Copy Link
Add to Bookmark
Report
Neuron Digest Volume 09 Number 27
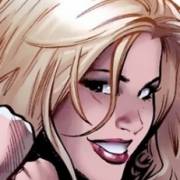
Neuron Digest Tuesday, 16 Jun 1992 Volume 9 : Issue 27
Today's Topics:
ISSNNet Nominations
Position Announcement: Arris Pharmaceutical
NetGene
TDNN network configuration file(s) for PlaNet
Send submissions, questions, address maintenance, and requests for old
issues to "neuron-request@cattell.psych.upenn.edu". The ftp archives are
available from cattell.psych.upenn.edu (128.91.2.173). Back issues
requested by mail will eventually be sent, but may take a while.
----------------------------------------------------------------------
Subject: ISSNNet Nominations
From: worth@cns.bu.edu (Andrew J. Worth)
Organization: The International Student Society for Neural Netwoks
Date: 03 Jun 92 18:28:30 +0000
______________________________________________________________________
ISSNNet Official Call for Nominations
______________________________________________________________________
The International Student Society for Neural Netwoks (ISSNNet) is in the
process of re-organizing. The founders are no longer students and it is
time to create a new administration. The first step in this process is
to hold elections as per the existing bylaws. The current officers are
preparing reports on what has been accomplished so far and are laying the
groundwork for the new organization. The newly elected officers should
be willing to take the task of finalizing this re-organization.
Official nomination period: June 1st through 30th. Nominations will be
accepted by email, surface mail, or in person at IJCNN92 (look for
ISSNNet signs in the exhibition hall). As per the bylaws, nominations
will be approved by the Governing board and the current Officers. At
least two and no more than four nominees will be placed on the ballot for
each Officer position. Selection will be based on the number of member
nominations.
Election ballots will be mailed (either by surface mail or electronic
mail) on August 1st, 1992 and voting shall be closed on August 31st,
1992. Election to Officer positions will be based on plurality of votes
among the selected nominees.
All four officer positions are up for election:
Position: Duties:
============== =================================================
President Chief execute officer and Spokesperson. The
President is responsible for making sure that the
society continues to function as described in the
Bylaws.
Vice President Assist the President.
Director Oversees practical organizational matters.
Responsible for elections.
Treasurer Responsible for all monies.
Qualifications for potential nominees: The nominee must be enrolled at a
recognized academic institution (proof of student status will be
required) AND HAVE RELIABLE ACCESS TO ELECTRONIC MAIL. Each nomination
must be supported by at least 10 student members. No more than two
Officers may belong to the same Area of Jurisdiction (Country, State,
Province, Region, etc. with at least five student members). Moreover, the
President and Vice President may not belong to the same Area of
Jurisdiction.
Because ISSNNet membership processing has been suspended for some time,
anyone who has been a member of ISSNNet in the past can submit or support
a nomination. This includes students outside the USA who were unable to
submit dues because of exchange problems, but who have been on our
mailing list in the past, directly through ISSNNet or through one of the
Governors.
Fill out the information below, and return the following form to the
address shown (e-mail or surface).
---------------------------- cut here ------------------------------
ISSNNet NOMINATION FORM
Please include as much information about the nominee as possible. Add
lines where necessary. If using surface mail, please type.
NOMINEE INFORMATION:
Position: [President, Vice President, Director, Treasurer]
Name (Last,First): ______________________________________
University: ______________________________________
Surface
Address: ______________________________________
______________________________________
______________________________________
______________________________________
Email: ______________________________________ (please type!)
Phone: ______________________________________
SUPPORTING MEMBERS:
Name and University: _______________________________________________
Name and University: _______________________________________________
Name and University: _______________________________________________
Name and University: _______________________________________________
Name and University: _______________________________________________
Name and University: _______________________________________________
Name and University: _______________________________________________
Name and University: _______________________________________________
Name and University: _______________________________________________
Name and University: _______________________________________________
----------------------------- cut here -------------------------------
Return your nomination with the above information to:
issnnet@cns.bu.edu
or to
ISSNNet Elections
P.O. Box 15661
Boston, MA 02215 USA
Thank you for your support!
Andy.
----------------------------------------------------------------------
Andrew J. Worth (617) 353-6741 ISSNNet, Inc.
ISSNNet Acting Director P.O. Box 15661
worth@cns.bu.edu Boston, MA 02215 USA
----------------------------------------------------------------------
------------------------------
Subject: Position Announcement: Arris Pharmaceutical
From: Tom Dietterich <tgd@arris.com>
Date: Wed, 03 Jun 92 14:03:26 -0800
RESEARCH SCIENTIST in
Machine Learning, Neural Networks, and Statistics
Arris Pharmaceutical
Arris Pharmaceutical is a start-up pharmaceutical company founded in 1989
and dedicated to the efficient discovery and development of novel,
orally-active human therapeutics through the application of artificial
intelligence, machine learning, and pattern recognition methods.
We are seeking a person with a PhD in Computer Science, Mathematics,
Statistics, or related fields to join our team developing new machine
learning algorithms for drug discovery. The team currently includes
contributions from Tomas Lozano-Perez, Rick Lathrop, Roger Critchlow, and
Tom Dietterich. The ideal candidate will have a strong background in
mathematics (including spatial reasoning methods) and five years'
experience in machine learning, neural networks, or statistical
model-building methods. The candidate should be eager to learn the
relevant parts of computational chemistry and to interact with medicinal
chemists and molecular biologists.
To a first approximation, the Arris drug design strategy begins by
identifying a pharmaceutical target (e.g., an enzyme or a cell-surface
receptor), developing assays to measure chemical binding with this
target, and screening large libraries of peptides (short amino acid
sequences) with these assays. The resulting data, which indicates for
each compound, how well it binds to the target, will then be analyzed by
machine learning algorithms to develop hypotheses that explain why some
compounds bind well to the target while others do not. Information from
X-ray crystallography or NMR spectroscopy may also be available to the
learning algorithms. Hypotheses will then be refined by synthesizing and
testing additional peptides. Finally, medicinal chemists will synthesize
small organic molecules that satisfy the hypothesis, and these will
become candidate drugs to be tested for medical safety and effectiveness.
For more information, send your resume with the names and addresses of
three references to Tom Dietterich (email: tgd@arris.com; voice:
415-737-8600; FAX: 415-737-8590).
Arris Pharmaceutical Corporation
385 Oyster Point Boulevard, Suite 12
South San Francisco, CA 94080
------------------------------
Subject: NetGene
From: BRUNAK@nbivax.nbi.dk
Date: Sun, 17 May 92 13:31:00 +0100
******** Announcement of the NetGene Mail-server: *********
DESCRIPTION:
The NetGene mail server is a service producing neural network
predictions of splice sites in vertebrate genes as described in:
Brunak, S., Engelbrecht, J., and Knudsen, S. (1991) Prediction of
Human mRNA Donor and Acceptor Sites from the DNA Sequence. Journal
of Molecular Biology, 220, 49-65.
ABSTRACT OF JMB ARTICLE:
Artificial neural networks have been applied to the prediction of
splice site location in human pre-mRNA. A joint prediction scheme
where prediction of transition regions between introns and exons
regulates a cutoff level for splice site assignment was able to
predict splice site locations with confidence levels far better than
previously reported in the literature. The problem of predicting
donor and acceptor sites in human genes is hampered by the presence
of numerous amounts of false positives - in the paper the
distribution of these false splice sites is examined and linked to a
possible scenario for the splicing mechanism in vivo. When the
presented method detects 95% of the true donor and acceptor sites it
makes less than 0.1% false donor site assignments and less than 0.4%
false acceptor site assignments. For the large data set used in this
study this means that on the average there are one and a half false
donor sites per true donor site and six false acceptor sites per true
acceptor site. With the joint assignment method more than a fifth of
the true donor sites and around one fourth of the true acceptor sites
could be detected without accompaniment of any false positive
predictions. Highly confident splice sites could not be isolated
with a widely used weight matrix method or by separate splice site
networks. A complementary relation between the confidence levels of
the coding/non-coding and the separate splice site networks was
observed, with many weak splice sites having sharp transitions in the
coding/non-coding signal and many stronger splice sites having more
ill-defined transitions between coding and non-coding.
INSTRUCTIONS:
In order to use the NetGene mail-server:
1) Prepare a file with the sequence in a format similar to the fasta
format: the first line must start with the symbol '>', the next
word on that line is used as the sequence identifier. The
following lines should contain the actual sequence, consisting of
the symbols A, T, U, G, C and N. U is converted to T, letters not
mentioned are converted to N. All letters are converted to upper
case. Numbers, blanks and other nonletter symbols are skipped.
The lines should not be longer than 80 characters. The minimum
length analyzed is 451 nucleotides, and the maximum is 100000
nucleotides (your mail system may have a lower limit for the
maximum size of a message). Due to the non-local nature of the
algorithm sites closer than 225 nucleotides to the ends of the
sequence will not be assigned.
2) Mail the file to netgene@virus.fki.dth.dk. The response time will
depend on system load. If nothing else is running on the machine
the speed is about 1000 nucleotides/min. It may take several
hours before you get the answer, so please do not resubmit a job
if you get no answer within a short while.
REFERENCING AND FURTHER INFORMATION
Publication of output from NetGene must be referenced as follows:
Brunak, S., Engelbrecht, J., and Knudsen, S. (1991) Prediction of
Human mRNA Donor and Acceptor Sites from the DNA Sequence. Journal
of Molecular Biology, 220, 49-65.
CONFIDENTIALITY
Your submitted sequence will be deleted automatically immediately
after processing by NetGene.
PROBLEMS AND SUGGESTIONS:
Should be addressed to:
Jacob Engelbrecht
e-mail: engel@virus.fki.dth.dk
Department of Physical Chemistry
The Technical University of Denmark
Building 206
DK-2800 Lyngby
Denmark
phone: +45 4288 2222 ext. 2478 (operator)
phone: +45 4593 1222 ext. 2478 (tone)
fax: +45 4288 0977
EXAMPLE:
A file test.seq is prepared with an editor with the following contents:
>HUMOPS
GGATCCTGAGTACCTCTCCTCCCTGACCTCAGGCTTCCTCCTAGTGTCACCTTGGCCCCTCTTAGAAGC
CAATTAGGCCCTCAGTTTCTGCAGCGGGGATTAATATGATTATGAACACCCCCAATCTCCCAGATGCTG
. Here come more lines with sequence.
.
.
This is sent to the NetGene mail-server, on a Unix system like this:
mail netgene@virus.fki.dth.dk < test.seq
In return an answer similar to this is produced:
>From netgene@virus.fki.dth.dk Fri Mar 20 13:30 MET 1992
Received: by virus.fki.dth.dk
(16.7/16.2) id AA05624; Fri, 20 Mar 92 13:30:41 +0100
Date: Fri, 20 Mar 92 13:30:41 +0100
From: virus mail server <netgene@virus.fki.dth.dk>
Return-Path: <netgene@virus.fki.dth.dk>
To: engel@virus.fki.dth.dk
Subject: HUMOPS: NetGene splice site prediction
Status: RO
- ------------------------------------------------------------------------
NetGene
Neural Network Prediction of Splice Sites
Reference:
Brunak, S., Engelbrecht, J., and Knudsen, S. (1991). Prediction of
Human mRNA donor and acceptor sites from the DNA sequence. Journal of
Molecular Biology 220:49-65.
- ------------------------------------------------------------------------
Report ERRORS to Jacob Engelbrecht engel@virus.fki.dth.dk.
Potential splice sites are assigned by combining output from a local and
a global network. The prediction is made with two cutoffs: 1) Highly
confident sites (no or few false positives, on average 50% of the true
sites detected); 2) Nearly all true sites (more false positives - on
average of all positions 0.1% false positive donor sites and 0.4% false
positive acceptor sites, at 95% detection of true sites). The network
performance on sequences from distantly related organisms has not been
quantified. Due to the non-local nature of the algorithm sites closer
than 225 nucleotides to the ends of the sequence cannot be assigned.
Column explanations, field identifiers:
POSITION in your sequence (either first or last base in intron).
Joint CONFIDENCE level for the site (relative to the cutoff).
EXON INTRON gives 20 bases of sequence around the predicted site.
LOCAL is the site confidence from the local network.
GLOBAL is the site confidence from the global network.
- ------------------------------------------------------------------------
The sequence: HUMOPS contains 6953 bases, and has the following composition:
A 1524 C 2022 G 1796 T 1611
1) HIGHLY CONFIDENT SITES:
==========================
ACCEPTOR SITES:
POSITION CONFIDENCE INTRON EXON LOCAL GLOBAL
4094 0.27 TGTCCTGCAG^GCCGCTGCCC 0.63 0.66
5167 0.20 TGCCTTCCAG^TTCCGGAACT 0.59 0.64
3812 0.17 CTGTCCTCAG^GTACATCCCC 0.68 0.54
3164 0.02 TCCTCCTCAG^TCTTGCTAGG 0.79 0.32
2438 0.01 TGCCTTGCAG^GTGAAATTGC 0.78 0.33
DONOR SITES:
POSITION CONFIDENCE EXON INTRON LOCAL GLOBAL
3979 0.38 CGTCAAGGAG^GTACGGGCCG 0.92 0.74
2608 0.17 GCTGGTCCAG^GTAATGGCAC 0.85 0.54
4335 0.06 GAACAAGCAG^GTGCCTACTG 0.83 0.41
2) NEARLY ALL TRUE SITES:
=========================
ACCEPTOR SITES:
POSITION CONFIDENCE INTRON EXON LOCAL GLOBAL
4094 0.55 TGTCCTGCAG^GCCGCTGCCC 0.63 0.66
3812 0.52 CTGTCCTCAG^GTACATCCCC 0.68 0.54
3164 0.49 TCCTCCTCAG^TCTTGCTAGG 0.79 0.32
5167 0.49 TGCCTTCCAG^TTCCGGAACT 0.59 0.64
2438 0.48 TGCCTTGCAG^GTGAAATTGC 0.78 0.33
4858 0.39 TCATCCATAG^AAAGGTAGAA 0.77 0.20
3712 0.36 CCTTTTCCAG^GGAGGGAATG 0.88 -0.01
4563 0.33 CCCTCCACAG^GTGGCTCAGA 0.81 0.05
5421 0.33 TTTTTTTAAG^AAATAATTAA 0.75 0.13
3783 0.29 TCCCTCACAG^GCAGGGTCTC 0.64 0.26
3173 0.25 GTCTTGCTAG^GGTCCATTTC 0.52 0.36
4058 0.24 CTCCCTGGAG^GAGCCATGGT 0.43 0.51
1784 0.22 TCACTGTTAG^GAATGTCCCA 0.68 0.08
6512 0.21 CCCTTGCCAG^ACAAGCCCAT 0.67 0.08
2376 0.20 CCCTGTCTAG^GGGGGAGTGC 0.61 0.16
1225 0.18 CCCCTCTCAG^CCCCTGTCCT 0.65 0.07
1743 0.13 TTCTCTGCAG^GGTCAGTCCC 0.62 0.03
3834 0.13 GGGCCTGCAG^TGCTCGTGTG 0.26 0.58
4109 0.13 TGCCCAGCAG^CAGGAGTCAG 0.29 0.54
6557 0.13 CATTCTGGAG^AATCTGCTCC 0.56 0.12
1638 0.11 CCATTCTCAG^GGAATCTCTG 0.62 0.00
247 0.10 GCCTTCGCAG^CATTCTTGGG 0.55 0.11
6766 0.09 CTATCCACAG^GATAGATTGA 0.64 -0.06
906 0.08 AATTTCACAG^CAAGAAAACT 0.61 -0.02
6499 0.08 CAGTTTCCAG^TTTCCCTTGC 0.55 0.06
378 0.07 GTACCCACAG^TACTACCTGG 0.24 0.52
3130 0.07 CTGTCTCCAG^AAAATTCCCA 0.51 0.12
4272 0.07 ACCATCCCAG^CGTTCTTTGC 0.58 0.00
4522 0.07 TGAATCTCAG^GGTGGGCCCA 0.51 0.12
5722 0.07 ACCCTCGCAG^CAGCAGCAAC 0.55 0.05
2316 0.06 CTTCCCCAAG^GCCTCCTCAA 0.40 0.27
2357 0.06 GCCTTCCTAG^CTACCCTCTC 0.39 0.28
2908 0.06 TTTGGTCTAG^TACCCCGGGG 0.51 0.10
4112 0.06 CCAGCAGCAG^GAGTCAGCCA 0.25 0.50
1327 0.05 TTTGCTTTAG^AATAATGTCT 0.52 0.06
844 0.04 GTTTGTGCAG^GGCTGGCACT 0.62 -0.11
1045 0.04 TCCCTTGGAG^CAGCTGTGCT 0.54 0.01
1238 0.03 CTGTCCTCAG^GTGCCCCTCC 0.50 0.06
2976 0.03 CCTAGTGCAG^GTGGCCATAT 0.62 -0.12
3825 0.03 CATCCCCGAG^GGCCTGCAGT 0.16 0.60
1508 0.02 TGAGATGCAG^GAGGAGACGC 0.43 0.16
2257 0.02 CTCTCCTCAG^CGTGTGGTCC 0.53 0.00
5712 0.02 ATCCTCTCAG^ACCCTCGCAG 0.51 0.05
2397 0.00 CCCTCCTTAG^GCAGTGGGGT 0.41 0.16
4800 0.00 CATTTTCTAG^CTGTATGGCC 0.47 0.07
5016 0.00 TGCCTAGCAG^GTTCCCACCA 0.59 -0.11
DONOR SITES:
POSITION CONFIDENCE EXON INTRON LOCAL GLOBAL
3979 0.75 CGTCAAGGAG^GTACGGGCCG 0.92 0.74
2608 0.51 GCTGGTCCAG^GTAATGGCAC 0.85 0.54
4335 0.38 GAACAAGCAG^GTGCCTACTG 0.83 0.41
656 0.32 ACCCTGGGCG^GTATGAGCCG 0.56 0.66
5859 0.11 ACCAAAAGAG^GTGTGTGTGT 0.85 0.07
4585 0.09 GCTCACTCAG^GTGGGAGAAG 0.86 0.03
1708 0.06 TGGCCAGAAG^GTGGGTGTGC 0.85 0.01
6196 0.05 CCCAATGAGG^GTGAGATTGG 0.86 -0.01
667 0.03 TATGAGCCGG^GTGTGGGTGG 0.23 0.71
------------------------------
Subject: TDNN network configuration file(s) for PlaNet
From: Ben Bryant <bdbryan@eng.clemson.edu>
Date: Wed, 03 Jun 92 20:21:33 -0500
I recently sent a message concerning the above that was somehow garbled
in the transmission. I apologize for this. The file that was sent in the
last mailing was an ascii text file containing our current "best
estimate" of how the training of a TDNN takes place implemented as a
PlaNet network configuration file. If there is anyone there who has
experience with PlaNet and has written a correct TDNN network config file
for this package, I wonder if you might be kind enough to send us a copy.
If you cannot do this for non-disclosure reasons, could you please simply
look ove the following implementation and tell me whether we have
implemented the training procedure correctly. I would be much obliged.
The following is our "best guess" TDNN:
#### file for 3-layer TDNN network with input 40x15 N=2; hidden 20x13
#### N=4 ; Output 3x9
# DEFINITIONS OF DELAY
define NDin 3
define NDhid 5
define NDin_1 2
define NDhid_1 4
# DEFINITIONS OF UNITS
define NUin 40
define NUhid 20
define NUout 3
define NUin_1 39
define NUhid_1 19
define NUout_1 2
#DEFINITION OF INPUT FRAME
define NFin 15
define NFhid (NFin-NDin+1)
define NFout (NFin-NDin+2-NDhid)
define BiasHid 0
define BiasOut 0
## DEFINITIONS OF LAYERS
layer Input NFin*NUin
layer Hidden NUhid*NFhid
layer Output NFout*NUout
layer Result NUout
define biasd user1
## DEFINITIONS OF INPUT/TARGET BUFFERS
target NFout*NUout
input NFin*NUin
## DEFINITIONS OF CONNECTIONS
define Win (NUin*NDin_1+NUin_1)
define Whids 0
define Whid (NUhid_1)
connect InputHidden1 Input[0-Win] to Hidden[0-Whid]
define WHid (NUhid*NDhid_1+NUhid_1)
define Wout (NUout_1)
connect HiddenOutput1 Hidden[0-WHid] to Output[0-Wout]
## n.3layer.expr: implementation of a 3layer-feedforward-net with expressions.
## define Nin, Nhid, Nout, BiasHid and BiasOut as desired.
define ErrMsg \n\tread\swith\s'network\sNin=<no-of-input>\sNhid=<no-of-hidden>\sNout=<no-of-output>\sBiasHid=<bias-of-hidden>\sBiasO
ut=<bias-of-output>\sn.3layer.expr'\n
#IFNDEF NDin; printf ErrMsg; exit; ENDIF
#IFNDEF Nhid; printf ErrMsg; exit; ENDIF
#IFNDEF Nout; printf ErrMsg; exit; ENDIF
IFNDEF BiasHid; printf ErrMsg; exit; ENDIF
IFNDEF BiasOut; printf ErrMsg; exit; ENDIF
# macro definitions of the derivarives of the sigmoid for Hidden and Output
IF $min==0&&$max==1
define HiddenDer Hidden*(1-Hidden)
define OutputDer Output*(1-Output)
ELSE
define HiddenDer (Hidden-$min)*($max-Hidden)/($max-$min)
define OutputDer (Output-$min)*($max-Output)/($max-$min)
ENDIF
## PROCEDURE FOR ACTIVATING NETWORK FORWARD
procedure activate
scalar i
i=0
Input=$input
while i<NFhid
Hidden:net[i*NUhid->i*NUhid+NUhid_1]=InputHidden1 \
**T(Input[i*NUin->i*NUin+NUin*NDin_1+NUin_1])
i+=1
endwhile
Hidden = logistic(Hidden:net+(BiasHid*Hidden:bias))
i=0
while i<NFout
Output:net[i*NUout->i*NUout+NUout_1] = HiddenOutput1 \
**T(Hidden[i*NUhid->i*NUhid+NUhid*NDhid_1+NUhid_1])
i+=1
endwhile
Output=logistic(Output:net+(BiasOut*Output:bias))
$Error=mean((Output:delta=$target-Output)^2)/2
Output:delta*=OutputDer
end
## PROCEDURE FOR TRAINING NETWORK
matrix Hidden_delta NFout NDhid*NUhid
procedure learn
call activate
scalar i;scalar j
i=0
while i<NFout
Hidden_delta[i]=Output:delta[i*NUout->i*NUout+NUout_1] \
**HiddenOutput1*HiddenDer[i*NUhid->i*NUhid+NUhid*NDhid_1+NUhid_1]
i+=1
endwhile
Hidden:delta=0
i=0
while i<NFout
j=0
while j<NDhid
Hidden:delta[(i+j)*NUhid->(i+j)*NUhid+NUhid_1] \
+= Hidden_delta[i][j*NUhid->j*NUhid+NUhid_1]
j+=1
endwhile
i+=1
endwhile
i=0
while i<NFhid
if (i<NDhid ) then
Hidden:delta[i*NUhid->i*NUhid+NUhid_1]/=(i+1)
endif
if (NFhid-i<NDhid) then
Hidden:delta[i*NUhid->i*NUhid+NUhid_1]/=(NFhid-i)
endif
if ((NFhid-i>=NDhid) && (i>=NDhid)) then
Hidden:delta[i*NUhid->i*NUhid+NUhid_1]/=(NDhid)
endif
i+=1
endwhile
i = 0
InputHidden1:delta*=$alpha*(NDhid*(NFout))
while i<NFout
j=0
while j<NDhid
InputHidden1:delta \
+= $eta*T(Hidden_delta[i][j*NUhid->j*NUhid+NUhid_1]) \
**Input[(i+j)*NUin->(i+j)*NUin+NDin_1*NUin+NUin_1]
j+=1
endwhile
i+=1
endwhile
InputHidden1 += InputHidden1:delta/=(NDhid*(NFout))
i=0
HiddenOutput1:delta*=$alpha*(NFout)
while i<NFout
HiddenOutput1:delta+=$eta*T(Output:delta[i*NUout->i*NUout+NUout_1]) \
**Hidden[i*NUhid->i*NUhid+NUhid*NDhid_1+NUhid_1]
i+=1
endwhile
HiddenOutput1:delta/=(NFout)
HiddenOutput1+=HiddenOutput1:delta
Hidden:bias+=Hidden:biasd=Hidden:delta*$eta+Hidden:biasd*$alpha
Output:bias+=Output:biasd=Output:delta*$eta+Output:biasd*$alpha
end
Thanks in advance for your help.
-Ben Bryant
<bdbryan@eng.clemson.edu>
------------------------------
End of Neuron Digest [Volume 9 Issue 27]
****************************************