Copy Link
Add to Bookmark
Report
Neuron Digest Volume 04 Number 22
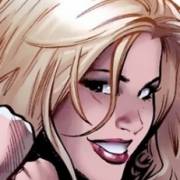
Neuron Digest Sunday, 13 Nov 1988 Volume 4 : Issue 22
Today's Topics:
Seminar: A Connectionst Framework for visual recognition
Reprints avail.
Congress on Cybernetics and Systems
TR available
Tech report available
Paul Thagard to speak on Analogical thinking
Schedule of remaining talks this semester
Tech. Report available
Tech report on connectionist knowledge processing
Technical Report Available
[[Editor's Note: This issue and the next will be devoted to the backlog of
technical talks and papers. Apologies in advance for notice of past talks.
The issue on Consciousness will also have to wait. :-( -PM ]]
Send submissions, questions, address maintenance and requests for old issues to
"neuron-request@hplabs.hp.com" or "{any backbone,uunet}!hplabs!neuron-request"
------------------------------------------------------------
Subject: Seminar: A Connectionst Framework for visual recognition
From: pratt@paul.rutgers.edu (Lorien Y. Pratt)
Date: Tue, 18 Oct 88 09:49:23 -0400
I saw this posted locally, thought you might like to attend. Don't
forget Josh Alspector's talk this Friday (10/21) on his Boltzmann chip!
Rutgers University
CAIP
(Center for Computer Aids for Industiral Productivity. (CAIP)
Seminar:
A Connectionist Framework for Visual Recognition
Ruud Bolle
Exploratory Computer Vision Group
IBM Thomas J. Watson Research Center
Abstract
This talk will focus on the organization and implementation of a vision
system to recognize 3D objects. The visual world being modeled is assumed
to consist of objects that can be represented by planar patches, patches of
quadrics of revolution, and the intersection curves of those quadric
surfaces. A significant portion of man-made objects can be represented
using such prmitives.
One of the contributions of this vision system is that fundamentally
different feature types, like survface and curve descriptions, and
simultaneously extracted and combined to index into a database of objects.
The input to the system is a depth map of a scene comprising of one or more
objects. From the depth map, surface parameters and surface
intersection/object limb parameters are extracted. Parameter extraction is
modeled as a set of layered and concurrent parameter space transforms. Any
one transform computes only a partial geometric description that forms the
input to a next transform. The final transform is a mapping into an object
database, which can be viewed as the highest-level of confidence for
geomeetric descriptoins and 3D objects within the parameter spaces. The
approach is motivated by connectionist model of visual recognition systems.
Date: Friday, November 4, 1988
Time: 3:00 PM
Place: Conference room 139, CAIP center, Busch Campus
For information, call (201) 932-3443
------------------------------
Subject: Reprints avail.
From: gluck@psych.Stanford.EDU (Mark Gluck)
Date: Mon, 24 Oct 88 10:07:57 -0700
Reprints of the following two papers are available by netrequest
to gluck@psych.stanford.edu or by writing: Mark Gluck, Dept. of Psychology,
Jordan Hall; Bldg. 420, Stanford Univ., Stanford, CA 94305.
Gluck, M. A., & Bower, G. H. (1988) From conditioning to category learning:
An adaptive network model. Journal of Experimental Psychology: General,
V. 117, N. 3, 227-247
Abstract
--------
We used adaptive network theory to extend the Rescorla-Wagner (1972)
least mean squares (LMS) model of associative learning to phenomena
of human learning and judgment. In three experiments subjects
learned to categorize hypothetical patients with particular symptom
patterns as having certain diseases. When one disease is far more
likely than another, the model predicts that subjects will sub-
stantially overestimate the diagnosticity of the more valid symptom
for the rare disease. The results of Experiments 1 and 2 provide clear
support for this prediction in contradistinction to predictions from
probability matching, exemplar retrieval, or simple prototype learning
models. Experiment 3 contrasted the adaptive network model with one
predicting pattern-probability matching when patients always had
four symptoms (chosen from four opponent pairs) rather than the
presence or absence of each of four symptoms, as in Experiment 1.
The results again support the Rescorla-Wagner LMS learning rule as
embedded within an adaptive network.
Gluck, M. A., Parker, D. B., & Reifsnider, E. (1988) Some biological
implications of a differential-Hebbian learning rule.
Psychobiology, Vol. 16(3), 298-302
Abstract
--------
Klopf (1988) presents a formal real-time model of classical
conditioning which generates a wide range of behavioral Pavlovian
phenomena. We describe a replication of his simulation results and
summarize some of the strengths and shortcomings of the drive-
reinforcement model as a real-time behavioral model of classical
conditioning. To facilitate further comparison of Klopf's model
with neuronal capabilities, we present a pulse-coded reformulation
of the model that is more stable and easier to compute than the
original, frequency-based model. We then review three ancillary
assumptions to the model's learning algorithm, noting that each
can be seen as dually motivated by both behavioral and biological
considerations.
------------------------------
Subject: Congress on Cybernetics and Systems
From: SPNHC@CUNYVM.CUNY.EDU (Spyros Antoniou)
Organization: The City University of New York - New York, NY
Date: 28 Oct 88 04:15:46 +0000
WORLD ORGANIZATION OF SYSTEMS AND CYBERNETICS
8 T H I N T E R N A T I O N A L C O N G R E S S
O F C Y B E R N E T I C S A N D S Y S T E M S
JUNE 11-15, 1990 at Hunter College, City University of New York, USA
This triennial conference is supported by many international
groups concerned with management, the sciences, computers, and
technology systems.
The 1990 Congress is the eighth in a series, previous events
having been held in London (1969), Oxford (1972), Bucharest (1975),
Amsterdam (1978), Mexico City (1981), Paris (1984) and London (1987).
The Congress will provide a forum for the presentation
and discussion of current research. Several specialized sections
will focus on computer science, artificial intelligence, cognitive
science, biocybernetics, psychocybernetics and sociocybernetics.
Suggestions for other relevant topics are welcome.
Participants who wish to organize a symposium or a section,
are requested to submit a proposal ( sponsor, subject, potential
participants, very short abstracts ) as soon as possible, but not
later than September 1989. All submissions and correspondence
regarding this conference should be addressd to:
Prof. Constantin V. Negoita
Congress Chairman
Department of Computer Science
Hunter College
City University of New York
695 Park Avenue, New York, N.Y. 10021 U.S.A.
=-=-=-=-=-=-=-=-=-=-=-=-=-=-=-=-=-=-=-=-=-=-=-=-=-=-=-=-=-=-=-=-=-=-=
| Spyros D. Antoniou SPNHC@CUNYVM.BITNET SDAHC@HUNTER.BITNET |
| |
| Hunter College of the City University of New York U.S.A. |
=-=-=-=-=-=-=-=-=-=-=-=-=-=-=-=-=-=-=-=-=-=-=-=-=-=-=-=-=-=-=-=-=-=-=
------------------------------
Subject: TR available
From: Mark.Derthick@MCC.COM
Date: Fri, 28 Oct 88 13:27:00 -0500
For copies of my thesis, ask copetas@cs.cmu.edu for CMU-CS-88-182
"Mundane Reasoning by Parallel Constraint Satisfaction."
I am 1200 miles away from the reports, so asking me doesn't do you any
good:
Mark Derthick
MCC
3500 West Balcones Center Drive
Austin, TX 78759
(512)338-3724
Derthick@MCC.COM
If you have previously asked me for this report, it should be arriving
soon. There aren't many extra copies right now, so requests to copetas may
be delayed for a while.
ABSTRACT: Connectionistq networks are well suited to everyday common sense
reasoning. Their ability to simultaneously satisfy multiple soft
constraints allows them to select from conflicting information in finding a
plausible interpretation of a situation. However these networks are poor
at reasoning using the standard semantics of classical logic, based on
truth in all possible models.
This thesis shows that using an alternate semantics, based on truth in a
single most plausible model, there is an elegant mapping from theories
expressed using the syntax of propositional logic onto connectionist
networks. An extension of this mapping to allow for limited use of
quantifiers suffices to build a network from knowledge bases expressed in a
frame language similar to KL-ONE. Although finding optimal models of these
theories is intractable, the networks admit a fast hill climbing search
algorithm that can be tuned to give satisfactory answers in familiar
situations. The Role Shift problem illustrates the potential of this
approach to harmonize conflicting information, using structured distributed
representations. Although this example works well, much remains before
realistic domains are feasible.
------------------------------
Subject: Tech report available
From: Tony Robinson <ajr@DSL.ENG.CAM.AC.UK>
Date: Mon, 31 Oct 88 11:14:50 +0000
Here is the summary of a tech report which demonstates that the error
propagation algorithm is not limited to weighted-sum type nodes, but can
be used to train radial-basis-function type nodes and others. Send me some
email if you would like a copy.
Tony.
`'`'`'`'`'`'`'`'`'`'`'`'`'`'`'`'`'`'`'`'`'`'`'`'`'`'`'`'`'`'`'`'`'`'`'`'`'`'`'
Generalising the Nodes of the Error Propagation Network
CUED/F-INFENG/TR.25
A J Robinson, M Niranjan, F Fallside
Cambridge University Engineering Department
Trumpington Street, Cambridge, England
email: ajr@uk.ac.cam.eng.dsl
1 November 1988
Gradient descent has been used with much success to train connectionist
models in the form of the Error Propagation Network (Rumelhart Hinton and
Williams, 1986). In these nets the output of a node is a non-linear
function of the weighted sum of the activations of other nodes. This type
of node defines a hyper-plane in the input space, but other types of nodes
are possible. For example, the Kanerva Model (Kanerva 1984), the Modified
Kanerva Model (Prager and Fallside 1988), networks of Spherical Graded
Units (Hanson and Burr, 1987), networks of Localised Receptive Fields
(Moody and Darken, 1988) and the method of Radial Basis Functions (Powell,
1985; Broomhead and Lowe 1988) all use nodes which define volumes in the
input space. Niranjan and Fallside (1988) summarise these and compare the
class boundaries formed by this family of networks with feed-forward
networks and nearest neighbour classifiers. This report shows that the
error propagation algorithm can be used to train general types of node.
The example of a gaussian node is given and this is compared with other
connectionist models for the problem of recognition of steady state vowels
from multiple speakers.
------------------------------
Subject: Paul Thagard to speak on Analogical thinking
From: pratt@paul.rutgers.edu (Lorien Y. Pratt)
Date: Mon, 31 Oct 88 12:57:09 -0500
COGNITIVE PSYCHOLOGY FALL COLLOQUIUM SERIES
(Rutgers University)
Date: 9 November 1988
Time: 4:30 PM
Place: Room 307, Psychology Building, Busch Campus
Paul Thagard, Cognitive Science Program, Princeton University
ANALOGICAL THINKING
Analogy is currently a very active area of research in both cognitive
psychology and artificial intelligence. Keith Holyoak and I have developed
connectionist models of analogical retrieval and mapping that are
consistent with the results of psychological experiments. The new models
use localist networks to simultaneously satisfy a set of semantic,
structural, and pragmatic constraints. After providing a general view of
analogical thinking, this talk will describe our model of analog retrieval.
------------------------------
Subject: Schedule of remaining talks this semester
From: pratt@paul.rutgers.edu (Lorien Y. Pratt)
Date: Mon, 31 Oct 88 13:13:18 -0500
Speaker schedule as of 10/31/88 for end of the semester talks in the
Fall, 1988 Neural Networks Colloquium Series at Rutgers.
Speaker Date Title
- ------- ---- -----
Jack Gelfand 11/4/88 Neural nets, Intelligent Machines, and the AI wall
Mark Jones 11/11/88 Knowledge representation in connectionist networks,
including inheritance reasoning and default logic.
E. Tzanakou 11/18/88 ALOPEX: Another optimization method
Stefan Shrier 12/9/88 Abduction Machines for Grammar Discovery
------------------------------
Subject: Tech. Report available
From: Vijaykumar Gullapalli 545-1596 <VIJAYKUMAR@cs.umass.EDU>
Date: Mon, 31 Oct 88 15:57:00 -0400
The following Tech. Report is available. Requests should be sent to
"SMITH@cs.umass.edu".
A Stochastic Algorithm for Learning Real-valued Functions
via Reinforcement Feedback
Vijaykumar Gullapalli
COINS Technical Report 88-91
University of Massachusetts
Amherst, MA 01003
ABSTRACT
Reinforcement learning is the process by which the probability of the
response of a system to a stimulus increases with reward and decreases
with punishment. Most of the research in reinforcement learning (with
the exception of the work in function optimization) has been on
problems with discrete action spaces, in which the learning system
chooses one of a finite number of possible actions. However, many
control problems require the application of continuous control
signals. In this paper, we present a stochastic reinforcement learning
algorithm for learning functions with continuous outputs. Our
algorithm is designed to be implemented as a unit in a connectionist
network.
We assume that the learning system computes its real-valued output as some
function of a random activation generated using the Normal distribution.
The activation at any time depends on the two parameters, the mean and the
standard deviation, used in the Normal distribution, which, in turn, depend
on the current inputs to the unit. Learning takes place by using our
algorithm to adjust these two parameters so as to increase the probability
of producing the optimal real value for each input pattern. The
performance of the algorithm is studied by using it to learn tasks of
varying levels of difficulty. Further, as an example of a potential
application, we present a network incorporating these real-valued units
that learns the inverse kinematic transform of a simulated 3
degree-of-freedom robot arm.
------------------------------
Subject: Tech report on connectionist knowledge processing
From: Charles Dolan <cpd@CS.UCLA.EDU>
Date: Mon, 31 Oct 88 13:28:05 -0800
Implementing a connectionist production
system using tensor products
September, 1988
UCLA-AI-88-15 CU-CS-411-88
Charles P. Dolan Paul Smolensky
AI Center Department of Computer Science &
Hughes Research Labs Institute of Cognitive Science
3011 Malibu Canyon Rd. University of Colorado
Malibu, CA 90265 Boulder, CO 80309-0430
&
UCLA AI Laboratory
Abstract
In this paper we show that the tensor product technique for
constructing variable bindings and for representing symbolic
structure-used by Dolan and Dyer (1987) in parts of a
connectionist story understanding model, and analyzed in general
terms in Smolensky (1987)-can be effectively used to build a
simplified version of Touretzky & Hinton's (1988) Distributed
Connectionist Production System. The new system is called the
Tensor Product Product System (TPPS).
Copyright c 1988 by Charles Dolan & Paul Smolensky.
For copies send a message to
valerie@cs.ucla.edu at UCLA
or
kate@boulder.colorado.edu Boulder
------------------------------
Subject: Technical Report Available
From: Dr Michael G Dyer <dyer@CS.UCLA.EDU>
Date: Tue, 01 Nov 88 11:24:52 -0800
Symbolic NeuroEngineering for Natural Language Processing:
A Multilevel Research Approach.
Michael G. Dyer
Tech. Rep. UCLA-AI-88-14
Abstract:
Natural language processing (NLP) research has been built on the assumption
that natural language tasks, such as comprehension, generation,
argumentation, acquisition, and question answering, are fundamentally
symbolic in nature. Recently, an alternative, subsymbolic paradigm has
arisen, inspired by neural mechanisms and based on parallel processing over
distributed representations. In this paper, the assumptions of these two
paradigms are compared and contrasted, resulting in the observation that
each paradigm possesses strengths exactly where the other is weak, and vice
versa. This observation serves as a strong motivation for synthesis. A
multilevel research approach is proposed, involving the construction of
hybrid models, to achieve the long-term goal of mapping high-level
cognitive function into neural mechanisms and brain architecture.
Four levels of modeling are discussed: knowledge engineering level,
localist connectionist level, distributed processing level, and artificial
neural systems dynamics level. The two major goals of research at each
level are (a) to explore its scope and limits and (b) to find mappings to
the levels above and below it. In this paper the capabilities of several
NLP models, at each level, are described, along with major research
questions remaining to be resolved and major techniques currently being
used in an attempt to complete the mappings. Techniques include: (1)
forming hybrid systems with spreading activation, thresholds and markers to
propagate bindings, (2) using extended back-error propagation in reactive
training environments to eliminate microfeature representations, (3)
transforming weight matrices into patterns of activation to create virtual
semantic networks, (4) using conjunctive codings to implement role
bindings, and (5) employing firing patterns and time-varying action
potential to represent and associate verbal with visual sequences.
(This report to appear in J. Barnden and J. Pollack (Eds.) Advances in
Connectionist and Neural Computation Theory. Ablex Publ. An initial
version of this report was presented at the AAAI & ONR sponsored Workshop
on HIgh-Level Connectionism, held at New Mexico State University, April
9-11, 1988.)
For copies of this tech. rep., please send requests to:
Valerie@CS.UCLA.EDU
or
Valerie Aylett
3532 Boelter Hall
Computer Science Dept.
UCLA, Los Angeles, CA 90024
------------------------------
End of Neurons Digest
*********************