Copy Link
Add to Bookmark
Report
Neuron Digest Volume 07 Number 38
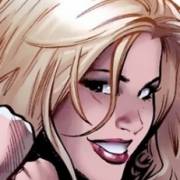
Neuron Digest Tuesday, 2 Jul 1991 Volume 7 : Issue 38
Today's Topics:
RE: Neuron Digest V7 #37
Job offer
Call For Votes: comp.org.issnnet
3D reference
Auditory Modeling
don't forget to register for COLT '91
Various IJCNN-91-Seattle info.
Re: Sixth Generation Project
TR available from neuroprose; Turing equivalence
Preprint - Efficient Visual Search
Reprints - hysteresis binary McCulloch-Pitts neuron
TR available from neuroprose; learning algorithms
preprint - Corporate Bond Rating Prediction
CRG TR - Bayesian Mixture Modeling by Monte Carlo Simulation
Send submissions, questions, address maintenance and requests for old issues to
"neuron-request@hplabs.hp.com" or "{any backbone,uunet}!hplabs!neuron-request"
Use "ftp" to get old issues from hplpm.hpl.hp.com (15.255.176.205).
----------------------------------------------------------------------
Subject: RE: Neuron Digest V7 #37
From: avlab::mrgate::"a1::raethpg"%avlab.dnet@wrdc.af.mil
Date: Mon, 27 May 91 04:11:25 -0400
From: NAME: Major Peter G. Raeth
FUNC: WRDC/AAWP-1
TEL: AV-785 513-255-7854 <RAETHPG AT A1 AT AVLAB>
To: NAME: VMSMail User "neuron <"neuron@hplpm.hpl.hp.com"@LABDDN@MRGATE>
A technical note on the use of neural networks for surface interpolation
is available. The neural network uses radial basis functions to develop
the shape of a surface based on just a few sample points. The current
proposed application is in solid modeling for CAD/CAM but there may also
be implications for classification and static-machine extrapolation. A
copy of this technical note may be obtained by slow mail:
Peter Raeth
University of Dayton Research Institute
Kettering Laboratory; KL-463
Dayton, OH 45469-0140 USA
------------------------------
Subject: Job offer
From: David Stork <stork@GUALALA.CRC.RICOH.COM>
Date: Mon, 24 Jun 91 17:36:49 -0700
The Ricoh California Research Center has an oppening for a staff
programmer or researcher in neural networks and connectionism. This
opening is for a B.S. or possibly M.S.-level graduate in Physics,
Computer Science, Math, Electrical Engineering, Cognitive Science,
Psychology, and related topics. A background in some hardware design is
a plus.
The Ricoh California Research Center is located in Menlo Park, about one
mile from Stanford University.
Contact:
Dr. David G. Stork
Ricoh California Research Center
2882 Sand Hill Road #115
Menlo Park, CA 94025-7022
stork@crc.ricoh.com
------------------------------
Subject: Call For Votes: comp.org.issnnet
From: issnnet@park.bu.edu
Date: Tue, 25 Jun 91 15:39:29 -0400
CALL FOR VOTES
----------------
GROUP NAME: comp.org.issnnet
STATUS: unmoderated
CHARTER: The newsgroup shall serve as a medium for discussions
pertaining to the International Student Society for
Neural Networks (ISSNNet), Inc., and to its activities
and programs as they pertain to the role of students
in the field of neural networks. Details were posted
in the REQUEST FOR DISCUSSION, and can be requested
from <issnnet@park.bu.edu>.
VOTING PERIOD: JUNE 25 - JULY 25, 1991
******************************************************************************
VOTING PROCESS
If you wish to vote for or against the creation of comp.org.issnnet,
please send your vote to:
issnnet@park.bu.edu
To facilitate collection and sorting of votes, please include one of
these lines in your "subject:" entry:
If you favor creation of comp.org.issnnet, your subject should read:
YES - comp.org.issnnet
If you DO NOT favor creation of comp.org.issnnet, use the subject:
NO - comp.org.issnnet
YOUR VOTE ONLY COUNTS IF SENT DIRECTLY TO THE ABOVE ADDRESS.
==----------------------------------------------------------------------
For more information, please send e-mail to issnnet@park.bu.edu (ARPANET)
write to:
ISSNNet, Inc.
PO Box 557, New Town Br.
Boston, MA 02258 USA
ISSNNet, Inc. is a non-profit corporation in the Commonwealth of
Massachusetts.
NOTE -- NEW SURFACE ADDRESS:
ISSNNet, Inc.
P.O. Box 15661
Boston, MA 02215 USA
------------------------------
Subject: 3D reference
From: demelerb@cgrb.orst.edu (Borries Demeler - Biochem)
Date: Tue, 25 Jun 91 12:47:55 -0700
>I am seeking references to recent literature on model based 3-d object
>recognition by using neural network approaches. Hope any related
>response. Thanks in advance.
A novel approach to prediction of the 3-dimensional structures of protein
backbones by neural networks.
H. Bohr et. al.
FEBS letters, Vol. 261, Number 1, 43-46, February 1990
Hope that helps, -Borries
***********************************************
* Borries Demeler *
* Oregon State University *
* Department of Biochemistry and Biophysics *
* Corvallis, OR 97331-6503 *
***********************************************
* Internet: demelerb@cgrb.orst.edu *
* Bitnet: demelerb@orstvm *
***********************************************
------------------------------
Subject: Auditory Modeling
From: xue@nimbus.anu.edu.au (Xue YANG)
Date: Sun, 30 Jun 91 11:13:48 -0500
I'm interested in recent literature on Auditory Modeling(peripheral
and/or central) by using NN techniques, and its application to speech
processing, e.g. as a front-end for speech recognition system. Hope any
related responses. Thanks in advance.
Xue YANG
Computer Sciences Lab.
Research School of Physical Sciences &Engineering
Australian National University
Canberra, ACT 2601
Australia
xue@nimbus.anu.edu.au
------------------------------
Subject: don't forget to register for COLT '91
From: David Haussler <haussler@saturn.ucsc.edu>
Date: Mon, 01 Jul 91 15:57:37 -0700
Don't forget to register for the
Workshop on Computational Learning Theory
Monday, August 5 through Wednesday, August 7, 1991
University of California, Santa Cruz, California
Conference information, program and room registration forms can be
obtained by anonymous FTP. Connect to midgard.ucsc.edu and look in the
directory pub/colt. Alternatively, send E-mail to "colt@cis.ucsc.edu"
for instructions on obtaining the forms by electronic mail. Early
registration discounts are ending NOW.
If you have questions regarding registration or accommodations, contact:
Jean McKnight, COLT '91, Dept. of Computer Science, UCSC, Santa Cruz, CA
95064. Her emergency phone number is (408) 459-2303, but she prefers
E-mail to jean@cs.ucsc.edu or facsimile at (408) 429-0146. Proceedings
from the workshop will be published by Morgan Kaufmann.
-David
------------------------------
Subject: Various IJCNN-91-Seattle info.
From: Don Wunsch <dwunsch@atc.boeing.com>
Date: Mon, 01 Jul 91 18:15:00 -0700
IJCNN-91-Seattle is almost upon us! Come to Seattle July 8-12 for the
largest neural network, hybrid, and fuzzy systems conference in the
world. Researchers, scientists, engineers, consultants, applications
specialists and students from a variety of disciplines will present
invited and contributed papers, tutorials, panel discussions, exhibits
and demos. Exhibitors will also present the latest in neural networks,
including neurocomputers, VLSI neural networks, software systems and
applications.
Conference registration is $395 for IEEE or INNS members, $495 for
non-members, and $95 for students. Tutorials are $295 for everyone
except students, who pay $85. One-day registration is $125 for members
or $175 for non-members. The tutorial fee includes all the tutorials you
choose to attend, not just one. Also, students are full conference
participants, receiving a proceedings and admission to all events,
including social events.
To register by VISA or MasterCard, call (206) 543-2310, or FAX a
registration form (containing your name, title, affiliation, full
address, country, full daytime phone number, FAX number, e-mail address,
and registration for the conference or tutorials, with total fees and
credit card number with name as it appears on card) to (206) 685-9359.
Finally, for those of you who will be attending the conference, you have
an opportunity to attend a tour of the world's largest manufacturing
facility. Read on for further details and reservation information:
Boeing will be offering a limited number of free tours of its
manufacturing plant to attendees of the International Joint Conference on
Neural Networks, on a first-come, first-served basis. Families may also
be accomodated, but only if space is available. See the 747 and 767
being built, in the largest volume building in the world. We will also
be providing transportation leaving he conference site one hour before
the tour begins. The tour lasts approximately one hour. Tour times are
Monday through Friday at 10:30 AM and 3:00 PM. Therefore, be prepared to
leave the conference site at 9:30 AM or 2:00 PM for your chosen tour.
Please indicate your preferred tour times and send a fax of this form
with your name, number of persons on the tour, and hotel (if known).
Preferred time:_________________ Alternate choice:___________________
Second alternate:_____________________________________________________
Name (please print): _________________________________________________
Number of persons: __________ Hotel:___________________________
Please do not reply to this address. Instead, please send a FAX to Jean
Norris at (206) 865-2957. If you have any questions, call her at (206)
865-5616. Alternatively, you may e-mail the form or any questions to
David Newman at: dnewman@caissa.boeing.com. If you will not be staying
at a hotel, please put down a number where you can be reached.
There will be a tour coordination booth available at the time of
registration, where further information is available, and tickets may be
picked up. The best time to schedule your tour will probably be Monday,
before things start heating up. This tour is actually a very hot tourist
attraction around here, because of the universal fascination with flight.
So it will be well worth your while--don't miss it!
We look forward to seeing you in Seattle!
Don Wunsch
Local Arrangements Chair
IJCNN-91-Seattle
------------------------------
Subject: Re: Sixth Generation Project
From: David Kanecki <kanecki@vacs.uwp.edu>
Date: Mon, 01 Jul 91 19:41:26 -0500
Re: Comment on Sixth Generation Project
Basis, the ten year goal of the sixth generation program is to develop
computers to mimic the human brain and improve intelligent manufacturing
systems.
Based upon an article in Nature the New Information Processing Technology
project, sixth generation, is planning to invest $30-40 million per year
for ten years which I think will help expand the use and range of
computing and information solving technologies.
One suggestion I would make is that the group in charge of the project
should open it bids to individuals, academia, and industry. The reason
for this was that with PDP-8's and the cooperation of many talented
individuals mankind was able to land on the moon in 1969. But, with
advanced micros and a fragmented non cooperative culture the best
achievement thus far has been the space shuttle.
I think that if the computer and knowledge based communities can unify
and accept each other's diversity one will see the growth reappear that
was present in the early computer age (1956-1970) and early micro
computer age (1973-1980). During these stages the diversity allowed
groups to train future mentors and leaders. I think the above diversity
was better than the one operating system and one computer system mind
think that is currently in vogue. Or, in another way it its better to
try different alternatives and learn something each day than nothing at
all...
"We Came in Peace...", 1969 Apollo Program
"Dare to be different", 1990 New Age Phrase
"Logic is the integral of Excellence, Creativity, Technology, and the
Analytical Mind", 1991 David H. Kanecki
David H. Kanecki, Bio. Sci., A.C.S.
kanecki@vacs.uwp.wisc.edu
------------------------------
Subject: TR available from neuroprose; Turing equivalence
From: siegelma@yoko.rutgers.edu
Date: Sun, 09 Jun 91 10:56:40 -0400
The following report is now available from the neuroprose archive:
NEURAL NETS ARE UNIVERSAL COMPUTING DEVICES
H. T. Siegelmann and E.D. Sontag. (13pp.)
Abstract: It is folk knowledge that neural nets should be capable of
simulating arbitrary computing devices. Past formalizations of this fact
have been proved under the hypotheses that there are potentially
infinitely many neurons available during a computation and/or that
interconnections are multiplicative. In this work, we show the existence
of a finite network, made up of sigmoidal neurons, which simulates a
universal Turing machine. It is composed of less than 100,000
synchronously evolving processors, interconnected linearly.
-Hava
=---------------------------------------------------------------------------
To obtain copies of the postscript file, please use Jordan Pollack's service:
Example:
unix> ftp cheops.cis.ohio-state.edu # (or ftp 128.146.8.62)
Name (cheops.cis.ohio-state.edu:): anonymous
Password (cheops.cis.ohio-state.edu:anonymous): <ret>
ftp> cd pub/neuroprose
ftp> binary
ftp> get
(remote-file) siegelman.turing.ps.Z
(local-file) siegelman.turing.ps.Z
ftp> quit
unix> uncompress siegelman.turing.ps.Z
unix> lpr -P(your_local_postscript_printer) siegelman.turing.ps
=------------------------------------------------------------------------
If you have any difficulties with the above, please send e-mail to
siegelma@paul.rutgers.edu. DO NOT "reply" to this message, please.
------------------------------
Subject: Preprint - Efficient Visual Search
From: Subutai Ahmad <ahmad@ICSI.Berkeley.EDU>
Date: Mon, 10 Jun 91 15:58:12 -0700
The following paper (to appear in this Cognitive Science proceedings) is
available from the neuroprose archives as ahmad.cogsci91.ps.Z (ftp
instructions below).
Efficient Visual Search: A Connectionist Solution
by
Subutai Ahmad & Stephen Omohundro
International Computer Science Institute
Abstract
Searching for objects in scenes is a natural task for people and has been
extensively studied by psychologists. In this paper we examine this task
from a connectionist perspective. Computational complexity arguments
suggest that parallel feed-forward networks cannot perform this task
efficiently. One difficulty is that, in order to distinguish the target
from distractors, a combination of features must be associated with a
single object. Often called the binding problem, this requirement
presents a serious hurdle for connectionist models of visual processing
when multiple objects are present. Psychophysical experiments suggest
that people use covert visual attention to get around this problem. In
this paper we describe a psychologically plausible system which uses a
focus of attention mechanism to locate target objects. A strategy that
combines top-down and bottom-up information is used to minimize search
time. The behavior of the resulting system matches the reaction time
behavior of people in several interesting tasks.
A postscript version of the paper can be obtained by ftp from
cheops.cis.ohio-state.edu. The file is ahmad.cogsci91.ps.Z in the
pub/neuroprose directory. You can either use the Getps script or follow
these steps:
unix:2> ftp cheops.cis.ohio-state.edu
Connected to cheops.cis.ohio-state.edu.
Name (cheops.cis.ohio-state.edu:): anonymous
331 Guest login ok, send ident as password.
Password: neuron
230 Guest login ok, access restrictions apply.
ftp> cd pub/neuroprose
ftp> binary
ftp> get ahmad.cogsci91.ps.Z
ftp> quit
unix:4> uncompress ahmad.cogsci91.ps.Z
unix:5> lpr ahmad.cogsci91.ps
Subutai
ahmad@icsi.berkeley.edu
------------------------------
Subject: Reprints - hysteresis binary McCulloch-Pitts neuron
From: takefuji@axon.eeap.cwru.edu (Yoshiyasu Takefuji)
Date: Tue, 11 Jun 91 21:00:34 -0400
The following reprints are available from Center for Automation and
Intelligent Systems Research (CAISR) at Case Western Reserve
University. Send your request to Lawrence Boyd, CAISR, Case Western
Reserve University, Cleveland, OH 44106. Phone 216-368-6434.
Each paper may cost $2 including handling and mailing.
TR 91-131: Y. Takefuji and K. C. Lee, An artificial hysteresis binary
neuron: a model suppressing the oscillatory behaviors of neural dynamics,
published in Biological Cybernetics, 64, 353-356, 1991.
ABSTRACT
A hysteresis binary McCulloch-Pitts neuron model is proposed in order to
suppress the complicated oscillatory behaviors of neural dynamics. The
artificial hysteresis binary neural network is used for scheduling
time-multiplex crossbar switches in order to demonstrate the effects of
hysteresis. Time-multiplex crossbar switching system must control
traffic on demand such that packet blocking probability and packet
waiting time are minimized. The system using n x n processing elements
solves an n x n crossbar-control problem with O(1) time, while the best
existing parallel algorithm requires O(n) time. The hysteresis binary
neural network maximizes the throughput of packets through a crossbar
switch. The solution quality of our system does not degrade with the
problem size.
------------------------------
Subject: TR available from neuroprose; learning algorithms
From: Knut Moeller <moeller@kiti.informatik.uni-bonn.de>
Date: Thu, 13 Jun 91 09:50:34 +0200
The following report is now available from the neuroprose archive:
LEARNING BY ERROR-DRIVEN DECOMPOSITION
D.Fox V.Heinze K.Moeller S.Thrun G.Veenker (6pp.)
Abstract: In this paper we describe a new selforganizing decomposition
technique for learning high-dimensional mappings. Problem decomposition
is performed in an error-driven manner, such that the resulting subtasks
(patches) are equally well approximated. Our method combines an
unsupervised learning scheme (Feature Maps [Koh84]) with a nonlinear
approximator (Backpropagation [RHW86]). The resulting learning system is
more stable and effective in changing environments than plain
backpropagation and much more powerful than extended feature maps as
proposed by [RMW89]. Extensions of our method give rise to active
exploration strategies for autonomous agents facing unknown environments.
The appropriateness of this technique is demonstrated with an example
from mathematical function approximation.
=-----------------------------------------------------------------------------
To obtain copies of the postscript file, please use Jordan Pollack's service:
Example:
unix> ftp cheops.cis.ohio-state.edu # (or ftp 128.146.8.62)
Name (cheops.cis.ohio-state.edu:): anonymous
Password (cheops.cis.ohio-state.edu:anonymous): <ret>
ftp> cd pub/neuroprose
ftp> binary
ftp> get
(remote-file) fox.decomp.ps.Z
(local-file) fox.decomp.ps.Z
ftp> quit
unix> uncompress fox.decomp.ps.Z
unix> lpr -P((your_local_postscript_printer) fox.decomp.ps.Z
=----------------------------------------------------------------------------
If you have any difficulties with the above, please send e-mail to
moeller@kiti.informatik.uni-bonn.de
DO NOT "reply" to this message!!
------------------------------
Subject: preprint - Corporate Bond Rating Prediction
From: Joachim Utans <utans-joachim@CS.YALE.EDU>
Date: Sat, 15 Jun 91 12:48:45 -0400
The following preprint has been placed in the neuroprose archive at Ohio
State University:
Selecting Neural Network Architectures via the Prediction Risk:
Application to Corporate Bond Rating Prediction
Joachim Utans John Moody
Department of Electrical Engineering Department of Computer Science
Yale University Yale University
New Haven, CT 06520 New Haven, CT 06520
Abstract:
Intuitively, the notion of generalization is closely related to the
ability of an estimator to perform well with new observations. In
this paper, we propose the prediction risk as a measure of the
generalization ability of multi-layer perceptron networks and use it
to select the optimal network architecture. The prediction risk needs
to be estimated from the available data; here we approximate the
prediction risk by v-fold cross-validation and asymtotic estimates of
generalized cross-validation or Akaike's final prediction error. We
apply the technique to the problem of predicting corporate bond
ratings. This problem is very attractive as a case study, since it is
characterized by the limited availability of the data and by the lack
of complete a priori information that could be used to impose a
structure to the network architecture.
To retrieve it by anonymous ftp:
unix> ftp cheops.cis.ohio-state.edu # (or ftp 128.146.8.62)
Name (cheops.cis.ohio-state.edu:): anonymous
Password (cheops.cis.ohio-state.edu:anonymous): neuron
ftp> cd pub/neuroprose
ftp> binary
ftp> get utans.bondrating.ps.Z
ftp> quit
unix> uncompress utans.bondrating.ps
unix> lpr -P(your_local_postscript_printer) utans.bondrating.ps
Joachim Utans
------------------------------
Subject: CRG TR - Bayesian Mixture Modeling by Monte Carlo Simulation
From: Maureen Smith <maureen@ai.toronto.edu>
Date: Wed, 19 Jun 91 11:38:49 -0400
The following technical report is available for ftp from the neuroprose
archive. A hardcopy may also be requested. (See below for details.)
Though written for a statistics audience, this report should be of
interest to connectionists and others interested in machine learning, as
it reports a Bayesian solution for one type of "unsupervised concept
learning". The technique employed is also related to that used in
Boltzmann Machines.
Bayesian Mixture Modeling by Monte Carlo Simulation
Radford M. Neal
Technical Report CRG-TR-91-2
Department of Computer Science
University of Toronto
It is shown that Bayesian inference from data modeled by a mixture
distribution can feasibly be performed via Monte Carlo simulation.
This method exhibits the true Bayesian predictive distribution,
implicitly integrating over the entire underlying parameter space.
An infinite number of mixture components can be accommodated without
difficulty, using a prior distribution for mixing proportions that
selects a reasonable subset of components to explain any finite
training set. The need to decide on a ``correct'' number of
components is thereby avoided. The feasibility of the method is shown
empirically for a simple classification task.
To obtain a compressed PostScript version of this report from neuroprose,
ftp to "cheops.cis.ohio-state.edu" (128.146.8.62), log in as "anonymous"
with password "neuron", set the transfer mode to "binary", change to the
directory "pub/neuroprose", and get the file "neal.bayes.ps.Z". Then use
the command "uncompress neal.bayes.ps.Z" to convert the file to
PostScript.
To obtain a hardcopy version of the paper by physical mail, send mail
to : Maureen Smith
Department of Computer Science
University of Toronto
6 King's College Road
Toronto, Ontario
M5A 1A4
------------------------------
End of Neuron Digest [Volume 7 Issue 38]
****************************************