate say 0.001, no momentum and
try both per-pattern and batch modes. i have found quicker convergence
with per-pattern updates.
Hope this helps.
---ajay
>From reinagel@husc.harvard.edu Tue Oct 12 13:31:47 1993
Hi.
You posted in the NN digest about a problem you're having with bp
solving protein folding, which you attribute to the size of your data
set. As it happens, I also once (long ago) built a bp net to predict
protein folding, (actually millions of people have done it, it seems).
So I am interested in your problem.
I train nets on data sets of 20,000 patterns (40 inputs, 1 output,
and 5 to 20 hidden U's) all the time and I have NEVER seen the behaviour
you describe. I don't think your problem is due to too big a data set,
but due to a bad representation of the data or to bad net architecture.
Have you tested that bp in your hands behaves normally for, eg., XOR?
What happens if you train on just 100 patterns? Same thing?
You say your net has 65 binary inputs--how is the primary sequence
coded??! The three outputs are? Alpha, beta, other? 3-D spatial
coordinates? Phi, Psi, & something else? I am having trouble imagining
how a sufficient amount of primary sequence could ever be represented
in 65 bits, nor where you can find 8,000 independent sequences of known
structure, unless it is that you're using way to few aa's, or way too
little information about each aa, to specify structure.
I'm asking because it's possible that your input codes carry NO
information about your output codes, and even BP cannot extract
information that is not there. In such a case, the net will train to
learn intrinsic regularities in the output patterns independent of the
inputs--eg. if the outputs are so coded that the unit's target is 0
most of the time, the net will learn that just guessing 0 minimizes
its MSE.
If you are convinced that the information is there to be found, try:
1. incremental training (start with a few patterns and add more and
more) 2. heirarchy (make a net to remap inputs to an intermediary code
and then another net to go from that code to the final one) if you
know already of an intermediary representation that makes sense 3. try
fewer hidden units or local connectivity of hidden units, at least in
the early part of training.
Good luck.
Pam Reinagel
Harvard University
------------------------------
Subject: Summary of ANNs and secondary protein structures pt 2
From: pb@cse.iitb.ernet.in (Pushpak Bhattacharya)
Date: Thu, 21 Oct 93 12:48:42 +0700
Here are some more reponses to our query on the
prediction of secondery structures of proteins by
neural nets. We post it to the neuron-request for
the benefit of fellow-researchers. Thanks very much
again to the respondents.
Pushpak Bhattacharyya
pb@cse.iitb.ernet.in
Susmita De
susmita@cse.iitb.ernet.in
>From markb@orl.mmc.com Fri Oct 8 01:28:03 1993
I doubt your problems have much to do with the size of your problem
as I routinely train BP classifiers on data sets of 8,000 examples using
nets roughly the size of 65x20x3 with great success.
More likely is some little snag with how you are presenting data to the net
and so I am going to go through how you stated your problem. Please let me
know if any of the following is in error. You say you present the net with
the primary sequence of the protein and it has 65 binary values. I assume
you are encoding the amino acids acids in some way ( 20 amino acids requires
5 bits to represent ) so I assume you are working on a sequence of length
13; i.e. 65/5. You have then chosen 20 hidden nodes ( because there are 20
amino acids? ) and finally have some data which suggests these 13 residues
have three distinct conformations. Question: how do you assign the training
values? Like
Conformation 1: 1.0 0.0 0.0
Conformation 2: 0.0 1.0 0.0
Conformation 3: 0.0 0.0 1.0
or do you use 0.9 and 0.1? i.e.
Conformation 1: 0.9 0.1 0.1
Conformation 2: 0.1 0.9 0.1
Conformation 3: 0.1 0.1 0.9
a related question would be do you use a sigmoid or other squashing function
on the output layer of nodes?
Finally, are the three conformations presented to the net in random order or
do you present the BP net with all of the Conformation 1 examples, then all of
the Conformation 2 examples, ...?
I would suggest:
1) making sure you always put the 'N-terminal' residue at the same spot
2) putting a squashing function at the output
3) putting "teacher values" of 0.9 and 0.1 one the training examples
4) presenting the examples in random order
Finally, make sure you
1) set the learning constant sufficiently low so it does not "peg"
initial movements to either 1 or 0
2) include the "bias nodes".
I would absolutely LOVE to see your data! I have worked BP nets as classifiers
for years, but have no access to protein data
Mark.
&&&&&&&&&&&&&&&&&&&&&&&&&&&&&&&&
>From may@apple.com Fri Oct 8 09:31:38 1993
> We are trying to train a BP neural net (PDP-3 package of Rumelhart and
> Mclleland is being used) with the input as the primary structure and the
> output as the conformational state of the amino acids. The net has 65
> binary inputs and 3 binary outputs. The number of patterns to be trained
> are more than eight thousand. We have 20 neurons in the hidden layer.
>
> Now, just after one epoch the hidden neurons go into saturation state -
> all their outputs are 1. At the same time the output neurons also go into
> saturation. All their outputs become 0. We understand that the primary
> reason for this is that A LARGE number of patterns are being trained on a
> net with large no of inputs. The weights to the o/p layer keep on getting
> the same kind of "Credit", so that their values go on decreasing to large
> negative values.
>
> Could anybody suggest a way out of this difficulty - which to our mind is
> a result of applying BP on a large-size real life problem ? (those
> applying BP on large problems will appreciate our difficulty !).
Hi,
If you're allowed to release your training set I'd be happy to run
it through my own backprop simulator and send you the results (structure
and weights). I can also send you the code when I've polished the user-
interface (probably another man-month of work).
Regards,
Patrick May
may@apple.com
>From /PN=luca/OU=aeolus/O=ethz/PRMD=switch/ADMD=arcom/C=ch/@x400-gate.iitb.ernet.in Fri Oct 8 10:21:02 1993
Dear Sirs,
I have read your post on neural network and your current problems.
I am fiishing a PH.D. in protein crystallography ,and I am interested
into the applicationof neural network pattern recognition on prediction
of proteins structures. I used it as well in classical crystallographical
problems, since 2 years.
I suppose you are all well aware about the work done by Cotteril few
years ago about threedimensional protein structure prediction appeared
on a small paper on FEBS. I do not remeba the exact reference but I will
look up in the lab tomorrow ( I know I have it ), if you haven't yet read
it. I also worked on topological approach to predict conformations of
aminoacids.
My opinion on the problem as you posed is that it is ill-posed.
That is: 1) are you really SURE that you posed the problem in the more
straight way ? 2) 8000 patterns are not much compared to the 1400 weights
that the net has to solve , are you sure that they are enough ?
I never had problems in making a net converging and generalyze, when I
posed the problem in the right way ...
I applyed my problem to 20000 (twenty thousands) neurons as input layer,
each input being varying between 0. and 1. and using sigmoidal function
as first step, 5000 hiddens and 20000 (twenty thousands) outputs. I was
running it on a CRAY-YMP and then lastly on a faster machine capable of
storing up to 30 GigaBytes in Memory (NEC-SX-3) and had never the pro-
blems you show.
therefore I suppose that the problem you are encountering is not due to
the size of the problem but of the way you designed it ...
neural networks have nothing magic ! all the business is to use them
correctly and in an approprate way ....
OK ?
in case I can be of any help, give me a ring :-)
Luca
luca@aeolus.ethz.ch
>From stein@mars.fiz.huji.ac.il Fri Oct 8 14:12:14 1993
> We are trying to train a BP neural net (PDP-3 package of Rumelhart and
> Mclleland is being used) with the input as the primary structure and the
> output as the conformational state of the amino acids. The net has 65
> binary inputs and 3 binary outputs. The number of patterns to be trained
> are more than eight thousand. We have 20 neurons in the hidden layer.
> Now, just after one epoch the hidden neurons go into saturation state -
> all their outputs are 1. At the same time the output neurons also go into
> saturation. All their outputs become 0. We understand that the primary
> reason for this is that A LARGE number of patterns are being trained on a
> net with large no of inputs. The weights to the o/p layer keep on getting
> the same kind of "Credit", so that their values go on decreasing to large
> negative values.
> Could anybody suggest a way out of this difficulty - which to our mind is
> a result of applying BP on a large-size real life problem ? (those
> applying BP on large problems will appreciate our difficulty !).
Your problem does not really classify as a large-size problem with 65
inputs and 3 outputs. We routinely handle hundreds of inputs and tens
of outputs with no saturation problems. I suggest that you consult our
article that appeared in IJCNN-92 (Baltimore) I-932 for some tips.
First, make sure that your binary inputs are represented by -1 and +1
and NOT by 0 and 1. Otherwise you will have to deal with the derivative
becoming zero by one of several techniques (see references in our article).
Next you are probably being drawn into a global attractor, to check this
try stretching your sigmoid to pull the values out of saturation and
continue training. If these don't help drop me an email.
Dr. Yaakov Stein
Neural Network Group
Efrat Future Technology
Tel Aviv, ISRAEL
>From FLOTZI@dpmi.tu-graz.ac.at Fri Oct 8 14:45:45 1993
Hi!
I read about your problems with your backprop application with 65
binary inputs and 3 binary outputs. I have had problems with
backprop, too, and although I don't know the package you are using I
can think of some reasons why the nodes go into saturation so quickly:
1) the learning rate may be too large: depending on the implementation
and on whether you use a momentum term or not you should use quite
small values like 0.001 or even smaller, look at the error curve to find
out if that is too small.
2) I guess you use batch learning, i.e. you look at all training examples
before changing the weights. I have found that this is not so useful if
the patterns are not well seperable. I prefer 'on-line' learning, i.e.
changing the weights after each training example. Unfortunately, with
'on-line' learning, the momentum technique is not applicable, but I don't
know whether you use it or not.
3) try using more than 20 hidden nodes, but I must confess I would
have started with about the same number.
I hope my suggestions will be of help
Good luck
Doris Flotzinger
Medical Informatics
Technical University Graz / Austria
>From iain.strachan@aea.orgn.uk Fri Oct 8 18:58:31 1993
I read your query in neuroprose, and have a few suggestions that might
help. If you've already tried this, please ignore.
(1) The most common cause of premature saturation is setting of the
initial values of the weights too high. If the expectation value of
the input to any sigmoid unit is greater than about 3.5, then the
slope of the sigmoid is 0.1 times the slope at value zero, and hence
learning is very slow. A useful heuristic is to determine a basic
range for the weights (say -1 to 1) and then to scale by the inverse
of the square root of the number of inputs to the particular unit. If
the problem still persists, reduce the initial range even further.
(2) A good discussion of premature saturation is given in:
"An analysis of Premature Saturation in Back Propagation
Learning".
Lee,Oh and Kim, Neural Networks Vol 6(1993) pps 719-728.
(3) If the learning rate is too high, this can also immediately cause
the neurons to go into saturation.
- ------------------------------------------------------------------------------
Mr. Iain G. D. Strachan iain.strachan@aea.orgn.uk
Tel: +44 (0)235 435321
Fax: +44 (0)235 432726
AEA Technology
Applied Neurocomputing Centre
B521.1 Harwell
Oxfordshire
OX11 0RA
UK
- ------------------------------------------------------------------------------
>From /PN=jmerelo/O=ugr/PRMD=iris/ADMD=mensatex/C=es/@x400-gate.iitb.ernet.in Fri Oct 8 22:19:41 1993
Hi, this is JJ Merelo, from Spain...
I don't know if you have checked the literature, but just in case, there
is a classical article by Qian and Sejnowski, as well as new papers
by people at the EMBL that achieve just what you're looking for. They
don't manage to achieve more than a 70% of accuracy, from protein sequence
to secondary structure.
Several other approaches haven been tried; for instande, ours (Andrade
et al, that has appeared in Protein Engineering recently), which tries
to predict secondary structure from circular dichroism spectra, achieving
somewhat better results.
Hope this helps,
JJ
>From drt@genesis.mcs.com Sat Oct 9 19:42:59 1993
Pushpak, you wrote in Neuron Digest:
>We are trying to train a BP neural net (PDP-3 package of Rumelhart and
>Mclleland is being used) with the input as the primary structure and the
>output as the conformational state of the amino acids. The net has 65
>binary inputs and 3 binary outputs. The number of patterns to be trained
>are more than eight thousand. We have 20 neurons in the hidden layer.
>
>Now, just after one epoch the hidden neurons go into saturation state -
>all their outputs are 1. At the same time the output neurons also go into
>saturation. All their outputs become 0. We understand that the primary
>reason for this is that A LARGE number of patterns are being trained on a
>net with large no of inputs. The weights to the o/p layer keep on getting
>the same kind of "Credit", so that their values go on decreasing to large
>negative values.
>
>Could anybody suggest a way out of this difficulty - which to our mind is
>a result of applying BP on a large-size real life problem ? (those
>applying BP on large problems will appreciate our difficulty !).
The easiet solution is to change all those binary 1s in the input
layer to smaller values, like 0.1. A harder way to do this
is to change the activation function from:
1 / (1 + exp(-x))
to:
1 / (1 + exp(-D*x))
and make D less than 1. This require modifying the code but then I
guess you have it so you can do it. While harder, this is more
convenient because you can keep changing D (called the gain or
sharpness) until you get good results.
Also you will probably want to use very small initial random weights.
But I'll give you some other good advice too: try some of the newer
variations on backprop like delta-bar-delta and quickprop. But I'll
give you a caution about this too: I was recently puttering around
with the sonar data using backprop and quickprop and the backprop
network was generalizing better than quickprop. Another trick is
to fudge the derivative term for the output units only so instead of
using s(1-s) where s is the value of the output unit use 0.1 + s(1-s)
or just plain 1. Given that you have 3 binary outputs I think this
may help because I bet some of them will get stuck at the wrong values
and when they do the normal s(1-s) term slows learning down by A LOT.
Finally I could offer you my backprop code that happens to do these
things. I've posted the backprop source in C for UNIX and DOS in
comp.sources.misc a number of times and people around the world have
used it and liked it. Its free for ordinary uses, $200 for businesses
and government agencies, if you find it useful. In case you find it
useful you get the new improved "professional" version as well.
Don Tveter
5228 N. Nashville Ave.
Chicago, Illinois 60656-2233
USA
drt@genesis.mcs.com
>From huyser@nova3.Stanford.EDU Sun Oct 10 04:27:23 1993
Dear Drs. Bhattacharyya and De,
I should think your learning rate is too large. I would try batch learning
(one step per epoch on the accumulated errors) and a step-size (lrate) of
roughly 0.1/npatterns, which for you is about 1e-5. It's possible that
1e-4 would still be stable; it depends on the data.
Best wishes,
Karen Huyser
------------------------------
End of Neuron Digest [Volume 12 Issue 11]
*****************************************