Copy Link
Add to Bookmark
Report
Neuron Digest Volume 12 Number 12
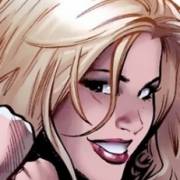
Neuron Digest Tuesday, 9 Nov 1993 Volume 12 : Issue 12
Today's Topics:
Santa Fe Time Series Competition book out
Hidden layer representations
Contact request
Post-doc at Purdue
Benchmarks - Summary of Responses
Send submissions, questions, address maintenance, and requests for old
issues to "neuron-request@psych.upenn.edu". The ftp archives are
available from psych.upenn.edu (130.91.68.31). Back issues requested by
mail will eventually be sent, but may take a while.
----------------------------------------------------------------------
Subject: Santa Fe Time Series Competition book out
From: weigend@sabai.cs.colorado.edu
Date: Fri, 22 Oct 93 01:37:55 -0700
Announcing book on the results of the Santa Fe Time Series Competition:
____________________________________________________________________
Title: TIME SERIES PREDICTION:
Forecasting the Future and Understanding the Past.
Editors: Andreas S. Weigend and Neil A. Gershenfeld
Publisher: Addison-Wesley, September 1993.
Paperback ISBN 0-201-62602-0 US$32.25 (672 pages)
Hardcover ISBN 0-201-62601-2 US$49.50 (672 pages)
The rest of this message gives some background,
ordering information, and the table of contents.
____________________________________________________________________
Most observational disciplines, such as physics, biology, and finance,
try to infer properties of an unfamiliar system from the analysis of a measured
time record of its behavior. There are many mature techniques associated with
traditional time series analysis. However, during the last decade, several new
and innovative approaches have emerged (such as neural networks and time-delay
embedding), promising insights not available with these standard methods.
Unfortunately, the realization of this promise has been difficult.
Adequate benchmarks have been lacking, and much of the literature has been
fragmentary and anecdotal.
This volume addresses these shortcomings by presenting the results of a
careful comparison of different methods for time series prediction and
characterization. This breadth and depth was achieved through the Santa Fe
Time Series Prediction and Analysis Competition, which brought together an
international group of time series experts from a wide variety of fields to
analyze data from the following common data sets:
- A physics laboratory experiment (NH3 laser)
- Physiological data from a patient with sleep apnea
- Tick-by-tick currency exchange rate data
- A computer-generated series designed specifically for the Competition
- Astrophysical data from a variable white dwarf star
- J. S. Bach's last (unfinished) fugue from "Die Kunst der Fuge."
In bringing together the results of this unique competition, this volume serves
as a much-needed survey of the latest techniques in time series analysis.
Andreas Weigend received his Ph.D. from Stanford University
and was a postdoc at Xerox PARC. He is Assistant Professor in
the Computer Science Department and at the Institute of
Cognitive Science at the University of Colorado at Boulder.
Neil Gershenfeld received his Ph.D. from Cornell University
and was a Junior Fellow at Harvard University. He is Assistant
Professor at the Media Lab at MIT.
____________________________________________________________________
Order it through your bookstore, or directly from the publisher by
- calling the Addison-Wesley Order Department at 1-800-358-4566,
- faxing 1-800-333-3328,
- emailing <marcuss@world.std.com>, or
- writing to Advanced Book Marketing
Addison-Wesley Publishing
One Jacob Way
Reading, MA 01867, USA.
VISA, Mastercard, and American Express and checks are accepted. When you prepay by check, Addison-Wesley pays shipping and ha
ndling charges. If payment does not accompany your order, shipping charges will be added to your invoice. Addison-Wesley is requir
ed to remit sales tax to the following states: AZ, AR, CA, CO, CT, FL, GA, IL, IN, LA, ME, MA, MI, MN, NY, NC, OH, PA, RI, SD, TN, T
X, UT, VT, WA, WV, WI.
_____________________________________________________________________
TABLE OF CONTENTS
xv Preface
Andreas S. Weigend and Neil A. Gershenfeld
1 The Future of Time Series: Learning and Understanding
Neil A. Gershenfeld and Andreas S. Weigend
Section I. DESCRIPTION OF THE DATA SETS__________________________________
73 Lorenz-Like Chaos in NH3-FIR Lasers
Udo Huebner, Carl-Otto Weiss, Neal Broadus Abraham, and Dingyuan Tang
105 Multi-Channel Physiological Data: Description and Analysis
David R. Rigney, Ary L. Goldberger, Wendell C. Ocasio, Yuhei Ichimaru, George B. Moody, and Roger G. Mark
131 Foreign Currency Dealing: A Brief Introduction
Jean Y. Lequarre
139 Whole Earth Telescope Observations of the White Dwarf Star (PG1159-035)
J. Christopher Clemens
151 Baroque Forecasting: On Completing J.S. Bach's Last Fugue
Matthew Dirst and Andreas S. Weigend
Section II. TIME SERIES PREDICTION________________________________________
175 Time Series Prediction by Using Delay Coordinate Embedding
Tim Sauer
195 Time Series Prediction by Using a Connectionist Network with Internal Delay Lines
Eric A. Wan
219 Simple Architectures on Fast Machines: Practical Issues in Nonlinear Time Series Prediction
Xiru Zhang and Jim Hutchinson
243 Neural Net Architectures for Temporal Sequence Processing
Michael C. Mozer
265 Forecasting Probability Densities by Using Hidden Markov Models with Mixed States
Andrew M. Fraser and Alexis Dimitriadis
283 Time Series Prediction by Using the Method of Analogues
Eric J. Kostelich and Daniel P. Lathrop
297 Modeling Time Series by Using Multivariate Adaptive Regression Splines (MARS)
P.A.W. Lewis, B.K. Ray, and J.G. Stevens
319 Visual Fitting and Extrapolation
George G. Lendaris and Andrew M. Fraser
323 Does a Meeting in Santa Fe Imply Chaos?
Leonard A. Smith
Section III. TIME SERIES ANALYSIS AND CHARACTERIZATION___________________
347 Exploring the Continuum Between Deterministic and Stochastic Modeling
Martin C. Casdagli and Andreas S. Weigend
367 Estimating Generalized Dimensions and Choosing Time Delays: A Fast Algorithm
Fernando J. Pineda and John C. Sommerer
387 Identifying and Quantifying Chaos by Using Information-Theoretic Functionals
Milan Palus
415 A Geometrical Statistic for Detecting Deterministic Dynamics
Daniel T. Kaplan
429 Detecting Nonlinearity in Data with Long Coherence Times
James Theiler, Paul S. Linsay, and David M. Rubin
457 Nonlinear Diagnostics and Simple Trading Rules for High-Frequency Foreign Exchange Rates
Blake LeBaron
475 Noise Reduction by Local Reconstruction of the Dynamics
Holger Kantz
Section IV. PRACTICE AND PROMISE_________________________________________
493 Large-Scale Linear Methods for Interpolation, Realization, and Reconstruction of Noisy, Irregularly Sampled Data
William H. Press and George B. Rybicki
513 Complex Dynamics in Physiology and Medicine
Leon Glass and Daniel T. Kaplan
529 Forecasting in Economics
Clive W.J. Granger
539 Finite-Dimensional Spatial Disorder: Description and Analysis
V.S. Afraimovich, M.I. Rabinovich, and A.L. Zheleznyak
557 Spatio-Temporal Patterns: Observations and Analysis
Harry L. Swinney
569 Appendix: Accessing the Server
571 Bibliography (800 references)
631 Index
------------------------------
Subject: Hidden layer representations
From: garry_k <G.Kearney@greenwich.ac.uk>
Date: Fri, 22 Oct 93 12:49:43 +0000
As a newcomer to NN I would value direction in the area of how we can
discover real world representations in the layers of the net. I would
appreciate some guidance as to reading matter on this. I understand
that pca and cluster analysis is involved usually. Is this the only
method? What applications derive from discovering these
representations? Thanks.
------------------------------
Subject: Contact request
From: Rowan Limb <rlimb@hfnet.bt.co.uk>
Date: Fri, 22 Oct 93 15:31:21 +0000
I have a copy of an abstract submitted to the International Neural Network
Conference held in Paris in July 1990 (INNC-90) but no full paper was
submitted. I would like further information on this submission and/or
a contact address (email if possible) for the authors. The details are:
Title: Associative Relational Database: Design and Implementation
Authors: Vladimir Cherkassky & Michael J Endrizzi
Dept. of Electrical Engineering & Dept. of Computer Science
University of Minnesota
Minneapolis, MN 55455 USA
Thanks in advance,
Rowan Limb
Decision Support Systems
BT Laboratories
Martlesham Heath
IPSWICH IP5 7RE
England
email: limb_p_r@bt-web.bt.co.uk
or: rlimb@hfnet.bt.co.uk
------------------------------
Subject: Post-doc at Purdue
From: Frank Doyle <fdoyle@ecn.purdue.edu>
Date: Fri, 22 Oct 93 12:12:30 -0600
Postdoctoral position available in :
NEURO-MODELING
in the Department of Chemical Engineering, Purdue University
Position for 2 years (beginning Fall 1993; salary: $25,000 per year).
Subject: Neuro-modeling of blood pressure control
This project is part of an interdisciplinary program involving
industrial and academic participants from DuPont, Purdue University,
the University of Pennsylvannia, and Louisiana State University. The
program encompasses the disciplines of chemical engineering, automatic
control, and neuroscience. Active interactions with engineers and
nonlinear control and modeling community at Purdue and DuPont as well
as with the neuroscientists at DuPont and Penn will be necessary for
the success of the project. A strong background in neuro-modeling is
required. The facilities at Purdue include state-of-the art
computational workstations (HP 735s and Sun 10/41s).
The postdoctoral candidate will work on the development of models of
the control mechanisms responsible for blood pressure regulation. The
neural system under investigation is the cardiorespiratory control
system, which integrates sensory information on respiratory and
cardiovascular variables to regulate and coordinate cardiac, vascular
and respiratory activity. In order to better understand this system our
program does neurobiolgical research and computational modeling. In
effect, these results reverse engineer neuronal and systems
function, which can have implications for engineering application; and
the engineering applications of our first interest are in chemical
engineering. The overall effort involves neurobiologists, chemical
engineers, computer scientists, bioengineers and neural systems
modelers. The present position is meant to contribute to the neural
systems modeling - chemical engineering interaction.
The neural computational-modeling work is progressing at several
levels: (1) systems-level modeling modeling of the closed-loop
cardiorespiratory system, (2) cellular level modeling of nonlinear
computation in Hodgkin-Huxley style neuron models, and (3) network
modeling of networks built-up from HH-style neurons incorporating
channel kinetics and synaptic conductances to capture the mechanisms in
the baroreceptor vagal reflex. The macroscopic model will be used (in
conjunction with experimental data from the literature and from the
laboratory of Dr. Schwaber) in developing structures to represent the
control functions. The synaptic level modeling activities will be used
in developing the building blocks which achieve the control function.
The present position will focus towards research goals, under the
supervision of Dr. Frank Doyle, that include the identification of
novel control and modeling techniques.
Interested candidates should send their curriculum vitae to BOTH:
Prof. Francis J. Doyle III
School of Chemical Engineering
Purdue University
West Lafayette, IN 47907-1283
(317) 497-9228
E-mail: fdoyle@ecn.purdue.edu
&
Dr. James Schwaber
Neural Computation Group
E.I. DuPont deNemours & Co., Inc.
P.O. Box 80352
Wilmington, DE 19880-0352
(302) 695-7136
E-mail: schwaber@eplrx7.es.duPont.com
------------------------------
Subject: Benchmarks - Summary of Responses
From: stjaffe@vaxsar.vassar.edu (steve jaffe)
Date: 22 Oct 93 14:18:47 -0500
Thanks to those who responded to my request for information on collections
of benchmarks with which to test and compare various nn architectures and
algorithms. Specific thanks to Nadine Tschichold-Guerman
<nadine@ifr.ethz.ch>, John Reynolds <reynolds@cns.bu.edu>, Tim Ross
<ross@toons.aar.wpafb.af.mil>, and Peter G. Raeth
<raethpg%wrdc.dnet@wl.wpafb.af.mil>. I list below their specific
recommendations along with others I have discovered.
Most correspondents mentioned the UCI database, and it would seem to be the
largest and best-known such collection. It is, not surprisingly, also
listed in the FAQ for comp.ai.neural-nets.
=====================================
1. From "FAQ for comp.ai.neural-nets":
written by: Lutz Prechelt (email: prechelt@ira.uka.de)
(Note: the current FAQ can be obtained by ftp from
rtfm.mit.edu. Look in the anonymous ftp directory "/pub/usenet/news.answers")
Question 19:-A19.) Databases for experimentation with NNs ?
[are there any more ?]
1. The nn-bench Benchmark collection
accessible via anonymous FTP on
"pt.cs.cmu.edu"
in directory
"/afs/cs/project/connect/bench"
or via the Andrew file system in the directory
"/afs/cs.cmu.edu/project/connect/bench"
In case of problems email contact is "nn-bench-request@cs.cmu.edu".
Data sets currently avaialable are:
nettalk Pronunciation of English words.
parity N-input parity.
protein Prediction of secondary structure of proteins.
sonar Classification of sonar signals.
two-spirals Distinction of a twin spiral pattern.
vowel Speaker independant recognition of vowels.
xor Traditional xor.
2. UCI machine learning database
accessible via anonymous FTP on
"ics.uci.edu" [128.195.1.1]
in directory
"/pub/machine-learning-databases"
3. NIST special databases of the National Institute Of Standards
And Technology:
NIST special database 2:
Structured Forms Reference Set (SFRS)
The NIST database of structured forms contains 5,590 full page images
of simulated tax forms completed using machine print. THERE IS NO REAL
TAX DATA IN THIS DATABASE. The structured forms used in this database
are 12 different forms from the 1988, IRS 1040 Package X. These
include Forms 1040, 2106, 2441, 4562, and 6251 together with Schedules
A, B, C, D, E, F and SE. Eight of these forms contain two pages or
form faces making a total of 20 form faces represented in the
database. Each image is stored in bi-level black and white raster
format. The images in this database appear to be real forms prepared
by individuals but the images have been automatically derived and
synthesized using a computer and contain no "real" tax data. The entry
field values on the forms have been automatically generated by a
computer in order to make the data available without the danger of
distributing privileged tax information. In addition to the images
the database includes 5,590 answer files, one for each image. Each
answer file contains an ASCII representation of the data found in the
entry fields on the corresponding image. Image format documentation
and example software are also provided. The uncompressed database
totals approximately 5.9 gigabytes of data.
NIST special database 3:
Binary Images of Handwritten Segmented Characters (HWSC)
Contains 313,389 isolated character images segmented from the
2,100 full-page images distributed with "NIST Special Database 1".
223,125 digits, 44,951 upper-case, and 45,313 lower-case character
images. Each character image has been centered in a separate
128 by 128 pixel region, error rate of the segmentation and
assigned classification is less than 0.1%.
The uncompressed database totals approximately 2.75 gigabytes of
image data and includes image format documentation and example software.
NIST special database 4:
8-Bit Gray Scale Images of Fingerprint Image Groups (FIGS)
The NIST database of fingerprint images contains 2000 8-bit gray scale
fingerprint image pairs. Each image is 512 by 512 pixels with 32 rows
of white space at the bottom and classified using one of the five
following classes: A=Arch, L=Left Loop, R=Right Loop, T=Tented Arch,
W=Whirl. The database is evenly distributed over each of the five
classifications with 400 fingerprint pairs from each class. The images
are compressed using a modified JPEG lossless compression algorithm
and require approximately 636 Megabytes of storage compressed and 1.1
Gigabytes uncompressed (1.6 : 1 compression ratio). The database also
includes format documentation and example software.
More short overview:
Special Database 1 - NIST Binary Images of Printed Digits, Alphas, and Text
Special Database 2 - NIST Structured Forms Reference Set of Binary Images
Special Database 3 - NIST Binary Images of Handwritten Segmented Characters
Special Database 4 - NIST 8-bit Gray Scale Images of Fingerprint Image Groups
Special Database 6 - NIST Structured Forms Reference Set 2 of Binary Images
Special Database 7 - NIST Test Data 1: Binary Images of Handprinted Segmented
Characters
Special Software 1 - NIST Scoring Package Release 1.0
Special Database 1 - $895.00
Special Database 2 - $250.00
Special Database 3 - $895.00
Special Database 4 - $250.00
Special Database 6 - $250.00
Special Database 7 - $1,000.00
Special Software 1 - $1,150.00
The system requirements for all databases are a 5.25" CD-ROM drive
with software to read ISO-9660 format.
Contact: Darrin L. Dimmick
dld@magi.ncsl.nist.gov (301)975-4147
If you wish to order the database, please contact:
Standard Reference Data
National Institute of Standards and Technology
221/A323
Gaithersburg, MD 20899
(301)975-2208 or (301)926-0416 (FAX)
4. CEDAR CD-ROM 1: Database of Handwritten
Cities, States, ZIP Codes, Digits, and Alphabetic Characters
The Center Of Excellence for Document Analysis and Recognition (CEDAR)
State University of New York at Buffalo announces the availability of
CEDAR CDROM 1: USPS Office of Advanced Technology
The database contains handwritten words and ZIP Codes
in high resolution grayscale (300 ppi 8-bit) as well as
binary handwritten digits and alphabetic characters (300 ppi
1-bit). This database is intended to encourage research in
off-line handwriting recognition by providing access to
handwriting samples digitized from envelopes in a working
post office.
Specifications of the database include:
+ 300 ppi 8-bit grayscale handwritten words (cities,
states, ZIP Codes)
o 5632 city words
o 4938 state words
o 9454 ZIP Codes
+ 300 ppi binary handwritten characters and digits:
o 27,837 mixed alphas and numerics segmented
from address blocks
o 21,179 digits segmented from ZIP Codes
+ every image supplied with a manually determined
truth value
+ extracted from live mail in a working U.S. Post
Office
+ word images in the test set supplied with dic-
tionaries of postal words that simulate partial
recognition of the corresponding ZIP Code.
+ digit images included in test set that simulate
automatic ZIP Code segmentation. Results on these
data can be projected to overall ZIP Code recogni-
tion performance.
+ image format documentation and software included
System requirements are a 5.25" CD-ROM drive with software to read ISO-
9660 format.
For any further information, including how to order the
database, please contact:
Jonathan J. Hull, Associate Director, CEDAR, 226 Bell Hall
State University of New York at Buffalo, Buffalo, NY 14260
hull@cs.buffalo.edu (email)
==========================================
2. From John Reynolds <reynolds@cns.bu.edu>:
We've come across several benchmarks which were proposed as standards
for categorizers. More information is available on each in a couple
of papers we wrote, which were printed in Neural Networks and IEEE
Transactions on Neural Networks.
The mushroom database was introduced by Schlimmer in 1987. The
task is to tell poisonous and non-poisonous musrhooms apart. There
are 8124 training patterns, of which about 50% are poisonous and 50%
are non-poisonous. The database is available by anonymous ftp, and
is described in:
Carpenter, G.A., Grossberg, S., and Reynolds, J., (1991). ARTMAP:
Supervised real-time learning and classification of nonstationary data
by a self-organizing neural network, {\sl Neural Networks}, {\bf 4},
565--588.
Three more benchmark problems, described briefly below, are detailed
in the following article:
Carpenter, G.A., Grossberg, S., Markuzon, N., Reynolds, J., and
Rosen,D., (1992). Fuzzy ARTMAP: A neural network architecture for
incremental supervised learning of analog multidimensional maps, {\em
IEEE Transactions on Neural Networks}, {\bf 3}, 698--713.
Frey and Slate developed a benchmark machine learning task in 1991 in
which a system has to identify an input exemplar as one of 26 capital
letters A--Z. The database was derived from 20,000 different binary
pixel images. There are a wide variety of letter types represented --
different stroke styles, letter styles, and random distortions. This
database is available from the UCI Repository of Machine Learning
Databases maintained by David repository@ics.uci.edu
Lang and Witbrock Alexis P. Wieland introduced the nested spirals
problem, and it has been used as a benchmark by Lang and Witbrock,
1989. The two spirals of the benchmark task each make three complete
turns in the plane, with 32 points per turn plus an endpoint,
totalling 97.
The circle in the square problem, which requires a system to identify
which points of a square lie inside and which lie outside a circle
whose area equals half that of the square, was specified as a
benchmark problem for system performance evaluation in the DARPA
Artificial Neural Network Technology (ANNT) Program (Wilensky, 1990).
Last time I checked, there are a variety of different learning tasks
in the UCI repository, and that would probably be worth looking into.
I hope that helps. Good luck with your search! -John
=================================================
3. From Tim Ross <ross@toons.aar.wpafb.af.mil>:
I'm sure you're aware of the uci machine learning database (ics.uci.edu)
and the logic synthesis benchmarks (gloster@mcnc.org) that are also used
as machine learning test cases.
We at Wright Lab use a set of 30 benchmark functions, each on 8 binary
inputs and a single binary output. These functions were selected for a
variety of type (numeric, symbolic, images, ...), complexity (measured by
decomposed function cardinality, an especially robust measure), and
number of minority elements (i.e. fraction of inputs whose output is
ONE). We have done/are doing experiments (using these benchmarks) with a
BP NN, Abductory Inference Mechanism, C4.5 and an in-house method. We
are also developing a similar set of benchmark functions on larger (esp.
12 and 16) numbers of input variables. We, of course, would be happy to
see these benchmarks used elsewhere.
=======================================
4. A set of examples comes with the distribution of the nn simulator
package "Aspirin/Migraines", available from two FTP sites:
CMU's simulator collection on "pt.cs.cmu.edu" (128.2.254.155)
in /afs/cs/project/connect/code/am6.tar.Z".
and UCLA's cognitive science machine "ftp.cognet.ucla.edu" (128.97.50.19)
in alexis/am6.tar.Z
These are the examples provided:
xor: from RumelHart and McClelland, et al,
"Parallel Distributed Processing, Vol 1: Foundations",
MIT Press, 1986, pp. 330-334.
encode: from RumelHart and McClelland, et al,
"Parallel Distributed Processing, Vol 1: Foundations",
MIT Press, 1986, pp. 335-339.
bayes: Approximating the optimal bayes decision surface
for a gauss-gauss problem.
detect: Detecting a sine wave in noise.
iris: The classic iris database.
characters: Learing to recognize 4 characters independent
of rotation.
ring: Autoregressive network learns a decaying sinusoid
impulse response.
sequence: Autoregressive network learns to recognize
a short sequence of orthonormal vectors.
sonar: from Gorman, R. P., and Sejnowski, T. J. (1988).
"Analysis of Hidden Units in a Layered Network Trained to
Classify Sonar Targets" in Neural Networks, Vol. 1, pp. 75-89.
spiral: from Kevin J. Lang and Michael J, Witbrock, "Learning
to Tell Two Spirals Apart", in Proceedings of the 1988 Connectionist
Models Summer School, Morgan Kaufmann, 1988.
ntalk: from Sejnowski, T.J., and Rosenberg, C.R. (1987).
"Parallel networks that learn to pronounce English text" in
Complex Systems, 1, 145-168.
perf: a large network used only for performance testing.
monk: The backprop part of the monk paper. The MONK's problem were
the basis of a first international comparison
of learning algorithms. The result of this comparison is summarized in
"The MONK's Problems - A Performance Comparison of Different Learning
algorithms" by S.B. Thrun, J. Bala, E. Bloedorn, I. Bratko, B.
Cestnik, J. Cheng, K. De Jong, S. Dzeroski, S.E. Fahlman, D. Fisher,
R. Hamann, K. Kaufman, S. Keller, I. Kononenko, J. Kreuziger, R.S.
Michalski, T. Mitchell, P. Pachowicz, Y. Reich H. Vafaie, W. Van de
Welde, W. Wenzel, J. Wnek, and J. Zhang has been published as
Technical Report CS-CMU-91-197, Carnegie Mellon University in Dec.
1991.
wine: From the ``UCI Repository Of Machine Learning Databases
and Domain Theories'' (ics.uci.edu: pub/machine-learning-databases).
------------------------------
End of Neuron Digest [Volume 12 Issue 12]
*****************************************