Copy Link
Add to Bookmark
Report
Neuron Digest Volume 06 Number 19
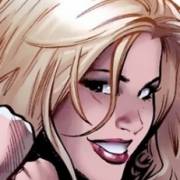
Neuron Digest Friday, 9 Mar 1990 Volume 6 : Issue 19
Today's Topics:
Edelman's Neural Darwinism References
Neurocomputing Hardware and Software
book reference?
N.N. & CW!
Re: N.N. & CW!
Re: AI: Dead or Alive?
Update for 'AI: Dead or Alive'
BackPropagation example in TurboPascal+Question
Send submissions, questions, address maintenance and requests for old issues to
"neuron-request@hplabs.hp.com" or "{any backbone,uunet}!hplabs!neuron-request"
Use "ftp" to get old issues from hplpm.hpl.hp.com (15.255.176.205).
------------------------------------------------------------
Subject: Edelman's Neural Darwinism References
From: Dario Ringach <dario%TECHUNIX.BITNET@CUNYVM.CUNY.EDU>
Date: Tue, 06 Mar 90 08:48:22 +0200
Can anyone briefly explain Edelman's argument, and provide a few
references to his work? Thanks in advance! -Dario.
------------------------------
Subject: Neurocomputing Hardware and Software
From: "D.Sbarbaro" <gnmv73%sun1.eng.glasgow.ac.uk@NSFnet-Relay.AC.UK>
Date: Thu, 08 Mar 90 16:18:46 +0000
To neurocomputing users
We are planning to submit a proposal to get some equipment and software
for a Neural Network proyect, so we would like to have any information or
comments on the following topics:
1. - Companies that produce neurocomputing coprocessor to be used with a
Sun workstation
1.1- Characteristic of the Hardware
1.2- Characteristic of the Software
1.3- Prices
1.4- And perhaps a comparation with other alternatives
2.- Somebody has some experience working with this kind of products?
3.- Software to simulate and implement Neural models on Transputer systems
(We know about the Edinburgh Supercomputing proyect)
Thanks in advance
D. Sbarbaro
Control Group
Dept of Mech Eng
University of Glasgow
Glasgow G12 8QQ
Scotland, UK
JANET: gnmv73@uk.ac.gla.eng.sun1
------------------------------
Subject: book reference?
From: UAP001%DDOHRZ11.BITNET@CUNYVM.CUNY.EDU (Dick Cavonius)
Date: Thu, 08 Mar 90 09:34:08 +0700
There was a recent reference to a new book by Carver Mead: Analog VSLI.
Do you happen to know the publisher.
[[ Editor's Note: Although I've seen the book many times, I don't have a
complete reference. Any help, readers? -PM ]]
------------------------------
Subject: N.N. & CW!
From: Sylvan.Katz@weyr.FIDONET.ORG (Sylvan Katz)
Organization: Benden Weyr, Saskatoon Sk. (306)-382-5746
Date: 20 Feb 90 16:45:39 +0000
Does any one know of any work in progress using neural nets to receive
morse code ? Is this a suitable applications for nn's? Thanks - VE5ZX
Sylvan Katz - via FidoNet node 1:140/22
UUCP: alberta!dvinci!weyr!Sylvan.Katz
Internet: Sylvan.Katz@weyr.FIDONET.ORG
Standard Disclaimers Apply...
------------------------------
Subject: Re: N.N. & CW!
From: comp.vuw.ac.nz!massey!GMoretti (Giovanni Moretti)
Organization: Massey University, Palmerston North, New Zealand
Date: 21 Feb 90 02:23:02 +0000
>> Are neural nets suitable for decoding CW (morse code)?
Sylvan
I won't venture to offer an opinion on the above question (I started
reading about NN four days ago) but refer you to a couple of readable
articles in BYTE August 1989.
The second of these is "Building blocks for Speech" (page 235) which
deals with the use of nets to recognise the "B" and "D" consonants, and
builds up from there. It's a very readable article about the use of nets
on input data that's time varying (there's probably a jargon term for
this :-).
I've also found a very simple backpropagation program written in Turbo
Pascal (about one and a half pages) written by David Parker and included
in Dr Dobbs article "Programming Paradigms" by Michael Swaine DDJ October
1989, p112 (listing starts p146). The source is available from SIMTEL-20
in the DDJMAG (I think) directory.
These articles aren't exactly high-brow stuff but they're a very good
introduction, especially the interview with Dave Parker.
I can mail you the Turbo Pascal source if you like.
Cheers
Giovanni (ZL2BOI)
-----------------------------------------------------------------------------
| GIOVANNI MORETTI, Consultant | EMail: G.Moretti@massey.ac.nz |
|Computer Centre, Massey University | Ph 64 63 69099 x8398, FAX 64 63 505607 |
| Palmerston North, New Zealand | QUITTERS NEVER WIN, WINNERS NEVER QUIT |
-----------------------------------------------------------------------------
------------------------------
Subject: Re: AI: Dead or Alive?
From: cocteau@sun.acs.udel.edu (Daniel J Pirone)
Organization: The Lab Rats
Date: 21 Feb 90 02:48:33 +0000
Howdy,
Just wondering If I could solicite and comments or feedback
on a book called:
_A Connectionist Machince for Genetic Hillclimbing_
David H. Ackley ( @ CMU )
Kluwer Academic Pub.
1987
isbn 0-89838-236-X
thanks in advance
daniel pirone
dept. of CIS
U. of Delaware
------------------------------
Subject: Update for 'AI: Dead or Alive'
From: dietrich@bingvaxu.cc.binghamton.edu (Eric Dietrich)
Organization: SUNY Binghamton, NY
Date: 20 Feb 90 14:33:53 +0000
*** Call for papers and discussion ***
(This is updated from the call for papers sent
the week of Feb. 12, 1990. Clark Glymour from
the Philosophy Department at Carnegie Mellon
University is now one of the target speakers.
His abstract is included.)
------------------------------------------------------------------------
ARTIFICIAL INTELLIGENCE: AN EMERGING SCIENCE OR A DYING ART FORM?
------------------------------------------------------------------------
June 21-23, 1990
A workshop funded by the
Research Foundation of the State University of New York and the
American Association for Artificial Intelligence
What kind of pursuit is artificial intelligence? Is AI the study of
intelligence without regard to its realization, or a branch of psychology
which adds computer modeling to the psychologist's experimental
repertoire, or something else entirely?
There are pragmatic reasons for answering this question. AI
researchers could better utilize time and funds if their research were
based on a deeper understanding of the nature of their endeavor. If, for
example, AI is an engineering discipline, then research strategies based
on the view that AI is a science might waste considerable time and money.
Beyond pragmatic concerns are the nagging doubts that AI, at least as
it is standardly conceived, is merely a way to keep our hands busy while
the neuroscientists and neural net modelers come up to speed. This is
the view that AI is not only NOT a science, but isn't even a very good
engineering discipline, and will one day be abandoned. Since this is a
view that is gaining adherents, it is important to either refute it or
establish its plausibility, and move on.
The workshop will address these questions. Four major papers will be
given; the abstracts are below. Those interested are invited to submit
four copies of an abstract (2 to 7 pages) by May 1, 1990. To help keep
the workshop focused, authors are encouraged to use one of the four
abstracts as a springboard for discussion, or to address a question of
similar scope and content. Examples of such questions are:
1. Is AI a branch of theoretical biology? Should AI abandon its
traditional home in computer science, and seek instead to unify
models of intelligence that apply to all species and even to
species considered as a unit?
2. Is it possible to formulate testable hypotheses about intelligence
which are not solely predictions about human cognitive behavior,
and then test these hypotheses experimentally? If not, then how
important is scientific AI to the study of the human brain and
the study of human psychology?
If authors prefer structure their paper as a commentary, they can request
an extended abstract
The workshop will be kept small -- about 40 participants. The
contribution of each author will be considered for inclusion as a chapter
in a subsequent edited book, or for a paper in the Journal of
Experimental and Theoretical Artificial Intelligence.
Location: SUNY -- Binghamton, Binghamton, New York; June 21-23, 1990
Workshop Chair: Eric Dietrich
Department of Philosophy
SUNY -- Binghamton
Binghamton, New York 13901 (607) 777-2305
e-mail: dietrich@bingvaxu.cc.binghamton.edu
Accomodations: Can be arranged by calling
Holiday Inn SUNY Patti Koval
Vestal Parkway East OR Conference Coordinator
Binghamton, NY 13901 Residential Life
(607) 729-6371 SUNY -- Binghamton
(607) 777-6200
For further information, contact the workshop chair.
-----------------------------------------------------------------------
Accompanying four abstracts:
#1
ARTIFICIAL INTELLIGENCE AND COMPUTATION THEORY
Clark Glymour
Carnegie Mellon University
Abstract
Disciplines exist partly to guarantee their members that certain things
don't have to be thought about. Artificial intelligence has become
sufficiently disciplined that many of its practitioners behave as though
they can ignore large branches of theory.
Sometimes they can. One going enterprise in machine learning is much
like literary interpretation: Some problem area in science or elsewhere
is identified, and one shows that from a reconstruction of the evidence
and the problems of the domain some fairly simple machine algorithm can
solve discovery problems. Nothing is proved or claimed (usually) about
the optimality or limitations of the algorithm. The proceedings of the
Machine Learning conferences are filled with papers of this kind. The
work of Simon's associates provides many examples, and so does much of
the work on learning to plan. One can think of these efforts as sort of
"toy" expert systems work. I have nothing against it; some of this work
is clever, some dull. Some of it is incredibly trivial.
On the other hand, there is a great deal of work in artificial
intelligence that is not just interpretive and that intends to reveal
better ways to do things of importance. In this paper, I argue that
work in non-monotonic inference, explanation-based learning,
simulation studies of the superiority of one or another inference
procedure, as well as an abundance of work in "cogntiive science" that
aims to describe human "computational architecture" all suffer from
failures to take account of the frameworks and results of aspects of
computation theory, especially of learning theory.
#2
Down with Solipsism!
The Challenge to AI from connectionism
J. Hendler
Dept. of Computer Science
Univ. of Maryland
College Park, Md. 20742
hendler@cs.umd.edu
Now, as never before in its short existence as a field, AI is facing a
challenge from the ``cognitivists'' in our ranks. For too long, too
much of the emphasis in AI research has focused on producing systems
which manipulate arbitrary symbol systems to produce other arbitrary
symbols. The growing interest in connectionist models and neural
networks, however, has been focusing on the perceptual level of
cognition. The analogy sometimes used is that AI has been looking at
the parts of the cognitive iceberg that are above water. The bulk of
the iceberg of cognition, however, still underwater, is perception,
emotion, etc. Can any field of ``intelligence'' become a science
while ignoring the bulk of the issue?
In this paper I will try to demonstrate some of the rudiments of
cognition which I believe are growing out of the connectionist
paradigm. Those of us in traditional AI must pay attention to these
results, as well as to cognitive phenomena, which derive from the fact
that intelligent entities are situated in an environment, as opposed
to solipsistic islands unto themselves. This paper is not, however, a
philosphical treatise on symbol grounding. Rather, we report on both
experimental and theoretical research being conducted which is aimed
at exploring the differences in representation learned by
connectionist systems, at understanding how well-documented cognitive
phenomena (such as priming) can be replicated in these models, and at
some types of reasoning (particularly in the area of perceptual
similiarity) which must be accounted for in a model of intelligence.
#3
What is Cognitive Science?
Bill Rapaport
Dept of Computer Science
SUNY Buffalo
Buffalo, NY 14260
rapaport@cs.buffalo.edu
My paper (as I currently conceive it), which will be based on an
encyclopedia article on cognitive science that I am writing, will survey
the nature of cognitive science as a single discipline with a particular
"outlook". Although the encyclopedia article tends to the objective and
netural, my paper for the workshop will take a firm stand on several
issues. I will (1) suggest a distinction between "multidisciplinary" and
"interdisciplinary" research (with examples from one of my own cognitive
science research projects), (2) argue that cognitive science can (or, at
least, should) be considered as a single cohesive discipline that applies
diverse methodologies to a common problem (viz., what is mind/mentality)--
as opposed to most/many other disciplines, which apply a single
methodology to diverse problems, and (3) consider to what extent the
computational view of cognitive science, whether in its weak form (the
computational "metaphor") or its strong form (cognition _is_
computation), leads to a position like that Searle calls "strong AI".
Topics that will briefly be dealt with will include: the Chinese Room
Argument, the intentional stance, and how syntax can yield semantics.
Reference:
Rapaport, William J. (1988), ``Syntactic Semantics: Foundations
of Computational Natural-Language Understanding,'' in J. H. Fetzer (ed.)
Aspects of Artificial Intelligence (Dordrecht, Holland: Kluwer Academic
Publishers): 81-131.
#4
CRYSTALLIZING THEORIES OUT OF KNOWLEDGE SOUP
John F. Sowa
IBM Systems Research
Thornwood, NY 10594
In very large knowledge bases, global consistency is practically
impossible to achieve, yet local consistency is essential for deduction
and problem solving. To preserve local consistency in an environment of
global inconsistency, this paper proposes a two-level structure: an
enormous reservoir of loosely organized encyclopedic knowledge, called
"knowledge soup"; and floating in the soup, much smaller, tightly
organized theories that resemble the typical microworlds of AI. The two
kinds of knowledge require two distinct kinds of reasoning: "abduction"
uses associative search, measures of relevance, and belief revision for
finding appropriate chunks of knowledge in the soup and assembling them
into consistent theories; and "deduction" uses classical theorem-proving
techniques for reasoning within a theory. The resulting two-level
system can attain the goals of nonmonotonic logic, while retaining the
simplicity of classical logic; it can use statistics for dealing with
uncertainty, while preserving the precision of logic in dealing with
hard-edged facts; and it can relate logics with discrete symbols to
models of continuous systems.
------------------------------
Subject: BackPropagation example in TurboPascal+Question
From: GMoretti@massey.ac.nz (Giovanni Moretti)
Organization: Massey University, Palmerston North, New Zealand
Date: 26 Feb 90 01:50:02 +0000
Since I posted an article replying to the use of Neural nets to decode
morse code and indicating that I had an example of a back-propagation
algorithm in Turbo Pascal (tm), I've had several requests for it.
Rather than reply to each individually and since I have a question
relating to it, here it is.
-----------------------------------------------------------------------------
AND THE QUESTION:
In this 2*2 net, the cells are arranged as a 3 * 3 matrix with row 0 being
used as inputs - no problem with that - simplifies programming.
However, why is column zero needed - It's filled with ones (0.95) and used
only in the calculation of weights. If I take out this column (change
"for i:= 0 ..." to "for i:= 1 ..." in the forward and backup updating
procedures, convergence suffers badly - maybe fatally, I didn't wait to
find out.
WHY IS COLUMN ZERO NEEDED - what's it for ???
-----------------------------------------------------------------------------
Anyway here follows the program, and a big thank you to Dave Parker for
something simple for neophytes to cut their teeth on.
If this program is about your level, then get hold of the accompanying
article - it's got some great insights into NNs, and, wait for it - its
easy to understand :-)
{---------program as in Dr Dobbs' follows with slightly altered layout------}
{ I've altered the indentation a little and added a few blank lines and
added the USES statement, I don't believe there are any other differences.
You can get the original from SIMTEL20 in the DDJMAG directory.
Written by Dave Parker - one of the inventors of the back-propagation
algorithm.
From "Programming Paradigms" by Michael Swaine, Doctor Dobbs' Journal,
October 1989, p112, listing starts p146.
}
Program BackPropagationDemo;
uses crt,dos;
Const NumOfRows = 2; (* Number of rows of cells. *)
NumOfCols = 2; (* Number of columns of cells. *)
LearningRate = 0.25; (* Learning rate. *)
Criteria = 0.005; (* Convergence criteria. *)
Zero = 0.05; (* Anything below 0.05 counts as zero. *)
One = 0.95; (* Anything above 0.95 counts as one. *)
Type CellRecord = Record
Output : Real; (* Output of the current cell. *)
Error : Real; (* Error signal for the current cell. *)
Weights: Array[0..NumOfCols] Of Real; (* Weights in cell. *)
End;
Var CellArray : Array[0..NumOfRows,0..NumOfCols] Of CellRecord; (* Cells. *)
Inputs : Array[1..NumOfCols] Of Real; (* Input signals. *)
DesiredOutputs: Array[1..NumOfCols] Of Real; (* Desired output signals. *)
Procedure CalculateInputsAndOutputs( Iteration: Integer );
Var I: Integer;
Begin (* Calculate the inputs and desired outputs for the current iteration. *)
(* The inputs cycle through the 4 patterns (0.05,0.05), (0.95,0.05), *)
(* (0.05,0.95), (0.95,0.95). The corresponding desired outputs are *)
(* (0.05,0.05), (0.05,0.95), (0.05,0.95), (0.95,0.05). The first *)
(* desired output is the logical AND of the inputs, and the second *)
(* desired output is the logical XOR. *)
If (Iteration Mod 2) = 1 Then Inputs[1] := One Else Inputs[1] := Zero;
If (Iteration Mod 4) > 1 Then Inputs[2] := One Else Inputs[2] := Zero;
If (Inputs[1] > 0.5) And (Inputs[2] > 0.5) Then DesiredOutputs[1] := One
Else DesiredOutputs[1] := Zero;
If (Inputs[1] > 0.5) Xor (Inputs[2] > 0.5) Then DesiredOutputs[2] := One
Else DesiredOutputs[2] := Zero;
End;
Procedure UpdateCellOnForwardPass( Row, Column: Integer );
Var J : Integer;
Sum: Real;
Begin (* Calculate the output of the cell at the specified row and column. *)
With CellArray[Row,Column] Do
Begin
Sum := 0.0; (* Clear weighted sum of inputs. *)
For J := 0 To NumOfCols Do (* Form weighted sum of inputs. *)
Sum := Sum + Weights[J]*CellArray[Row-1,J].Output;
Output := 1.0/(1.0+Exp(-Sum)); (* Calculate output of cell. This *)
(* is called a sigmoid function. *)
Error := 0.0; (* Clear error for backward pass. *)
End;
End;
Procedure UpdateCellOnBackwardPass( Row, Column: Integer );
Var J: Integer;
Begin (* Calculate error signals and update weights on the backward pass. *)
Begin
For J := 1 To NumOfCols Do (* Back propagate the error to the cells *)
CellArray[Row-1,J].Error := (* below the current cell. *)
CellArray[Row-1,J].Error+Error*Output*(1.0-Output)*Weights[J];
For J := 0 To NumOfCols Do (* Update the weights in the current cell. *)
Weights[J] :=
Weights[J] +
LearningRate*Error*Output*(1.0-Output)*CellArray[Row-1,J].Output;
End;
End;
Var I, J, K : Integer; (* I loops over rows, J loops over columns,*)
(* and K loops over weights. *)
ConvergedIterations: Integer; (* Network must remain converged for four *)
(* iterations (one for each input pattern).*)
Iteration : Integer; (* Total number of iterations so far. *)
ErrorSquared : Real; (* Error squared for current iteration. *)
Begin
ClrScr; (* Initialize the screen. *)
Writeln('Iteration Inputs Desired Outputs Actual Outputs');
Iteration := 0; (* Start at iteration 0. *)
ConvergedIterations := 0; (* The network hasn't converged yet. *)
For I := 1 To NumOfRows Do (* Initialize the weights to small random numbers.*)
For J := 1 To NumOfCols Do
For K := 0 To NumOfCols Do
CellArray[I,J].Weights[K] := 0.2*Random-0.1;
For I := 0 To NumOfRows Do (* Initialize outputs of dummy constant cells. *)
CellArray[I,0].Output := One;
Repeat
CalculateInputsAndOutputs(Iteration);
For J := 1 To NumOfCols Do (* Copy inputs to dummy input cells. *)
CellArray[0,J].Output := Inputs[J];
For I := 1 To NumOfRows Do (* Propagate inputs forward through network. *)
For J := 1 To NumOfCols Do
UpdateCellOnForwardPass(I,J);
For J := 1 To NumOfCols Do (* Calculate error signals. *)
CellArray[NumOfRows,J].Error :=
DesiredOutputs[J]-CellArray[NumOfRows,J].Output;
For I := NumOfRows Downto 1 Do (* Propagate errors backward through *)
For J := 1 To NumOfCols Do (* network, and update weights. *)
UpdateCellOnBackwardPass(I,J);
ErrorSquared := 0.0; (* Clear error squared. *)
For J := 1 To NumOfCols Do (* Calculate error squared. *)
ErrorSquared := ErrorSquared + Sqr(CellArray[NumOfRows,J].Error);
If ErrorSquared < Criteria Then (* If network has converged, increment *)
ConvergedIterations := ConvergedIterations + 1 (* convergence *)
Else ConvergedIterations := 0; (* count, else clear convergence count. *)
If (Iteration Mod 100) < 4 Then (* Every 100 iterations, write out *)
Begin (* information on the 4 patterns. *)
If (Iteration Mod 100) = 0 Then GotoXY(1,2);
Write(' ',Iteration:5,' '); (* Write iteration number. *)
For J := 1 To NumOfCols Do (* Write out input pattern. *)
Write(Inputs[J]:4:2,' '); Write(' ');
For J := 1 To NumOfCols Do (* Write out desired outputs. *)
Write(DesiredOutputs[J]:4:2,' ');
Write(' ');
For J := 1 To NumOfCols Do (* Write out actual outputs. *)
Write(CellArray[NumOfRows,J].Output:4:2,' ');
Writeln;
End;
Iteration := Iteration + 1; (* Increment iteration count *)
Until (ConvergedIterations = 4) Or (Iteration = 32767);
(* Stop when the network has converged on all 4 input patterns, or when*)
(* we are about to get integer overflow. *)
If ConvergedIterations <> 4 (* Write a final message. *)
Then Writeln('Network didn''t converge')
Else Writeln('Network has converged to within criteria');
End.
{-----------------------------------------------------------------------------}
{Now a version that I've laid out according to my own taste and hacked slightly
so I could better follow what was going on:
NB although the matrix is supposedly only 2 * 2, in reality because it has a
zero origin, it's 3*3.
Row zero is used for the inputs (ie inputs across the top, outputs from
the bottom.
Column zero is filled with ONES (0.95) for reasons I don't yet understand
but appear, for the purposes of evaluating the new weights, to be necessary.
i.e. If you change the weight updating loop to start at 1 instead of 0 (as
it is now, it doesn't seem to converge (or maybe it's just very slow).
The filling of the weight matrix with -99 is just so I could see whether
row and column zero of the weight matrix were altered - they weren't.
The LearningRate must be less than one. If it's 0.25 the program converges
after approximately 12000 iterations, with the rate = 0.95 it takes around
3000 iterations.
}
{ Written by Dave Parker - From "Programming Paradigms" by Michael Swaine
Doctor Dobbs' Journal, October 1989, p112
}
Program BackPropagationDemo;
uses crt,dos;
Const NumOfRows = 2; (* Number of rows of cells. *)
NumOfCols = 2; (* Number of columns of cells. *)
LearningRate = 0.95 {0.25}; (* Learning rate. *)
Criteria = 0.005; (* Convergence criteria. *)
Zero = 0.05; (* Anything below 0.05 counts as zero. *)
One = 0.95; (* Anything above 0.95 counts as one. *)
Type CellRecord = Record
Output : Real; (* Output of the current cell. *)
Error : Real; (* Error signal for the current cell. *)
Weights: Array[0..NumOfCols] Of Real; (* Weights in cell. *)
End;
Var CellArray : Array[0..NumOfRows,0..NumOfCols] Of CellRecord; (* Cells. *)
Inputs : Array[1..NumOfCols] Of Real; (* Input signals. *)
DesiredOutputs: Array[1..NumOfCols] Of Real; (* Desired output signals. *)
Procedure CalculateInputsAndOutputs( Iteration: Integer );
Var I: Integer;
Begin (* Calculate the inputs and desired outputs for the current iteration. *)
(* The inputs cycle through the 4 patterns (0.05,0.05), (0.95,0.05), *)
(* (0.05,0.95), (0.95,0.95). The corresponding desired outputs are *)
(* (0.05,0.05), (0.05,0.95), (0.05,0.95), (0.95,0.05). The first *)
(* desired output is the logical AND of the inputs, and the second *)
(* desired output is the logical XOR. *)
If (Iteration Mod 2) = 1 Then Inputs[1] := One Else Inputs[1] := Zero;
If (Iteration Mod 4) > 1 Then Inputs[2] := One Else Inputs[2] := Zero;
If (Inputs[1] > 0.5) And (Inputs[2] > 0.5) Then DesiredOutputs[1] := One
Else DesiredOutputs[1] := Zero;
If (Inputs[1] > 0.5) Xor (Inputs[2] > 0.5) Then DesiredOutputs[2] := One
Else DesiredOutputs[2] := Zero;
End;
Procedure UpdateCellOnForwardPass( Row, Column: Integer );
Var J : Integer;
Sum: Real;
Begin (* Calculate the output of the cell at the specified row and column. *)
With CellArray[Row,Column] Do
Begin
Sum := 0.0; (* Clear weighted sum of inputs. *)
For J := 0 To NumOfCols Do (* Form weighted sum of inputs. *)
Sum := Sum + Weights[J]*CellArray[Row-1,J].Output;
Output := 1.0/(1.0+Exp(-Sum)); (* Calculate output of cell. This *)
(* is called a sigmoid function. *)
Error := 0.0; (* Clear error for backward pass. *)
End;
End;
Procedure UpdateCellOnBackwardPass( Row, Column: Integer );
Var J: Integer;
Begin (* Calculate error signals and update weights on the backward pass. *)
Begin
For J := 1 To NumOfCols Do (* Back propagate the error to the cells *)
CellArray[Row-1,J].Error := (* below the current cell. *)
CellArray[Row-1,J].Error+Error*Output*(1.0-Output)*Weights[J];
For J := 0 To NumOfCols Do (* Update the weights in the current cell. *)
Weights[J] :=
Weights[J] +
LearningRate*Error*Output*(1.0-Output)*CellArray[Row-1,J].Output;
End;
End;
Var I, J, K : Integer; (* I loops over rows, J loops over columns,*)
(* and K loops over weights. *)
ConvergedIterations: Integer; (* Network must remain converged for four *)
(* iterations (one for each input pattern).*)
Iteration : Integer; (* Total number of iterations so far. *)
ErrorSquared : Real; (* Error squared for current iteration. *)
Begin
ClrScr; (* Initialize the screen. *)
Writeln('Iteration Inputs Desired Outputs Actual Outputs');
Iteration := 0; (* Start at iteration 0. *)
ConvergedIterations := 0; (* The network hasn't converged yet. *)
for i:= 0 to numofrows do {Fill cell weights with odd value}
for j:= 0 to numofcols do {So I can tell which ones are altered}
for k:= 0 to numofcols do
cellarray[i,j].weights[k]:= -99;
For I := 1 To NumOfRows Do (* Initialize the weights to small random numbers.*)
For J := 1 To NumOfCols Do
For K := 0 To NumOfCols Do
CellArray[I,J].Weights[ K] := 0.2*Random-0.1;
For I := 0 To NumOfRows Do (* Initialize outputs of dummy constant cells. *)
CellArray[I,0].Output := One;
Repeat
CalculateInputsAndOutputs(Iteration);
For J := 1 To NumOfCols Do (* Copy inputs to dummy input cells. *)
CellArray[0,J].Output := Inputs[J];
For I := 1 To NumOfRows Do (* Propagate inputs forward through network. *)
For J := 1 To NumOfCols Do
UpdateCellOnForwardPass(I,J);
For J := 1 To NumOfCols Do (* Calculate error signals. *)
CellArray[NumOfRows,J].Error :=
DesiredOutputs[J]-CellArray[NumOfRows,J].Output;
For I := NumOfRows Downto 1 Do (* Propagate errors backward through *)
For J := 1 To NumOfCols Do (* network, and update weights. *)
UpdateCellOnBackwardPass(I,J);
ErrorSquared := 0.0; (* Clear error squared. *)
For J := 1 To NumOfCols Do (* Calculate error squared. *)
ErrorSquared := ErrorSquared + Sqr(CellArray[NumOfRows,J].Error);
If ErrorSquared < Criteria Then (* If network has converged, increment *)
ConvergedIterations := ConvergedIterations + 1 (* convergence *)
Else ConvergedIterations := 0; (* count, else clear convergence count. *)
If (Iteration Mod 100) < 4 Then (* Every 100 iterations, write out *)
Begin (* information on the 4 patterns. *)
If (Iteration Mod 100) = 0 Then GotoXY(1,2);
Write(' ',Iteration:5,' '); (* Write iteration number. *)
For J := 1 To NumOfCols Do (* Write out input pattern. *)
Write(Inputs[J]:4:2,' '); Write(' ');
For J := 1 To NumOfCols Do (* Write out desired outputs. *)
Write(DesiredOutputs[J]:4:2,' ');
Write(' ');
For J := 0 To NumOfCols Do (* Write out actual outputs. *)
Write(CellArray[NumOfRows,J].Output:4:2,' ');
Writeln;
End;
Iteration := Iteration + 1; (* Increment iteration count *)
Until (ConvergedIterations = 4) Or (Iteration = 32767);
(* Stop when the network has converged on all 4 input patterns, or when*)
(* we are about to get integer overflow. *)
Writeln('Weights');
for i:= 0 to numofrows do
begin
for j:= 0 to numofcols do
begin
for k:= 0 to numofcols do
write(' ',cellarray[i,j].weights[k]:4:2);
write(' ');
end;
writeln;
end;
If ConvergedIterations <> 4 (* Write a final message. *)
Then Writeln('Network didn''t converge')
Else Writeln('Network has converged to within criteria');
End.
- --
- -------------------------------------------------------------------------------
| GIOVANNI MORETTI, Consultant | EMail: G.Moretti@massey.ac.nz |
|Computer Centre, Massey University | Ph 64 63 69099 x8398, FAX 64 63 505607 |
| Palmerston North, New Zealand | QUITTERS NEVER WIN, WINNERS NEVER QUIT |
- -------------------------------------------------------------------------------
------------------------------
End of Neuron Digest [Volume 6 Issue 19]
****************************************