Copy Link
Add to Bookmark
Report
Neuron Digest Volume 09 Number 28
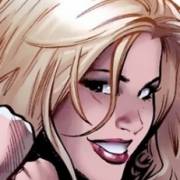
Neuron Digest Tuesday, 23 Jun 1992 Volume 9 : Issue 28
Today's Topics:
enquiry & remark
Answer: HELP TO START
Financial forecasting using ANN?
Research Fellowship
Pattern classifier - NN s/w available
Re. Financial Applications of NN
Grant deadline August 1
Send submissions, questions, address maintenance, and requests for old
issues to "neuron-request@cattell.psych.upenn.edu". The ftp archives are
available from cattell.psych.upenn.edu (128.91.2.173). Back issues
requested by mail will eventually be sent, but may take a while.
----------------------------------------------------------------------
Subject: enquiry & remark
From: TOTH@TAFF.CARDIFF.AC.UK
Date: Fri, 05 Jun 92 09:11:00 +0000
I. Enquiry
Dear members of the NN Community,
Could anybody advice me on how
- to acquire the following software :
AUTO:software for continuation problem in ordinary differential
equations; Authors: E. Doedel & J.P. Kernevez, 1986 (Caltech?)
or
- to access the technical report published at Caltech bearing
the same title
or
- to contact the authors.
Thank you for your help in advance.
T.I. Toth, Ph.D.
Dept. Physiol.
Univ. Wales Coll. Cardiff
P.O. Box 902
CARDIFF CF1 1SS
U.K.
tel.: +44(222)874088
fax: +44(222)641002
e-mail:toth@uk.ac.cardiff.taff
II. Remark on book advertisment
I find it quite useful that attention is drawn to newly published works
(textbooks, monographs, etc.) and would like this practice to be
continued. A short recension of the book in question could occasionally
prove useful, too, though I can see that this would put extra burden on
you and others involved.
------------------------------
Subject: Answer: HELP TO START
From: Marcus Speh <marcus@apollo.desy.de>
Date: Mon, 08 Jun 92 22:02:37 +0100
> I have asked for help to start at the subject of Neural Networks. I
> indicated that my field of interest is the use of the vision science in
> illuminating engineering. Though you have kindly announced my request I
> got no response. So, I would like to put it in another form: I would like
> to know introductory and tutorial papers on the modelling of the visual
> system using Neural Networks. Thanks a lot in advance,
[Here are two ref's which might fit into Fattah's request.
Unfortunately, I do not know whether these preprints from NYU (=New
York University) are already published, but I assume so. A. N. Redlich
is at Princeton now, I think. Please feel free to contact me if you have
further questions.]
J. J. Atick, A. Norman Redlich,
quantitative tests of a theory of retinal processing: contrast
sensitivity curves, IASSNS-HEP-90/51, NYU-NN-90/2.
J. J. Atick, Z. Li, A. Norman Redlich,
color coding and its interaction with spatiotemporal processing in the
retina, IASSNS-HEP-90/75, NYU-NN-90/3.
Marcus Speh
===================================================================
Marcus Speh INTERnet <marcus@apollo.desy.de>
II. Institut f. Theor. Physik BITnet <I02MSP@DHHDESY3>
Luruper Chaussee 149 DECnet <13313::SPEH>
2000 Hamburg 50/Germany Tel.(040)8998-2260 FAX:(040)8998-2267
------------------------------
Subject: Financial forecasting using ANN?
From: Marcus Speh <marcus@apollo.desy.de>
Date: Mon, 08 Jun 92 22:08:17 +0100
The following paper was announced in the Connectionist's mailing list.
It might be what you meant.
------------------------------ CUT HERE -------------------------------
Date: Wed, 20 May 92 16:54:53 SST
From: Goh Tiong Hwee <thgoh@iss.nus.sg>
I have place the following paper in the neuroprose archive.
Hardcopy request by snailmail to me at the institute.
Thanks to Steve Pollack for availing the archive service.
Neural Networks And Genetic Algorithm For Economic Forecasting
Francis Wong, PanYong Tan
Institute of Systems Science
National University of Singapore
Abstract: This paper describes the application of an enhanced neural
network and genetic algorithm to economic forecasting. Our proposed
approach has several significant advantages over conventional forecasting
methods such as regression and the Box-Jenkins methods. Apart from being
simple and fast in learning, a major advantage is that no assumption need
to be made about the underlying function or model, since the neural
networks is able to extract hidden information from the historical data.
In addition, the enhanced neural network offers selective activation and
training of neurons based on the instantaneous causal relationship
between the current set of input training data and the output target.
This causal relationship is represented by the Accumulated Input Error
(AIE) indices, which are computed based on the accumulated errors
back-propagated to the input layers during training. The AIE indices are
used in the selection of neurons for activation and training. Training
time can be reduced significantly, especially for large networks designed
to capture temporal information. Although neural networks represent a
promising alternative for forecasting, the problem of network design
remains a bottleneck that could impair widespread applications in
practice. The genetic algorithm is used to evolved optimal neural network
architectures automatically, thus eliminating the many pitfalls
associated with human-engineering approaches. The proposed concepts and
design paradigm were tested on serveral real applications ( Please email
thgoh@iss.nus.sg for a copy of the software ), including the forecast of
GDP, air passenger arrival and currency exchage rates.
ftp Instructions:
unix> ftp archive.cis.ohio-state.edu (or 128.146.8.52)
Name: anonymous
Password: neuron
ftp> cd pub/neuroprose
ftp> binary
ftp> get wong.nnga.ps.Z
ftp> quit
__________________________________________________________
Tiong Hwee Goh
Institute of Systems Science
National University of Singapore
Heng Mui Keng Terrace
Kent Ridge
Singapore 0511.
Telephone:(65)7726214
Fax :(65)7782571
------------------------------
Subject: Research Fellowship
From: STAY8026@bureau.ucc.ie
Date: Tue, 09 Jun 92 09:14:00 +0000
THE FOLLOWING IS LIKELY TO BE OF INTEREST TO EUROPEAN RESEARCHERS
Dermot Barnes and I intend to make an application to the EC Human Capital
and Mobility scheme for funding toward a post doctoral research
fellowship to work on a project on connectionist simulations of recent
developments in human learning which have implications for our
understanding of inference.
We would be interested in hearing from any post-doc connectionist who
might like to put in an application for such a fellowship in tandem with
ours. Our reading of the `Euro-blurb' is that the closing date for joint
applications is 29 June, which is very close, but that individuals
wishing to apply for a fellowship can do so continuously throughout
1992-94 with periodic selection every four months.
For more information contact us by e-mail or at the address below.
Dermot will be at the Quantitative Aspects of Behavior Meeting in Harvard
later this week and I will be at the Belfast Neural Nets Meeting at the
end of the month.
P.J. Hampson
Department of Applied Psychology
University College
Cork
Ireland
tel 353-21-276871 (ext 2101)
fax 353-21-270439
e-mail stay8026@iruccvax.ucc.ie
------------------------------
Subject: Pattern classifier - NN s/w available
From: "Atilla Gunhan" <atilla@ifi.uib.no>
Date: Tue, 09 Jun 92 14:36:48 +0100
``PATTERN CLASSIFIER'', NEW UNSUPERVISED NEURAL NETWORK ALGORITHM .
--------------------------------------------------------------------
The software is available from FTP site at the University of
Texas at Arlington (FTP: 129.107.2.20)
How to get Pattern Classifier
..............................
1. Create an FTP connection
>ftp 129.107.2.20
2. Log in as user "anonymous" with password your username@your-site
3. Change to the requisite directory
> cd pub/neural/annsim
4. Set binary mode by typing the command
>bin
5. Get the file
>get ptclass.zip
6. Disconnect from server
>quit
* This program is written for PC's. The program has unique quality
of graphical documentations and simulation abilities.
* ``Pattern classifier'' is a new unsupervised neural network which
is developed by Atilla Gunhan, Department of Information Science,
University of Bergen, Norway.
Following papers are recommended to read.
Gunhan E. Atilla, Csernai L. P. Randrup J., ``Unsupervised Competitive
Learning in Neural Networks'', International Journal of Neural Systems,
World Scientific, London, Vol. 1, No. 2, 177-186
Gunhan E. Atilla, ``Pattern Classifier, An Alternative Method of
Unsupervised Learning'', Neural Network World, International Journal on
Neural and Mass-Parallel Computing, Czechoslovakia, Vol. 1, No. 6,
349-354, (December 1991)
* Please if there are any questions, do not hesitate to contact me.
Atilla E. Gunhan
Department of Information Science,
University of Bergen,
Hightechnology center, N-5020 Bergen, Norway
E-mail: gunhan@cc.uib.no
Telefax: (47-5) 544107
**********************
Pattern classifier: *
**********************
This multi layer network has 64 input units, a hidden layer with 64
hidden units and an output layer with 64 units. The layers are fully
interconnected to the next layer. The output and hidden layers have
internal lateral inhibitions.
The first part that is between input units and hidden layer accepts input
patterns one at a time In other words, when we present the first pattern
to this part, only this part of the network will be trained until we
obtain internal representations in the hidden unit for this pattern.
Before we can present the second pattern this part of the network will be
initialized. After we have obtained an internal representation for the
first pattern in the hidden layer, this representation presented to the
second part of the network as an input for final classification of the
pattern. There are two possible preprocessing in the first layer. We can
train the network until we obtain internal representations of the input
patterns. In this type of training, it is possible that patterns can have
common elements. In the second type of training, we can train the
network by eliminating common activated units (this part is not
implemented in the program) until we obtain unique representations for
each input patterns. In this second method patterns will be reduced into
their orthogonal representations.
The second part of the network can be considered as another single layer
network between the hidden layer and output layer. The function of this
second network differs from the first part of the network in terms of the
way of training. This part accepts the internal representation as input
and the layer is trained untill the pattern is classified. The next
internal representation, which is the result of the second pattern that
is presented in the first part, will also be accepted as second input
pattern to the second part of the network without any initialization. In
this way, the second part of the network is capable of training and
memorizing a sequence of patterns as in traditional training.
A ``neighbour inhibition'' method with an Eight-directional Inhibition
Strategy is used in the layer of the first part of the network that is in
fact the hidden layer for the whole network. In the program user can
define the neighbour distance in X and Y directions. Different distance
on different directions are the most effective way to carry input
informations into the next layers. In this strategy most active unit
inhibits the activity of its closest neighbour units. Units in the layer
organized in a two dimensional array. In the output layer of the network,
the ``Winner-take-all'' method is used.
Features of the network
- -----------------------
The learning algorithm and architecture of the network
explained above are different from other network models.
The advantages of the two layer model presented above are:
Ability of preprocessing the incoming pattern.
- ----------------------------------------------
The network has ability to preprocess the incoming patterns
and reduce the dimensions and the linear dependency among these
patterns then memorize them. Experiments indicate that the network
has ability to classify all input vectors as long as those vectors
have less than 40\% common elements with each other.
Network architecture and learning algorithm are
similar to biological mechanisms.
- -------------------------------------------------
The learning algorithm is based on neurophysiological activity of real
neurons. If the membrane potential, v, of a neuron at a given time
exceeds a certain threshold value, theta, then the neuron becomes active
(fired) and inhibits its neighbours with a certain inhibition value. Only
the weights of the active neuron are adjusted. Active neuron also resets
itself into resting membrane potential value.
Network accepts repeated patterns.
- -----------------------------------
Repeated patterns during training do not cause any side effect. They
activate always the same output units.
Values of parameters are fixed.
- ------------------------------------
Choice of parameter values for learning and the number of training
iterations are fixed. The network may be trained with a value of learning
rate 0.1 and 150 cycle for each pattern for each layer. If distance of
neighbour neurons are bigger then 1 then you can reduce the training
cycle of the network (min. 50 cyc.). These values are valid for any type
of pattern. Inhibition values between the units are set to 0.9 when the
method is neighbour inhibition.
------------------------------
Subject: Re. Financial Applications of NN
From: dmt@sara.inesc.pt (Duarte Trigueiros)
Date: Tue, 09 Jun 92 18:36:41 -0200
Regarding Dr. Ramos question, I know of a forthcoming book of readings
entitled "Neural Networks in Business". The publisher is Boyd and Fraser,
Boston, MA, and the editors are Tripi, R. and Turban, E. It should be
published during 1992
I can also apportion, from the same series, two references on financial
applications of NN. They represent the two most usual applications of NN
in finance: Forecasting and classification.
@inproceedings(Dudda90,
author = "Dudda, S. and Shekhar, S.",
title = "A Non-Conservative Application of Neural Networks",
Booktitle = "Investment Management, Decision Support and Expert
Systems",
Year = 1990,
Editor = "Trippi, R. and Turban, E.",
Publisher = "Boyd and Fraser",
Pages = "271-282" )
@inproceedings(White90,
author = "White, H.",
title = "Economic Prediction Using Neural Networks: The case of
IBM Daily Returns",
Booktitle = "Investment Management, Decision Support and Expert
Systems",
Year = 1990,
Editor = "Trippi, R. and Turban, E.",
Publisher = "Boyd and Fraser",
Pages = "283-292" )
I've been testing the ability of the MLP to extract knowledge from
accounting information. I found that the MLP is very promising in
financial statement analysis since it can point out which ratios are more
appropriate for a given task. Some results are published:
@inproceedings(Trigueiros91,
author = "Trigueiros, D. and Berry, R.",
title = "The Application of Neural Network Based Methods to the
Extraction of knowledge From Accounting Reports",
Booktitle = "Proceedings of the $24^{th}$ Hawaii International
Conference on Systems Science",
Year = 1991,
Publisher = "IEEE Computer Science Press",
Editor = "Nunamaker, E. and Sprague, R.")
The Hawaii International Conference on Systems Science referred to above
has a minitrack devoted to the use of NN in organisations. Several
interesting papers on the use of NN in finance can be found in its
proceedings.
Duarte Trigueiros
INESC,
R. Alves Redol 9 2 esq
Lisbon 1096 CODEX,
Portugal
E-mail dmt@sara.inesc.pt
------------------------------
Subject: Grant deadline August 1
From: Terry Sejnowski <terry@helmholtz.sdsc.edu>
Date: Thu, 18 Jun 92 16:40:05 -0800
COGNITIVE NEUROSCIENCE - Individual Grants in Aid
The McDonnell-Pew Program in Cognitive Neuroscience is accepting
proposals for support of research and training in cognitive neuroscience.
Preference is given for projects that are not currently funded and are
interdisciplinary, involving at least two areas among clinical and basic
neurosciences, computer science, psychology, linguistics and philosophy.
Research support is limited to $30,000 a year for two years.
Postdoctoral grants are limted to three years. Graduate student support
is not available.
Applications should be postmarked by August 1, 1992 to:
Dr. George Miller
McDonnell-Pew Program in Cognitive Neuroscience
Green Hall, 1-N-6
Princeton University
Princeton, NJ 08544-1010
For more information call (609) 258-5014, FAX (609) 258-3031
or e-mail cns@clarity.princeton.edu
------------------------------
End of Neuron Digest [Volume 9 Issue 28]
****************************************