Copy Link
Add to Bookmark
Report
Neuron Digest Volume 09 Number 39
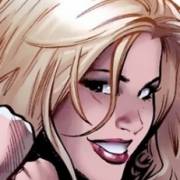
Neuron Digest Thursday, 23 Jul 1992 Volume 9 : Issue 39
Today's Topics:
Administrivia - ND on vacation for over 3 weeks
NIPS 92 advance program?
research post in cortical neural networks
NEURAL NETWORK WORKSHOP
Call for Papers, Applications of AI (XI)
IJCNN 92 Beijing Call For Papers *Extension*
TR - Determining the Number of Hidden Units
Send submissions, questions, address maintenance, and requests for old
issues to "neuron-request@cattell.psych.upenn.edu". The ftp archives are
available from cattell.psych.upenn.edu (128.91.2.173). Back issues
requested by mail will eventually be sent, but may take a while.
----------------------------------------------------------------------
Subject: Administrivia - ND on vacation for over 3 weeks
From: "N-D Moderator, Peter Marvit" <neuron@cattell.psych.upenn.edu>
Date: Thu, 23 Jul 92 22:21:08 -0500
Greetings,
As promised, the Neuron Digest Moderator will be goig on vacation for
about three weeks starting immediately. As a result, the Digest will
suspend publication with this issue, to resume sometime during the week
of 17 August. Obviously, no email/correspondence will be answered during
this time.
This issue is a mix of items which seem somewhat time-sensitive.
Observant readers will note a lack of paper announcements recently.
There are some 80 papers in the queue, but they will have to wait until
after break at which time I will try to send out the backlog.
As always, thanks to you, faithful readers, for making this Digest
possible and spreading information about Artifical Neural Networks aroudn
the globe.
-Peter Marvit
N-D Moderator
------------------------------
Subject: NIPS 92 advance program?
From: Juan Carlos Guzman <guzman-juan@CS.YALE.EDU>
Date: Fri, 17 Jul 92 14:18:37 -0500
Hi,
I would like to know where I can find an advance schedule for NIPS'92
(Neural Information Processing Systems), to be held in Colorado,Nov 30--
Dec 4, this year. Any leads would be greatly appreciated.
Juan Carlos Guzman.
[[ Editor's Note: I expect we'll see a reasonable version in this Digest
in September. If someone has earlier information... -PM ]]
------------------------------
Subject: research post in cortical neural networks
From: Peter Foldiak <peter@psy.ox.ac.uk>
Date: Tue, 21 Jul 92 12:15:54 +0000
UNIVERSITY OF OXFORD
DEPARTMENT OF EXPERIMENTAL PSYCHOLOGY
RESEARCH POST IN CORTICAL NEURAL NETWORKS
Applications are invited for a postdoctoral or graduate position to work
on the operation of neuronal networks in the brain, with special
reference to cortical computation. The post available is for a
theoretician to perform analytic and/or simulation work collaboratively
with experimental neuroscientists on biologically realistic models of
computation in cortical structures such as the hippocampus and visual
cortex. The salary is on the RS1A scale, #12,129-#16,432, and is funded
by a three-year grant which provides for international collaboration.
Applications including the names of two referees, or enquiries, to Dr.
Edmund T. Rolls, University of Oxford, Department of Experimental
Psychology, South Parks Road, Oxford OX1 3UD, England (telephone
0865-271348, e-mail: erolls@vax.ox.ac.uk). The University is an Equal
Opportunities Employer.
Peter Foldiak
------------------------------
Subject: NEURAL NETWORK WORKSHOP
From: anshu@lexington.rutgers.edu
Date: Fri, 17 Jul 92 16:32:40 -0500
NEURAL NETWORK WORKSHOP
27-29 October, 1992
held at
Ramada Renaissance Hotel, East Brunswick, NJ.
sponsored by
The Aviation Security Research and Development Service of
The Federal Aviation Administration (FAA)
and
CAIP Center, Rutgers University, NJ
Leaders in the field from academia, industry and government will
present the state-of-the-art in Neural Network theory and Applications.
There will be focussed sessions and panels on Neural Networks for vision,
speech recognition, speaker identification, language acquisition,
hardware implementations and security systems. Special emphasis will
be given on the future impact of Neural Networks on Aviation Technology.
The workshop will begin with registration at 8:30 a.m. on Tuesday, 27
October and end at 4:30 p.m. on Thursday. The $395 registration fee
($295 for participants from CAIP member organizations), includes the
cost of dinners and banquet. Proceedings of the workshop will be
published in book form.
Registration is limited to 90 participants, including 30 invited
speakers and panelists. Individuals wishing to attend the workshop
should register by September 27, 1992.
For further information regarding registration, please contact
Ms. Sandra Epstein
email: sepstein@caip.rutgers.edu
Telephone: (908)932-4208
Fax: (908)932-4775
Telex: 65-2497820 mci
- ------------------------------------------------------------------------------
NEURAL NETWORK WORKSHOP
27-29 October, 1992
|--------------------------------------------------------------------|
| WORKSHOP REGISTRATION FORM |
| |
| YES! I want to attend the Neural Network Workshop, October 27-29, |
| 1992. I understand my registration fee includes all sessions, |
| dinners, refreshment breaks, reception and working materials. |
| |
| Name ___________________________________________________________ |
| |
| Company ________________________________________________________ |
| |
| Address ________________________________________________________ |
| |
| City/State/Zip _________________________________________________ |
| |
| Telephone No. __________________________________________________ |
| |
|--------------------------------------------------------------------|
REGISTRATION IS LIMITED! APPLICATIONS WILL ONLY BE CONSIDERED WHEN
ACCOMPANIED WITH PAYMENT. MAKE CHECKS PAYABLE TO THE CAIP CENTER,
RUTGERS UNIVERSITY.
Registration: Non-member fee ($395) $____________
Member fee for participants from
CAIP member organizations ($295) $____________
EARLY REGISTRATION IS ADVISED! Mail form & payment to: CAIP Center,
Rutgers Univ, 7th floor, CoRE Blgd., PO Box-1390, Piscataway,NJ-08855.
...........................................................................
|--------------------------------------------------------------------|
| HOTEL REGISTRATION FORM |
| |
| Name ___________________________________________________________ |
| |
| Company ________________________________________________________ |
| |
| Address ________________________________________________________ |
| |
| Daytime Phone No. ______________________________________________ |
| |
| A block of rooms for this conference has been reserved at a special|
| University room rate of $81 per single/double room per night. |
| Hotel Reservations will be made through the CAIP Center. |
| ------------------------------------------------------- |
| I will require room(s): |
| Monday, October 26 ( ) |
| Tuesday, October 27 ( ) |
| Wednesday, October 28( ) |
| Thursday, October 29 ( ) |
|--------------------------------------------------------------------|
------------------------------
Subject: Call for Papers, Applications of AI (XI)
From: fayyad@ai-cyclops.Jpl.Nasa.Gov (Usama Fayyad)
Date: Tue, 21 Jul 92 18:11:39 -0800
______________________________________________________________________________
CALL FOR PAPERS 9/14/92 -- CALL FOR PAPERS 9/14/92 -- CALL FOR PAPERS 9/14/92
______________________________________________________________________________
APPLICATIONS OF AI (XI): Knowledge-Based Systems in Aerospace & Industry
------------------------------------------------------------------------
April 12-14, 1993
Marriott's Orlando World Center
Resort and Convention Center
Orlando, Florida, U.S.A.
Sponsored by: SPIE -- The Society for Optical Engineering
In cooperation with: AAAI -- The American Association for Artificial
Intelligence
AIAA -- The American Institute of Aeronautics
and Astronautics
IEEE Computer Society
IEEE Systems, Man, and Cybernetics Society
The Eleventh Applications of Artificial Intelligence Conference will be
help April 12-14 in Orlando, FL. We invite you to submit a paper by the
deadline of Sept. 14, 1992. Details of areas and deadlines given below.
Conference Co-Chairs:
Usama M. Fayyad Ramasamy Uthurusamy
Jet Propulsion Lab General Motors Research Laboratories
California Institute of Technology
Program Committee:
Ray Bareiss, Northwestern University
Steven Lytinen, The University of Michigan
James Bezdek, University of West Florida
Stephen C.Y. Lu, University of Illinois
Gautam Biswas, Vanderbilt University
Ray Mooney, University of Texas at Austin
Wray Buntine, NASA Ames Research Center
Gregory Piatetsky-Shapiro, GTE Laboratories
Steve Chien, Jet Propulsion Lab
J. Ross Quinlan, Univ. of Sydney, Australia
Tharam Dillon, La Trobe Univ., Australia
Ethan Scarl, Boeing Computer Services
Richard Doyle, Jet Propulsion Lab
Jude Shavlik, University of Wisconsin, Madison
Doug Fisher, Vanderbilt University
Prakash Shenoy, University of Kansas
Paul Fishwick, University of Florida
N.S. Sridharan, Intel Corporation
David Franke, MCC
Evangelos Simoudis, Lockheed Aerospace
Ashok Goel, Georgia Tech.
Stephen Smith, Carnegie Mellon University
Larry Hall, University of South Florida
Jon Sticklen, Michigan State University
Yumi Iwasaki, Stanford University
R. Zurawsky, Swinburne Inst. of Tech., Australia
Ramesh Jain, The University of Michigan
This year we will focus on techniques and applications that deal with
actual industrial and aerospace applications of AI, machine learning, and
reasoning systems.
Topics of interest include but are not limited to:
1. Machine Learning
2. Industrial and Aerospace Applications
3. Diagnostic Systems
4. Knowledge Acquisition and Refinement
5. Knowledge Based Systems: Verification and Validation
6. Manufacturing Systems
7. Case-Based Reasoning
8. Functional Reasoning
9. Model-Based and Qualitative Reasoning
10. Multilevel and Integrated Reasoning Systems
11. Planning and Scheduling
12. Design
13. Training and Tutoring Systems
14. Intelligent Interfaces and Natural Language Processing
15. Intelligent Database Systems
16. Parallel Architectures
In addition there will be 2-3 plenary sessions, and one or more panel
discussions. We also solicit suggestions for special sessions (e.g.,
Case-Based Tutoring, Reactive Planning in Space Missions). A one-page
description of such a suggestion should be sent to the Conference Chairs,
who will then forward it to appropriate members of the Program Committee
for evaluation. Selection will be based on how well the topic relates to
the general theme of the conference, and the level of interest it is
likely to generate.
To submit a paper, send four copies of a complete paper not exceeding 10
pages single-spaced (approx. 5000 words) including figures and
bibliography by September 14, 1992 to:
Applications of AI XI: KBS
SPIE, P.O. Box 10
1000 20th Street
Bellingham, WA 98225.
Tele: (206)-676-3290; Telefax: (206)-647-1445.
Submissions will be reviewed by at least two members of the program
committee and reviews will be returned to the authors. It is important
that each paper clearly state the problem which is being addressed, the
contribution that has been made, and the relation to the current state of
the art.
The program committee and conference chairs will make a selection of the
best papers accepted, and these authors will be invited to submit a
revised version of their paper to one or more special issues of journals
in AI (to be decided later).
Papers submitted to the Knowledge-Based Systems conference should not
also be submitted to the Machine Vision and Robotics conference of
Applications of AI XI. Questions about which conference is most suitable
for a particular paper should be directed to the program chairmen.
Each presenter is generally allowed 20 to 25 minutes for presentation,
plus a brief discussion period (about 5 minutes). SPIE will provide the
following media equipment free of charge: 35 mm carousel slide
projectors, overhead projectors, electronic pointers and VHS format video
display.
Author Benefits
Authors and coauthors who attend the conference will be accorded a
reduced-rate registration fee, a complimentary one-year non-voting
membership in SPIE (if never before a member), and other special benefits.
IMPORTANT DATES: PAPERS DUE: September 14, 1992.
ACCEPT/REJECT LETTERS SENT BY: November 20, 1992
CAMERA-READY PAPERS (5000 words) DUE: January 18, 1993.
CONFERENCE DATES: April 12-16, 1993.
Further questions may be directed to (e-mail preferred):
Dr. Usama Fayyad Dr. Ramasamy Uthurusamy
AI Group M/S 525-3660 Computer Science Department
Jet Propulsion Lab General Motors Research Labs
California Institute of Technology 30500 Mound Rd.
Pasadena, CA 91109 Warren, MI 48090-9055
phone: (818) 306-6197 phone: (313) 986-1989
fax: (818)-306-6912. fax: (313) 986-9356
e-mail: Fayyad@aig.jpl.nasa.gov e-mail: Samy@gmr.com
______________________________________________________________________________
CALL FOR PAPERS 9/14/92 -- CALL FOR PAPERS 9/14/92 -- CALL FOR PAPERS 9/14/92
______________________________________________________________________________
------------------------------
Subject: IJCNN 92 Beijing Call For Papers *Extension*
From: Wesley R Elsberry <elsberry@cse.uta.edu>
Date: Tue, 21 Jul 92 15:09:37 -0600
IJCNN 92 BEIJING, CHINA
November 3-6, 1992
CALL FOR PAPERS
--- Extension ---
Papers for the upcoming IJCNN 92 Beijing conference will continue to be
accepted through July 31, 1992. Papers should be submitted in standard
IJCNN format (maximum of six pages, etc.) to:
Dr. Harold Szu
9402 Wildoak Drive
Bethesda, MD 20814
Dr. Szu indicates that because of the limited technical communication
between the rest of the international ANN research community and the
People's Republic of China, papers submitted may be original work *or*
review articles.
Researchers are encouraged to contribute on any topic related to
artificial neural networks.
Wesley R. Elsberry
Sysop, CNS BBS 509-627-6267; Moderator Int'l NEURAL_NET Echo;
elsberry@cse.uta.edu; POB 1187, Richland, WA 99352; 509-627-3947 voice
------------------------------
Subject: TR - Determining the Number of Hidden Units
From: Shun-ichi Amari <amari@sat.t.u-tokyo.ac.jp>
Date: Fri, 17 Jul 92 11:16:33 +0200
The following technical report has been placed in the Neuroprose Archives
at Ohio State University:
Network Information Criterion ---
Determining the Number of Hidden Units
for an Artificial Neural Network Model
Noboru Murata, Shuji Yoshizawa and Shun-ichi Amari
Technical Report METR 92-05, June 1992
Department of Mathematical Engineering and Information Physics
University of Tokyo
Hongo 7-3-1, Bunkyo-ku, Tokyo 113, Japan
SUMMARY: (We recommend typesetting this region by Latex.)
\documentstyle{article}
\title{Model selection and effective number of parameters}
\author{Noboru MURATA \and Shuji YOSHIZAWA \and Shun-ichi AMARI}
\begin{document}
\maketitle
It is an important problem of the neural computation to select an
adequate model for a given set of training data. This problem
naturally leads us a generalization of the AIC criterion of the model
selection of statistical models (see Murata et al. [1991], Moody
[1992]). The present note points out a natural generalization of the
AIC criterion, showing that the results of the above two papers are
equivalent and that they are applicable to more general situations.
Let us consider a stochastic neural network, parameterized by a set of
$m$ weights $\theta=(\theta^1,\cdots,\theta^m)$, whose input-output
behavior is specified by a conditional probability $p(y|x,\theta)$,
namely the probability distribution of output $y$ when the input
signal is $x$ (the signal $x$ might be multi dimensional). Let
$q(y|x)$ be the true probability from which $n$ examples
$(y_i,x_i)$,$(i=1,\cdots,n)$ are generated, where $x_i$ is generated
from an unknown probability distribution $q(x)$ independently. We
define a sample $\Xi_t=\{(y_i,x_i); i=1,\cdots,t\}$ as a set of $t$
examples. The main problem is to find the best candidate $\theta^*$
which approximates the conditional distribution $q(y|x)$ and to
evaluate the model ability, where there might not exist $\hat\theta$
such that $p(y|x,\hat\theta)=q(y|x)$.
We use a test criterion
\begin{displaymath}
D(q,p(\theta))=K[q:p(\theta)]+S(\theta)
\end{displaymath}
to be minimized, where $K[q:p(w)]$ represents a general divergence
measure between two conditional probabilities $q$ and $p(\theta)$, and
$S(\theta)$ is a regularization term which restricts the parameter
$\theta$ within an appropriate area (Moody [1992]). We give two
typical case of $K$. One is the Kullback-Leibler divergence
\begin{displaymath}
K[q:p(\theta)]=\int q(x)q(y|x)\log{\frac{q(y|x)}{p(y|x,\theta)}}dydx,
\end{displaymath}
and the other is the squared error
\begin{displaymath}
K[q:p(\theta)]=\int\|y-f(x,\theta)\|^2q(x)q(y|x)dydx,
\end{displaymath}
where
\begin{displaymath}
f(x,\theta)=\int y\ p(y|x,\theta)dy.
\end{displaymath}
Any divergence measure of the type
\begin{displaymath}
K[q:p(\theta)]=\int q(x)q(y|x)k(x,y;\theta)dydx
\end{displaymath}
works well.
Given the sample $\Xi_t$, we search for the best parameter $\theta^*$
that minimize
\begin{displaymath}
D(q^*,p(\theta))=K[q^*:p(\theta)]+S(\theta),
\end{displaymath}
where $q^*$ is the empirical distribution given by the sample $\Xi_t$,
that is,
\begin{displaymath}
K[q^*:p(\theta)]=\frac{1}{t}\sum_{i=1}^{t} k(x_i,y_i;\theta).
\end{displaymath}
This is because the true distributions $q(x)$ and $q(y|x)$ are
unknown, so we substitute the empirical distribution for the true
joint distribution $q(x)q(y|x)$. The minimized $D(q^*,p(\theta^*))$ is
called the training loss or the training error, and it can be used for
estimating the test loss or the generalization error $D(q,p(\theta^*))$,
which shows the averaged behavior of the trained network when a new
example is given.
Since these quantities depend on the sample $\Xi_t$ whose elements are
chosen randomly, we take the expectation $\langle\cdot\rangle$ with respect to
the sample $\Xi_t$. We can the prove the following relation
\begin{displaymath}
\langle D(q,p(\theta^*))\rangle=\langle D(q^*,p(\theta^*))\rangle
+\frac{1}{t}\mbox{\rm tr}GQ^{-1}+\frac{1}{\sqrt{t}}U,
\end{displaymath}
where $U$ is an unbiased random value of order $1$, common to all the
models which have the same architecture, $G$ is the covariance matrix
of $\nabla\{k(x,y;\theta^*)+S(\theta^*)\}$ and $Q$ is the Hessian
matrix of $D(q,p(\theta^*)$. These quantities are to be evaluated by
using the empirical distribution $q^*$ instead of the true but unknown
$q(x)$ and $q(y|x)$. When we compare the abilities of two different
models and chose better model, we can use a quantity
\begin{displaymath}
D(q^*,p(\theta^*))+\frac{1}{t}\mbox{\rm tr}GQ^{-1},
\end{displaymath}
and select one model whose value of the quantity is smaller than the
other's.
Now we can compare this result with others. If the probability
distribution is unconditional, $K$ is the Kullback-Leibler divergence,
$D$ includes no regularization term (S(w)=0), and there exist $\hat\theta$
such that $q=p(\hat\theta)$, then $Q$ and $G$ coincide with the Fisher
information matrix and
\begin{displaymath}
m^*=\mbox{\rm tr}GQ^{-1}=m,
\end{displaymath}
holds, where $m$ is the dimension number of the parameter $\theta$.
This is the classic AIC result. When no such $\hat\theta$ exists, $G$
and $Q$ never coincide with the Fisher information matrix (Takeuchi
[1980]), and an effective dimension $m^*$ is different from $m$. When
$q$ and the optimal $p$ is close, Amari [1980] calculated $m^*$ by
using an ancillary statistics and the $m$-curvature of the model
surface.
A sketch of the proof of the present result was given in Murata et al.
[1991], where the regularization term is not taken into account but
the proof remains valid. Moody [1992] defined the effective dimension
by
\begin{displaymath}
m^*=\mbox{\rm tr}(TQ^{-1}T^T),
\end{displaymath}
where
\begin{displaymath}
G=T^TT
\end{displaymath}
holds, so that it coincides with the above result. It should be noted
that the above result holds without assuming the linearity of the
model, existence of $\hat\theta$, the additive noise $\xi$ of the form
of
\begin{displaymath}
z=f(x,w)+\xi,
\end{displaymath}
etc. Therefore, the result of Moody holds under another framework and
the AIC criterion can be applied in a general non-linear model.
On the other hand, it should be emphasized that this generalized criterion
cannot be used when we compare two models of different architectures,
say a neural network model and a radial basis expansion model.
This is because the quantity $U$ of the order $1/\sqrt{t}$ term is common
only for two models in which one model is included in the other as a submodel.
The AIC criterion is valid only for such a family of models of submodels.
This important remark should more clearly be recognized,
since it is not clearly written in the original AIC theory.
\end{document}
END OF SUMMARY
***** HOW TO OBTAIN A COPY *****
a) Via FTP:
unix> ftp archive.cis.ohio-state.edu (or 128.146.8.52)
Name: anonymous
Password: (type your E-mail address)
ftp> cd pub/neuroprose
ftp> binary
ftp> get murata.nic.ps.Z
ftp> quit
unix> uncompress murata.nic.ps.Z
unix> lpr murata.nic.ps
b) Via postal mail:
Request
Noboru Murata,
Department of Mathematical Engineering and Information Physics
University of Tokyo
Hongo 7-3-1, Bunkyo-ku, Tokyo 113
Japan.
or E-mail: mura@sat.t.u-tokyo.ac.jp
------------------------------
End of Neuron Digest [Volume 9 Issue 39]
****************************************