Copy Link
Add to Bookmark
Report
Neuron Digest Volume 05 Number 49
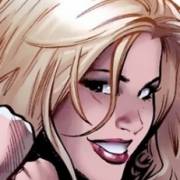
Neuron Digest Monday, 27 Nov 1989 Volume 5 : Issue 49
Today's Topics:
CONFERENCE-ON-PRAGMATICS-IN-AI
News on JNNS(Japanese Neural Network Society)
International Conference Announcement
TR available
MIT Industrial Liaison Program -- Networks and Learning
Connectionist Learning/Representation: Call for Commentators
Tri-Service NN Working Group
Send submissions, questions, address maintenance and requests for old issues to
"neuron-request@hplabs.hp.com" or "{any backbone,uunet}!hplabs!neuron-request"
Use "ftp" to get old issues from hplpm.hpl.hp.com (15.255.176.205).
------------------------------------------------------------
Subject: CONFERENCE-ON-PRAGMATICS-IN-AI
From: lambda!opus!paul@lanl.gov (Paul McKevitt)
Organization: NMSU Computer Science
Date: 14 Nov 89 19:54:05 +0000
PRAGMATICS AI PRAGMATICS AI PRAGMATICS AI PRAGMATICS AI PRAGMATICS AI PRAGMATI
PRAGMATICS AI PRAGMATICS AI PRAGMATICS AI PRAGMATICS AI PRAGMATICS AI PRAGMATI
FROM: Organizing Committee RMCAI-90:
Paul Mc Kevitt Yorick Wilks
Research Scientist Director
CRL CRL
SUBJECT: Please post the following in your Laboratory/Department/Journal:
Cut---------------------------------------------------------------------------
SUBJECT: Please post the following in your Laboratory/Department/Journal:
CALL FOR PAPERS
Pragmatics in Artificial Intelligence
5th Rocky Mountain Conference on Artificial Intelligence (RMCAI-90)
Las Cruces, New Mexico, USA, June 28-30, 1990
PRAGMATICS PROBLEM: The problem of pragmatics in AI is one of developing
theories, models, and implementations of systems that make effective use of
contextual information to solve problems in changing environments.
CONFERENCE GOAL: This conference will provide a forum for researchers from
all subfields of AI to discuss the problem of pragmatics in AI. The
implications that each area has for the others in tackling this problem are
of particular interest.
ACKNOWLEDGEMENTS: In cooperation with: Association for Computing Machinery
(ACM) (pending approval) Special Interest Group in Artificial Intelligence
(SIGART) (pending approval) U S WEST Advanced Technologies and the Rocky
Mountain Society for Artificial Intelligence (RMSAI)
With grants from: Association for Computing Machinery (ACM) Special
Interest Group in Artificial Intelligence (SIGART) U S WEST Advanced
Technologies and the Rocky Mountain Society for Artificial Intelligence
(RMSAI)
THE LAND OF ENCHANTMENT: Las Cruces, lies in THE LAND OF ENCHANTMENT (New
Mexico), USA and is situated in the Rio Grande Corridor with the scenic
Organ Mountains overlooking the city. The city is close to Mexico, Carlsbad
Caverns, and White Sands National Monument. There are a number of Indian
Reservations and Pueblos in the Land Of Enchantment and the cultural and
scenic cities of Taos and Santa Fe lie to the north. New Mexico has an
interesting mixture of Indian, Mexican and Spanish culture. There is quite
a variation of Mexican and New Mexican food to be found here too.
GENERAL INFORMATION: The Rocky Mountain Conference on Artificial
Intelligence is a major regional forum in the USA for scientific exchange
and presentation of AI research.
The conference emphasizes discussion and informal interaction as well as
presentations.
The conference encourages the presentation of completed research, ongoing
research, and preliminary investigations.
Researchers from both within and outside the region are invited to
participate.
Some travel awards will be available for qualified applicants.
FORMAT FOR PAPERS: Submitted papers should be double spaced and no more
than 5 pages long. E-mail versions will not be accepted.
Send 3 copies of your paper to:
Paul Mc Kevitt,
Program Chairperson, RMCAI-90,
Computing Research Laboratory (CRL),
Dept. 3CRL, Box 30001,
New Mexico State University,
Las Cruces, NM 88003-0001, USA.
DEADLINES:
Paper submission: March 1st, 1990
Pre-registration: April 1st, 1990
Notice of acceptance: May 1st, 1990
Final papers due: June 1st, 1990
LOCAL ARRANGEMENTS: Jennifer Griffiths, Local Arrangements Chairperson,
RMCAI-90. (same postal address as above).
INQUIRIES: Inquiries regarding conference brochure and registration form
should be addressed to the Local Arrangements Chairperson.
Inquiries regarding the conference program should be addressed to the
program Chairperson.
Local Arrangements Chairperson: E-mail: INTERNET: rmcai@nmsu.edu
Phone: (+ 1 505)-646-5466
Fax: (+ 1 505)-646-6218.
Program Chairperson: E-mail: INTERNET: paul@nmsu.edu
Phone: (+ 1 505)-646-5109
Fax: (+ 1 505)-646-6218.
TOPICS OF INTEREST: You are invited to submit a research paper addressing
Pragmatics in AI , with any of the following orientations:
Philosophy, Foundations and Methodology
Knowledge Representation
Neural Networks and Connectionism
Genetic Algorithms, Emergent Computation, Nonlinear Systems
Natural Language and Speech Understanding
Problem Solving, Planning, Reasoning
Machine Learning
Vision and Robotics
Applications
INVITED SPEAKERS: The following researchers have agreed to speak at the
conference (a number of others have been invited):
Martin Casdagli, Los Alamos National Laboratory USA
(Dynamical systems, Artificial neural nets, Applications)
Arthur Cater, University College Dublin IRELAND
(Robust Parsing)
James Martin, University of Colorado at Boulder USA
(Metaphor and Context)
Derek Partridge, University of Exeter UK
(Connectionism, Learning)
Philip Stenton, Hewlett Packard UK
(Natural Language Interfaces)
PROGRAM COMMITTEE:
John Barnden, New Mexico State University
(Connectionism, Beliefs, Metaphor processing)
Hans Brunner, U S WEST Advanced Technologies
(Natural language interfaces, Dialogue interfaces)
Martin Casdagli, Los Alamos National Laboratory
(Dynamical systems, Artificial neural nets, Applications)
Mike Coombs, New Mexico State University
(Problem solving, Adaptive systems, Planning)
Thomas Eskridge, Lockheed Missile and Space Co.
(Analogy, Problem solving)
Chris Fields, New Mexico State University
(Neural networks, Nonlinear systems, Applications)
Roger Hartley, New Mexico State University
(Knowledge Representation, Planning, Problem Solving)
Paul Mc Kevitt, New Mexico State University
(Natural language interfaces, Dialogue modeling)
Joe Pfeiffer, New Mexico State University
(Computer Vision, Parallel architectures)
Keith Phillips, University of Colorado at Colorado Springs
(Computer vision, Mathematical modeling)
Yorick Wilks, New Mexico State University
(Natural language processing, Knowledge representation)
Scott Wolff, U S WEST Advanced Technologies
(Intelligent tutoring, User interface design, Cognitive modeling)
REGISTRATION:
Pre-Registration: Professionals $50.00; Students $30.00
(Pre-Registration cutoff date is April 1st 1990)
Registration: Professionals $70.00; Students $50.00
(Copied proof of student status is required).
Registration form (IN BLOCK CAPITALS).
Enclose payment
(ONLY checks in dollars and drawn on a US bank accepted).
Send to the following address (MARKED REGISTRATION):
Jennifer Griffiths,
Local Arrangements Chairperson, RMCAI-90
Computing Research Laboratory
Dept. 3CRL, Box 30001, NMSU
Las Cruces, NM 88003-0001, USA.
Name:_______________________________ E-mail_____________________________ Phone__________________________
Affiliation: ____________________________________________________
Fax: ____________________________________________________
Address: ____________________________________________________
____________________________________________________
____________________________________________________
COUNTRY__________________________________________
Organizing Committee RMCAI-90:
Paul Mc Kevitt Yorick Wilks
Research Scientist Director
CRL CRL
cut----------------------------------------------------------------------
**********************************************
Paul Mc Kevitt,
Computing Research Laboratory,
Dept. 3CRL, Box 30001,
New Mexico State University,
Las Cruces, NM 88003-0001, USA.
505-646-5109/5466
CSNET: paul@nmsu.edu
**********************************************
------------------------------
Subject: News on JNNS(Japanese Neural Network Society)
From: Hideki KAWAHARA <kawahara@av-convex.ntt.jp>
Date: Fri, 17 Nov 89 04:52:41 +0900
- --------------------------
JNNS (Japanese Neural Network Society) have delivered its first
newsletter and started a mailing list
- --------------------------
Japanese Neural Network society, which was founded in July 1989,
have delivered its first newsletter on 14 November 1989. Prof.
Shiro Usui of Toyohasi University of Technology, who is in
charge of editor in chief have also started a mailing list to
encouredge discussions among active researchers in Japan. Prof.
Usui and I would like to introduce the connectionists mailing
list to JNNS's mailing list and to quit delivering to BBORD
eventually.
Electronic communications in Japan is still in its infancy.
JUNET, the largest one, is a volunteer based (mainly UUCP)
network. However, the number of researchers who are accessible
to some electronic communication systems is increasing rapidly.
I look forward to see some Japanese researchers to contribute
this global electronic research community.
Hideki Kawahara
NTT Basic Research Labs. JAPAN.
PS: JNNS President is Prof.Kunihiko Fukushima
JNNS V.P. is Prof.Shun'ichi Amari
If you need more detailes, please e-mail to:
kawahara%siva.ntt.jp@RELAY.CS.NET .
------------------------------
Subject: International Conference Announcement
From: entlyon@frensl61.bitnet (Valerie Roger)
Organization: LIP-IMAG, Ecole Normale Superieure de Lyon
Date: 17 Nov 89 14:15:33 +0000
International Conference on NEURAL NETWORKS :
Biological Computers or Electronic Brains
6/7/8 March 1990
Ecole Normale Superieure de Lyon, FRANCE
The Lyon conference provides, at a regular two-year interval, a
complete outlook of the knowledge, equipment and applications developed
worldwide, in selected domains of the general field "Computer Science and
Life".
Experts in a wide range of scientific and technical sectors will
gather, whose contribution appears quite significant.
Therefore, the Lyon Conference will offer the adequate environment
for stimulating exchanges. The synergy thus created will open up new
perspectives.
Theme of the March 1990 Lyon Conference : "NEURAL NETWORKS,
BIOLOGICAL COMPUTERS OR ELECTRONIC BRAINS".
Following each lecture, time will be devoted to discussions, so
that the applications of this type of networks might exchange information.
Between sessions, participants wishing to further discuss particular
topics, either among themselves or with lecturers, will be provided with
all adequate facilities.
Participants will be provided with the proceedings of the
conference.
For more information leave your postal address at the following
e-mail:
ENTLYON@FRENSL61.BITNET
Opening Session
. Sir John ECCLES (GB) - Noble Prize in Medecine
. Leon COOPER (USA) - Nobel Prize in Physics
. Terrence SEJNOWSKI (USA) -
Formal Neural Networks and Neurobiology
. Yves FREGNAC (F)
. Nicolas FRANCESCHINI (F)
. Walter HEILIGENBERG (USA)
. Moshe ABELES (IL)
. Hanoch GUTFREUND (IL)
. John J. HOPFIELD (USA)
Learning
. Sarah SOLLA (USA)
. Teuvo KOHONEN (SF)
. Giancarlo MAURI (I)
. William PHILIPPS (GB)
. Guy TIBERGHIEN (F)
Applications
. Bernard ANGENIOL (F)
. Jean-Sylvain LIENARD (F)
. Christopher G.ATKESON (USA)
. Alan LAPEDES (USA)
. Jean-Claude PEREZ (F)
. Kazuhlko KAKEHI (J)
Computer Science and Neural Networks
. Marc MEZARD (F)
. Pierre PERETTO (F)
. Robert AZENCOTT (F)
. Colin BLAKEMORE (GB)
. Edmund ROLLS (GB)
Specialized Circuits and Algorithmic Machines
. Michel COSNARD (F)
. Larry JACKEL (USA)
. Michel WEINFELD (F)
. Guy MAZARE (F)
. Jeanny HERAULT (F)
------------------------------
Subject: TR available
From: THEPCAP%SELDC52.BITNET@VMA.CC.CMU.EDU
Date: Fri, 17 Nov 89 19:32:00 +0100
October 1989
LU TP 89-19
"TEACHERS AND CLASSES" WITH NEURAL NETWORKS
Lars Gislen, Carsten Peterson and Bo Soderberg
Department of Theoretical Physics, University of Lund
Solvegatan 14A, S-22362 Lund, Sweden
Submitted to International Journal of Neural Systems
Abstract:
A convenient mapping and an efficient algorithm for solving scheduling
problems within the neural network paradigm is presented. It is based on a
reduced encoding scheme and a mean field annealing prescription, which was
recently successfully applied to TSP.
Most scheduling problems are characterized by a set of hard and soft
constraints. The prime target of this work is the hard constraints. In this
domain the algorithm persistently finds legal solutions for quite difficult
problems. We also make some exploratory investigations by adding soft
constraints with very encouraging results. Our numerical studies cover
problem sizes up to O(5*10^4) degrees of freedom with no parameter
sensitivity.
We stress the importance of adding certain extra terms to the energy
functions which are redundant from the encoding point of view but
beneficial when it comes to ignoring local minima and to stabilizing the
good solutions in the annealing process.
For copies of this report send requests to: THEPCAP@SELDC52. NOTICE: Those
of you who requested our previous report, "A New Way of Mapping
Optimization.... (LU TP 89-1), will automatically receive this one so no
request is necessary.
------------------------------
Subject: MIT Industrial Liaison Program -- Networks and Learning
From: bwk@MBUNIX.MITRE.ORG (Kort)
Organization: The MITRE Corp. Bedford, MA
Date: 17 Nov 89 19:02:59 +0000
MIT Industrial Liaison Program -- Networks and Learning
On Wednesday and Thursday (November 15-16) I attended the MIT Industrial
Liaison Program entitled "Networks and Learning". Here is my report...
Professor Thomaso Poggio of the MIT Department of Brain and Cognitive
Sciences opened the symposium by reviewing the history of advances in the
field. About every 20 years there is an "epidemic" of activity lasting
about 12 years, followed by about 8 years of inactivity. Sixty years ago
there began the Gestalt school in Europe. Forty years ago Cybernetics
emerged in the US. Twenty years ago Perceptrons generated a flurry of
research. Today, Neural Networks represent the latest breakthrough in this
series. [Neural Networks are highly interconnected structures of
relatively simple units, with algebraic connection weights.]
Professor Leon Cooper, Co-Director of the Center for Neural Science at
Brown University, spoke on "Neural Networks in Real-World Applications."
Neural Nets learn from examples. Give them lots of examples of
Input/Output pairs, and they build a smooth mapping from the input space to
the output space. Neural Nets work best when the rules are vague or
unknown. The classical 3-stage neural net makes a good classifier. It can
divide up the input space into arbitrarily shaped regions. At first the
network just divides the space in halves and quarters, using straight line
boundaries ("hyperplanes" for the mathematically minded). Eventually (and
with considerable training) the network can form arbitrarily curved
boundaries to achieve arbitrarily general classification. Given enough of
the critical features upon which to reach a decision, networks have been
able to recognize and categorize diseased hearts from heartbeat patterns.
With a sufficiently rich supply of clues, the accuracy of such classifiers
can approach 100%. Accuracy depends on the sample length of the heartbeat
pattern--a hurried decision is an error-prone decision.
Professor Ron Rivest, Associate Director of MIT's Laboratory for Computer
Science, surveyed "The Theoretical Aspects of Learning and Networks." He
addresses the question, "How do we discover good methods of solution for
the problems we wish to solve?" In studying Neural Networks, he notes
their strengths and characteristics: learning from example, expressiveness,
computational complexity, sample space complexity, learning a mapping. The
fundamental unit of a neural network is a linear adder followed by a
threshold trigger. If the algebraic sum of the input signals exceeds
threshold, the output signal fires. Neural nets need not be constrained to
boolean signals (zero/one), but can handle continuous analog signal levels.
And the threshold trigger can be relaxed to an S-shaped response. Rivest
tells us that any continuous function mapping the unit interval [-1, 1]
into itself can be approximated arbitrarily well with a 3- stage neural
network. (The theorem extends to the Cartesian product: the mapping can be
from an m-fold unit hypercube into an n-fold unit hypercube.) Training the
neural net amounts to finding the coefficients which minimize the error
between the examples and the neural network's approximation. The so-called
Error Backpropagation algorithm is mathematically equivalent to least
squares curve fitting using steepest descent. While this method works, it
can be very slow. In fact, training a 3-stage neural network is an
NP-complete problem--the work increases exponentially with the size of the
network. The classical solution to this dilemma is to decompose the
problem down into smaller subproblems, each solvable by a smaller system.
Open issues in neural network technology include the incorporation of prior
domain knowledge, and the inapplicability of powerful learning methods such
as Socratic-style guided discovery and experimentation. There is a need to
merge the statistical paradigm of neural networks with the more traditional
knowledge representation techniques of analytical and symbolic approaches.
Professor Terry Sejnowski, Director of the Computational Neurobiology
Laboratory at the Salk Institute for Biological Studies, gave a captivating
lecture on "Learning Algorithms in the Brain." Terry, who studies
biological neural networks, has witnessed the successful "reverse
engineering" of several complete systems. The Vestibular Occular Reflex is
the feedforward circuit from the semicircular canals of the inner ear to
the eye muscles which allow us to fixate on a target even as we move and
bob our heads. If you shake your head as you read this sentence, your eyes
can remain fixed on the text. This very old circuit has been around for
hundreds of millions of years, going back to our reptilian ancestors. It
is found in the brain stem, and operates with only a 7-ms delay. (Tracking
a moving target is more complex, requiring a feedback circuit that taps
into the higher cognitive centers.) The Vestibular Occular Reflex appears
to be overdesigned, generating opposing signals which at first appear to
serve no function. Only last week, a veteran researcher finally explained
how the dynamic tension between opposing signals allows the long-term
adaptation to growth of the body and other factors (such as new eyeglasses)
which could otherwise defeat the performance of the reflex. Terry also
described the operation of one of the simplest neurons, found in the
hippocampus, which mediates long-term memory. The Hebbs Synapse is one
that undergoes a physiological change when the neuron happens to fire
during simultaneous occurrence of stimuli representing the input/output
pair of a training sample. After the physiological change, the neuron
becomes permanently sensitized to the input stimulus. The Hebbs Synapse
would seem to be the foundation for superstitious learning.
After a refreshing lunch of cold roast beef and warm conversation,
Professor Thomaso Poggio returned to the podium to speak on "Networks for
Learning: A Vision Application." He began by reviewing the theoretical
result that equates the operation of a 2-layer neural network to linear
regression. To achieve polynomial regression, one needs a 3-layer neural
network. Such a neural net can reconstruct a (smooth) hypersurface from
sparse data. (An example of a non-smooth map would be a telephone
directory which maps names into numbers. No smooth interpolation will
enable you to estimate the telephone number of someone whose name is not in
the directory.) Professor Poggio explored the deep connection between
classical curve fitting and 3-stage neural networks. The architecture of
the neural net corresponds to the so- called HyperBasis Functions which are
fitted to the training data. A particularly simple but convenient basis
function is a gaussian centered around each sample x-value. The
interpolated y-value is then just the average of all the sample y-values
weighted by their gaussian multipliers. In other words, the nearest
neighbors to x are averaged to estimate the output, y(x). For smooth maps,
such a scheme works well.
Dr. Richard Lippmann of the MIT Lincoln Laboratory spoke on "Neural Network
Pattern Classifiers for Speech Recognition." Historically, classification
has progressed through four stages--Probabalistic Classifiers using linear
discriminant functions, Hyperplane Separation using piecewise linear
boundaries, Receptive Field Classification using radial basis functions,
and the new Exemplar Method using multilayer Perceptrons and feature maps.
Surveying and comparing alternate architectures and algorithms for speech
recognition, Dr. Lippmann, reviewed the diversity of techniques, comparing
results, accuracy, speed, and computational resources required. From the
best to the worst, they can differ by orders of magnitude in cost and
performance.
Professor Michael Jordan of MIT's Department of Brain and Cognitive Science
spoke on "Adaptive Networks for Motor Control and Robotics." There has
been much progress in this field over the last five years, but neural nets
do not represent a revolutionary breakthrough. The "Inverse Problem" in
control theory is classical: find the control sequence which will drive the
system from the current state to the goal state. It is well known from
Cybernetics that the controller must compute (directly or recursively) an
inverse model of the forward system. This is equivalent to the problem of
diagnosing cause from effect. The classical solution is to build a model
of the forward system and let the controller learn the inverse through
unsupervised learning (playing with the model). The learning proceeds
incrementally, corresponding to backpropagation or gradient descent based
on the transposed Jacobian (first derivative). This is essentially how
humans learn to fly and drive using simulators.
Danny Hillis, Founding Scientist of Thinking Machines Corporation, captured
the audience with a spellbinding talk on "Intelligence as an Emergent
Phenomenon." Danny began with a survey of computational problems
well-suited to massively parallel architectures--matrix algebra and
parallel search. He uses the biological metaphor of evolution as his model
for massively parallel computation and search. Since the evolution of
intelligence is not studied as much as the engineering approach (divide and
conquer) or the biological approach (reverse engineer nature's best ideas),
Danny chose to apply his connection machine to the exploration of
evolutionary processes. He invented a mathematical organism (called a
"ramp") which seeks to evolve and perfect itself. A population cloud of
these ramps inhabits his connection machine, mutating, evolving, and
competing for survival of the fittest. Danny's color videos show the
evolution of the species under different circumstances. He found that the
steady state did not generally lead to a 100 percent population of perfect
ramps. Rather 2 or more immiscible populations of suboptimal ramps formed
pockets with seething boundaries. He then introduced a species of
parasites which attacked ramps at their weakest points, so that stable
populations would eventually succumb to a destructive epidemic. The
parasites did not clear the way for the emergence of perfect and immune
ramps. Rather, the populations cycled through a roiling rise and fall of
suboptimal ramps, still sequestered into camps of Gog and Magog. The eerie
resemblance to modern geopolitics and classical mythology was palpable and
profound.
Professor John Wyatt of the MIT Department of Electrical Engineering and
Computer Science closed the first day's program with a talk on "Analog VLSI
Hardware for Early Vision: Parallel Distributed Computation without
Learning." Professor Wyatt's students are building analog devices that can
be stimulated by focusing a scene image onto the surface of a chip. His
devices for image processing use low precision (about 8 bits) analog
processing based on the inherent bulk properties of silicon. His goal is
to produce chips costing $4.95. One such chip can find the fixed point
when the scene is zoomed. (Say you are approaching the back of a slow
moving truck. As the back of the truck looms larger in your field of view,
the fixed point in the scene corresponds to the point of impact if you fail
to slow down.) Identification of the coordinates of the fixed point and
the estimated time to impact are the output of this chip. Charged-coupled
devices and other technologies are being transformed into such image
processing devices as stereo depth estimation, image smoothing and
segmentation, and motion vision.
The second day of the symposium focused on the Japanese, European, and
American perspectives for the development and application of neural nets.
Professor Shun-ichi Amari of the Department of Mathematical Engineering and
Information Physics at the University of Tokyo explored the mathematical
theory of neural nets. Whereas conventional computers operate on symbols
using programmed sequential logic, neural nets correspond more to intuitive
styles of information processing--pattern recognition, dynamic parallel
processing, and learning. Professor Amari explored neural network
operation in terms of mathematical mapping theory and fixed points. Here,
the fixed points represent the set of weights corresponding to the stable
state after extensive training.
Dr. Wolfram Buttner of Siemens Corporate Research and Development discussed
several initiatives in Europe to develop early commercial applications of
neural net technology. Workpiece recognition in the robotic factory and
classification of stimuli into categories are recurring themes here. There
is also interest in unsupervised learning (playing with models or exploring
complex environments), decision support systems (modeling, prediction,
diagnosis, scenario analysis, optimal decision making with imperfect
information) and computer languages for neural network architectures. Dr.
Buttner described NeuroPascal, an extension to Pascal for parallel
neurocomputing architectures.
Dr. Scott Kirkpatrick, Manager of Workstation Design at IBM's Thomas J.
Watson Research Center, explored numerous potential applications of neural
nets as information processing elements. They can be viewed as filters,
transformers, classifiers, and predictors. Commercial applications include
routine processing of high-volume data streams such as credit-checking and
programmed arbitrage trading. They are also well-suited to adaptive
equalization, echo cancellation, and other signal processing tasks. SAIC
is using them in its automated luggage inspection system to recognize the
telltale signs of suspect contents of checked luggage. Neurogammon 1.0,
which took two years to build, plays a mean game of backgammon, beating all
other machines and giving world class humans a run for their money. Hard
problems for neural nets include 3D object recognition in complex scenes,
natural language understanding, and "database mining" (theory
construction). Today's commercially viable applications of neural nets
could only support about 200 people. It will be many years before
neurocomputing becomes a profitable industry.
Marvin Minsky, MIT's Donner Professor of Science, gave an entertaining talk
on "Future Models". The human brain has over 400 specialized
architectures, and is equivalent in capacity to about 200 Connection
Machines (Model CM-2). There are about 2000 data buses interconnecting the
various departments of the brain. As one moves up the hierarchy of
information processing, one begins at Sensory-Motor and advances through
Concrete Thinking, Operational Thinking, "Other Stages", and arrives at
Formal Thinking as the highest cognitive stage. A human subject matter
expert who is a world class master in his field has about 20-50 thousand
discrete "chunks" of knowledge. Among the computational paradigms found in
the brain, there are Space Frames (for visual information), Script Frames
(for stories), Trans- Frames (for mapping between frames), K-Lines
(explanation elided), Semantic Networks (for vocabulary and ideas), Trees
(for hierarchical and taxonomical knowledge), and Rule-Based Systems (for
bureaucrats). Minsky's theory is summarized in his latest book, Society of
Mind. Results with neural networks solving "interesting" problems such as
playing backgammon or doing freshman calculus reveal that we don't always
know which problems are hard. It appears that a problem is hard until
somebody shows an easy way to solve it. After that, it's deemed trivial.
As to intelligence, Minsky says that humans are good at what humans do. He
says, "A frog is very good at catching flies. And you're not."
The afternoon panel discussion, led by Patrick Winston, provided the
speakers and audience another chance to visit and revisit topics of
interest. That commercial neural networks are not solving profoundly deep
and important problems was a source of dismay to some, who thought that we
had enough programmed trading and credit checking going on already, and we
don't need more robots turning down our loans and sending the stock markets
into instability.
The deeper significance of the symposium is that research in neural
networks is stimulating the field of brain and cognitive science and giving
us new insights into who we are, how we came to be that way, and where we
can go, if we use our higher cognitive functions to best advantage.
- --Barry Kort
------------------------------
Subject: Connectionist Learning/Representation: Call for Commentators
From: srh@wind.bellcore.com (Stevan R Harnad)
Organization: Bellcore, Morristown, NJ
Date: 24 Nov 89 23:33:36 +0000
Below is the abstract of a forthcoming target article to appear in
Behavioral and Brain Sciences (BBS), an international,
interdisciplinary journal that provides Open Peer Commentary on important
and controversial current research in the biobehavioral and cognitive
sciences. Commentators must be current BBS Associates or nominated by a
current BBS Associate. To be considered as a commentator on this article,
to suggest other appropriate commentators, or for information about how
to become a BBS Associate, please send email to:
harnad@confidence.princeton.edu harnad@pucc.bitnet or write to:
BBS, 20 Nassau Street, #240, Princeton NJ 08542 [tel: 609-921-7771]
____________________________________________________________________
WHAT CONNECTIONIST MODELS LEARN:
LEARNING AND REPRESENTATION IN CONNECTIONIST NETWORKS
Stephen J Hanson David J Burr
Learning and Knowledge Artificial Intelligence and
Acquisition Group Communications Research Group
Siemens Research Center Bellcore
Princeton NJ 08540 Morristown NJ 07960
Connectionist models provide a promising alternative to the traditional
computational approach that has for several decades dominated cognitive
science and artificial intelligence, although the nature of connectionist
models and their relation to symbol processing remains controversial.
Connectionist models can be characterized by three general computational
features: distinct layers of interconnected units, recursive rules for
updating the strengths of the connections during learning, and "simple"
homogeneous computing elements. Using just these three features one can
construct surprisingly elegant and powerful models of memory, perception,
motor control, categorization and reasoning. What makes the connectionist
approach unique is not its variety of representational possibilities
(including "distributed representations") or its departure from explicit
rule-based models, or even its preoccupation with the brain metaphor.
Rather, it is that connectionist models can be used to explore
systematically the complex interaction between learning and representation,
as we try to demonstrate through the analysis of several large networks.
Stevan Harnad INTERNET: harnad@confidence.princeton.edu
srh@flash.bellcore.com harnad@elbereth.rutgers.edu harnad@princeton.uucp
BITNET: harnad1@umass.bitnet harnad@pucc.bitnet (609)-921-7771
------------------------------
Subject: Tri-Service NN Working Group
From: Waters <twaters@nswc-wo.ARPA>
Date: Mon, 27 Nov 89 12:54:12 -0500
Subject: Tri-Service NN Working Group
From: twaters@nswc-wo.arpa
Organization: Naval Surface Warfare Center
Date: 27 Nov 89
CLASSIFIED MEETING OF TRI-SERVICE NEURAL NETWORKS WORKING GROUP
1. The Tri-Service Neural Networks Working Group Meeting will be
held at the Naval Surface Warfare Center (NSWC), Silver Spring,
Maryland on 19 January 1990. Please be aware that this meeting
overlaps with the last day of the International Joint Conference
on Neural Net (IJCNN) Meeting in Washington, DC, 15 -19 January,
1990.
2. The meeting will be classified SECRET. Please send your
clearance to:
Commander
Naval Surface Warfare Center
Attn: Visitor Control (Code X11)
10901 New Hampshire Avenue
Silver Spring, Maryland 20903-5000
FAX #: (202) 394-5807
Verifying #: (202) 394-1471
The point of contact is Dr. Ali Farsaie, NSWC, G42, (202) 394-
3850.
3. This will be an informal discussion at the working group level
within the Tri-Service community on the application of neural
networks technology to DOD problems. I would encourage
representatives from every DOD Research and Development Laboratory
to participate and present their progress in neural network
projects.
4. To help in preparing the agenda for this meeting, I would like
those who are interested in presenting their progress to return
enclosure (1) no later than 18 December 1989.
5. If there are any questions, please contact Dr. Ali Farsaie at
Autovon 290-3850 or (202) 394-3850.
Enclosure (1):
SPEAKER TOPIC INFORMATION FORM
TRI-SERVICE NEURAL NETWORKS WORKING GROUP MEETING
NAVAL SURFACE WARFARE CENTER/WO
SILVER SPRING, MARYLAND
19 JANUARY 1990
1. TITLE:
2. SPEAKER'S NAME:
3. TELEPHONE NUMBER:
4. MAILING ADDRESS:
5. ABSTRACT: (one or two paragraphs)
Mailing Address:
Dr. Ali Farsaie
Code G42
Naval Surface Warfare Center
Silver Spring, Maryland 20903-5000
FAX # (202) 394-4651
------------------------------
End of Neurons Digest
*********************