Copy Link
Add to Bookmark
Report
Neuron Digest Volume 10 Number 20
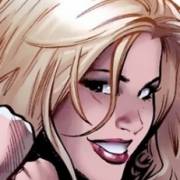
Neuron Digest Saturday, 21 Nov 1992 Volume 10 : Issue 20
Today's Topics:
workshop announcement
Call for Papers, NNSP'93
Call for Participation
NIPS 92 Workshop on Training Issues
Post-NIPS Robot Learning workshop program
Send submissions, questions, address maintenance, and requests for old
issues to "neuron-request@cattell.psych.upenn.edu". The ftp archives are
available from cattell.psych.upenn.edu (130.91.68.31). Back issues
requested by mail will eventually be sent, but may take a while.
----------------------------------------------------------------------
Subject: workshop announcement
From: Joachim Beer <beer@ICSI.Berkeley.EDU>
Date: Tue, 03 Nov 92 10:47:35 -0800
***************************************************
* Workshop on Software & Programming Issues for *
* Connectionist Supercomputers *
***************************************************
April 19-20, 1993
at
International Computer Science Institute (ICSI)
1947 Center Street
Berkeley, CA 94704
Sponsored by:
Adaptive Solutions, Inc.
ICSI
Siemens AG
The goal of this workshop is to bring together connectionist researchers
to address software and programming issues in the framework of large
scale connectionist systems. Scope and technical theme of the workshop is
outlined below. Due to space considerations the workshop will be by
invitation only. Interested parties are encouraged to submit a one-page
proposal outlinig their work in this area by January 31. Submissions
should be send to ICSI at the address above or by e-mail to
beer@icsi.berkeley.edu
The increased importance of ANNs for elucidating deep conceptual
questions in artificial intelligence and their potential for attacking
real world problems warrant the design and construction of connectionist
supercomputers. Several research labs have undertaken to develop such
machines. These machines will allow researchers to investigate and apply
ANNs on a scale which up to now was not computationally feasible. As with
other parallel hardware, the main problem is adequate software for
connectionist supercomputers.
Most "solutions" offer isolated instances which deal only with a limited
class of particular ANN algorithms rather than providing a comprehensive
programming model for this new paradigm. This approach was acceptable
for small and structurally simple ANNs. However, to fully utilize the
emerging connectionist supercomputers an expressive, clean, and flexible
software environment is called for. This is being recognized by the
developers of the connectionist supercomputers, and an intergral part of
these projects is the development of an appropriate software environment.
While each connectionist supercomputer project has unique goals and
possibly a focus on particular application areas, it would nevertheless
be very fruitful to compare how the fundamental software questions that
everybody in this field faces are being approached. The following
(incomplete) list outlines some of the issues:
* Embedding connectionist systems in traditional
software environments, eg. client/server models
vs. integrated "seamless" environments.
* ANN description languages
* Handling of sparse and irregular nets
* Facilities for mapping nets onto the underlying
architecture
* Handling of complete applications including embedded
non-connectionist instructions
* Should there be a machine independent intermediate
language? What would be the disadvantages?
* Software issues for dedicated embedded ANNs vs.
"general purpose" connectionist supercomputers.
* Graphical user interfaces for ANN systems
* System support for high I/O rates (while this is
a general question in comp. sci. there are nevertheless
some unique problems for ANN systems in dealing
with large external data sets).
------------------------------
Subject: Call for Papers, NNSP'93
From: "Gary M. Kuhn" <gmk@osprey.siemens.com>
Date: Wed, 04 Nov 92 12:33:46 -0500
CALL FOR PAPERS
_______________
1993 IEEE Workshop on Neural Networks for Signal Processing
September 7-9, 1993 Baltimore, MD, USA
Sponsored by the IEEE Technical Committee on Neural Networks
in cooperation with the IEEE Neural Networks Council
The third of a series of IEEE workshops on Neural Networks for Signal
Processing will be held at the Maritime Institute of Technology and
Graduate Studies, Linthicum, Maryland, USA, in September of 1993. Papers
are solicited for, but not limited to, the following topics:
1. Applications:
Image processing and understanding, speech recognition,
communications, sensor fusion, medical diagnoses, nonlinear adaptive
filtering and other general signal processing and pattern recognition
topics.
2. Theory:
Neural network system theory, identification and spectral estimation,
and learning theory and algorithms.
3. Implementation:
Digital, analog, and hybrid technologies and system development.
Prospective authors are invited to submit 4 copies of extended summaries
of no more than 6 pages. The top of the first page of the summary should
include a title, authors' names, affiliations, address, telephone and
fax numbers and email address if any. Camera-ready full papers
of accepted proposals will be published in a hard-bound volume by IEEE
and distributed at the workshop. Due to workshop facility constraints,
attendance will be limited with priority given to those who submit
written technical contributions. For further information, please
contact Karin Cermele at the NNSP'93 Princeton office,
(Tel.) +1 609 734 3383, (Fax) +1 609 734 6565, (e-mail)
kic@learning.siemens.com.
PLEASE SEND PAPER SUBMISSIONS TO:
_______________
NNSP'93
Siemens Corporate Research
755 College Road East
Princeton, NJ 08540
USA
SCHEDULE
_______________
Submission of extended summary: February 15
Notification of acceptance: April 19
Submission of photo-ready paper: June 1
Advanced registration, before: June 1
WORKSHOP COMMITTEE
_______________
General Chairs
Gary Kuhn Barbara Yoon
Siemens Corporate Research DARPA-MTO
755 College Road East 3701 N. Fairfax Dr.
Princeton, NJ 08540, USA Arlington, VA 22203-1714 USA
gmk@learning.siemens.com byoon@a.darpa.mil
Program Chair Proceedings Chair
Rama Chellappa Candace Kamm
Dept. of Electrical Engineering Box 1910
University of Maryland Bellcore, 445 South Street
College Park, MD 20742, USA Morristown, NJ 07962, USA
chella@eng.umd.edu cak@bellcore.com
Finance Chair
Raymond Watrous
Siemens Corporate Research
755 College Road East
Princeton, NJ 08540, USA
watrous@learning.siemens.com
Program Committee
Joshua Alspector John Makhoul
Les Atlas B.S. Manjunath
Charles Bachmann Tomaso Poggio
Gerard Chollet Jose Principe
Frank Fallside Ulrich Ramacher
Lee Giles Noboru Sonehara
S.J. Hanson Eduardo Sontag
Y.H. Hu J.A.A. Sorensen
B.H. Juang Yoh'ichi Tohkura
Shigeru Katagiri Christoph von der Malsburg
S.Y. Kung Christian Wellekens
Yann LeCun
------------------------------
Subject: Call for Participation
From: "Dr. Francis T. Marchese" <MARCHESF%PACEVM.BITNET@BITNET.CC.CMU.EDU>
Date: 07 Nov 92 16:28:14 -0500
*** Call For Participation ***
Conference on Understanding Images
Sponsored By
NYC ACM/SIGGRAPH
and
Pace University's
School of Computer Science and Information Systems
To Be Held at:
Pace University
New York City, New York
May 21-22,1993
Artists, designers, scientists, engineers and educators share the problem
of moving information from one mind to another. Traditionally, they have
used pictures, words, demonstrations, music and dance to communicate
imagery. However, expressing complex notions such as God and infinity or a
seemingly well defined concept such as a flower can present challenges
which far exceed their technical skills.
The explosive use of computers as visualization and expression tools has
compounded this problem. In hypermedia, multimedia and virtual reality
systems vast amounts of information confront the observer or participant.
Wading through a multitude of simultaneous images and sounds in possibly
unfamiliar representations, a confounded user asks: What does it all mean?
Since image construction, transmission, reception, decipherment and
ultimate understanding are complex tasks strongly influenced by physiology,
education and culture; and since electronic media radically amplify each
processing step, then we, as electronic communicators, must determine the
fundamental paradigms for composing imagery for understanding.
Therefore, the purpose of this conference is to bring together a breadth of
disciplines, including, but not limited to, the physical, biological and
computational sciences, technology, art, psychology, philosophy and
education, in order to define and discuss the issues essential to image
understanding within the computer graphics context. To this end we seek
proposals for individual presentations, panel discussions, static displays,
interactive environments, performances and beyond.
Submissions:
Contributors are requested to submit a one page proposal by January 15,
1993. Accepted presentations will be included in the proceedings.
Direct all inquires and submissions to:
Professor Francis T. Marchese
Department of Computer Science
Pace University
New York, NY 10038 USA
Email: MARCHESF@PACEVM.Bitnet
Phone: 212-346-1803
Fax: 212-346-1933
------------------------------
Subject: NIPS 92 Workshop on Training Issues
From: "Scott A. Markel x2683" <sam@vaxserv.sarnoff.com>
Date: Thu, 19 Nov 92 11:24:12 -0500
**************************** NIPS 92 Workshop ****************************
"Computational Issues in Neural Network Training"
or
Why is Back-Propagation Still So Popular?
*******************************************************************************
Roger Crane and I are are leading a NIPS '92 workshop on "Computational
Issues in Neural Network Training". Our workshop will be on Saturday, 5
December, the second of two days of workshops in Vail.
The discussion will focus on optimization techniques currently used by
neural net researchers, and include some other techniques that are
available. Back- propagation is still the optimization technique of
choice even though there are obvious problems in training with BP: speed,
convergence, ... . Several innovative algorithms have been proposed by
the neural net community to improve upon BP, e.g., Scott Fahlman's
QuickProp. We feel that there are classical optimization techniques that
are superior to back-propagation. In fact, gradient descent (BP) fell
out of favor with the mathematical optimization folks way backin the
60's! So why is BP still so popular?
Topics along these lines include:
* Why are classical methods generally ignored?
* Computational speed
* Convergence criteria (or lack thereof!)
Broader issues to be discussed include:
* Local minima
* Selection of starting points
* Conditioning (for higher order methods)
* Characterization of the error surface
If you would like to present something on any of these or similar topics,
please contact me by e-mail and we can discuss details.
Workshops are scheduled for a total of four hours. We're allowing for
approximately 8 presentations of 10-20 minutes each, since we want to
make sure that ample time is reserved for discussion and informal
presentations. We will encourage (incite) lively audience participation.
By the way, none of the NIPS workshops are limited to presenters only.
People who want to show up and just listen are more than welcome.
Scott Markel Computational Science Research
smarkel@sarnoff.com David Sarnoff Research Center
Tel. 609-734-2683 CN 5300
FAX 609-734-2662 Princeton, NJ 08543-5300
------------------------------
Subject: Post-NIPS Robot Learning workshop program
From: David Cohn <cohn@psyche.mit.edu>
Date: Wed, 04 Nov 92 15:52:45 -0500
PROGRAM FOR THE POST-NIPS WORKSHOP "ROBOT LEARNING"
Vail, Colorado, Dec 5th, 1992
NIPS=92 Workshop: Robot Learning
=================
Intended Audience: Connectionists and Non-Connectionists in Robotics,
================== Control, and Active Learning
Organizers:
===========
Sebastian Thrun (CMU) Tom Mitchell (CMU) David Cohn (MIT)
thrun@cs.cmu.edu mitchell@cs.cmu.edu cohn@psyche.mit.edu
Program:
========
Robot learning has grasped the attention of many researchers over the
past few years. Previous robotics research has demonstrated the
difficulty of manually encoding sufficiently accurate models of the robot
and its environment to succeed at complex tasks. Recently a wide variety
of learning techniques ranging from statistical calibration techniques to
neural networks and reinforcement learning have been applied to problems
of perception, modeling and control. Robot learning is characterized by
sensor noise, control error, dynamically changing environments and the
opportunity for learning by experimentation.
This workshop will provide a forum for researchers active in the area of
robot learning and related fields. It will include informal tutorials
and presentations of recent results, given by experts in this field, as
well as significant time for open discussion. Problems to be considered
include: How can current learning robot techniques scale to more complex
domains, characterized by massive sensor input, complex causal
interactions, and long time scales? How can previously acquired
knowledge accelerate subsequent learning? What representations are
appropriate and how can they be learned?
Although each session has listed "speakers," the intent is that each
speaker will not simply present their own work, but will introduce
their work interactively, as a launching point for group discussion on
their chosen area. After all speakers have finished, the remaining
time will be used to discuss at length issues that the group feels
need most urgently to be addressed.
Below, we have listed the tentative agenda, which is followed by brief
abstracts of each author's topic. For those who wish to get a head
start on the workshop, we have included a list of references and/or
recommended readings, some of which are available by anonymous ftp.
=====================================================================
=====================================================================
AGENDA
=====================================================================
=====================================================================
SESSION ONE (early morning session), 7:30 - 9:30:
-------------------------------------------------
TITLE: "Robot learning: scaling up and state of the art"
Keynote speaker: Chris Atkeson (30 min)
"Paradigms for Robot Learning"
Speakers: Steve Hanson (15 min)
(title to be announced)
Satinder Singh (15 min)
Behavior-Based Reinforcement Learning
Andrew W. Moore(15 min)
The Parti-Game Algorithm for Variable
Resolution Reinforcement Learning
Richard Yee (15 min)
Building Abstractions to Accelerate
Weak Learners
SESSION TWO (apres-ski session), 4:30 - 6:30:
---------------------------------------------
PANEL: "Robot learning: Where are the new ideas coming from?"
Keynote speaker: Andy Barto (30 min)
Speakers: Tom Mitchell (10 min each)
Chris Atkeson
Dean Pomerleau
Steve Suddarth
=====================================================================
=====================================================================
ABSTRACTS
=====================================================================
Session 1: Scaling up and the state of the art
When: Saturday, Dec 5, 7:30-9:30 a.m.
=====================================================================
=====================================================================
Keynote: Chris Atkeson (cga@ai.mit.edu)
Title: Paradigms for Robot Learning
Abstract: This talk will survey a variety of robot learning tasks and
learning paradigms to perform those tasks. The tasks include pattern
classification, regression/function approximation, root finding,
function optimization, designing feedback controllers, trajectory
following, stochastic modeling, stochastic control, and strategy
generation. Given this wide range of tasks it seems reasonable to ask
if there is any commonality among them, or any way in which solving one
task might make other tasks easier to perform. In our own work we have
typically taken an indirect approach: our learning algorithms explicitly
form models, and then solve the problem using algorithms that assume
complete knowledge. It is not at all clear which learning tasks are
best dealt with using an indirect approach, and which are handled better
with a direct approach in which the control strategy is learned
directly. Nor is it clear how to cope with uncertainty and incomplete
knowledge, either by modeling it explicitly, using stochastic models, or
using game theory and assuming a malevolent world. I hope to provoke a
discussion on these issues.
======================================================================
Presenter: Satinder Pal Singh (singh@cs.umass.edu)
Title: Behavior-Based Reinforcement Learning
Abstract: Control architectures based on reinforcement learning have
been successfully applied to agents/robots that use their repertoire
of primitive control actions to achieve goals in an external
environment. The optimal policy for any goal is a state-dependent
composition of the given "primitive" policies (a primitive policy "A"
assigns action A to every state). In that sense, the primitive
policies form the "basis" set from which optimal solutions can be
"composed". I argue that reinforcement learning can be greatly
accelerated by redefining the basis set of policies available to the
agent. These redefined basis policies should correspond to
"behaviors" that are useful across the set of tasks faced by the
agent. Behavior-based RL, i.e., the application of RL to
behavior-based robotics (ref Brooks), has several advantages: it can
drastically reduce the effective dimensionality of the action space,
it provides a framework for incorporating prior knowledge into RL
architectures, it provides a technique for achieving transfer of
learning, and finally by restricting the rules of composition and the
types of behaviors it may become possible to perform "robust"
reinforcement learning. I will provide examples from my own work and
that of others to illustrate these ideas.
(Refs 4, 5, 6)
======================================================================
Presenter: Andrew W. Moore (awm@ai.mit.edu)
Title The Parti-Game Algorithm for Variable Resolution
Reinforcement Learning
Can we efficiently learn in continuous state-spaces, while requiring
only relatively few real-world experienvces during the learning stage?
Dividing a continuous state-space into a fine grid can mean a
tragically large number of unnecessary experiences, while a coarse
grid or parametric representation can become stuck. This talk
overviews a new algorithm which, in real time, tries to adaptively
alter the resolution of a state space partitioning to be coarse where
it can and fine where it must to be if it is to avoid becoming stuck.
The key idea turns out to be the treatment of the problem as a game
instead of a Markov decision task.
Possible prior reading:
Ref 7 (Overview of some other uses of kd-trees in Machine learning)
Ref 8 (A non-real-time algorithm which uses a different partitioning strategy)
Ref 9 (A search control technique which Parti-Game uses)
Refs 9, 10
======================================================================
Presenter: Richard Yee, (yee@cs.umass.edu)
Title: Building Abstractions to Accelerate Weak Learners
Abstract: Learning methods based on dynamic programming (DP) are
promising approaches to the problem of controlling dynamical systems.
Practical DP-based learning will require function approximation
methods that are well-suited for learning optimal value functions,
which map system states into numeric estimates of utility. Such
approximation problems are generally characterized by non-stationary,
dependent training data and, in many cases, little prospect for
incorporating strong {\em a priori\/} learning biases. Consequently.
this talk considers learning approaches that begin weakly (e.g., using
rote memorization) but strengthen their learning biases as experiences
accrue. Abstracting from stored experiences should accelerate
learning by improving generalization. Bootstrapping such abstraction
processes (cf.\ "hypothesis boosting") might be a practical means for
scaling DP-based learning across a wide variety of applications.
(Refs 1, 2, 3, 4)
=====================================================================
Session 2: Where are the new ideas coming from?
When: Saturday, Dec 5, 4:30-6:30 p.m.
=====================================================================
=====================================================================
Keynote: Andrew G. Barto (barto@cs.umass.edu)
Title: Reinforcement Learning Theory
Although reinforcement learning is being studied more widely than ever
before, especially methods based on approximating dynamic programming
(DP), its theoretical foundations are not yet highly developed. In
this talk, I discuss what I percieve to be the current state and the
missing links in this theory. This topic raises such questions as the
following: Just what is DP-based reinforcement learning from a
mathematical perspective? What is the relationship between DP-based
reinforcement learning and other methods for approximating DP? What
theoretical justification exists for combining function approximation
methods (such as artificial neural networks) with DP-based learning?
What kinds of problems are best suited to DP-based reinforcement
learning? Is theory important?
=====================================================================
Presenter: Dean Pomerleau
Title: Combining artificial neural networks and symbolic
processing for autonomous robot guidance
Artificial neural networks are capable of performing the reactive
aspects of autonomous driving, such as staying on the road and avoiding
obstacles. This talk describes an efficient technique for training
individual networks to perform these reactive driving tasks. But
driving requires more than a collection of isolated capabilities. To
achieve true autonomy, a system must determine which capabilities should
be employed in the current situation to achieve its objectives. Such
goal directed behavior is difficult to implement in an entirely
connectionist system. This talk describes a rule-based technique for
combining multiple artificial neural networks with map-based symbolic
reasoning to achieve high level behaviors. The resulting system is not
only able to stay on the road, it is able follow a route to a
predetermined destination, turning appropriately at intersections and
stopping when it has reached its goal.
(Refs 11, 12, 13, 14, 15)
=====================================================================
=====================================================================
References
=====================================================================
=====================================================================
(#1) Yee, Richard, "Abstraction in Control Learning", Department of
Computer and Information Science, University of Massachusetts,
Amherst, MA 01003, COINS Technical Report 92-16, March 1992.
anonymous ftp: envy.cs.umass.edu:pub/yee.abstrn.ps.Z
(#2) Barto, Andrew G. and Richard S. Sutton and Christopher J. C. H.
Watkins, Sequential decision problems and neural networks, in Advances
in Neural Information Processing Systems 2, 1990, Touretzky, D. S.,
ed.
(#3) Barto, Andrew G. and Richard S. Sutton and Christopher J. C. H.
Watkins", Learning and Sequential Decision Making, in Learning and
Computational Neuroscience: Foundations of Adaptive Networks, 1990.
anonymous ftp:
archive.cis.ohio-state.edu:pub/neuroprose/barto.sequential_decisions.ps.Z
(#4) Barto, Andrew G. and Steven J. Bradtke and Satinder Pal Singh,
Real-time learning and control using asynchronous dynamic programming,
Computer and Information Science, University of Massachusetts,
Amherst, MA 01003, COINS Technical Report TR-91-57, August 1991.
anonymous ftp:
archive.cis.ohio-state.edu:pub/neuroprose/barto.realtime-dp.ps.Z
(#5) Singh, S.P.," Transfer of Learning by Composing Solutions for Elemental
Sequential Tasks, Machine Learning, 8:(3/4):323-339, May 1992.
anonymous ftp: envy.cs.umass.edu:pub/singh-compose.ps.Z
(#6) Singh, S.P., "Scaling reinforcement learning algorithms by
learning variable temporal resolution models, Proceedings of the Ninth
Machine Learning Conference, D. Sleeman and P. Edwards, eds., July
1992.
anonymous ftp: envy.cs.umass.edu:pub/singh-scaling.ps.Z
(#7) S. M. Omohundro, Efficient Algorithms with Neural Network
Behaviour, Journal of Complex Systems, Vol 1, No 2, pp 273-347, 1987.
(#8) A. W. Moore, Variable Resolution Dynamic Programming: Efficiently
Learning Action Maps in Multivariate Real-valued State-spaces, in
"Machine Learning: Proceedings of the Eighth International Workshop",
edited by Birnbaum, L. and Collins, G., published by Morgan Kaufman.
June 1991.
(#9) A. W. Moore and C. G. Atkeson, Memory-based Reinforcement
Learning: Converging with Less Data and Less Real Time, 1992. See the
NIPS92 talk or else preprints available by request to awm@ai.mit.edu
(#10) J. Peng and R. J. Williams, Efficient Search Control in Dyna,
College of Computer Science, Northeastern University, March, 1992
(#11) Pomerleau, D.A., Gowdy, J., Thorpe, C.E. (1991) Combining artificial
neural networks and symbolic processing for autonomous robot guidance.
In {\it Engineering Applications of Artificial Intelligence, 4:4} pp.
279-285.
(#12) Pomerleau, D.A. (1991) Efficient Training of Artificial Neural Networks
for Autonomous Navigation. In {\it Neural Computation 3:1} pp. 88-97.
(#13) Touretzky, D.S., Pomerleau, D.A. (1989) What's hidden in the hidden
units? {\it BYTE 14(8)}, pp. 227-233.
(#14) Pomerleau, D.A. (1991) Rapidly Adapting Artificial Neural Networks for
Autonomous Navigation. In {\it Advances in Neural Information Processing
Systems 3}, R.P. Lippmann, J.E. Moody, and D.S. Touretzky (ed.), Morgan
Kaufmann, pp. 429-435.
(#15) Pomerleau, D.A. (1989) ALVINN: An Autonomous Land Vehicle In a Neural
Network. In {\it Advances in Neural Information Processing Systems 1},
D.S. Touretzky (ed.), Morgan Kaufmann, pp. 305-313.
------------------------------
End of Neuron Digest [Volume 10 Issue 20]
*****************************************