Copy Link
Add to Bookmark
Report
Neuron Digest Volume 06 Number 12
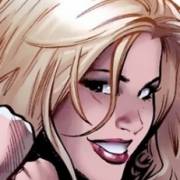
Neuron Digest Sunday, 11 Feb 1990 Volume 6 : Issue 12
Today's Topics:
New list and contact point for: neural networks and transputers
Call for Papers on Combined symbolic and numeric processing
Preprint Available (excerpts are given below)
Summer Course in Computational Neurobiology
Call for Papers - Progess in Neural Nets
Conference on Intelligent Control
Send submissions, questions, address maintenance and requests for old issues to
"neuron-request@hplabs.hp.com" or "{any backbone,uunet}!hplabs!neuron-request"
Use "ftp" to get old issues from hplpm.hpl.hp.com (15.255.176.205).
------------------------------------------------------------
Subject: New list and contact point for: neural networks and transputers
From: INMOS/ST Neurocomputer Lab <DUDZIAK@isnet.inmos.COM>
Date: Fri, 09 Feb 90 13:41:28 -0700
Two announcements:
(1) Proposal for New List / Request for Moderator
I would like to establish a new list focusing on transputer-based
neurocomputing research and applications. From my perspective there is
enough interest and activity in this area to merit a list on its own.
However, while I am perfectly willing to moderate and coordinate this
list, I am not physically located at a network site and have only a 2400
baud link to the machine which serves as the INMOS USA network site. Is
there anyone who would like to moderate this list? The situation will
hopefully change within a year, but with the volume of activity in this
area I would like to get things moving.
This is my description of the proposed news list NEURTRAN:
Purpose: neurtran is focused upon the development of neural network
architectures using transputers and transputer-based hardware platforms,
including ASIC transputers and transputer modules (trams). Discussion is
unmoderated and open to all facets of discussion. Submissions should be
on a non-proprietary nature. Welcome topics for submission include:
- on-going R&D, including applications and products
- parallelization of neural algorithms
- integrating neural and symbolic AI processes/programs
- transputers and specialized neural chips
- ASIC transputer designs for neural applications
Interested parties please respond to dudziak@isnet.inmos.com or use
voice/fax as indicated below.
(2) Establishment of information clearinghouse on same subject, via my
personal email address (dudziak@isnet.inmos.com).
For those wanting more individualized discussion/problem-solving on
these topics, and for all other interested parties until the above mail
list is in place, anyone should feel free to make contact with me through
my personal address. In my present capacity of directing neurocomputing
R&D and application efforts within INMOS (maker of the transputer,
surprise, surprise), I have been running an informal 'clearinghouse' of
sorts for some time. I realize that while a mail list will serve nicely
for a lot of info exchange, there seems to be a need for more personal
tech/theory dialogue. I would like people 'out there' to know that they
can call on me and through me reach other people, resources, & references
that I know about pertaining to research on these topics.
Martin J. Dudziak
Voice: (301) 995-6964
Fax: (301) 290-7047
Please note: I will be out of town from 2/10 until 2/18
N.B. This message is also being sent to the following news lists:
NEURON@HPLABS.HP.COM
TRANSPUTER@TCGOULD.TN.CORNELL.EDU
------------------------------
Subject: Call for Papers on Combined symbolic and numeric processing
From: P.Refenes@Cs.Ucl.AC.UK
Date: Fri, 09 Feb 90 15:12:42 +0000
The Knowledge Engineering Review is planning a special issue on "Combined
Symbolic & Numeric Processing Systems". Is there anyone out there with an
interest in "theories and techniques for mixed symbolic/numeric
processing systems" who is willing to write a comprehensive review paper?
==========================================================
The Knowledge Engineering Review
Published by Cambridge University Press
Special Issue on:
Combined Symbolic & numeric processing Systems
NOTES FOR CONTRIBUTORS
Editor:
Apostolos N. REFENES
Department of Computer Science BITNET: refenes%uk.ac.ucl.cs@ukacrl
University College London UUCP: {...mcvax!ukc!}ucl.cs!refenes
Gower Street, London, WC 1 6BT, UK. ARPANet: refenes@cs.ucl.ac.uk
THE KNOWLEDGE ENGINEERING REVIEW - SPECIAL ISSUE ON
Inferences and Algorithms: co-operating in computation
or
(Symbols and Numbers: co-operating in computation)
THEME
The theme of this special issue of KER is to review
developments in the subject of integrated symbolic and
numeric computation. The subject area of combined
symbolic and numeric computation is a prominent
emerging subject. In Europe, ESPRIT is already funding
a $15m project to investigate the integration of
symbolic and numeric computation and is planning to
issue a further call for a $20m type A project in
Autumn this year. In the USA, various funding agencies,
like the DoD and NSF, have been heavily involved in
supporting research into the integration of symbolic
and numeric computing systems over the last few years.
Algorithmic (or numeric) computational methods are
mostly used for low-level, data driven computations to
achieve problem solutions by exhaustive evaluation, and
are based on static, hardwired decision making
procedures. The statisticity and regularity of the
knowledge, data, and control structures that are
employed by such algorithmic methods permits their
efficient mapping and execution on conventional
supercomputers. However, the scale of the computation
increases often non-linearly with the problem size, and
the strength of the data inter-dependencies.
Symbolic (or inference) computational methods have the
capability to drastically reduce the required
computations by using high-level, model-driven
knowledge, and hypothesis-and-test techniques about the
application domain. However, the irregularity,
uncertainty, and dynamicity of the knowledge, data, and
control structures that are employed by symbolic
methods presents a major obstacle to their efficient
mapping and execution on conventional parallel
computers.
This has led many researchers to propose the
development of integrated numeric and symbolic
computation systems, which have the potential to
achieve optimal solutions for large classes of
problems, in which algorithmic and symbolic component
are engaged in close co-operation. The need for such
interaction is particularly obvious in such
applications as image understanding, speech
recognition, weather forecasting, financial
forecasting, the solution of partial differential
equations etc. In these applications, numeric software
components are tightly coupled with their symbolic
counterparts, which in turn, have the power to feed-
back adjustable algorithm parameters, and hence,
support a "hypothesis-and-test" capability required to
validate the numeric data.
It is this application domain that provided the
motivation for developing theoretical frameworks,
techniques, programming languages, and computer
architectures to efficiently support both symbolic and
numeric computation. The special issue of The
Knowledge Engineering Review KER aims to provide a
comprehensive and timely review of the state of the art
in integrated symbolic and numeric knowledge
engineering systems. The special issue will cover the
topics outlined in the next section.
TOPICS
There are four important topics that are related to the
subject area of integrated symbolic and numeric
computation. This special issue will have one
comprehensive review paper in each of the topics, and a
general overview article (or editorial) to link them
together.
1. Theory and Techniques
Traditional theoretical frameworks for decision
making are are generally considered to be too
restrictive for developing practical knowledge
based systems. The principal set of restrictions is
that classical algorithmic decision theories and
techniques do not address the need to reason about
the decision process itself. Classical techniques
cannot reflect on what the decision is, what the
options are, what methods should be (or have been)
used in making decision and so forth. Approaches
that accommodate numerical methods but extend them
with non-monotonic inference techniques are
described extensively in the literature e.g
[Coguen, Eberbach, Vanneschi, Fox et al, etc]. What
is needed is an in-depth analysis, taxonomy and
evaluation of these techniques. This review of the
theoretical approaches and background into
integrated symbolic and numeric computation should
be highly valuable to those involved in symbolic,
numeric, and integrated symbolic plus numeric
computation.
2. Applications
Here there would be a review of the applications
which provide the stimulus, and demonstrate
techniques for integrating symbolic and numeric
computing components. Effectiveness considerations
and performance gains should also be included where
appropriate. Model applications may include: image
understanding, weather forecasting, financial
forecasting, expert systems for PDE solving,
simulation, real-time process control,etc. The
review article should expose the common ground that
these applications share, the potential improvement
in reasoning and computation efficiency, the
requirements that they impose on the theoretical
frameworks, programming languages, and computer
architecture.
3. Programming Languages
This would be a review of the programming languages
which provide the means for integrating symbolic
and numeric computations. The article(s) should
describe the competing approaches, i.e. integration
through homogenisation, and integration through
interfacing heterogeneous systems. Language design
issues, features for parallelism, etc. Possible
languages that might be considered are: Spinlog,
Orient84K, LOOPS , Cornerstone, Solve, Parle, etc.
A comparative analysis of the involved languages
should be included.
4. Computer Architecture
This review should give a comprehensive review of
the novel computer architectures that are involved,
their basic operating principles, their internal
structure, a comparative analysis, etc. Possible
architectures that might be considered are:
PADMAVATI, SPRINT, ...
DEADLINES
March 15th - Extended Abstract.
April 30th - Full Manuscript.
------------------------------
Subject: Preprint Available (excerpts are given below)
From: jannaron@midway.ece.scarolina.edu
Date: Fri, 09 Feb 90 11:19:47 -0500
EXTENDED CONJUNCTOID THEORY AND IMPLEMENTATION: A
GENERAL MODEL FOR MACHINE COGNITION BASED ON CATEGORICAL DATA
(a preliminary report)
Robert J. Jannarone, Keping Ma, Kai Yu, and John W. Gorman
University of South Carolina
Abstract
An extended and completely formulated conjunctoid
framework is presented for a variety of noniterative and
separable learning and performance procedures that can be
applied in supervised and unsupervised settings. Several
complete examples are given based on realistic application
settings, along with two examples from learning theory for
describing key concepts. Related derivations and simulation
results are provided as well.
KEY WORDS: back propagation, conditioning, conjunc-
toids, control, machine cognition, machine learning,
neural networks, parallel distributed processing,
supervised and unsupervised learning, visual pattern
recognition, voice recognition and synthesis.
Introduction
[Instead of specifying design constraints (such as only
only linear associations among inputs and outputs) at the
outset and then learning within such constraints, back-
propagation carries the added potential of learning design
constraints, that is learning the actual MODEL as well. Such
devices are thus very powerful because then have the flexi-
bility to learn in all possible settings in a way that is
very easy to implement.]
The devices to be described here are much less ambi-
tious. Although they offer a broad range of design blue-
prints for machine learning and performance, they have the
distinct disadvantage of requiring that one such blueprint
be chosen at the outset. On the positive side, each such
blueprint can be made flexible enough to fit the needs of
many specific settings. Also, by carefully selecting the
blueprints optimal learning and performance can be achieved.
This article focuses on neurocomputing modules called
conjunctoids. Although conjunctoids were introduced in an
earlier paper, some key features had not yet been developed
including: sufficiently general extended versions to cover a
variety of supervised and unsupervised learning and perfor-
mance settings; completely specified learning and perfor-
mance algorithms that are both noniterative, hence fast, and
separable, hence easy to implement on parallel computers;
and a representative sample of possible applications.
The purpose of this article is to introduce an extended
conjunctoid cognitive theory, some new real-time conjunctoid
learning and performance algorithms, and some related appli-
cations. Although the material includes new results to sup-
plement earlier reports, it will be presented with an eye
toward demonstrating simple solutions to practical problems.
Toward that end major conjunctoid features will first be
introduced through a variety of examples after which general
implementation formulas will be provided. Conjunctoids will
next be contrasted with more "traditional" neural networks
and other related work will be reviewed. Finally, technical
details will be provided and key results will be summarized.
Key Concepts
INSTRUMENTAL CONDITIONING. Instrumental conditioning
theory is concerned with learning and performance based on
stimulus/response pairs that are either "positively rein-
forced" or "negatively reinforced". . . .
CLASSICAL CONDITIONING . . .
SIMILARITY-BASED REASONING. A pattern recognition
example will be used next to illustrate different modes of
learning and performance in pattern recognition settings
along with different modes of similarity-based reasoning.
[. . .]
FULL-BLOWN CONJUNCTOIDS FOR SMALL SCALE PROBLEMS. Now
that basic psychological, statistical, and neurocomputing
ideas have been introduced some complete conjunctoid exam-
ples will be given. The first example will feature a full-
blown conjunctoid, that is one having the most possible
parameters for a given [number of neurons, K] . . .
To sum up the third example, a small scale pattern com-
pletion problem has been used to show how full-blown con-
junctoids can be used to fill in missing information, detect
and correct errors, and provide similarity measures for
unsupervised learning. The model, estimation, similarity,
and performance formulas have also been completely specified
. . . so that readers can formulate
full-blown conjunctoids for similar problems. . . .
The remaining examples require alternatives to full-
blown conjunctoids because they involve large [K values].
The alternative devices and/or example settings will be
called MEDIUM SCALE if the number of required parameters is
of fixed degree in K and LARGE SCALE if the number of
required parameters is fixed for any K value.
PATTERN COMPLETION AND RECOGNITION IN MEDIUM SCALE
SETTINGS: NEAREST-NEIGHBOR CONJUNCTOIDS IN THE ONE-DIMENSION-
AL CASE. . . .
To sum up the third example, a completely specified
conjunctoid has been described for learning and performance
in nonstationary, one-dimensional nearest neighbor settings.
The device includes learning and performance algorithms that
can be simply and quickly implemented. Of more practical
importance, these algorithms may be extended to more useful
stationary extensions for large scale problems, as will be
seen in the final examples . . .
CATEGORICAL DECISION MAKING IN MEDIUM SCALE SETTINGS:
N-TH DEGREE CONJUNCTOIDS. The devices to be described next
might be used in applications ranging from pain treatment
diagnosis to chemical tank control. . . .
To sum up the fourth example, N-th degree conjunctoids
can be used in a variety of medium scale settings where dif-
ferent categorical variables have distinct meanings. They
can be used as (a) expert systems that are taught simply by
being programmed how to perform under a variety of condi-
tions, and/or (b) learning devices that modify their perfor-
mance rules as a function of learning trial variables and
"reinforcements"--reinforcement in this case appears in the
form of subjective ratings and/or objective measures that
are translated into learning/unlearning weight values.
Finally, as with all other conjunctoids, N-th order devices
can be programmed to learn and perform quickly even when the
involved coefficients number in the thousands.
PATTERN COMPLETION AND RECOGNITION IN LARGE SCALE
SETTINGS: . . .
final examples involve settings where K can number in the
thousands. The first example may be applied in settings
where a device must learn and perform at any point on a long
chain, as in certain speech synthesis and modem error
detection/correction applications. The second example may
be applied in settings where a device must learn and perform
at any point on a dense multidimensional grid, as in the
airplane pattern recognition and completion case that was
introduced earlier. In both examples the key assumptions
leading to viable conjunctoids are that: (a) associations
among variables are spatially LOCALIZED, that is associa-
tions among all [neurons] can be explained by [neurons] that
are near each other, and (b) the variables are spatially
STATIONARY, that is the relation between any two pairs of
variables that are equidistant is the same no matter where
they are located (in probability terms both examples are
based on stationary Markov processes).
CONJUNCTOID MODEL SYNOPSIS. In this section all neces-
sary formulas for implementation will be presented but not
derived--all derivations will be given later. . . .
CONJUNCTOID OPTIMALITY CHARACTERISTICS. Only a brief
outline of performance that may be expected of ...
conjunctoids will be given here. A more detailed discussion
will be given later. Conjunctoids are probability models
that belong in the so-called exponential family (JYT, Leh-
mann, 1983). Exponential family membership, in turn, means
that slow learning and performance algorithms are guaranteed
to be both consistent and efficient (in cases where parame-
ters for a given conjunctoid exist that can explain its
input data). Consistent performance means that after a suf-
ficiently large number of learning trials conjunctoid per-
formance based on estimated parameters becomes as good as if
the true parameters were used. Efficient performance means
that after any given number of trials expected conjunctoid
performance will be as good as that from any other possible
learning algorithm. Besides being efficient and consistent,
conjunctoids that use slow learning algorithms use search
procedures based on convex likelihood functions, hence they
will always converge to global optimum (so-called maximum
likelihood) solutions.
Such optimality properties do not hold for conjunctoids
that employ fast learning and performance algorithms
(although they do hold for faster versions in some specific
instances). However, since fast conjunctoid procedures are
based on reasonable approximations to their optimal counter-
parts, it seems likely that fast algorithm conjunctoid per-
formance will typically be about the same as optimal perfor-
mance. Also, simulation results that are given below for
some specific instances provide evidence toward that end
.
.
.
Since the complete paper includes 11 figures and over 50 formulas, sending
it by electronic mail would be awkward. For hard copies please contact
Robert Jannarone
Center for Machine Intelligence
Electrical and Computer Eng. Dept.
Univ. of South Carolina
Columbia SC, 29208
(803) 777-7930
jannaron@midway.ece.scarolina.edu
------------------------------
Subject: Summer Course in Computational Neurobiology
From: Jim Bower <jbower@smaug.cns.caltech.edu>
Date: Fri, 09 Feb 90 11:28:47 -0800
Summer Course Announcement
Methods in Computational Neurobiology
August 5th - September 1st
Marine Biological Laboratory
Woods Hole, MA
This course is for advanced graduate students and postdoctoral fellows in
neurobiology, physics, electrical engineering, computer science and
psychology with an interest in "Computational Neuroscience." A
background in programming (preferably in C or PASCAL) is highly desirable
and basic knowledge of neurobiology is required. Limited to 20 students.
This four-week course presents the basic techniques necessary to study
single cells and neural networks from a computational point of view,
emphasizing their possible function in information processing. The aim
is to enable participants to simulate the functional properties of their
particular system of study and to appreciate the advantages and pitfalls
of this approach to understanding the nervous system.
The first section of the course focuses on simulating the electrical
properties of single neurons (compartmental models, active currents,
interactions between synapses, calcium dynamics). The second section
deals with the numerical and graphical techniques necessary for modeling
biological neuronal networks. Examples are drawn from the invertebrate
and vertebrate literature (visual system of the fly, learning in
Hermissenda, mammalian olfactory and visual cortex). In the final
section, connectionist neural networks relevant to perception and
learning in the mammalian cortex, as well as network learning algorithms
will be analyzed and discussed from a neurobiological point of view.
The course includes lectures each morning and a computer laboratory in
the afternoons and evenings. The laboratory section is organized around
GENESIS, the Neuronal Network simulator developed at the California
Institute of Technology, running on 20 state-of-the-art, single-user,
graphic color workstations. Students initially work with GENESIS-based
tutorials and then are expected to work on a simulation project of their
own choosing.
Co-Directors:
James M. Bower and Christof Koch, Computation and Neural Systems
Program, California Institute of Technology
1990 summer faculty:
Ken Miller UCSF
Paul Adams Stony Brook
Idan Segev Jerusalem
David Rumelhart Stanford
John Rinzel NIH
Richard Andersen MIT
David Van Essen Caltech
Kevin Martin Oxford
Al Selverston UCSD
Nancy Kopell Boston U.
Avis Cohen Cornell
Rudolfo Llinas NYU
Tom Brown* Yale
Norberto Grzywacz* MIT
Terry Sejnowski UCSD/Salk
Ted Adelson MIT
*tentative
Application deadline: May 15, 1990
Applications are evaluated by an admissions committee and individuals are
notified of acceptance or non-acceptance by June 1. Tuition: $1,000
(includes room & board). Financial aid is available to qualified
applicants.
For further information contact:
Admissions Coordinator
Marine Biological Laboratory
Woods Hole, MA 02543
(508) 548-3705, ext. 216
------------------------------
Subject: Call for Papers - Progess in Neural Nets
From: <OOMIDVAR%UDCVAX.BITNET@CORNELLC.cit.cornell.edu>
Date: Fri, 09 Feb 90 15:49:00 -0400
PROGRESS IN NEURAL NETWORKS
CALL FOR PAPER
This is a call for papers for the Third Volume of the Progress In
Neural Networks Series. The first two volumes will be available this
year. These volumes contain original contributions from leading national
and international research institutions. If you like to receive more
information please contact the editor or Ablex Publishing Corporation.
This series will review the state-of-the-art research in neural
networks, natural and synthetic. Contributions from leading researchers
and experts will be sought. This series will help shape and define
academic and professional programs in this area. This series is intended
for a wide audience, those professionally involved in neural network
research, such as lecturers and primary investigators in neural
computing, neural modeling, neural learning, neural memory, and
neurocomputers. The initial introductory volumes will draw papers from a
broad area of topics, while later volumes will focus upon more specific
topics.
Authors are invited to submit an abstract, extended summary, or
manuscripts describing the recent progress in theoretical analysis,
modeling, design, or application developments in the area of neural
networks. The manuscripts should be self contained and of a tutorial
nature. Suggested topics include, but are not limited to:
* Neural modeling: physiologically based and synthetic
* Neural learning: supervised, unsupervised
* Neural networks: connectionist, random, goal seeking
* Neural and associative memory
* Neurocomputers: electronic implementation
* Self organization and control: adaptive systems
* Cognitive information processing: parallel and distributed
* Mathematical modeling
* Fuzzy set theory
* Vision: neural image processing
* Speech: natural language understanding
* Pattern recognition
* Robotics control
Ablex and the progress Series editor invite you to submit an abstract,
extended summary, or manuscript proposal or abstracts for consideration.
Please contact the series editor directly.
ABSTRACT DEADLINE : For Third Volume is March 30th, 1990.
***You may use fax or email to send your abstract***
Dr. Omid M. Omidvar, Associate Professor
Progress Series Editor
University of the District of Columbia
Computer Science Department, MB4204
4200 Connecticut Avenue, N.W.
Washington, D.C. 20008
Tel:(202)282-7345, Fax:(202)282-3677
Email: OOMIDVAR@UDCVAX.BITNET
------------------------------
Subject: Conference on Intelligent Control
From: KOKAR@northeastern.edu
Date: Fri, 09 Feb 90 14:58:00 -0500
The 5-th IEEE International Symposium
on Intelligent Control
Penn Tower Hotel, Philadelphia
September 5 - 7, 1990
Sponsored by IEEE Control Society
The IEEE International Symposium on Intelligent Control is the Annual
Meeting dedicated to the problems of Control Systems associated with
combined Control/Artificial Intelligence theoretical paradigm.
This particular meeting is dedicated to the
Perception - Representation - Action
Triad.
The Symposium will consist of three mini-conferences:
Perception as a Source of Knowledge for Control (Chair - H.Wechsler)
Knowledge as a Core of Perception-Control Activities (Chair - S.Navathe)
Decision and Control via Perception and Knowledge (Chair - H.Kwatny)
intersected by
Three Plenary 2-hour Panel Discussions:
I. On Perception in the Loop
II. On Action in the Loop
III. On Knowledge Representation in the Loop.
Suggested Topics of Papers are not limited to the following list:
- - Intractable Control Problems in the Perception-Representation-Action Loop
- - Control with Perception Driven Representation
- - Multiple Modalities of Perception, and Their Use for Control
- - Control of Movements Required by Perception
- - Control of Systems with Complicated Dynamics
- - Intelligent Control for Interpretation in Biology and Psychology
- - Actively Building-up Representation Systems
- - Identification and Estimation of Complex Events in Unstructured Environment
- - Explanatory Procedures for Constructing Representations
- - Perception for Control of Goals, Subgoals, Tasks, Assignments
- - Mobility and Manipulation
- - Reconfigurable Systems
- - Intelligent Control of Power Systems
- - Intelligent Control in Automated Manufacturing
- - Perception Driven Actuation
- - Representations for Intelligent Controllers (geometry, physics, processes)
- - Robust Estimation in Intelligent Control
- - Decision Making Under Uncertainty
- - Discrete Event Systems
- - Computer-Aided Design of Intelligent Controllers
- - Dealing with Unstructured Environment
- - Learning and Adaptive Control Systems
- - Autonomous Systems
- - Intelligent Material Processing: Perception Based Reasoning
D E A D L I N E S
Extended abstracts (5 - 6 pages) should be submitted to:
H. Kwatny, MEM Drexel University, Philadelphia, PA 19104 - CONTROL AREA
S. Navathe, Comp. Sci., University of Florida, Gainesville, FL 32911 -
KNOWLEDGE REPRESENTATION AREA
H. Wechsler, George Mason University, Fairfax, VA 22030 -
PERCEPTION AREA
NO LATER THAN MARCH 1, 1990.
Papers that are difficult to categorize, and/or related to all of these
areas, as well as proposals for tutorials, invited sessions,
demonstrations, etc., should be submitted to
A. Meystel, ECE, Drexel University, Philadelphia, PA 19104,
(215) 895-2220
before March 1, 1990.
REGISTRATION FEES:
On/before Aug.5, 1990 After Aug.5, 1990
Student $ 50 $ 70
IEEE Member $ 200 $ 220
Other $ 230 $ 275
Cancellation fee: $ 20.
Payment in US dollars only, by check. Payable to: IC 90.
Send check and registration form to: Intelligent Control - 1990,
Department of ECE, Drexel University, Philadelphia, PA 19104.
------------------------------
End of Neuron Digest [Volume 6 Issue 12]
****************************************