Copy Link
Add to Bookmark
Report
Neuron Digest Volume 11 Number 07
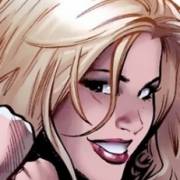
Neuron Digest Thursday, 28 Jan 1993 Volume 11 : Issue 7
Today's Topics:
CALL FOR PAPERS - ANN STANDARDS
Financial futures?
Quantum neural computer
integrating symbolic processing with neural networks
Previous post
FREE MUME version 0.5 for MSDOS platform
IND Version 2.1 tree software available
Re: Inability of feed forward nets to learn polynomials
Neural Networks for System Modelling
Cross-Val: Summary of Lit Survey and Request for References
Send submissions, questions, address maintenance, and requests for old
issues to "neuron-request@cattell.psych.upenn.edu". The ftp archives are
available from cattell.psych.upenn.edu (130.91.68.31). Back issues
requested by mail will eventually be sent, but may take a while.
----------------------------------------------------------------------
Subject: CALL FOR PAPERS - ANN STANDARDS
From: John Fulcher <john@cs.uow.edu.au>
Date: Mon, 04 Jan 93 14:47:24 -0500
CALL FOR PAPERS - ANN STANDARDS
COMPUTER STANDARDS & INTERFACES
For some time now, there has been a need to consolidate and formalise the
efforts of researchers in the Artificial Neural Network field. The
publishers of this North-Holland journal have deemed it appropriate to
devote a forthcoming special issue of Computer Standards & Interfaces to
ANN standards, under the guest editorship of John Fulcher, University of
Wollongong, Australia.
We already have the cooperation of the IEEE/NCC Standards Committee, but
are also interested in submissions regarding less formal, de facto
"standards". This could range from established, "standard" techniques in
various application areas (vision, robotics, speech, VLSI etc.), or ANN
techniques generally (such as the backpropagation algorithm & its
[numerous] variants, say). Accordingly, survey or review articles would
be particularly welcome.
If you are interested in submitting a paper for consideration, you will
need to send three copies (in either hard copy or electronic form) by
March 31st, 1993 to:
John Fulcher,
Department of Computer Science,
University of Wollongong,
Northfields Avenue,
Wollongong NSW 2522,
Australia.
fax: +61 42 213262
email: john@cs.uow.edu.au.oz
------------------------------
Subject: Financial futures?
From: "Mike Carrier" <mikecarrier@delphi.com>
Date: 06 Jan 93 10:26:17 -0500
Neural Networks and Financial Prognostication
Lately I've been perusing the back issues via the FTP cache,
and I'd first like to thank Mjr. Peter G. Raeth for his compilation of a
suggested reading list, as well as all of those who submitted titles to
him. This compilation was a key in my initiation to NN's and expert
systems.
I am interested in the possible applications of NN's and their
uses in predicting future market activity. I have reviewed Tiong Hwee
Goh's paper, and the NN/GA premise shows promise. Other than this, does
anyone have other papers/publications that they'd suggest to me? Thanks
in advance, FTP instructions for them are appreciated as well.
Mike Carrier
mikecarrier@delphi.com
------------------------------
Subject: Quantum neural computer
From: "Dr. S. Kak" <kak@max.ee.lsu.edu>
Date: Thu, 07 Jan 93 13:47:10 -0600
Hitherto all computers have been designed based on classical laws.
We consider the question of building a quantum neural computer and
speculate on its computing power. We argue that such a computer
could have the potential to solve artificial intelligence problems.
History tells us that paradigms of science and technology draw on
each other. Thus Newton's conception of the universe was based on
the mechanical engines of the day; thermodynamics followed the heat
engines of the 19th century; and computers followed the development
of telegraph and telephone. From another point of view, modern
computers are based on classical physics. Since classical physics
has been superseded by quantum mechanics in the microworld, one
might ask the question if a new paradigm of computing based on
quantum mechanics can be constructed.
Intelligence, and by implication consciousness, has been taken by
many computer scientists to emerge from the complexity of the
interconnections between the neurons. But if it is taken to be a
unity, as urged by Schrodinger and other physicists ,
then it should be described by a quantum mechanical wave
function. No representation in terms of networking of classical
objects, such as threshold neurons, can model a wave function.
This is another reason that one seeks a new computing paradigm.
A brain-mind identity hypothesis,
with a mechanistic or electronic representation of the brain
processes, does not explain how self-awareness could arise. At
the level of ordinary perception there exists a duality and
complementarity between an autonomous (and reflexive ) brain and
a mind with intentionality. The notion of self seems to hinge on
an indivisibility akin to that found in quantum mechanics. This
was argued most forcefully by Schrodinger, one of the creators of
quantum mechanics.
A quantum neural computer will start out with a wavefunction that
is a sum of several different problem functions. After the evolution
of the wavefunction the measurement operator will force the
wavefunction to reduce to the correct eigenfunction with the
corresponding measurement that represents the computation.
A discussion of these issues is contained in my TECHNICAL REPORT
ECE/LSU 92-13, December 15, 1993 entitled
CAN WE BUILD A QUANTUM NEURAL COMPUTER?
If you would like to have an electronic copy (minus the math)
do let me know. Hard-copies are also available.
- -Subhash Kak
Professor of Electrical & Computer Engineering
Louisiana State University
Baton Rouge, LA 70803-5901, USA
Tel:(504) 388-5552; Fax: 504-388-5200
------------------------------
Subject: integrating symbolic processing with neural networks
From: Ron Sun <rsun@athos.cs.ua.edu>
Date: Thu, 07 Jan 93 17:29:02 -0600
I have collected a bibliography of papers on integrating symbolic
processing with neural networks, and am looking for additional references.
It's available in Neuroprose under the name Sun.bib.Z (in an unedited
form); I'll also be happy to send it to you directly if you e-mail me. The
bibliography will be included in a book on this topic that I'm co-editing.
I'm looking for additional references to make the bibliography as
comprehensive as possible. So, I would like authors to send me (a possibly
annotated) list of their publications on this topic (this is a chance to
make your work better known.) Also, anyone who has already compiled such a
bib, please let me know; I would like to incorporate it. Due credit will
be given, of course.
Here is my address. E-mail response (rsun@cs.ua.edu) is strongly preferred.
================================================================
Ron Sun, Ph.D
Assistant Professor
Department of Computer Science phone: (205) 348-6363
The University of Alabama fax: (205) 348-8573
Tuscaloosa, AL 35487 rsun@athos.cs.ua.edu
================================================================
Thanks for your cooperation.
------------------------------
Subject: Previous post
From: Ron Sun <rsun@athos.cs.ua.edu>
Date: Wed, 13 Jan 93 13:18:50 -0600
In my previous posting regarding references on symbolic processing
and connectionist models, I mentioned a file FTPable from Neuroprose.
The correct file name is sun.hlcbib.asc.Z (not sun.bib.Z).
My apology.
- --Ron
------------------------------
Subject: FREE MUME version 0.5 for MSDOS platform
From: Multi-Module Environment <mume@sedal.su.oz.au>
Date: Fri, 08 Jan 93 16:08:11 +1100
The Multi-Module Neural Computing Environment (MUME) version 0.5 for the
MSDOS platform is now available FREE of charge via anonymous ftp on
brutus.ee.su.oz.au:/pub/MUME-0.5-DOS.zip
The full listing of the file is:
-rw-r----- 1 mume mume 1391377 Jan 8 15:45 MUME-0.5-DOS.zip
Unzipping it should create a directory called MUME-DOS and it is about 4.6 MB.
Following is the README file.
Have fun.
MUME-Request@sedal.su.OZ.AU
=----------------------------------------------------------------------------
Multi-Module Neural Computing Environment
(MUME)
Version 0.5 (FREE) for MSDOS 5.0
MUME is a simulation environment for multi-modules neural computing. It
provides an object oriented facility for the simulation and training
of multiple nets with various architectures and learning algorithms.
MUME includes a library of network architectures including feedforward,
simple recurrent, and continuously running recurrent neural networks.
Each architecture is supported by a variety of learning algorithms.
MUME can be used for large scale neural network simulations as it provides
support for learning in multi-net environments. It also provide pre and
post-processing facilities.
The object oriented structure makes simple the addition of new
network classes and new learning algorithms. New classes/algorithms can be
simply added to the library or compiled into a program at run-time. The
interface between classes is performed using Network Service Functions
which can be easily created for a new class/algorithm.
The architectures and learning algorithms currently available are:
Class Learning algorithms
------------ -------------------
MLP backprop, weight perturbation,
node perturbation, summed weight
perturbation
SRN backprop through time, weight
update driven node splitting,
History bound nets
CRRN Williams and Zipser
Programmable
Limited precision nets Weight perturbation, Combined
Search Algorithm, Simulated Annealing
Other general purpose classes include (viewed as nets):
o DC source
o Time delays
o Random source
o FIFOs and LIFOs
o Winner-take-all
o X out of Y classifiers
The modules are provided in a library. Several "front-ends" or clients are
also available.
MUME can be used to include non-neural computing modules (decision
trees, ...) in applications.
The software is the product of a number of staff and postgraduate students
at the Machine Intelligence Group at Sydney University Electrical
Engineering. It is currently being used in research, research and
development and teaching, in ECG and ICEG classification, and speech and
image recognition. As such, we are interested in institutions that
can exploit the tool (especially in educational courses) and build up on it.
The software is written in 'C' and is aviable on the following platforms:
- Sun (SunOS)
- DEC (Ultrix)
- Fujitsu VP2200 (UXP/M)
- IBM RS6000 (AIX)
- Hewlett Packard (HP-UX)
- IBM PC compatibles (MSDOS 5.0) -- does not run under MS-Windows'
DOS sessions
THE MSDOS version of MUME is available as a public domain software. And can be
ftp-ed from brutus.ee.su.oz.au:/pub/MUME-0.5-DOS.zip.
MUME for the other platforms is available to research institutions on
media/doc/postage cost arrangements. Information on how to acquire it may be
obtained by writing (or email) to:
Marwan Jabri
SEDAL
Sydney University Electrical Engineering
NSW 2006 Australia
Tel: (+61-2) 692-2240
Fax: 660-1228
Email: marwan@sedal.su.oz.au
A MUME mailing list is currently available by sending an email to
MUME-Requests@sedal.su.OZ.AU
Please put your subscription email address on the 'Subject:' line.
To send mail to everybody in the mailing list, send it to:
MUME@sedal.su.OZ.AU
All bugs reports should be sent to MUME-Bugs@sedal.su.OZ.AU and should include
the following details:
1. Date (eg. 12 Feb 1993).
2. Name (eg. John Citizen).
3. Company/Institution (eg. Sydney University Electrical Engineering).
4. Contact Address (eg. what-is-mume@sedal.su.OZ.AU).
5. Version of MUME (eg. MUME 0.5).
6. Machine Name/Type (eg. Sun Sparc 2).
7. Version of the Operating System (eg. SunOS 4.1.1).
8. Brief Description of the problem(s).
9. Error Messages (if any).
10. Related Files (Filename, Version and Relationship to problems).
------------------------------
Subject: IND Version 2.1 tree software available
From: Wray Buntine <wray@ptolemy.arc.nasa.gov>
Date: Sun, 10 Jan 93 21:59:14 -0800
IND Version 2.1 - creation and manipulation of decision trees from data
=----------------------------------------------------------------------
A common approach to supervised classification and prediction in
artificial intelligence and statistical pattern recognition is the use of
decision trees. A tree is "grown" from data using a recursive
partitioning algorithm to create a tree which (hopefully) has good
prediction of classes on new data. Standard algorithms are CART (by
Breiman, Friedman, Olshen and Stone) and Id3 and its successor C4.5 (by
Quinlan). More recent techniques are Buntine's smoothing and option
trees, Wallace and Patrick's MML method, and Oliver and Wallace's MML
decision graphs which extend the tree representation to graphs. IND
reimplements and integrates these methods. The newer methods produce
more accurate class probability estimates that are important in
applications like diagnosis.
IND is applicable to most data sets consisting of independent instances,
each described by a fixed length vector of attribute values. An
attribute value may be a number, one of a set of attribute specific
symbols, or omitted. One of the attributes is delegated the "target" and
IND grows trees to predict the target. Prediction can then be done on
new data or the decision tree printed out for inspection.
IND provides a range of features and styles with convenience for the
casual user as well as fine-tuning for the advanced user or those
interested in research. Advanced features allow more extensive search,
interactive control and display of tree growing, and Bayesian and MML
algorithms for tree pruning and smoothing. These often produce more
accurate class probability estimates at the leaves. IND also comes with
a comprehensive experimental control suite.
IND consist of four basic kinds of routines; data manipulation routines,
tree generation routines, tree testing routines, and tree display
routines. The data manipulation routines are used to partition a single
large data set into smaller training and test sets. The generation
routines are used to build classifiers. The test routines are used to
evaluate classifiers and to classify data using a classifier. And the
display routines are used to display classifiers in various formats.
IND is written in K&R C, with controlling scripts in the "csh" shell of
UNIX, and extensive UNIX man entries. It is designed to be used on any
UNIX system, although it has only been thoroughly tested on SUN
platforms. IND comes with a manual giving a guide to tree methods, and
pointers to the literature, and several companion documents.
Availability
- ------------
IND Version 2.0 will shortly be available through NASA's COSMIC facility.
IND Version 2.1 is available strictly as unsupported beta-test software.
If you're interested in obtaining a beta-test copy, with no obligation on
your part to provide feedback, contact
Wray Buntine
NASA Ames Research Center
Mail Stop 269-2
Moffett Field, CA, 94035
email: wray@kronos.arc.nasa.gov
------------------------------
Subject: Re: Inability of feed forward nets to learn polynomials
From: joerd@wsuaix.csc.wsu.edu (Wayne Joerding - Economics)
Date: Tue, 12 Jan 93 08:56:52 -0800
Using logistic network with polynomials
Many months ago Pushpak Bhattacharyya of IIT Bombay posted an enquiry
concerning the inability of logistic based feedforward networks to learn
polynomials. It seems that learning algorithms failed to converge in a
number of simple cases. Pushpak Bhattacharyya's email address no longer
seems active so I resort to this digest to followup on this issue.
Recently, a colleague and I have developed a reason for the above noted
failure, and examples of other similar failures for other functions. We
would like to prepare a paper on the idea but thought we should check to
see if the issue is already been resolved. So I have several questions:
Has anybody else encountered this problem? Is the solution well known?
Does there exists a published paper resolving the issue? If so, where
published?
Thanks for any information on the topic.
Wayne, joerd@wsuaix.csc.wsu.edu
------------------------------
Subject: Neural Networks for System Modelling
From: "Duane A. White" <KFRAMXX%TAIVM2.BITNET@TAIVM1.taiu.edu>
Date: Wed, 13 Jan 93 16:17:28 -0600
Can anyone suggest some good references for using neural networks for system
modelling?
I am investigating ways of generating computer models of real world systems
based on input/output records. I would like to compare classical approaches
with neural networks and fuzzy logic.
Any help would be greatly appreciated.
------------------------------
Subject: Cross-Val: Summary of Lit Survey and Request for References
From: Mark Plutowksi <pluto@cs.UCSD.EDU>
Date: Sun, 17 Jan 93 15:43:03 -0800
Hello,
This is a follow-on to recent postings on using cross-validation to
assess neural network models. It is a request for further references,
after an exhausting literature survey of my own which failed to find the
results I seek. A summary of my findings follows the request, followed
by an informative response from Grace Wahba, and finally, a list of the
references I looked at.
Thanks for any leads or tips,
=================
== Mark Plutowski pluto@cs.ucsd.edu
Computer Science and Engineering 0114
University of California, San Diego
La Jolla, California, USA.
THE REQUEST:
------------
Do you know of convergence/consistency results for justifying
cross-validatory model assessment for nonlinear compositions of basis
functions, such as the usual sigmoided feedforward network?
SUMMARY OF MY LIT SURVEY:
-------------------------
While the use of cross-validation to assess nonlinear neural network
models CAN be justified to a certain degree, (e.g., [Stone 76,77]) the
really nice theoretical results exist for other estimators, e.g., kernel
density, histograms, linear models, and splines (see references below.)
These results are not directly applicable to neural nets. They all
exploit properties of the particular estimators which are not shared by
neural networks, in general. In short, the proofs for linear models
exploit linear reductions, and the other (nonlinear) estimators for which
optimality results have been published have the property that deleting a
single example has negligible effect on the estimate outside a bounded
region surrounding the example (e.g., kernel density estimators and
splines.) In comparison, a single example can affect every weight of a
neural network - deleting it can have global effect on the estimate.
GRACE WAHBA SAYS:
------------------
Thanks to Grace Wahba for her informative response to my request to her
for information after I was unable to get hold of a copy of her relevant
book:
============================================================
Date: Wed, 13 Jan 93 22:32:29 -0600
From: wahba@stat.wisc.edu (Grace Wahba)
Message-Id: <9301140432.AA22884@hera.stat.wisc.edu>
Received: by hera.stat.wisc.edu; Wed, 13 Jan 93 22:32:29 -0600
To: pluto@cs.UCSD.EDU
Subject: Re: choose your own randomized regularizer
Very interesting request.. !!
I'm convinced (as you seem to be) that some interesting
results are to be obtained using CV or GCV in the
context of neural nets. In my book are brief discussions
of how GCV can be used in certain nonlinear inverse
problems (Sect 8.3), and when one is doing penalized
likelihood with non-Gaussian data (Sect 9.2).
(No theory is given, however).
Finbarr O'Sullivan (finbarr@stat.washington.edu)
has further results on problems like those in Sect 8.3.
However, I have not seen any theoretical results in the
context of sigmoidal feedforward networks (but that
sure would be interesting!!). However, if you make
a local quadratic approximation to an optimization
problem to get a local linear approximation to the
influence operator (which plays the role of A(\lambda)),
then you have to decide where you are going to take
your derivatives. In my book on page 113 (equation (9.2.19)
I make a suggestion as to where to
take the derivatives , but I later
got convinced that that was not the best way
to do it. Chong Gu,`Cross-Validating Non-Gaussian Data',
J. Computational and Graphical Statistics 1, 169-179, June, 1992
has a discussion of what he (and I) believe is a better way,
in that context. That context doesn't look at all like
neural nets, I only mention this in case you
get into some proofs in the neural network context -
in that event I think you may have to worry about
where you differentiate and Gu's arguments may be valid
more generally..
As far as missing any theoretical result due to not having my
book, the only theoretical cross validation result discussed
in any detail is that in Craven and Wahba(1979) which
has been superceded by the work of Li, Utreras and Andrews.
As far as circulating your request to the net do go right
ahead- I will be very interested in any answers you get!!
\bibitem[Wahba 1990]
Wahba,Grace. 1990.
"Spline Models for Observational Data"
v. 59 in the CBMS-NSF Regional Conference
Series in Applied Mathematics,
SIAM, Philadelphia, PA, March 1990.
Softcover, 169 pages, bibliography, author index.
ISBN 0-89871-244-0
ORDER INFO FOR WAHBA 1990:
==========================
List Price $24.75, SIAM or CBMS* Member Price $19.80
(Domestic 4th class postage free, UPS or Air extra)
May be ordered from SIAM by mail, electronic mail, or phone:
SIAM
P. O. Box 7260
Philadelphia, PA 19101-7260
USA
service@siam.org
Toll-Free 1-800-447-7426 (8:30-4:45 Eastern Standard Time,
the US only.
Regular phone: (215)382-9800
FAX (215)386-7999
May be ordered on American Express, Visa or Mastercard,
or paid by check or money order in US dollars,
or may be billed (extra charge).
CBMS member organizations include AMATC, AMS, ASA, ASL, ASSM,
IMS, MAA, NAM, NCSM, ORSA, SOA and TIMS.
============================================================
REFERENCES:
===========
\bibitem[Li 86]
Li, Ker-Chau. 1986.
``Asymptotic optimality of $C_{L}$ and generalized
cross-validation in ridge regression with
application to spline smoothing.''
{\em The Annals of Statistics}.
{\bf 14}, 3, 1101-1112.
\bibitem[Li 87]
Li, Ker-Chau. 1987.
``Asymptotic optimality for $C_{p}$, $C_{L}$,
cross-validation, and generalized cross-validation:
discrete index set.''
{\em The Annals of Statistics}.
{\bf 15}, 3, 958-975.
\bibitem[Utreras 87]
Utreras, Florencio I. 1987.
``On generalized cross-validation for
multivariate smoothing spline functions.''
{\em SIAM J. Sci. Stat. Comput.}
{\bf 8}, 4, July 1987.
\bibitem[Andrews 91]
Andrews, Donald W.K. 1991.
``Asymptotic optimality of generalized
$C_{L}$, cross-validation, and generalized
cross-validation in regression with heteroskedastic
errors.''
{\em Journal of Econometrics}. {\bf 47} (1991) 359-377.
North-Holland.
\bibitem[Bowman 80]
Bowman, Adrian W. 1980.
``A note on consistency of the kernel method for
the analysis of categorical data.''
{\em Biometrika} (1980), {\bf 67}, 3, pp. 682-4.
\bibitem[Hall 83]
Hall, Peter. 1983.
``Large sample optimality of least squares cross-validation
in density estimation.''
{\em The Annals of Statistics}.
{\bf 11}, 4, 1156-1174.
Stone, Charles J. 1984
``An asymptotically optimal window selection rule
for kernel density estimates.''
{\em The Annals of Statistics}.
{\bf 12}, 4, 1285-1297.
\bibitem[Stone 59]
Stone, M. 1959.
``Application of a measure of information
to the design and comparison of regression experiments.''
{\em Annals Math. Stat.} {\bf 30} 55-69
\bibitem[Marron 87]
Marron, M. 1987.
``A comparison of cross-validation techniques in density estimation.''
{\em The Annals of Statistics}.
{\bf 15}, 1, 152-162.
\bibitem[Bowman etal 84]
Bowman, Adrian W., Peter Hall, D.M. Titterington. 1984.
``Cross-validation in nonparametric estimation of
probabilities and probability densities.''
{\em Biometrika} (1984), {\bf 71}, 2, pp. 341-51.
\bibitem[Bowman 84]
Bowman, Adrian W. 1984.
``An alternative method of cross-validation for the
smoothing of density estimates.''
{\em Biometrika} (1984), {\bf 71}, 2, pp. 353-60.
\bibitem[Stone 77]
Stone, M. 1977.
``An asymptotic equivalence of choice of model by
cross-validation and Akaike's criterion.''
{\em J. Roy. Stat. Soc. Ser B}, {\bf 39}, 1, 44-47.
\bibitem[Stone 76]
Stone, M. 1976.
"Asymptotics for and against cross-validation"
??
------------------------------
End of Neuron Digest [Volume 11 Issue 7]
****************************************