Copy Link
Add to Bookmark
Report
Neuron Digest Volume 05 Number 07
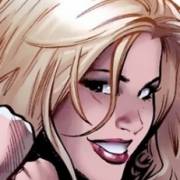
Neuron Digest Friday, 3 Feb 1989 Volume 5 : Issue 7
Today's Topics:
Joint Conference on Neural Networks
new tech report
A WORKSHOP ON CELLULAR AUTOMATA
Genetic Algorithms and Neural Networks
Tech. Report Available
oh boy, more tech reports...
Technical Report: LAIR 89-JP-NIPS
Kolmogorov's superposition theorem
Send submissions, questions, address maintenance and requests for old issues to
"neuron-request@hplabs.hp.com" or "{any backbone,uunet}!hplabs!neuron-request"
ARPANET users can get old issues via ftp from hplpm.hpl.hp.com (15.255.16.205).
------------------------------------------------------------
Subject: Joint Conference on Neural Networks
From: lehr@isl.stanford.EDU (Michael Lehr)
Organization: Stanford University
Date: 06 Jan 89 01:27:31 +0000
[[ Editor's note: The deadline has actually been advanced to 15 February!
-PM]]
An announcement that Bernie Widrow asked me to submit to the digest:
December 29, 1988
Fellow INNS Members:
In accord with the decisions of the INNS Board of Governors and its
Executive Committee, a negotiation with the IEEE has been undertaken on the
subject of joint technical meetings. The INNS and the IEEE have succeeded in
the negotiation! The first joint meeting will be held June 18-22, 1989 at
the Sheraton Washington Hotel. Please see the attached announcement and
call for papers.
In the past, the IEEE has held a series of meetings called ICNN
(International Conference on Neural Networks) and we held our first annual
meeting in Boston and called it the INNS Conference. In the future these
conferences will be combined and called the IJCNN, International Joint
Conference on Neural Networks.
The IEEE was scheduled to have the next ICNN in June 1989 in Washington D.C.
This will superseded by the first joint meeting, IJCNN-89. We were scheduled
to have our next INNS meeting at the Omni Shoreham Hotel in Washington, DC
in September 1989. The conference date has been changed to January 1990 and
this will become the second joint meeting, IJCNN-W'90 (IJCNN-Winter 1990).
A call for papers will go out in about two months.
The need for two international conferences per year in the United States was
clear. During the past summer, both the IEEE San Diego meeting and the INNS
Boston meeting were highly successful. Although their technical programs
were very similar and they were spaced only six weeks apart, both meetings
were attended by about 1700 people. There is a need for these U.S. meetings,
and geographical diversity is the key. We are also planning to have meetings
in Europe and Asia.
The following is a partial list of reasons expressed by various INNS members
on why joint meetings with IEEE are desired:
o Engender a spirit of cooperation among scientists and
engineers in the field.
o Bring together life scientists with technologists for the
betterment of all areas of neural network research.
o Enhance the scope and size of the field, facilitating public
and private support for research and commercialization
efforts.
o Increase the ability of the neural network industry to
exhibit their technical developments and products.
o Avoid scheduling conflicts.
Our agreement with the IEEE calls for a review after the first two meetings.
If we are successful, then we go on together for two more meetings. And so
forth. Once we get over the initial transients, we will try to have winter
meetings on the West Coast and summer meetings on the East Coast. The two
societies will alternate in taking primary responsibility for meeting
organization. The agreement is symmetrical, and both societies are 50-50
partners in the meetings. The IJCNN conferences are for the benefit of both
IEEE and INNS members. Both memberships will enjoy reduced conference fees
at all joint meetings, with students attending at even more reduced rates.
Fellow INNS'ers, I urge you to consider the attached call for papers!
IJCNN-89 serves as our annual meeting for 1989. The deadline on the call for
papers shows February 1, 1989, but has been extended to February 15, 1989
for INNS members. This is the absolute cut-off date. Get your papers into
Nomi Feldman, and incidentally don't forget to renew your INNS membership.
My best wishes to you for the New Year,
Dr. Bernard Widrow, President
INNS
- -------------------------call for papers-------------------------
N E U R A L N E T W O R K S
CALL FOR PAPERS
International Joint Conference on Neural Networks
June 18-22, 1989
Washington, D.C.
The 1989 IEEE/INNS International Joint Conference on Neural Networks
(IJCNN-89) will be held at the Sheraton Washington Hotel in Washington,
D.C., USA from June 18-22, 1989. IJCNN-89 is the first conference in a new
series devoted to the technology and science of neurocomputing and neural
networks in all of their aspects. The series replaces the previous IEEE
ICNN and INNS Annual Meeting series and is jointly sponsored by the IEEE
Technical Activities Board Neural Network Committee and the International
Neural Network Society (INNS). IJCNN-89 will be the only major neural
network meeting of 1989 (IEEE ICNN-89 and the 1989 INNS Annual Meeting have
both been cancelled). Thus, it behoves all members of the neural network
community who have important new results to present to prepare their papers
now and submit them by the IJCNN-89 deadline of 1 FEBRUARY 1989.
To provide maximum value to participants, the full text of those papers
presented orally in the technical sessions will be published in the
Conference Proceedings (along with some particularly outstanding papers from
the Poster Sessions). The title, author name, author affiliation, and
abstract portions of all Poster Session papers not published in full will
also be published in the Proceedings. The Conference Proceedings will be
distributed *at the registration desk* to all regular conference registrants
as well as to all student registrants. The conference will include a day of
tutorials (June 18), the exhibit hall (the neurocomputing industry's primary
annual trade show), plenary talks, and social events. Mark your calendar
today and plan to attend IJCNN-89--the definitive annual progress report on
the neurocomputing revolution!
**DEADLINE for submission of papers for IJCNN-89 is FEBRUARY 1, 1989**
[[Editor's note: I understand this has been extended to 15 Feb 89. -PM]]
Papers of 8 pages or less are solicited in the following areas:
* Real World Applications
* Neural Network Architectures and Theory
* Supervised Learning Theory
* Reinforcement Learning Theory
* Robotics and Control
* Optical Neurocomputers
* Optimization
* Associative Memory
* Image Analysis
* Self-Organization
* Neurobiological Models
* Vision
* Electronic Neurocomputers
Papers should be prepared in standard IEEE Conference Proceedings Format,
and typed on the special forms provided in the Author's Kit. Indicate which
of the above subject areas you wish your paper included in and whether you
wish your paper to be considered for oral presentation at a technical
session, presentation as a poster at a poster session, or both. Papers
submitted for oral presentation may, at the referees' discretion, be
designated for poster presentation instead, if they feel this would be more
appropriate. FULL PAPERS in camera-ready form (1 original on Author's Kit
forms and 5 reduced 8.5" x 11" copies) should be submitted to Nomi Feldman,
Conference Coordinator, at the address below. For more details, or to
request your IEEE Author's Kit, call or write:
Nomi Feldman, IJCNN-89 Conference Coordinator
3770 Tansy Street, San Diego, CA 92121
(619) 453-6222
------------------------------
Subject: new tech report
From: Geoffrey Hinton <hinton@ai.toronto.edu>
Date: Tue, 10 Jan 89 10:09:11 -0500
The following report can be obtained by sending an email request to
carol@ai.toronto.edu If this fails try carol%ai.toronto.edu@relay.cs.net
Please do not send email to me about it.
"Deterministic Boltzmann Learning Performs Steepest Descent in Weight-space."
Geoffrey E. Hinton
Department of Computer Science
University of Toronto
Technical report CRG-TR-89-1
ABSTRACT
The Boltzmann machine learning procedure has been successfully applied in
deterministic networks of analog units that use a mean field approximation
to efficiently simulate a truly stochastic system {Peterson and Anderson,
1987}. This type of ``deterministic Boltzmann machine'' (DBM) learns much
faster than the equivalent ``stochastic Boltzmann machine'' (SBM), but since
the learning procedure for DBM's is only based on an analogy with SBM's,
there is no existing proof that it performs gradient descent in any
function, and it has only been justified by simulations. By using the
appropriate interpretation for the way in which a DBM represents the
probability of an output vector given an input vector, it is shown that the
DBM performs steepest descent in the same function as the original SBM,
except at rare discontinuities. A very simple way of forcing the weights to
become symmetrical is also described, and this makes the DBM more
biologically plausible than back-propagation.
------------------------------
Subject: A WORKSHOP ON CELLULAR AUTOMATA
From: Gerard Vichniac (617)873-2762 <gerard@bbn.com>
Date: Sun, 15 Jan 89 23:56:23 -0500
A workshop on:
************************************************************
Cellular Automata and Modeling of Complex Physical Systems
************************************************************
will be held in les Houches, near Chamonix, France, from February 21 to
March 2, 1989.
The organizers are Roger Bidaux (Saclay), Paul Manneville (Saclay), Yves
Pomeau (Ecole Normale, Paris), and Gerard Vichniac (MIT and BBN).
The topics will include:
- - automata and discrete dynamical systems,
- - lattice-gas automata for fluid dynamics,
- - applications to solid-state physics (in particular, models of growth
and pattern formation),
- - parallel computation in statistical mechanics (in particular, in the
Ising model),
- - dedicated cellular-automata machines.
Workshops at les Houches are traditionally informal, there will be about
five talks a day, and ample time will be left for discussion.
A fee of 3700 FF includes full board lodging at the Physics Center in les
Houches.
Contact:
Paul Manneville, Roger Bidaux or Gerard Vichniac
Bitnet: MANNEV @ FRSAC11 Internet: gerard@alexander.bbn.com
tel.: 33 - 1 69 08 75 35 tel.: (617) 253 5893 (MIT)
fax: 33 - 1 69 08 81 20 (617) 873 2762 (BBN)
telex: 604641 ENERG F
BITNET: MANNEV @ FRSAC11
------------------------------
Subject: Genetic Algorithms and Neural Networks
From: DMONTANA%COOPER@rcca.bbn.com
Date: Mon, 16 Jan 89 20:53:00 -0500
We have written a paper that may be of interest to a number of readers on
both of these mailing lists:
"Training Feedforward Neural Networks Using Genetic Algorithms"
David J. Montana and Lawrence Davis
BBN Systems and Technologies Corp.
70 Fawcett St.
Cambridge, MA 02138
ABSTRACT
Multilayered feedforward neural networks possess a number of properties
which make them particularly suited to complex pattern classification
problems. However, their application to some real-world problems has been
hampered by the lack of a training algorithm which reliably finds a nearly
globally optimal set of weights in a relatively short time. Genetic
algorithms are a class of optimization procedures which are good at
exploring a large and complex space in an intelligent way to find values
close to the global optimum. Hence, they are well suited to the problem of
training feedforward networks. In this paper, we describe a set of
experiments performed on a set of data from a sonar image classification
problem. These experiments both 1) illustrate the improvements gained by
using a genetic algorithm rather than backpropagation and 2) chronicle the
evolution of the performance of the genetic algorithm as we added more and
more domain-specific knowledge into it.
In addition to outperforming backpropagation on the network we were
investigating (which had nodes with sigmoidal transfer function), the
genetic algorithm has the advantage of not requiring the nodes to have
differentiable transfer functions. In particular, it can be applied to a
network with thresholded nodes.
Requests for copies of the paper should be addressed to
dmontana@bbn.com.
Thanks for considering this submission.
Dave Montana
------------------------------
Subject: Tech. Report Available
From: <MUMME%IDCSVAX.BITNET@CUNYVM.CUNY.EDU>
Date: Tue, 17 Jan 89 20:22:00 -0800
The following tech. report is available from the University of Illinois
Dept. of Computer Science:
UIUCDCS-R-88-1485
STORAGE CAPACITY OF THE LINEAR ASSOCIATOR: BEGINNINGS OF A THEORY
OF COMPUTATIONAL MEMORY
by
Dean C. Mumme
May, 1988
ABSTRACT
This thesis presents a characterization of a simple connectionist-system,
the linear-associator, as both a memory and a classifier. Toward this end,
a theory of memory based on information-theory is devised. The principles
of the information-theory of memory are then used in conjunction with the
dynamics of the linear-associator to discern its storage capacity and
classification capabilities as they scale with system size. To determine
storage capacity, a set of M vector-pairs called "items" are stored in an
associator with N connection-weights. The number of bits of information
stored by the system is then determined to be about (N/2)logM. The maximum
number of items storable is found to be half the number of weights so that
the information capacity of the system is quantified to be (N/2)logN.
Classification capability is determined by allowing vectors not stored by
the associator to appear its input. Conditions necessary for the associator
to make a correct response are derived from constraints of information theory
and the geometry of the space of input-vectors. Results include derivation of
the information-throughput of the associator, the amount of information that
that must be present in an input-vector and the number of vectors that can
be classified by an associator of a given size with a given storage load.
Figures of merit are obtained that allow comparison of capabilities of
general memory/classifier systems. For an associator with a simple
non-linarity on its output, the merit figures are evaluated and shown to be
suboptimal. Constant attention is devoted to relative parameter size
required to obtain the derived performance characteristics. Large systems
are shown to perform nearest the optimum performance limits and suggestions
are made concerning system architecture needed for best results. Finally,
avenues for extension of the theory to more general systems are indicated.
This tech. report is essentially my Ph.D. thesis completed last May and can
be obtained by sending e-mail to:
erna@a.cs.uiuc.edu
Please do not send requests to me since I now live in Idaho and don't have
access to the tech. reports.
Comments, questions and suggestions about the work can be sent directly to
me at the address below.
Thank You!
Dean C. Mumme bitnet: mumme@idcsvax
Dept. of Computer Science
University of Idaho
Moscow, ID 83843
------------------------------
Subject: oh boy, more tech reports...
From: Michael C. Mozer <mozer%neuron@boulder.Colorado.EDU>
Date: Wed, 18 Jan 89 14:19:46 -0700
Please e-mail requests to "kate@boulder.colorado.edu".
Skeletonization: A Technique for Trimming the Fat
from a Network via Relevance Assessment
Michael C. Mozer
Paul Smolensky
University of Colorado
Department of Computer Science
Tech Report # CU-CS-421-89
This paper proposes a means of using the knowledge in a network to deter-
mine the functionality or _relevance_ of individual units, both for the
purpose of understanding the network's behavior and improving its perfor-
mance. The basic idea is to iteratively train the network to a certain
performance criterion, compute a measure of relevance that identifies which
input or hidden units are most critical to performance, and automatically
trim the least relevant units. This _skeletonization_ technique can be
used to simplify networks by eliminating units that convey redundant infor-
mation; to improve learning performance by first learning with spare hidden
units and then trimming the unnecessary ones away, thereby constraining
generalization; and to understand the behavior of networks in terms of
minimal "rules."
[An abridged version of this TR will appear in NIPS proceedings.]
- ---------------------------------------------------------------------------
And while I'm at it, some other recent junk, I mean stuff...
A Focused Back-Propagation Algorithm
for Temporal Pattern Recognition
Michael C. Mozer
University of Toronto
Connectionist Research Group
Tech Report # CRG-TR-88-3
Time is at the heart of many pattern recognition tasks, e.g., speech recog-
nition. However, connectionist learning algorithms to date are not well-
suited for dealing with time-varying input patterns. This paper introduces
a specialized connectionist architecture and corresponding specialization
of the back-propagation learning algorithm that operates efficiently on
temporal sequences. The key feature of the architecture is a layer of
self-connected hidden units that integrate their current value with the new
input at each time step to construct a static representation of the tem-
poral input sequence. This architecture avoids two deficiencies found in
other models of sequence recognition: first, it reduces the difficulty of
temporal credit assignment by focusing the back propagated error signal;
second, it eliminates the need for a buffer to hold the input sequence
and/or intermediate activity levels. The latter property is due to the
fact that during the forward (activation) phase, incremental activity
_traces_ can be locally computed that hold all information necessary for
back propagation in time. It is argued that this architecture should scale
better than conventional recurrent architectures with respect to sequence
length. The architecture has been used to implement a temporal version of
Rumelhart and McClelland's verb past-tense model. The hidden units learn
to behave something like Rumelhart and McClelland's "Wickelphones," a rich
and flexible representation of temporal information.
- ---------------------------------------------------------------------------
A Connectionist Model of Selective Attention in Visual Perception
Michael C. Mozer
University of Toronto
Connectionist Research Group
Tech Report # CRG-TR-88-4
This paper describes a model of selective attention that is part of a con-
nectionist object recognition system called MORSEL. MORSEL is capable of
identifying multiple objects presented simultaneously on its "retina," but
because of capacity limitations, MORSEL requires attention to prevent it
from trying to do too much at once. Attentional selection is performed by
a network of simple computing units that constructs a variable-diameter
"spotlight" on the retina, allowing sensory information within the
spotlight to be preferentially processed. Simulations of the model demon-
strate that attention is more critical for less familiar items and that at-
tention can be used to reduce inter-item crosstalk. The model suggests
four distinct roles of attention in visual information processing, as well
as a novel view of attentional selection that has characteristics of both
early and late selection theories.
------------------------------
Subject: Technical Report: LAIR 89-JP-NIPS
From: Jordan B. Pollack <pollack@cis.ohio-state.edu>
Date: Fri, 20 Jan 89 15:40:09 -0500
Preprint of a NIPS paper is now available.
Request LAIR 89-JP-NIPS From:
Randy Miller
CIS Dept/Ohio State University
2036 Neil Ave
Columbus, OH 43210
or respond to this message.
- ------------------------------------------------------------------------------
IMPLICATIONS OF RECURSIVE DISTRIBUTED REPRESENTATIONS
Jordan B. Pollack
Laboratory for AI Research
Ohio State University
Columbus, OH 43210
I will describe my recent results on the automatic development of
fixed-width recursive distributed representations of variable-sized
hierarchal data structures. One implication of this work is that certain
types of AI-style data-structures can now be represented in fixed-width
analog vectors. Simple inferences can be performed using the type of
pattern associations that neural networks excel at. Another implication
arises from noting that these representations become self-similar in the
limit. Once this door to chaos is opened, many interesting new questions
about the representational basis of intelligence emerge, and can (and will)
be discussed.
------------------------------
Subject: Kolmogorov's superposition theorem
From: sontag@fermat.rutgers.edu
Date: Tue, 17 Jan 89 14:08:03 -0500
*** I am posting this for Professor Rui de Figuereido, a researcher in Control
Theory and Circuits who does not subscribe to this list. Please direct
cc's of all responses to his e-mail address (see below).
-eduardo s. ***
KOLMOGOROV'S SUPERPOSITION THEOREM AND ARTIFICIAL NEURAL NETWORKS
Rui J. P. de Figueiredo
Dept. of Electrical and Computer Engineering
Rice University, Houston, TX 77251-1892
e-mail: rui@zeta.rice.edu
The implementation of the Kolmogorov-Arnold-Sprecher Superposition Theorem
[1-3] in terms of artificial neural networks was first presented and fully
discussed by me in 1980 [4]. I also discussed, then [4], applications of
these structures to statistical pattern recognition and image and multi-
dimensional signal processing. However, I did not use the words "neural
networks" in defining the underlying networks. For this reason, the current
researchers on neural nets including Robert Hecht-Nielsen [5] do not seem to
be aware of my contribution [4]. I hope that this note will help correct
history.
Incidentally, there is a misprint in [4]. In [4], please insert "no" in
the statement before eqn.(4). That statement should read: "Sprecher showed
that lambda can be any nonzero number which satisfies no equation ..."
[1] A.K.Kolmogorov, "On the representation of continuous functions of several
variables by superposition of continuous functions of one variable and
addition," Dokl.Akad.Nauk.SSSR,Vol.114,pp.369-373,1957.
[2] V.I.Arnol'd, "On functions of three variables," Dokl.Akad.Nauk.SSSR,
Vol.114,pp.953-956,1957.
[3] D.A.Sprecher, "An improvement in the superposition theorem of Kolmogorov,"
J.Math.Anal.Appl.,Vol.38,pp.208-213,1972.
[4] Rui J.P.de Figueiredo, "Implications and applications of Kolmogorov's
superposition theorem,"IEEE Trans.Auto.Contr.,Vol.AC-25,pp.1227-1231,1980.
[5] R.Hecht-Nielsen, "Kolmogorov's mapping neural network existence theorem,"
IEEE 1st Int.Conf.on Neural Networks, San Diego,CA,June 21-24,1987,paper
III-11.
------------------------------
End of Neurons Digest
*********************