Copy Link
Add to Bookmark
Report
Neuron Digest Volume 11 Number 13
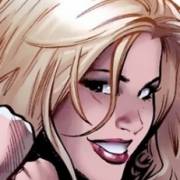
Neuron Digest Tuesday, 23 Feb 1993 Volume 11 : Issue 13
Today's Topics:
Biologically Plausible Dynamic Artificial Neural Networks Reviewed
Send submissions, questions, address maintenance, and requests for old
issues to "neuron-request@cattell.psych.upenn.edu". The ftp archives are
available from cattell.psych.upenn.edu (130.91.68.31). Back issues
requested by mail will eventually be sent, but may take a while.
----------------------------------------------------------------------
Subject: Biologically Plausible Dynamic Artificial Neural Networks Reviewed
From: Paul Fawcett <paulf@manor.demon.co.uk>
Date: Sat, 06 Feb 93 18:57:41 +0000
Thank you for publishing in Neuron Digest Vol. 11, Issue 6 my original
posting on the subject of 'Biologically Plausible Dynamic Artificial
Networks'.
As a follow-up to this post I circulated a summary of replies to those who
contacted me directly by email. Each contributor agreed to their comments
being quoted in this way. Please feel free to use this material, in full
or in part, for any future edition of the Neuron Digest.
The summary follows:
Last update: 20 JAN 93
DISTRIBUTION:
Ulf Andrick <andrick@rhrk.uni-kl.de>
Mark J. Crosbie <mcrosbie@unix1.tcd.ie>
S. E. Fahlman <sef@sef-pmax.slisp.cs.cmu.edu>
Bernie French <btf64@cas.org>
John R. Mcdonnell <mcdonn%bach.nosc.mil@nosc.mil>
Larry D. Pyeatt <pyeatt@texaco.com>
Bill Saidel <saidel@clam.rutgers.edu>
Mark W. Tilden <mwtilden@math.uwaterloo.ca>
Jari Vaario <jari@ai.rcast.u-tokyo.ac.jp>
Paul Verschure <verschur@ifi.unizh.ch>
Stanley Zietz <szietz@king.mcs.drexel.edu>
THIS BULLETIN SUMMARIZES THE MAJORITY OF E-MAIL REPLIES TO THE FOLLOWING
USENET ARTICLE:
All contributors have agreed to publication of their comments.
From: paulf@manor.demon.co.uk (Paul Fawcett)
Newsgroups: comp.ai,
comp.ai.neural-nets,
sci.cognitive,
comp.theory.cell-automata,
bionet.neuroscience,
bionet.molbio.evolution,
bionet.software
Subject: Biologically Plausible Dynamic Artificial Neural Networks
Date: Tue, 05 Jan 93 05:53:57 GMT
Biologically Plausible Dynamic Artificial Neural Networks.
------------------------------------------------------------
A *Dynamic Artificial Neural Network* (DANN) [1]
[my acronym] possesses processing elements that are
created and/or annihilated, either in real time or as
some part of a development phase [2].
Of particular interest is the possibility of
constructing *biologically plausible* DANN's that
models developmental neurobiological strategies for
establishing and modifying processing elements and their
connections.
Work with cellular automata in modeling cell genesis and
cell pattern formation could be applicable to the design
of DANN topologies. Likewise, biological features that are
determined by genetic or evolutionary factors [3] would
also have a role to play.
Putting all this together with a view to constructing a
working DANN, possessing cognitive/behavioral attributes of
a biological system is a tall order; the modeling of nervous
systems in simple organisms may be the best approach when
dealing with a problem of such complexity [4].
Any comments, opinions or references in respect of the
above assertions would be most welcome.
References.
1. Ross, M. D., et al (1990); Toward Modeling a Dynamic
Biological Neural Network, Mathl Comput. Modeling,
Vol 13 No.7, pp97-105.
2. Lee, Tsu-Chang,(1991); Structure Level Adaptation for
Artificial Neural Networks, Kluwer Academic Publishers.
3. Edleman, Gerald,(1987); Neural Darwinism the Theory of
Neural Group Selection, Basic Books.
4. Beer, Randal, D,(1990); Intelligence as Adaptive Behavior
: An Experiment in Computational Neuroethology.
Academic Press.
OVERVIEW
The cross-posting strategy was successful in bringing about replies from
contributors working in the computer and life sciences.
Of those with a computer science background Jari Vaario is constructing
(and publishing) DANN's inspired by biological systems and an evolutionary
metaphor, John Mcdonnell also reports he has been able to construct
evolving networks and Larry Pyeatt has had some success at modeling these
processes. Mark Crossbie is interested in combining cellular automata and
genetic algorithms to build simple machines. Mark Tilden offers a
robotics viewpoint and suggests there may be some 'simple' and 'elegant'
solutions. Paul Verschure offers some references to his own work in this
field.
Life science replies came from Bernie French who suggested the nematode
as a suitable organism as model for a DANN. Stanley Zietz has suggested
modeling simple structures rather than organisms, and draws attention to
the work at NASA-Ames where they are attempting to make 'real' neural
networks. To counter this Bill Saidel points out the distinction between
the type of neuron being studied in NASA-Ames research and those in the
cortex.
Thanks to Ulf Andrick and Scott Fahlman for their replies on the Usenet.
I hope this discussion will initiate further debate and those who
participated will take the opportunity to make some informal contacts with
the other contributors.
Many thanks
Paul.
c/o AI Division
School of Computer Science
University of Westminster
115 New Cavendish Street
London W1M 8JS
UK.
-------------------------------------------------------------
READING
The Wolpert and Brown references are suitable for those who do not have a
strong background in biology. I would describe Langton's two Artificial
Life volumes as essential reading. Artificial Life II contains several
papers exploring evolving artificial neural networks. Beer's book is so
new I have not been able to look at a copy yet.
Title: Biological neural networks in invertebrate neuroethology and
robotics / edited by Randall D. Beer, Roy E. Ritzmann, Thomas McKenna.
Publication Info: Boston : Academic Press, c1993. Phys. Description: xi,
417 p. : ill. ; 24 cm. Series Name: Neural networks, foundations to
applications
Subjects: Neural circuitry. Subjects: Invertebrates--Physiology. Subjects:
Neural networks (Computer science)
ISBN: 0-12-084728-0
Author: Wolpert, L. (Lewis)
Title: The triumph of the embryo / Lewis Wolpert ; with illustrations
drawn by Debra Skinner.
Publication Info: Oxford [England] ; New York : Oxford University Press,
1991.
Phys. Description: vii, 211 p. : ill. ; 25 cm.
Subjects: Embryology, Human--Popular works.
ISBN: 0-19-854243-7 : $22.95
Author: Brown, M. C. (Michael Charles)
Title: Essentials of neural development / M.C. Brown, W.G. Hopkins, and
R.J. Keynes.
Publication Info: Cambridge ; New York : Cambridge University Press,
c1991. Phys. Description: x, 176 p. : ill. ; 24 cm.
Notes: Rev. ed. of: Development of nerve cells and their connections / by
W.G. Hopkins and M.C. Brown. 1984.
Subjects: Developmental neurology. Subjects: Nerves. Subjects: Neurons.
Subjects: Nerve endings. Subjects: Neurons.
ISBN: 0-521-37556-8
ISBN: 0-521-37698-X (pbk.)
Title: Artificial life II : the proceedings of an interdisciplinary
workshop on the synthesis and simulation of living systems held 1990 in
Los Alamos, New Mexico / edited by Christopher G. Langton ... [et al.].
Publication Info: Redwood City, Calif. : Addison-Wesley, 1991. Series
Name: Santa Fe Institute studies in the sciences of complexity proceedings
; v. 10
Notes: Proceedings of the Second Artificial Life Workshop.
Subjects: Biological systems--Computer simulation--Congresses. Subjects:
Biological systems--Simulation methods--Congresses.
ISBN: 0-201-52570-4
ISBN: 0-201-52571-2 (pbk.)
Author: Interdisciplinary Workshop on the Synthesis and Simulation of
Living Systems (1987 : (Los Alamos, N.M.)
Title: Artificial life : the proceedings of an Interdisciplinary Workshop
on the Synthesis and Simulation of Living Systems, held September, 1987,
in Los Alamos, New Mexico / Christopher G. Langton, editor.
Publication Info: Redwood City, Calif. : Addison-Wesley Pub. Co., Advanced
Book Program, c1989. Phys. Description: xxix, 655 p., [10] p. of plates :
ill. (some col.) ; 25 cm.
Series Name: Santa Fe Institute studies in the sciences of complexity ; v.
6
ISBN: 0-201-09346-4
ISBN: 0-201-09356-1 (pbk.)
----------------------------------------------------------------------
From: Jari Vaario <jari@ai.rcast.u-tokyo.ac.jp>
I have been working already awhile to create a method to describe
dynamical neural networks as you describe above. The latest journal papers
of mine are
Jari Vaario and Setsuo Ohsuga: An Emergent Construction of Adaptive Neural
Architectures, Heuristics - The Journal of Knowledge Engineering, vol. 5,
No 2, 1992.
Abstract:
In this paper a modeling method for an emergent construction
of neural network architectures is proposed. The structure
and behavior of neural networks are an emergent of small
construction and behavior rules, that in cooperation with
extrinsic signals, define the gradual growth of the neural
network structure and its adaptation capability (learning
behavior). The inspiration for the work is taken directly
from biological systems, even though the simulation itself
is not an exact biological simulation. The example used to
demonstrate the modeling method is also from biological
context: the sea hare Aplysia (a mollusk).
Jari Vaario, Koichi Hori and Setsuo Ohsuga: Toward Evolutionary Design of
Autonomous Systems, The International Journal in Computer Simulation, A
Special Issue on High Autonomous Systems, to be appear 1993.
Abstract:
An evolutionary method for designing autonomous systems is
proposed. The research is a computer exploration on how the
global behavior of autonomous systems can emerge from neural
circuits. The evolutionary approach is used to increase the
repertoire of behaviors.
Autonomous systems are viewed as organisms in an
environment. Each organism has its own set of production
rules, a genetic code, that gives birth to the neural
structure. Another set of production rules describe the
environmental factors. These production rules together give
rise to a neural network embedded in the organism model. The
neural network is the only means to direct reproduction.
This gives rise to intelligence, organisms which have
``more'' intelligent methods to reproduce will have a
relative advantage for survival.
Jari Vaario
Research Center for Advanced Science and Technology
University of Tokyo, Japan
JARI HAS OFFERED TO PROVIDE COPIES OF HIS PAPERS TO READERS OF
THIS BULLETIN. PLEASE CONTACT HIM BY EMAIL FOR FURTHER DETAILS.
---------------------------------------------------------------------
From: "John R. Mcdonnell" <mcdonn%bach.nosc.mil@nosc.mil>
I have been developing networks which "evolve" structure as well as the
connection strengths. This is done using an evolutionary programming
paradigm as a mechanism for stochastic search. One thing I have noticed
is that particular structures tend to dominate the population (EP is a
multi-agent stochastic search technique). THis has caused me to backtrack
a little and investigate the search space for simultaneously determining
model structure and parameters.
Nevertheless, I have been able to "evolve" networks for simple binary
mappings such as XOR, 3-bit parity, and the T-C problem. In the future I
am aiming at more general (feedforward) networks which do not have layers
per se, but neuron class {input, hidden, output}. Self-organizing of
sub-groups of neurons would be an interesting phenomenon to observe for,
say, image recognition problems. I think that to accomplish this a
distance metric between neurons might be necessary.
Fahlman's cascade-correlation architecture is very interesting. However,
it has the constraint that the neurons be fully connected to subsequent
neurons in the network. This might not be detrimental in that unimportant
connections could have very small weights. From an information
standpoint, these free parameters should be included in a cost function.
I do like his approach in adding additional hidden nodes.
As one last comment, when I "evolve" (I use the term loosely) networks for
the XOR mapping with an additional input of U(0,1) noise, this third
(noisy) input node has all of its outputs disconnected. This was a nice
result since inputs which contain no information can be disconnected.
John McDonnell
NCCOSC, RDT&E Division
Code 731
Information & Signal Processing Dept.
San Diego, CA 92152-5000
<mcdonn@bach.nosc.mil>
-----------------------------------------------------------------------
From: "Larry D. Pyeatt" <pyeatt@texaco.com>
I have just completed some code to allow modelling of DANN's. It allows
PE's to be created, destroyed, and reconnected at any time........
I have been using genetic algorithms to construct networks with desired
properties. Encoding the genes is a major problem.......
I have been thinking that it would be interesting to try to evolve or
create a simple "creature" which lives in a computer simulated world. The
"creature" would have a small set of inputs and responses with which to
interact with the simulated world. Once the "creature" has evolved
sufficiently, you could make its world richer and give it more neurons.
Eventually, you might have a respectably complex organism.
Larry D. Pyeatt The views expressed here are not
Internet : pyeatt@texaco.com those of my employer or of anyone
Voice : (713) 975-4056 that I know of with the possible
exception of myself.
-----------------------------------------------------------------------
From: Bernie French <btf64@cas.org>
I noticed your Usenet post on the use of simple organisms as a model to
produce a biologically plausible DANN. One organism that would seem to
fit your need for producing a DANN is the nematode, Caenorhabditis
elegans. The positions of neuronal processes as well as the neuronal
connectivity has been extensively mapped in this organism. The
development in terms of cellular fates is also well studied for the
nervous system. Integration of neuronal subsystems into the neuronal
processes during development have been studied. This would fit your
description of a DANN where processing elements are created as part of a
development phase. Further, the two sexes of C. elegans (male and
hermaphrodite) have different numbers of total neurons as adults, 302 for
the hermaphrodite. Howver, during development of the neuronal processes
there is no difference between the two sexes. During a certain stage in
development there is the production of sex-specific neurons. This
sex-specificity occurs by a process of programmed cell death, in which
certain neurons are "programmed" to die. This fits your description of a
DANN where processing elements are annihilated as part of a development
phase.
This organism also provides some spatial information, since some neuronal
cells undergo migration within the organism. Disruption of this migration
results in synaptic differences in the neuronal connectivity, with
corresponding differences in the organism response to external stimuli.
A good starting reference, if your interested in looking at this organism
as a model, is "The Nematode Caenorhabditis Elegans". The book is edited
by William B. Wood and published by Cold Spring Harbor Laboratory.
-- Bernie (btf64@cas.org)
-------------------------------------------------------------------------
From: "Mark J. Crosbie" <mcrosbie@unix1.tcd.ie>
As part of a project which I am working on for my degree, I am studying
how to evolve machines for solving simple problems.
I saw that Cellular Automata were able to evolve colonies of cells and
control how these cells lived or died, but I felt that this was not a
powerful enough representation of cells to be of use. I have combined the
CA approach with a Genetic Algorithm approach within each CA to give me
colonies of evolving cells which can modify their behaviour over time.
Each cell can be pre-programmed to perform a certain task (an adder or
multiplexor say) and the first hurdle in the project is getting these
cells to grow together and interconnect properly. I think that study of
how cells grow and interconnect will lead to not only a better
understanding of the nervous systems of living organisms, but also of how
to solve problems using these "Genetic Programming" techniques.
I feel that this idea overlaps somewhat with your DANN which you described
in comp.theory.cell-automata. Do you agree? Would you agree that building
simple machines by genetic means would be a better starting point for
experimentation than trying to simulate a complex nervous system? Given
enough of these cells and a complex enough interconnection system, do you
feel that a system will evolve which will equal a nervous system in
functionality and intelligence?
Mark Crosbie
mcrosbie@vax1.tcd.ie
Dept. of Computer Science
Trinity College, Dublin
Dublin 2
Eire.
--------------------------------------------------------------------
From: "Mark W. Tilden" <mwtilden@math.uwaterloo.ca>
Forcing a DANN, as you call it, through simple topological structures with
recursive sub-elements does tend towards complexity befitting a functional
organism with surprisingly few components. In my lab I have a variety of
robotic devices with adaptive nervous systems not exceeding the equivalent
of 10 neurons. These devices not only learn to walk from first principles
but can also adapt to many different circumstances including severe
personal damage. There are no processors involved; my most complex device
uses only 50 transistors for its entire spectrum of behavior, response and
control.
My point is that there are simple, elegant solutions to "constructing a
working DANN". More so than you might expect.
I'm sorry I have no papers to quote as I am awaiting patents, but I will
be at the Brussels Alife show in May with some of my devices, or you can
check out an article on me in the September 92 issue of Scientific
American.
Mark W. Tilden
M.F.C.F Hardware Design Lab.
U of Waterloo. Ont. Can, N2L-3G1
(519)885-1211 ext. 2454
-----------------------------------------------------------------------
From: Stanley Zietz <szietz@king.mcs.drexel.edu>
05 Jan 93
You may not need to model simple organisms, but simple structures. Since
you quote Muriel Ross's paper, you probably know that the Biocomputation
Center at NASA-Ames is exhaustively studying the linear accelerometer in
the inner ear as a prototypical system to make 'real' biological neural
networks.
07 Jan 93
To paraphrase many of the papers, it has been shown that the geometry of
the connection of the hair cells (before the Spike initiation zone) is
critical to the summation of events, and indeed there is a lot of
variability (perhaps throwing in a stochastic element into the network).
Also, as Muriel reported at headquarters, the results of analyzing the
space flight animals have shown that the number of synapses is very
plastic in microgravity - the number of synapses increase in space (and
decrease if you put the animals in hypergravity on a centrifuge).
Therefore the synapses (and presumably the electrical conduction in the
network) is responding to the input. Such self adaption is probably very
important in biological communications systems (Steve Grossberg has
expounded such a concept in 1979). We already know that such environment
driven events are important in development.
Dr. Stanley Zietz email szietz@mcs.drexel.edu
Assoc. Director - Biomed. Eng. and Sci. Inst. tel (215) - 895-2681
Assoc. Prof. - Mathematics and Computer Sciences Fax (215) - 895-4983
Drexel University - 32nd and Chestnut Sts. Phila., PA 19104 USA
also
Biocomputation Center
NASA-Ames Research Center
--------------------------------------------------------------------------
From: Bill Saidel <saidel@clam.rutgers.edu>
The question that remains [see ref. 1 in my post, also the Zietz reply]
is whether a hard wired (where hard is a relative term,
relative to say cortex in the cns) set of connections from a
peripheral sensory receptor to the peripheral afferent fiber and
then to the brain comprises a "net" in the same sense as
neural net is used. An analogous example would be from cones to
bipolar (and NOT to ganglion cell). My omission of ganglion cell
is simply that ganglion cell in the serial ordering of processing
would be equivalent to the first order vestibular neuron in the
vestibular nuclei of the hindbrain. This series of connections seems
to qualify as a genetically-determined (and I am probably
overconstraining the use of determined) set of connections
(receptor to next layer). I know of no learning treatment that
changes this layer of connections. However, manipulating the
sensory input in the retina by strobing does produce strange
deficits in frog and cat velocity detection (and other features).
The Ross argument has depended on looking at the EM level of connections.
These connections are all at the level of the sensory periphery and
so they are under normal circumstances, not manipulable. Nets in
the cortex are manipulable as are nnets in computer simulations.
However, Ross has also been involved in an intriguing study
of the structure of synaptic connections in rats or mice that were born
in space (I think) and found that the synaptic connections are
fewer when gravity is missing or diminished.
I prefer to think of that manipulation of the nervous periphery more
as an example of experimental epistomology because the change occurred due
to changes inthe biophysical structure of experience. Again, let me
point out that at the periphery the neuronal processing is
driven by biophysics. In the cns, neuronal processing is driven by
preceeding nerve cells that know nothing about the outside world.
Perhaps, this distinction is the one that I use to distinguish between
nets and constructions (a possibly artificial distinction but to my
mind, useful one).
Bill Saidel
Dept. of Biology
(609) 225-6336 (phone)Rutgers University
Camden, NJ 08102
saidel@clam.rutgers.edu (email)
----------------------------------------------------------------------------
EDITED REPRINT OF NEWSPOST WITH ADDITIONAL REFERENCE
From: Scott E. Fahlman <sef@sef-pmax.slisp.cs.cmu.edu>
I would just point out that adding or subtracting neurons from the
functional net does not necessarily correspond to adding, destroying, or
moving any physical neurons. If a physical neuron (or functional group of
neurons) has a lot of excess connections, invisible changes in the
synapses can effectively wire it into the net in a large number of
different ways, or effectively remove it.
Something like my dynamic (additive) Cascade-Correlation model can be
implemented by a sort of phase change, rather than a re-wiring of the net:
A candidate unit has a lot of trainable inputs, but it produces no output
(or all the potential recipients ignore its output). After a unit is
tenured, a specific pattern of input weights is frozen in, but now the
neuron does produce effective outputs. I don't know if such a phase
transition has been observed in biological neurons -- it would be
interesting to find out. Note that what I'm calling a "unit" might
correspond to a group of biological neurons rather than a single one.
Scott E. Fahlman Internet: sef+@cs.cmu.edu
Senior Research Scientist Phone: 412 268-2575
School of Computer Science Fax: 412 681-5739
Carnegie Mellon University Latitude: 40:26:33 N
5000 Forbes Avenue Longitude: 79:56:48 W
Pittsburgh, PA 15213
REFERENCE
LEARNING WITH LIMITED NUMERICAL PRECISION USING THE
CASCADE-CORRELATION ALGORITHM
HOEHFELD M, FAHLMAN SE
IEEE TRANSACTIONS ON NEURAL NETWORKS 1992 VOL.3 NO.4 PP.602-611
------------------------------------------------------------------------
From: Paul Verschure <verschur@ifi.unizh.ch>
The work we're doing would fit pretty well in your approach. Let me give
you some references:
Verschure, P.F.M.J., Krose, B, Pfeifer, R.(1992) Distributed Adaptive
Control: The self-organization of structured behavior. Robotics and
Autonomous Systems, 9, 181-196. (Control architectures for Autonomous
agents based on self-organizing model for classical conditioning).
Verschure, P.F.M.J., & Coolen, T. (1991) Adaptive Fields: Distributed
representations of clasically conditioned associations. Network, 2,
189-206. (Two neural models for reinforcement learning which do NOT rely
on supervised learning and local representations and incorporate some
general properties of neural functioning, the models are analyzed using
techniques from statistical physics and not with simulations)
Verschure, P.F.M.J. (1992) Taking connectionism seriously: The vague
promis of subsymbolism and an alternative. In Proc. 14th Ann. Conf. of the
Cog. Sci. Soc., 653-658, Hillsdale, N.J.: Erlbaum.
Paul Verschure AI lab Department of Computer Science
University Zurich-Irchel Tel + 41 - 1 - 257 43 06
Winterthurerstrasse 190 Fax + 41 - 1 - 363 00 35
CH - 8057 Zurich, Switzerland verschur@ifi.unizh.ch
------------------------------------------------------------------
For after all what is man in nature? A nothing in relation to infinity,
all in relation to nothing, a central point between nothing and all, and
infinitely far from understanding either. The ends of things and their
beginnings are impregnably concealed from him in an impenetrable secret.
He is equally incapable of seeing the nothingness out of which he was
drawn and the infinite in which he is engulfed.
Blaise Pascal (1623-1662)
--------------------------------------------------------------------------
Paul Fawcett | Internet: paulf@manor.demon.co.uk
London, UK. | tenec@westminster.ac.uk
--------------------------------------------------------------------------
------------------------------
End of Neuron Digest [Volume 11 Issue 13]
*****************************************