Copy Link
Add to Bookmark
Report
Neuron Digest Volume 07 Number 33
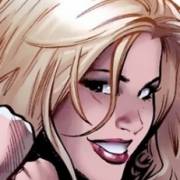
Neuron Digest Saturday, 8 Jun 1991 Volume 7 : Issue 33
Today's Topics:
NETWORK - contents of Volume 2, no 2 (May 1991)
Int. J. of Neural Systems - Contents and CFP
field computation papers
TR's available (via ftp)
TR - Connectionist Models of Rule-Based Reasoning
Technical report on learning in recurrent networks
Connectionist Book Announcement
ordering of announced book
Preprints on Statistical Mechanics of Learning
TR - Competitive Hebbian Learning
Preprint: Effects of Word Abstractness in a Connectionist Model of Deep Dyslexi
TR: Bayesian Inference on Visual Grammars by NNs that Optimize
Send submissions, questions, address maintenance and requests for old issues to
"neuron-request@hplabs.hp.com" or "{any backbone,uunet}!hplabs!neuron-request"
Use "ftp" to get old issues from hplpm.hpl.hp.com (15.255.176.205).
------------------------------------------------------------
Subject: NETWORK - contents of Volume 2, no 2 (May 1991)
From: David Willshaw <david@cns.edinburgh.ac.uk>
Date: Tue, 07 May 91 11:49:10 +0100
The forthcoming May 1991 issue of NETWORK will contain the following papers:
NETWORK
Volume 2 Number 2 May 1991
Minimum-entropy coding with Hopfield networks
H G E Hentschel and H B Barlow
Cellular automation models of the CA3 region of the hippocampus
E Pytte, G Grinstein and R D Traub
Competitive learning, natural images and cortical cells
C J StC Webber
Adaptive fields: distributed representations of classically
conditioned associations
P F M J Verschure and A C C Coolen
``Quantum'' neural networks
M Lewenstein and M Olko
----------------------
NETWORK welcomes research Papers and Letters where the findings have
demonstrable relevance across traditional disciplinary boundaries.
Research Papers can be of any length, if that length can be justified by
content. Rarely, however, is it expected that a length in excess of
10,000 words will be justified. 2,500 words is the expected limit for
research Letters. Articles can be published from authors' TeX source
codes.
NETWORK is published quarterly. The subscription rates are:
Institution 125.00 POUNDS (US$220.00)
Individual (UK) 17.30 POUNDS
(Overseas) 20.50 POUNDS (US$37.90)
For more details contact
IOP Publishing
Techno House
Redcliffe Way
Bristol BS1 6NX
United Kingdom
Telephone: 0272 297481
Fax: 0272 294318
Telex: 449149 INSTP G
EMAIL: JANET: IOPPL@UK.AC.RL.GB
------------------------------
Subject: Int. J. of Neural Systems - Contents and CFP
From: BRUNAK@nbivax.nbi.dk
Date: Fri, 17 May 91 12:03:00 +0200
INTERNATIONAL JOURNAL OF NEURAL SYSTEMS
The International Journal of Neural Systems is a quarterly journal
which covers information processing in natural and artificial neural
systems. It publishes original contributions on all aspects of this
broad subject which involves physics, biology, psychology, computer
science and engineering. Contributions include research papers, reviews
and short communications. The journal presents a fresh undogmatic
attitude towards this multidisciplinary field with the aim to be a
forum for novel ideas and improved understanding of collective and
cooperative phenomena with computational capabilities.
ISSN: 0129-0657 (IJNS)
==----------------------------------
Contents of Volume 2, issues number 1-2 (1991):
1. H. Liljenstrom:
Modelling the dynamics of olfactory cortex effects using
simplified network units and realistic architecture.
2. S. Becker:
Unsupervised learning procedures for neural networks.
3. Y. Chauvin:
Constrained Hebbian Learning: Gradient descent to global minima
in a n-dimensional landscape.
4. J. G. Taylor:
Neural network capacity for temporal sequence storage.
5. S. Z. Lerner and J. R. Deller:
Speech recognition by a self-organising feature finder.
6. Jefferey Lee Johnson:
Modelling head end escape behaviour in the earthworm: the
efferent arc and the end organ.
7. M.-Y. Chow, G. Bilbro and S. O. Yee:
Application of Learning Theory for a Single Phase Induction
Motor Incipient Fault Detector Artificial Neural Network.
8. J. Tomberg and K. Kaski:
Some IC implementations of artificial neural networks using
synchronous pulse-density modulation technique.
9. I. Kocher and R. Monasson:
Generalisation error and dynamical efforts in a two-dimensional
patches detector.
10. J. Schmidhuber and R. Huber:
Learning to generate fovea trajectories for attentive vision.
11. A. Hartstein:
A back-propagation algorithm for a network of neurons with
threshold controlled synapses.
12. M. Miller and E. N. Miranda:
Stability of multi-layered neural networks.
13. J. Ariel Sirat:
A fast neural algorithm for principal components analysis and
singular value decomposition.
14. D. Stork:
Review of "Introduction to the Theory of Neural Computation",
by J. Hertz, A. Krogh and R. Palmer.
==----------------------------------
Editorial board:
B. Lautrup (Niels Bohr Institute, Denmark) (Editor-in-charge)
S. Brunak (Technical Univ. of Denmark) (Assistant Editor-in-Charge)
D. Stork (Stanford) (Book review editor)
Associate editors:
B. Baird (Berkeley)
D. Ballard (University of Rochester)
E. Baum (NEC Research Institute)
S. Bjornsson (University of Iceland)
J. M. Bower (CalTech)
S. S. Chen (University of North Carolina)
R. Eckmiller (University of Dusseldorf)
J. L. Elman (University of California, San Diego)
M. V. Feigelman (Landau Institute for Theoretical Physics)
F. Fogelman-Soulie (Paris)
K. Fukushima (Osaka University)
A. Gjedde (Montreal Neurological Institute)
S. Grillner (Nobel Institute for Neurophysiology, Stockholm)
T. Gulliksen (University of Oslo)
D. Hammerstrom (Oregon Graduate Institute)
J. Hounsgaard (University of Copenhagen)
B. A. Huberman (XEROX PARC)
L. B. Ioffe (Landau Institute for Theoretical Physics)
P. I. M. Johannesma (Katholieke Univ. Nijmegen)
M. Jordan (MIT)
G. Josin (Neural Systems Inc.)
I. Kanter (Princeton University)
J. H. Kaas (Vanderbilt University)
A. Lansner (Royal Institute of Technology, Stockholm)
A. Lapedes (Los Alamos)
B. McWhinney (Carnegie-Mellon University)
M. Mezard (Ecole Normale Superieure, Paris)
J. Moody (Yale, USA)
A. F. Murray (University of Edinburgh)
J. P. Nadal (Ecole Normale Superieure, Paris)
E. Oja (Lappeenranta University of Technology, Finland)
N. Parga (Centro Atomico Bariloche, Argentina)
S. Patarnello (IBM ECSEC, Italy)
P. Peretto (Centre d'Etudes Nucleaires de Grenoble)
C. Peterson (University of Lund)
K. Plunkett (University of Aarhus)
S. A. Solla (AT&T Bell Labs)
M. A. Virasoro (University of Rome)
D. J. Wallace (University of Edinburgh)
D. Zipser (University of California, San Diego)
==----------------------------------
CALL FOR PAPERS
Original contributions consistent with the scope of the journal are
welcome. Complete instructions as well as sample copies and
subscription information are available from
The Editorial Secretariat, IJNS
World Scientific Publishing Co. Pte. Ltd.
73, Lynton Mead, Totteridge
London N20 8DH
ENGLAND
Telephone: (44)81-446-2461
or
World Scientific Publishing Co. Inc.
687 Hardwell St.
Teaneck
New Jersey 07666
USA
Telephone: (1)201-837-8858
or
World Scientific Publishing Co. Pte. Ltd.
Farrer Road, P. O. Box 128
SINGAPORE 9128
Telephone (65)382-5663
------------------------------
Subject: field computation papers
From: mclennan@cs.utk.edu
Date: Tue, 21 May 91 22:07:04 -0400
There have been several requests for my papers on field computation. In
addition to an early paper in the first IEEE ICNN (San Diego, 1987),
there are several reports in the neuroprose directory:
maclennan.contincomp.ps.Z -- a short introduction
maclennan.fieldcomp.ps.Z -- the current most comprehensive report
maclennan.csa.ps.Z -- continuous spatial automata
Of course I will be happy to send out hardcopy of these papers or several
others not in neuroprose.
Bruce MacLennan
Department of Computer Science
The University of Tennessee
Knoxville, TN 37996-1301
(615)974-5067
maclennan@cs.utk.edu
Here are the directions for accessing files from neuroprose. Note that
there is also in the directory a script called Getps that does all the
work.
unix> ftp cheops.cis.ohio-state.edu (or 128.146.8.62)
Name: anonymous
Password: neuron
ftp> cd pub/neuroprose
ftp> binary
ftp> get maclennan.csa.ps.Z
ftp> quit
unix> uncompress maclennan.csa.ps.Z
unix> lpr maclennan.csa.ps (or however you print postscript)
------------------------------
Subject: TR's available (via ftp)
From: "B. Fritzke" <fritzke@immd2.informatik.uni-erlangen.de>
Date: Wed, 22 May 91 18:03:46 +0700
Hi there,
I just have placed two short papers in the Neuroprose Archive at
cheops.cis.ohio-state.edu (128.146.8.62) in the directory pub/neuroprose.
The files are:
fritzke.cell_structures.ps.Z (to be presented at ICANN-91 Helsinki)
fritzke.clustering.ps.Z (to be presented at IJCNN-91 Seattle)
They both deal with a new self-organizing network based on the model of
Kohonen. The first one describes the model and the second one concentrates
one an application.
LET IT GROW -- SELF-ORGANIZING FEATURE MAPS WITH
PROBLEM DEPENDENT CELL STRUCTURE
Bernd FRITZKE
Abstract: The self-organizing feature maps introduced by T.
Kohonen use a cell array of fixed size and structure. In many
cases this array is not able to model a given signal distribution
properly. We present a method to construct two-dimensional cell
structures during a self-organization process which are specially
adapted to the underlying distribution: Starting with a small
number of cells new cells are added successively. Thereby signal
vectors according to the (usually not explicitly known) probabil-
ity distribution are used to determine where to insert or delete
cells in the current structure. This process leads to problem
dependent cell structures which model the given distribution with
arbitrary high accuracy.
UNSUPERVISED CLUSTERING WITH GROWING CELL STRUCTURES
Bernd FRITZKE
Abstract: A Neural Network model is presented which is able to
detect clusters of similar patterns. The patterns are n-
dimensional real number vectors according to an unknown proba-
bility distribution P(X). By evaluating sample vectors ac-
cording to P(X) a two-dimensional cell structure is gradually
built up which models the distribution. Through removal of
cells corresponding to areas with low probability density the
structure is then split into several disconnected substruc-
tures. Each of them identifies one cluster of similar patterns.
Not only the number of clusters is determined but also an ap-
proximation of the probability distribution inside each cluster.
The accuracy of the cluster description is increased linearly
with the number of evaluated sample vectors.
Enjoy,
Bernd
Bernd Fritzke ----------> e-mail: fritzke@immd2.informatik.uni-erlangen.de
University of Erlangen, CS IMMD II, Martensstr. 3, 8520 Erlangen (Germany)
------------------------------
Subject: TR - Connectionist Models of Rule-Based Reasoning
From: Ron Sun <rsun@chaos.cs.brandeis.edu>
Date: Thu, 23 May 91 16:32:23 -0400
The following paper will appear in the Proc.13th Annual Conference of
Cognitive Science Society. It is a revised version of an earlier TR
entitle "Integrating Rules and Connectionism for Robust Reasoning"
Connectionist Models of Rule-Based Reasoning
Ron Sun
Brandeis University
Computer Science Department
rsun@cs.brandeis.edu
We investigate connectionist models of rule-based reasoning, and show
that while such models usually carry out reasoning in exactly the same
way as symbolic systems, they have more to offer in terms of commonsense
reasoning. A connectionist architecture for commonsense reasoning,
CONSYDERR, is proposed to account for common reasoning patterns and to
remedy the brittleness problem in traditional rule-based systems. A dual
representational scheme is devised, which utilizes both localist and
distributed representations and explores the synergy resulting from the
interaction between the two. {CONSYDERR} is therefore capable of
accounting for many difficult patterns in commonsense reasoning. This
work shows that connectionist models of reasoning are not just
``implementations" of their symbolic counterparts, but better
computational models of commonsense reasoning.
=------------ FTP procedures -------------------------
(thanks to the service provided by Jordan Pollack) ---
ftp cheops.cis.ohio-state.edu
>name: anonymous
>passwork: neuron
>binary
>cd pub/neuroprose
>get sun.cogsci91.ps.Z
>quit
uncompress sun.integrate.ps.Z
lpr sun.cogsci91.ps
------------------------------
Subject: Technical report on learning in recurrent networks
From: Erol Gelenbe <erol@ehei.ehei.fr>
Date: Thu, 23 May 91 16:35:53
You may obtain a hard copy of the following tech report by sending me
e-mail :
Learning in the Recurrent Random Network
by
Erol Gelenbe
EHEI
45 rue des Saints-Peres
75006 Paris
This paper describes an "exact" learning algorithm for the recurrent
random network model (see E. Gelenbe in Neural Computation, Vol 2, No 2,
1990). The algorithm is based on the delta rule for updating the network
weights. Computationally, each step requires the solution of n non-linear
equations (solved in time Kn where K is a constant) and 2n linear
equations for the derivatives. Thus it is of O(n**3) complexity, where n
is the number of neurons.
------------------------------
Subject: Connectionist Book Announcement
From: jbarnden@NMSU.Edu
Date: Fri, 24 May 91 12:48:40 -0600
CONNECTIONIST BOOK ANNOUNCEMENT
===============================
Barnden, J.A. & Pollack, J.B. (Eds). (1991).
Advances in Connectionist and Neural Computation Theory, Vol. 1:
High Level Connectionist Models.
Norwood, N.J.: Ablex Publishing Corp.
=------------------------------------------------
ISBN 0-89391-687-0
Location index QA76.5.H4815 1990
389 pp.
Extensive subject index.
Cost $34.50 for individuals and course adoption.
For more information:
jbarnden@nmsu.edu, pollack@cis.ohio-state.edu
=------------------------------------------------
MAIN CONTENTS:
David Waltz
Foreword
John A. Barnden & Jordan B. Pollack
Introduction: problems for high level connectionism
David S. Touretzky
Connectionism and compositional semantics
Michael G. Dyer
Symbolic NeuroEngineering for natural language processing:
a multilevel research approach.
Lawrence Bookman & Richard Alterman
Schema recognition for text understanding:
an analog semantic feature approach
Eugene Charniak & Eugene Santos
A context-free connectionist parser which is not connectionist,
but then it is not really context-free either
Wendy G. Lehnert
Symbolic/subsymbolic sentence analysis:
exploiting the best of two worlds.
James Hendler
Developing hybrid symbolic/connectionist models
John A. Barnden
Encoding complex symbolic data structures
with some unusual connectionist techniques
Mark Derthick
Finding a maximally plausible model of an inconsistent theory
Lokendra Shastri
The relevance of connectionism to AI:
a representation and reasoning perspective
Joachim Diederich
Steps toward knowledge-intensive connectionist learning
Garrison W. Cottrell & Fu-Sheng Tsung
Learning simple arithmetic procedures.
Jiawei Hong & Xiaonan Tan
The similarity between connectionist and other parallel computation models
Lawrence Birnbaum
Complex features in planning and understanding:
problems and opportunities for connectionism
Jordan Pollack & John Barnden
Conclusion
------------------------------
Subject: ordering of announced book
From: jbarnden@NMSU.Edu
Date: Tue, 28 May 91 09:41:45 -0600
ADDENDUM TO A BOOK ANNOUNCEMENT
===============================
Several people have asked about ordering a copy of a book I announced
recently.
This message includes publisher's address and ordering-department phone
number.
Barnden, J.A. & Pollack, J.B. (Eds). (1991).
Advances in Connectionist and Neural Computation Theory, Vol. 1:
High Level Connectionist Models.
Norwood, N.J.: Ablex Publishing Corp.
355 Chestnut Street, Norwood, NJ 07648-2090
Order Dept.: (201) 767-8455
ISBN 0-89391-687-0
Location index QA76.5.H4815 1990
389 pp.
Extensive subject index.
Cost $34.50 for individuals and course adoption.
For more information:
jbarnden@nmsu.edu, pollack@cis.ohio-state.edu
------------------------------
Subject: Preprints on Statistical Mechanics of Learning
From: nzt@research.att.com
Date: Sat, 25 May 91 09:50:38 -0400
The following preprints are available by ftp from the neuroprose archive
at cheops.cis.ohio-state.edu.
1. Statistical Mechanics of Learning from Examples
I: General Formulation and Annealed Approximation
2. Statistical Mechanics of Learning from Examples
II: Quenched Theory and Unrealizable Rules
by: Sebastian Seung, Haim Sompolinsky, and Naftali Tishby
This is a two part detailed analytical and numerical study of learning
curves in large neural networks, using techniques of equilibrium
statistical mechanics.
Abstract - Part I
Learning from examples in feedforward neural networks is studied using
equilibrium statistical mechanics. Two simple approximations to the
exact quenched theory are presented: the high temperature limit and the
annealed approximation. Within these approximations, we study four
models of perceptron learning of realizable target rules. In each
model, the target rule is perfectly realizable because it is another
perceptron of identical architecture. We focus on the generalization
curve, i.e. the average generalization error as a function of the
number of examples. The case of continuously varying weights is
considered first, for both linear and boolean output units. In these
two models, learning is gradual, with generalization curves that
asymptotically obey inverse power laws. Two other model perceptrons,
with weights that are constrained to be discrete, exhibit sudden
learning. For a linear output, there is a first-order transition
occurring at low temperatures, from a state of poor generalization to a
state of good generalization. Beyond the transition, the
generalization curve decays exponentially to zero. For a boolean
output, the first order transition is to perfect generalization at all
temperatures. Monte Carlo simulations confirm that these approximate
analytical results are quantitatively accurate at high temperatures and
qualitatively correct at low temperatures. For unrealizable rules the
annealed approximation breaks down in general, as we illustrate with a
final model of a linear perceptron with unrealizable threshold.
Finally, we propose a general classification of generalization curves
in models of realizable rules.
Abstract - Part II
Learning from examples in feedforward neural networks is studied using
the replica method. We focus on the generalization curve, which is
defined as the average generalization error as a function of the number
of examples. For smooth networks, i.e. those with continuously
varying weights and smooth transfer functions, the generalization curve
is found to asymptotically obey an inverse power law. This implies
that generalization curves in smooth networks are generically gradual.
In contrast, for discrete networks, discontinuous learning transitions
can occur. We illustrate both gradual and discontinuous learning with
four single-layer perceptron models. In each model, a perceptron is
trained on a perfectly realizable target rule, i.e. a rule that is
generated by another perceptron of identical architecture. The replica
method yields results that are qualitatively similar to the approximate
results derived in Part I for these models. We study another class of
perceptron models, in which the target rule is unrealizable because it
is generated by a perceptron of mismatched architecture. In this class
of models, the quenched disorder inherent in the random sampling of the
examples plays an important role, yielding generalization curves that
differ from those predicted by the simple annealed approximation of
Part I. In addition this disorder leads to the appearance of
equilibrium spin glass phases, at least at low temperatures.
Unrealizable rules also exhibit the phenomenon of overtraining, in
which training at zero temperature produces inferior generalization to
training at nonzero temperature.
Here's what to do to get the files from neuroprose:
unix> ftp cheops.cis.ohio-state.edu (or 128.146.8.62)
Name: anonymous
Password: neuron
ftp> cd pub/neuroprose
ftp> binary
ftp> get tishby.sst1.ps.Z
ftp> get tishby.sst2.ps.Z
ftp> quit
unix> uncompress tishby.sst*
unix> lpr tishby.sst* (or however you print postscript)
Sebastian Seung
Haim Sompolinsky
Naftali Tishby
------------------------------
Subject: TR - Competitive Hebbian Learning
From: Ray White <white@teetot.acusd.edu>
Date: Wed, 29 May 91 11:51:32 -0700
This notice is to announce a short paper which will be presented
at IJCNN-91 Seattle.
COMPETITIVE HEBBIAN LEARNING
Ray H. White
Departments of Physics and Computer Science
University of San Diego
Abstract
Of crucial importance for applications of unsupervised learning
to systems of many nodes with a common set of inputs is how the
nodes may be trained to collectively develop optimal response to
the input. In this paper Competitive Hebbian Learning, a modified
Hebbian-learning rule, is introduced. In Competitive Hebbian
Learning the change in each connection weight is made proportional
to the product of node and input activities multiplied by a factor
which decreases with increasing activity on the other nodes. The
individual nodes learn to respond to different components of the
input activity while collectively developing maximal response.
Several applications of Competitive Hebbian Learning are then
presented to show examples of the power and versatility of this
learning algorithm.
This paper has been placed in Jordan Pollack's neuroprose archive at Ohio
State, and may be retrieved by anonymous ftp. The title of the file
there is
white.comp-hebb.ps.Z
and it may be retrieved by the usual procedure:
local> ftp cheops.cis.ohio-state.edu (or ftp 128.146.8.62)
Name(128.146.8.62:xxx) anonymous
password: neuron
ftp> cd pub/neuroprose
ftp> binary
ftp> get white.comp-hebb.ps.Z
ftp> quit
local> uncompress white.comp-hebb.ps.Z
local> lpr -P(your_local_postscript_printer) white.comp-hebb.ps
Ray White (white@teetot.acusd.edu or white@cogsci.ucsd.edu)
------------------------------
Subject: Preprint: Effects of Word Abstractness in a Connectionist Model
of Deep Dyslexia
From: David Plaut <dcp+@cs.cmu.edu>
Date: Mon, 03 Jun 91 15:51:50 -0400
The following paper is available in the neuroprose archive as
plaut.cogsci91.ps.Z. It will appear in this year's Cognitive Science
Conference proceedings. A much longer paper presenting a wide range of
related work is in preparation and will be announced shortly.
Effects of Word Abstractness in a Connectionist Model of Deep Dyslexia
David C. Plaut Tim Shallice
School of Computer Science Department of Psychology
Carnegie Mellon University University College, London
dcp@cs.cmu.edu ucjtsts@ucl.ac.uk
Deep dyslexics are patients with neurological damage who exhibit a
variety of symptoms in oral reading, including semantic, visual and
morphological effects in their errors, a part-of-speech effect, and
better performance on concrete than abstract words. Extending work by
Hinton & Shallice (1991), we develop a recurrent connectionist network
that pronounces both concrete and abstract words via their semantics,
defined so that abstract words have fewer semantic features. The
behavior of this network under a variety of ``lesions'' reproduces the
main effects of abstractness on deep dyslexic reading: better correct
performance for concrete words, a tendency for error responses to be more
concrete than stimuli, and a higher proportion of visual errors in
response to abstract words. Surprisingly, severe damage within the
semantic system yields better performance on *abstract* words,
reminiscent of CAV, the single, enigmatic patient with ``concrete word
dyslexia.''
To retrieve this from the neuroprose archive type the following:
unix> ftp 128.146.8.62
Name: anonymous
Password: neuron
ftp> binary
ftp> cd pub/neuroprose
ftp> get plaut.cogsci91.ps.Z
ftp> quit
unix> zcat plaut.cogsci91.ps.Z | lpr
=---------------------------------------------------------------------
David Plaut dcp+@cs.cmu.edu
School of Computer Science 412/268-8102
Carnegie Mellon University
Pittsburgh, PA 15213-3890
------------------------------
Subject: TR: Bayesian Inference on Visual Grammars by NNs that Optimize
From: Eric Mjolsness <mjolsness-eric@CS.YALE.EDU>
Date: Wed, 05 Jun 91 15:50:55 -0400
The following paper is available in the neuroprose archive as
mjolsness.grammar.ps.Z:
Bayesian Inference on Visual Grammars
by Neural Nets that Optimize
Eric Mjolsness
Department of Computer Science
Yale University
New Haven, CT 06520-2158
YALEU/DCS/TR854
May 1991
Abstract:
We exhibit a systematic way to derive neural nets for vision problems.
It involves formulating a vision problem as Bayesian inference or
decision on a comprehensive model of the visual domain given by a
probabilistic {\it grammar}. A key feature of this grammar is the way in
which it eliminates model information, such as object labels, as it
produces an image; correspondance problems and other noise removal tasks
result. The neural nets that arise most directly are generalized
assignment networks. Also there are transformations which naturally
yield improved algorithms such as correlation matching in scale space and
the Frameville neural nets for high-level vision. Deterministic
annealing provides an effective optimization dynamics. The grammatical
method of neural net design allows domain knowledge to enter from all
levels of the grammar, including ``abstract'' levels remote from the
final image data, and may permit new kinds of learning as well.
The paper is 56 pages long.
To get the file from neuroprose:
unix> ftp cheops.cis.ohio-state.edu (or 128.146.8.62)
Name: anonymous
Password: neuron
ftp> cd pub/neuroprose
ftp> binary
ftp> get mjolsness.grammar.ps.Z
ftp> quit
unix> uncompress mjolsness.grammar.ps.Z
unix> lpr mjolsness.grammar.ps (or however you print postscript)
-Eric
------------------------------
End of Neuron Digest [Volume 7 Issue 33]
****************************************