Copy Link
Add to Bookmark
Report
A back-propagation network for Atari ST
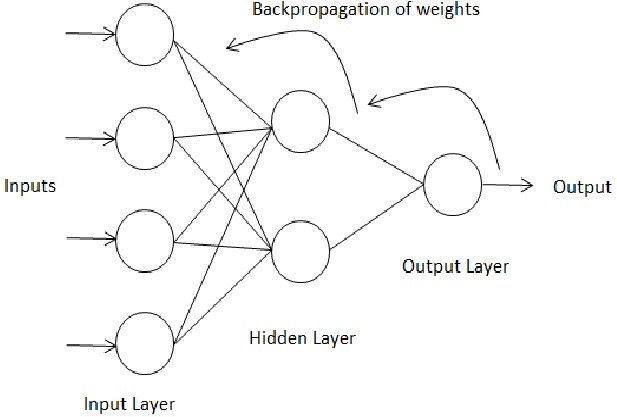
BACKPROP.C
#include <stdio.h>
#include <nn_decl.h>
/*******************************************************************************
*
* 1992.04.13
*
* A back-propagation network.
*
* This is a slightly modified version of the network found in Dr. Dobb's
* Journal, January 1989. Page 96.
*
* The modifications are:
*
* - The definition of the network is held in the external nn_decl.h instead
* of in the sourc code. The reason for this is that I am currently working
* with a tool to create network configurations.
*
* - The sigmoid is modified.
*
*
* Further extensions/modifications to be made soon:
*
* - Reading/saving networks and training sets to disk.
*
* - Graphical network displays.
*
*
* This code is compiled using the Sozobon C.
*
* Are you interested in neural networks? Do you know of any neural
* network software for the ST? Then write.
*
* Anders Oestlund at Synchron City (or at the most of the of the ST BBS's
* in Sweden.
*
*
*******************************************************************************/
double sigmoid();
double RRand();
void RandWts();
void Recall();
double Learn();
double fabs();
double fabs( x )
double x;
{
return (x >= 0.0) ? x : (-1.0 * x);
}
/*******************************************************************************
*
* Compute the sigmoid of a value
*
*******************************************************************************/
double sigmoid( x )
register double x;
{
double r; /* the result */
if (x>0.0)
{
x = (x + 1) * (x + 1) * (x + 1);
r = 1.0 - (0.5 / x);
}
else
{
x = -1.0 * x;
x = (x + 1) * (x + 1) * (x + 1);
r = (0.5 / x);
}
return r;
}
/*******************************************************************************
*
* Compute a random in a range
*
*******************************************************************************/
double RRand( low, high )
double low, high; /* low-high limits */
{
double r; /* result */
r = low + (rand() / (double)RAND_MAX) * (high - low);
return( r );
}
/*******************************************************************************
*
* RandWts, randomize all of the weights in the network
*
*******************************************************************************/
void RandWts( low, high, LLp )
double low, high; /* limits for random */
PE ***LLp; /* layer list pointer */
{
PE **PePP; /* PE pointer */
PE *PeP; /* PE itself */
CONN *WtP; /* Connection pointer */
for ( ; (PePP = *LLp) != (PE **)0; LLp++ ) /* layer loop */
{
printf("Layer %ld\n", PePP );
for ( ; (PeP = *PePP) != (PE *)0; PePP++) /* PE loop */
{
printf(" PE %ld:\n", PeP);
for ( WtP = &PeP->Conns[0]; WtP->PESource != 0; WtP++ ) /* conn loop */
{
WtP->ConnWt = RRand( low, high);
WtP->LastDelta = 0.0;
printf(" %f <= [%d\n", WtP->ConnWt, WtP->PESource );
}
}
}
}
/*******************************************************************************
*
* Recall, recall information from the network.
*
*******************************************************************************/
void Recall( ov, iv, LLp, rcf )
double *ov; /* output vector */
double *iv; /* input vector */
PE ***LLp; /* layer list pointer */
int rcf; /* recall flag */
{
PE **PePP; /* PE pointer */
PE **LastPP; /* Last non-zero PE list pointer */
PE *PeP; /* PE itself */
CONN *WtP; /* connection pointer */
double sum; /* weighted sum */
/* Copy input vector to input of network */
for ( PePP = *LLp++; (PeP = *PePP) != (PE *)0; PePP++)
{
PeP->Output = *iv++;
}
/* compute the weighted sum and transform it */
for ( ; (PePP = *LLp) != (PE **)0; LLp++ ) /* Layer loop */
{
LastPP = PePP; /* Save last non-zero layer */
for ( ; (PeP = *PePP) != (PE *)0; PePP++) /* for all PE's in a layer */
{
/* weighted sum of the inputs */
sum = 0;
for ( WtP = &PeP->Conns[0]; WtP->PESource != 0; WtP++)
{
sum += WtP->ConnWt * PEList[ WtP->PESource ]->Output;
}
/* sigmoid transform */
PeP->Output = sigmoid( sum );
/* if learn mode then set error to zero */
if ( rcf == 0 ) PeP->Error = 0.0;
}
}
/* if not in learn-mode, copy the result to the output array */
if ( rcf != 0 )
{
for ( ; (PeP = *LastPP) != (PE *)0; LastPP++ )
{
*ov++ = PeP->Output;
}
}
}
/*******************************************************************************
*
* Learn, learn an association.
*
*******************************************************************************/
double Learn( ov, iv, LLp, alpha, eta )
double *ov; /* output vector */
double *iv; /* onput vector */
PE ***LLp; /* layer list pointer */
double alpha; /* learning rate */
double eta; /* momentum */
{
double MAErr; /* Maximum output error */
double rv; /* work value */
double SigErr; /* back-propagated error */
PE ***ALp; /* alternate layer pointer */
PE **PePP; /* PE pointer */
PE **LastPP; /* last non-zero PE-list pointer */
PE *PeP; /* PE itself */
CONN *WtP; /* connection pointer */
/*
printf("Learn... ");
*/
Recall( ov, iv, LLp, 0 ); /* perform a recall */
/* Find the output layer */
for ( ALp = LLp; ALp[1] != (PE **)0; ALp++ )
;
/* Compute the square error in the output vector */
for (MAErr = 0.0, PePP = *ALp; (PeP = *PePP) != (PE *)0; PePP++)
{
rv = (*ov++) - PeP->Output; /* output error */
PeP->Error = rv;
if ( fabs(rv) > MAErr ) MAErr = fabs(rv);
}
/*
printf("MaxE=%f ", MAErr );
*/
/* back-propagate the error and update the weights */
for ( ; ALp > LLp; ALp-- ) /* layer loop */
{
PePP = *ALp;
for ( ; (PeP = *PePP) != (PE *)0; PePP++ ) /* PE's in a layer */
{
/* commute the error prior to the sigmoid function */
SigErr = PeP->Output * (1.0 - PeP->Output) * PeP->Error;
/* back-propagate the errors & adjust weights */
for ( WtP = &PeP->Conns[0]; WtP->PESource != 0; WtP++)
{
PEList[ WtP->PESource ]->Error += WtP->ConnWt * SigErr;
rv = alpha * PEList[ WtP->PESource ]->Output * SigErr +
eta * WtP->LastDelta;
WtP->ConnWt += rv;
WtP->LastDelta = rv;
/*
printf("~");
*/
}
}
}
/*
printf("\n");
*/
return MAErr;
}
/* Training data for the XOR problem */
static double iv1[] = { -1.0, -1.0 }; static double ov1[] = { 0.0 };
static double iv2[] = { 1.0, -1.0 }; static double ov2[] = { 1.0 };
static double iv3[] = { -1.0, 1.0 }; static double ov3[] = { 1.0 };
static double iv4[] = { 1.0, 1.0 }; static double ov4[] = { 0.0 };
static double *ivp[] = { &iv1[0], &iv2[0], &iv3[0], &iv4[0] };
static double *ovp[] = { &ov1[0], &ov2[0], &ov3[0], &ov4[0] };
main ()
{
long wx; /* work index */
long x; /* index into samples array */
double r; /* work value */
double MAErr; /* maximum abs error */
double wo[ sizeof(ivp)/sizeof(*ivp) ];
/* randomize the weights in the network */
RandWts( -1.0, 1.0, &LayList[0] );
MAErr = 100.0;
for ( wx = 0; ; wx++ )
{
x = wx % (sizeof(ivp)/sizeof(*ivp));
if ( (x == 0) && (wx != 0) )
{
if ( (wx % 100) == 0 )
{
printf("Pres %ld, Max abs err = %f\n", wx, MAErr);
}
if (MAErr <= 0.05) break;
MAErr = 0.0;
}
r = Learn( ovp[x], ivp[x], &LayList[0], 0.9, 0.5 );
if (r > MAErr) MAErr = r;
}
/* Test the network */
printf("\n\nTraining complete\n");
for ( wx = 0; wx < (sizeof(ivp)/sizeof(*ivp)); wx++ )
{
Recall(wo, ivp[wx], &LayList[0], 1);
printf("Input %f : %f => %f\n", ivp[wx][0], ivp[wx][1], wo[0] );
}
}
NN_DECL.H
#define MAXCONN 4
/*****************************************************/
/*****************************************************/
/*****************************************************/
typedef struct _conn { /* conn to PE */
int PESource; /* index to a PE source */
float ConnWt; /* connection weight */
float LastDelta; /* last weight change */
} CONN;
typedef struct _pe { /* processing element */
float Output; /* PE output */
float Error; /* Accumulated error */
CONN Conns[MAXCONN+1]; /* connections */
} PE;
/*** PE 1 in layer "inp" (Bias) ***/
static PE pe1 = {0.0, 0.0, /* default output & error */
0,0,0 };
/*** PE 2 in layer "inp" ***/
static PE pe2 = {0.0, 0.0, /* default output & error */
0,0,0 };
/*** PE 3 in layer "inp" ***/
static PE pe3 = {0.0, 0.0, /* default output & error */
0,0,0 };
/*** PE 4 in layer "hidd" ***/
static PE pe4 = {0.0, 0.0, /* default output & error */
1,0,0,
2,0,0,
3,0,0,
0,0,0 };
/*** PE 5 in layer "out" ***/
static PE pe5 = {0.0, 0.0, /* default output & error */
1,0,0,
2,0,0,
3,0,0,
4,0,0,
0,0,0 };
/* PE list. For reference by number/index */
static PE *PEList[] = { (PE *)0,
&pe2,
&pe3,
&pe4,
&pe5,
(PE *)0 };
/* Layer definitions */
static PE *Layinp[] = {
&pe1,
&pe2,
&pe3,
(PE *)0 };
static PE *Layhidd[] = {
&pe4,
(PE *)0 };
static PE *Layout[] = {
&pe5,
(PE *)0 };
/* Network list */
static PE **LayList[] = {
&Layinp[0],
&Layhidd[0],
&Layout[0],
(PE **)0 };